Chapter 9: Non-Experimental Design I (Corr & Survey) PDF
Document Details
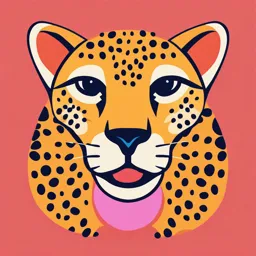
Uploaded by EloquentMossAgate6040
York University
Tags
Summary
This chapter explores the correlational method of research, which examines relationships between variables without manipulating them. It explains how scatterplots represent correlational data and how correlation coefficients indicate the strength and direction of these relationships. The use of the Pearson r coefficient and its interpretation in psychology are also discussed.
Full Transcript
NON-EXPERIMENTAL DESIGNS I: SURVEY METHOD – CHAPTER 9 (but FOCUSING ON the CORRELATIONAL METHOD FIRST) IMPORTANT: in this edition of the text, correlations are discussed as a way of analyzing data collected in surveys. It is true that correlations can be used as a statistical technique, but over an...
NON-EXPERIMENTAL DESIGNS I: SURVEY METHOD – CHAPTER 9 (but FOCUSING ON the CORRELATIONAL METHOD FIRST) IMPORTANT: in this edition of the text, correlations are discussed as a way of analyzing data collected in surveys. It is true that correlations can be used as a statistical technique, but over and above that, correlations represent a method of research – the correlational method of research. I’ll be discussing correlations as a method of research. CORRELATIONAL METHOD OF RESEARCH With the experiment, the researcher manipulates variables, tries to control other variables, and randomly assigns participants to the different groups (control and experimental groups). With the correlational method, however, variables are not manipulated or controlled. Rather, any naturally-occurring changes in the 2 variables of interest are measured. In other words, you’re not imposing anything, but rather, are simply measuring 2 variables as they are naturally occurring or existing, and seeing whether there is a relationship between them. “Correlation” means “the relationship between two things.” Correlations can help us predict one variable from another. A scatterplot is a type of graph that represents correlational data. Each dot in the scatterplot represents the value of two variables for that given person. When the dots are haphazardly scattered (no pattern), that is indicative of little or no relationship between both variables. If the dots follow a straight line pattern, there is a very strong relationship between the variables. The stronger the correlation, the more closely the dots follow a linear pattern, and the better we can predict the value of one variable from the other variable. 1 HIGH CORRELATION 2 A scatterplot, however, just gives you a general idea of how both variables are related. You want to be able to determine the exact correlation, and this is where the correlation coefficient formula comes into play. The value of each person’s 2 variables is put into this formula, and you end up with a number that tells you exactly how both variables are related. For the purpose of this course, I don’t expect you to calculate anything but you should know how to interpret the correlation coefficient (r). The correlation coefficient “r” consists of a + (plus) or – (minus) sign and a number. Ex. r = +.73 -The number tells you how strongly the 2 variables are correlated (related) and the + or – sign tells you the direction of their relationship. The number can range from 0 to 1.00, where 0 indicates no relationship (and no predictability), and 1.00 indicates a perfect relationship (and perfect predictability). (It’s impossible to get a value greater than 1.00). Coefficients will typically fall somewhere between 0 and 1.00, and a coefficient of.50 is indicative of a moderate relationship. The closer the coefficient is to 1.00, the stronger the relationship between the 2 variables, and the greater the predictability. (In Psychology, a coefficient of.7 is considered high). The + or – sign tells you something else about the direction of the relationship and it must be interpreted separately from the number. If the coefficient has a + sign, this means that the values of both variables are changing in the same direction. As the value of one variable increases, so does the value of the other variable. Another way of saying this is that as the value of one variable decreases so does the value of the other variable. In other words, high scores on one variable are associated with corresponding high scores on the other variable. If the coefficient has a – sign, this means that the variables are changing in the opposite direction. As the value of one variable increases, the value of the other variable decreases (or vice versa). In other words, high scores on one variable are associated with corresponding low scores on the other variable. 3 Example: if you found that the correlation between being bullied and self-esteem was r = -.29, the minus sign tells you that as bullying increases, self- esteem decreases (or that as bullying decreases self-esteem increases) and this numerical value tells you that the relationship between both variables is weak. There are different types of correlation coefficients and the “Pearson r” is the most common one (it’s used for ratio and interval scales). You must still perform inferential statistics to determine whether the correlation coefficient is statistically significant. Goodwin and Goodwin, 2017 Linearity Some relationships aren’t linear, and this would probably just be obvious if you looked at the scatterplot. In this case, using “Pearson r” wouldn’t be appropriate and there are other stats used for curvilinear relationships. 4 Restriction of Range – when only a narrow range of scores is used for one or both variables and this misrepresents the correlation. In correlational research it’s essential that you have a large sample so that it includes people with a wide range of scores. Restriction of range also reduces predictability. Goodwin and Goodwin, 2017 5 REGRESSION ANALYSIS I said earlier that correlations allow you to make predictions and making predictions on the basis of correlational research is called regression analysis. Knowing the size of the correlation, and the value of X (predictor variable) allows you to predict Y (criterion variable). The regression line (the “line of best fit”) provides the best way of summarizing the points on the scatterplot and is the line used for making predictions. Goodwin and Goodwin, 2017 Bivariate Analysis examines the relationship between 2 variables. Multivariate Analysis examines the relationship between 3 or more variables. Regression Analysis is bivariate (you predict Y from X). Multiple Regression Analysis is an example of a multivariate analysis because it involves 2 or more predictor variables and a criterion variable. This enables you to determine if each X variable can predict the Y variable, and it allows you to determine the relative strengths of these predictions (taken independently or jointly). Prediction is usually greater when more than one predictor variable is used. 6 OUTLIERS An outlier is a score that’s extremely different from the others in the data set and can seriously distort “r”. The best way to spot an outlier is to examine the scatterplot. In Goodwin & Goodwin, 2017 When $500 was included in the calculation, r = +.39 but when it was omitted, r = -.14 (a more accurate account). Including the outlier gives the false impression. COEFFICIENT of DETERMINATION Measures the proportion (percentage) of variability in one variable that can be determined from its relationship with the other variable and can be calculated with r 2 (just square the correlation coefficient). Ex – suppose the correlation between study time and grade was: r = +.60 r 2 =.36 or 36% (coefficient of determination) This means that of all of the things that could be affecting grade, study time accounts for or can explain 36% of it.(We don’t know what the other 64% is.) Given that r is squared, the coefficient of determination will always be a positive number and will always be a smaller number then r. INTERPRETATIONAL PROBLEMS WITH CORRELATIONS Unlike in an experiment, you CANNOT INFER CAUSATION because you don’t manipulate and control variables. A) Directionality Problem – variables might be correlated but you don’t know which variable is the cause and which is the effect. Example: if there’s a correlation between the amount of time you exercise and your well-being, you don’t know if it’s the exercise that’s causing your well- 7 being, or whether it’s your well-being that’s making you more likely to exercise. There’s a correlation between watching violent TV and aggression in kids but does watching violent TV cause aggression or do already-aggressive kids choose to watch violent TV? Researchers are OK with attributing causality between X and Y when X precedes Y in time. Cross-Lagged Panel Correlation – is a technique that can help determine which variable is likely the cause and the effect. If X and Y are measured at two different points in time, and if X precedes Y, then X might cause Y, but Y can’t cause X. It’s a type of longitudinal design. The important comparisons are the diagonal correlations. A correlation of.31 is greater than.01 so that’s the one to focus on. What are the two variables associated with r = +.31 and which one occurred first? Watching violent TV in Gr 3 came before aggression in Gr 13, so watching violent TV is likely the causal variable. Goodwin and Goodwin, 2017 8 b) Third Variable Problem This refers to drawing causal conclusions with correlations. A third variable is any uncontrolled variable that could underlie and be responsible for a correlation between X and Y. Possible Causal Relationships if Low Self-Esteem and Depression Are Correlated Example: suppose there’s a correlation between the number of churches and the number of crimes. Crime could cause people to become religious or religion could be the cause of certain types of crimes. But this apparent correlation could be due to a 3 rd underlying variable: size of city. If a city is large, there will likely be more churches and more crime. 9 TWO TYPES OF THIRD VARIABLES: A) Mediator variable: explains how or why the relationship between two variables exist (ie, X leads to the mediator, which in turn leads to Y) B) Moderator variable: explains under what conditions the relationship between the two variables exist (ie, the moderator cause both X and Y) Goodwin and Goodwin, 2017 10 If you suspect that a 3rd variable might be responsible for an apparent correlation, you can measure the effect of a 3rd variable by performing a partial correlation. PARTIAL CORRELATION -provides a way of statistically controlling a potential third variable. It basically statistically teases out the contribution of a potential third variable, from the correlation between the two original variables of interest. If the correlation between X and Y remains the same after a 3rd variable has been “partialled out” (teased out), then it can be eliminated as a 3rd variable. Example: There’s a correlation of +.60 between your calculus and stats grade but you suspect that “I.Q” might be acting as a third variable that’s responsible for the apparent correlation between these two grades. First, determine whether IQ is actually correlated to each your calculus grade and stats grade. If it is, then proceed with the partial correlation analysis. In other words, statistically tease out the contribution of IQ to these grades. If the correlation between these two grades changes after IQ has been parialled out, then IQ was a 3rd variable. If the correlation remains unchanged, then IQ was not a 3rd variable. 11 NON-EXPERIMENTAL DESIGNS I: SURVEY METHOD – CHAPTER 9 Survey – is a structured set of questions to measure people’s attitudes, beliefs or behaviors (it can be delivered through the mail, on-line, or in a face-to-face or phone interview). SAMPLING PROCEDURES (who to measure). This topic appears in Chapter 4 (8th edition of the text) but it’s related to the survey method so I’ll be discussing it here. TWO TYPES of SAMPING – probability and non-probability A) PROBABILITY SAMPLING - used if you want to learn something about an identifiable group (population) from a sub-group (sample). -the population is the group that you want to generalize to and draw conclusions about. -the sample must reflect (be a “mini-version” of) the population. In order to generalize from your sample to the population, the sample must be representative of the population. If the sample isn’t representative, then it’s “biased”. Example: if you wanted to determine Ont. teenagers’ attitudes toward drug use and sampled only teenagers from the GTA that would be a biased sample because it doesn’t adequately represent the teenagers in other parts of the province. Improper sampling procedures or a self-selection problem can lead to a biased sample. Self-Selection or Nonresponse Bias - when a sample is composed only of those who choose to voluntarily respond to a survey. There may be something peculiar/different about people who choose to respond versus those who don’t, and so you could have a biased sample. -If the return rate is less than 60% you probably have a self-selection problem. Psychologists are usually happy with return rates of 70% or higher. TYPES OF PROBABILITY SAMPLING A) SIMPLE RANDOM SAMPLING – every member of the target population has an equal chance of being selected for the sample. Ex – if you want to survey 50 students out of a class of 450, all 450 would put their name in a hat and you’d pull 50 names (there are more sophisticated ways of doing this!!) Problem: Sometimes there are specific features of the population that you want to be reflected in your sample and simple random assignment won’t accomplish that. Solution: 12 B) STRATIFIED SAMPLING (“strata” means layer; type of probability sampling) -this is where proportions (percentages) of important sub-groups in the population are exactly represented in your sample. Ex Suppose you want to sample York’s psych students’ attitudes toward drug use. Let’s pretend that 2,000 are 20yrs old and 500 are over 40yrs. You need a sample of 100. You have reason to believe that attitude differences between these age groups exist and you want to capture that. Stratified sampling would accomplish this. (Simple random sampling wouldn’t because you could end up with a sample consisting of all young people). Example of stratified sampling: Population Sample 500 old 20 old (randomly selected from the 500) 2,000 young 80 young (randomly selected from the 2,000) Total=2,500 Total = 100 Notice how the proportion in the sample matches that in the population. Problem: Simple random sampling might not be practical if the population is large because you’d have to gather a complete list of everyone in the target population. Solution: C) CLUSTER SAMPLING (type of probability sampling) Here, you start off by randomly selecting a cluster (group) having a common feature. Ex – you want to assess student satisfaction with life in campus high-rises and there are 20 buildings. -randomly select 8 out of the 20 buildings (each building is a cluster) -randomly pick 3 out of 25 floors from each building (floor is a sub-cluster) -randomly pick 10 people out of 50 on each floor The entire population is 20 x 25 x 50 =25,000 (random sampling would be impractical) Your chosen sample: 8 x 3 x 10 =240 (a more practical approach) NON-PROBABILITY SAMPLING (Ss are not randomly chosen) Failure to use probability sampling will be a problem in survey research but not in experimental research because the latter has a different goal (determining how the IV and DV are related). In an experiment, random assignment to groups is required – not random sampling. TYPES OF NON-PROBABILITY SAMPLING: A) Convenience Sampling (most common) – you recruit subjects from a group of available people who meet the general requirements of the study (ex -Intro Psych students partaking in URPP studies). B) Purposive Sampling – a convenience sampling strategy where a specific type of person is recruited. Ex- only bilingual Intro Psych students 13 C) Quota Sampling – you stop recruiting after you get the number of subjects you need in a sub- group. Ex. you need a quota of 50 males and 50 females and you stop recruiting when you reach each number. D) Snowball Sampling (also called referral sampling) – ask a S to recruit some of their friends or co-workers etc TYPES OF SURVEY QUESTIONS Questions can be either open-ended or closed questions. A) Open-ended – answers require written out, descriptive answers. The disadvantage is that it’s difficult to score and Ss are often reluctant to put in the effort to answer the question. B) Closed Questions - rather than requiring “yes”/”no” answers, Ss are usually asked to rate how much they agree/disagree with something. A Likert Scale (interval scale) consisting of either 5 or 7 points is typically used (the middle point is always neutral) Strongly agree Agree Neutral/Undecided Disagree Strongly Disagree 1 2 3 4 5 Considerations When Designing Survey Questions (always do a pilot study to ensure questions are properly interpreted) Don’t use: -double barreled questions -leading questions -ambiguous questions -Avoid negatively phrased sentences -Word some questions favorably and others unfavorably to avoid response acquiescence (tendency to always agree) -Don’t overuse “don’t know” (DK) options -Sequencing of items -if some questions are “sensitive” put them at the end of the survey; put easy and interesting questions first. 14