Experimental Design II: Factorial Designs PDF
Document Details
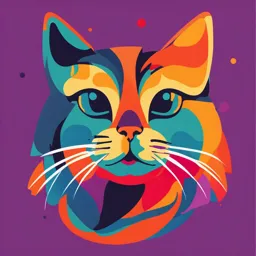
Uploaded by AccomplishedSodium
York University
Tags
Summary
This document describes factorial designs in experimental research. It explains how to identify main effects and interaction effects.
Full Transcript
EXPERIMENTAL DESIGN II: FACTORIAL DESIGNS – CHAPTER 8 (“factor” is synonymous with independent variable (IV); “factorial” implies more than one IV) Factorial Design – involves an experiment with more than one IV (most studies involve 2 to 4 IVs) Factorial Notation A) A factorial is described with a...
EXPERIMENTAL DESIGN II: FACTORIAL DESIGNS – CHAPTER 8 (“factor” is synonymous with independent variable (IV); “factorial” implies more than one IV) Factorial Design – involves an experiment with more than one IV (most studies involve 2 to 4 IVs) Factorial Notation A) A factorial is described with a numbering system that simultaneously identifies the # of IVs and the # of levels of each IV. The # of digits = # of IVs; the value of the digit = the # of levels of that IV. Ex- in a 3 x 5 (3 by 5) factorial there are 2 IVs; one IV has 3 levels and the other IV has 5 levels. - in a 2 x 2 x 4 factorial there are 3 IVs and each IV has 2, 2, and 4 levels, respectively B) the conditions to be tested or compared can be determined by looking at all of the combinations of different levels of each IV. # of conditions to be compared = # of cells in the matrix To determine the # of conditions or cells, simply multiply the numbers in the notation. Ex- 2 x 4 = 8 conditions or cells in the matrix. If you have a 2 x 2 x 4 factorial, there are 16 conditions. Note: In factorial designs, “levels” and “conditions” can no longer be used interchangeably (as they could be in single factor designs). They now refer to different things. 1 ANALYZING EFFECTS WITH FACTORIAL DESIGNS -The inferential statistic to use in a single-factor two level design is a t-test. -The inferential statistic to use in a single factor multilevel design is a one-way analysis of variance (ANOVA), followed by post-hoc tests if the ANOVA is significant. With factorial designs, an ANOVA is performed and within that ANOVA, inferential stats are performed on main effects and interaction effects (discussed below). MAIN EFFECT – refers to the overall effect of one particular IV. The number of main effects = the # of IVs. A 2 x 2 factorial has 2 IVs, and thus, 2 main effects. To determine the main effect of one IV, collapse (combine) the data over all levels of the other factor. TESTING FOR A MAIN EFFECT OF PRESENTATION RATE (2secs vs 4secs) Adapted from Goodwin and Goodwin, 2017 2 TESTING FOR A MAIN EFFECT OF MEMORIZATION TYPE (imagery vs rote memory) Adapted from Goodwin and Goodwin, 2017 INTERACTION EFFECTS – occur when the effect of one IV depends upon the level of another IV. The advantage of factorials over single-factor designs is that it’s possible to detect interactions (which provide more interesting and detailed results). Interaction effects generally take precedence over main effects. EXAMPLE OF AN INTERACTION EFFECT Goodwin and Goodwin, 2017 Which yields higher grades, Science or Humanities? It depends on whether it’s a lab or a lecture course. When our answer is “it depends” it usually means there’s an interaction effect. 3 It’s a good idea to put the data in a matrix because it organizes the data and makes it easier to plot the data in a line graph. (In factorial designs a line graph is always used rather than a bar graph or histogram). Each cell of the matrix is populated with the mean for that given condition. You can get some idea of whether there are main effects by comparing the column and row means, but inferential stats are needed to determine whether these differences are statistically significant. To determine whether an interaction effect is present, you would compare the means within the cells of the matrix, but that can be difficult to “eyeball”. An easier way of “eyeballing” an interaction is to look at the data plotted in a line graph. If the lines are not parallel, then there’s an interaction. Again, inferential stats would be required to determine if the interaction is statistically significant. In the example below there appears to be a main effect of recall strategy (imagery vs no imagery or the control), a main effect of presentation rate (2 vs 4 words/sec), and an interaction effect. Of course, you would have to establish that these are effects statistically significant. To describe the main effects you would say: recall was higher with imagery than with the control, and it was higher with the 4 sec rate than the 2 sec rate. You would describe the interaction by saying that recall was better with the 4 sec rate than with the 2 sec rate, but that advantage was more pronounced for the control than for imagery. Alternatively, you could describe it by saying that recall was better for imagery than for the control but there was less of a difference between the two, at the 4 sec rate than at the 2 sec rate. The interaction is considered to be more important that the main effects because it provides a more detailed account of how these variables are related. Goodwin and Goodwin, 2017 4 If you have a study with 2 IVs (A and B) and want to determine if main and interaction effects are statistically significant, you would perform an ANOVA. An ANOVA is an “umbrella term” for the inferential stats that you perform on each possible effect. Each inferential test is called an “f-test” (as opposed to a t-test). ANOVA (umbrella term that involves a number of f-tests) A (main effect of A f-test) B (main effect of B f-test) AxB (interaction effect f-test) If you had 3 IVs (A, B, C), the effects that you could test for in an ANOVA are: A, B, C, AxB, AxC, BxC, AxBxC. The AxBxC interaction would be considered the most important result if it was found to be significant. It’s possible for all, none, or just some of the effects to be significant. If a study has more than one dependent variable, you would have to draw a separate graph for each dependent variable. CEILING EFFECT When you look at a graph, it can sometimes look as though there is an interaction, when in fact it’s really a “ceiling effect” that’s happening. This occurs when the means for 2 or more conditions are near the maximum, and it gives the impression that no difference exists between these conditions. The means are so close to the maximum performance that there isn’t enough “room” for an increase to be demonstrated. (A “floor effect” is just the opposite). Goodwin and Goodwin, 2017 5 VARIETIES OF FACTORIAL DESIGNS In addition to the 4 designs that we already identified for a single-factor design (independent groups, matched groups, ex post facto, repeated measures), factorials have 2 additional types of designs: Mixed Factorials and PxE Factorials. MIXED FACTORIAL DESIGN Factorial designs can be completely between-subject (all IVs are between-subject factors; each condition has a different set of Ss ), or completely within-subject (all IVs are within-subject factors; the same set of Ss is tested across all conditions). 6 The above should read “Different Ss in each condition” 7 In a MIXED FACTORIAL, at least one IV is between-subject and at least one IV is within- subject. So now you have two control problems: creating equivalent groups for the between- subject factor and controlling for order effects for the within-subject factor. OR 8 HOW MANY SUBJECTS ARE NEEDED IN A MIXED FACTORIAL DESIGN? 9 10 PxE FACTORIAL DESIGN These between-subject designs have both a manipulated IV and a subject IV. (ie, P represents “person” or subject variable, and E represents “environment” or manipulated variable.) PxE MIXED FACTORIAL DESIGN This is a further breakdown of the PxE design. This is a design that involves a between-subject factor, a within-subject factor, a subject variable and manipulated variable. Remember, if you have a subject variable, it must be a between-subject factor. I.V. I.V. between-subject within-subject also the subject variable also the manipulated variable SUMMARY DIAGRAM (where circle represents an IV) 11 12 SIMPLE EFFECTS ANALYSIS If your ANOVA indicates that the interaction effect is statistically significant, that gives you the “green light” to perform this simple effects analysis to determine the more detailed nature of that interaction and whether these subsequent comparisons are statistically significant. (don’t do this analysis if the interaction is not significant). In other words, you’re breaking down the interaction further to understand it. It examines the effects of each IV at each of the levels of the other IV. Notice that you don’t compare the conditions that “are on the diagonal”. COMPARISONS MADE IN A SIMPLE EFFECTS ANALYSIS: Compare Condition 1 and 2 (do color conditions differ at 0oz?) Compare Condition 3 and 4 (do color conditions differ at 2oz?) Compare Condition 1 and 3 (do alcohol conditions differ at red?) Compare Condition 2 and 4 (do alcohol conditions differ at green?) Note: you do not compare the conditions on the diagonal (1vs 4, or 3 vs 2). 13