BMS2043 Statistics & Data Analysis Lecture 1 2024 PDF
Document Details
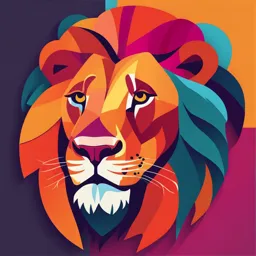
Uploaded by CongratulatoryIntelligence5915
University of Surrey
2024
null
Youngchan Kim
Tags
Related
- Statistics for Marketing Notebook PDF
- Statistics & Data Analysis Lecture 2 2024 PDF
- BMS2043 Statistics & Data Analysis Lecture 3 2024 PDF
- BMS2043 Statistics & Data Analysis Lecture 4 2024 PDF
- Biochemistry of Nutrition Topic 12 Nutrition Methodology PDF
- Principles and Techniques of Biochemistry and Molecular Biology PDF
Summary
These are lecture notes from an undergraduate Analytical and Clinical Biochemistry course at the University of Surrey, Spring 2024. The lecture covers descriptive and inferential statistics.
Full Transcript
Statistics & Data Analysis Analytical and Clinical Biochemistry (BMS2043) Spring 2024 Lecture 1 Youngchan Kim, PhD Lecturer in Quantum Biology University of Surrey [email protected] | 01AZ04 Outline of the lectures and material 2 x 2h lectures: Lecture 1: Introduction, recap of previous kno...
Statistics & Data Analysis Analytical and Clinical Biochemistry (BMS2043) Spring 2024 Lecture 1 Youngchan Kim, PhD Lecturer in Quantum Biology University of Surrey [email protected] | 01AZ04 Outline of the lectures and material 2 x 2h lectures: Lecture 1: Introduction, recap of previous knowledge, descriptive statistics Lecture 2: Inferential statistics, part 1 Lecture 3: Inferential statistics, part 2 Lecture 4: Statistical software – GraphPad Prism BMS2043 – Statistics and Data Analysis, 2024 Practical things Course work deadlines: Report 1 (HPLC & Drug Metabolism) due Tue. 16 April 2024 4:00 PM through SurreyLearn Report 2 (Creatinine Clearance) due Fri. 10 May 2024 4:00 PM through SurreyLearn MCQ invigilated exam – Thur. 23 May 2024 (to be confirmed) Questions: SurreyLearn Discussion Board (Dr Youngchan Kim) BMS2043 – Statistics and Data Analysis, 2024 Learning Objectives By the end of the lectures it is expected that you should: o Be able to describe basic concepts in statistics o Understand the difference between descriptive and inferential statistics o Understand the basic principles in conducting a statistical test o Be able to choose a correct statistical test for your problem o Be able to do basic statistical analysis using GraphPad Prism BMS2043 – Statistics and Data Analysis, 2024 Further resources StatQuest with Josh Starmer in Youtube, https://www.youtube.com/@statquest GraphPad Software Tutorials in Youtube, https://www.youtube.com/@GraphPadSoftware Statistics without Tears, An Introduction for Non-Mthematicians, Derek Rowntree, Penguin Random House, 2018. CatchUp Maths & Stats for the Life and Medical Sciences. Michael Harris, Gordon Taylor, Jacquelyn Taylor. Second Edition, Scion Publishing Ltd, 2013 BMS2043 – Statistics and Data Analysis, 2024 Introduction Beginning of session poll PollEV.com/youngchan Statistics poll 1 BMS2043 – Statistics and Data Analysis, 2024 Statistics Dictionary.com The practice or science of collecting and analysing numerical data in large quantities, especially for the purpose of inferring proportions in a whole from those in a representative sample. https://www.merriam-webster.com/dictionary/statistics 1: a branch of mathematics dealing with the collection, analysis, interpretation, and presentation of masses of numerical data 2: a collection of quantitative data BMS2043 – Statistics and Data Analysis, 2024 Why do we need statistics? To distinguish between randomness and systematic features in large datasets Main tasks of statistical analysis Design of experiments and data collection Data description: summary statistics, graphs, tables, etc. Tests of hypotheses: estimation of parameters, comparison of groups Model-fitting: investigation of (complex) association structures, prediction, classification, etc. BMS2043 – Statistics and Data Analysis, 2024 Statistics in our day-to-day life Office for National Statistics, https://www.ons.gov.uk How do we know climate change is real? https://climate.nasa.gov/evidence/ Regional ethnic diversity in the UK (UK Gov., 22 December 2022, https://www.ethnicity-factsfigures.service.gov.uk/uk-population-by-ethnicity/national-and-regional-populations/regionalethnic-diversity/latest/ Covid map: Coronavirus cases, deaths, vaccinations by country (BBC News, 1 March 2021) https://www.bbc.co.uk/news/world-51235105 Bowel cancer screening to start earlier at age 50 in England (BBC News, 10 August 2018) https://www.bbc.co.uk/news/health-45143895 HPV vaccine has almost wiped out infections in young women, figures show (The Telegraph, 18 June 2018) https://www.telegraph.co.uk/science/2018/06/17/hpv-vaccine-has-almost-wipedinfections-young-women-figures/ Genetic risk scores could help the NHS but they aren't ready yet (NewScientist, 20 March 2019) https://www.newscientist.com/article/2197109-genetic-risk-scores-could-help-the-nhs-butthey-arent-ready-yet/ https://consumer.huawei.com/uk/mobileservices/health/ Where do you need statistics? Ability to read and evaluate (scientific) news and papers Your course work Your PTY Your final year dissertation work Your future career? BMS2043 – Statistics and Data Analysis, 2024 Theoretical basis of statistics Based on the theory of probability Probability is a measure of likelihood of an event on a scale ranging from 0 to 1. In other words, the probability that an event will occur is the fraction of times you expect to see that event in many trials. An event with probability 0 cannot happen An event with probability 1 is certain to happen Often probability can be interpreted as a population relative frequency – a fraction, proportion or percentage E.g., if 0.5% of a population of interest has a certain genetic mutation, the probability of occurrence of that mutation is 0.005 or 0.5%. BMS2043 – Statistics and Data Analysis, 2024 Probability and odds The odds are defined as the probability that the event will occur divided by the probability that the event will not occur. If probability of an event is p, odds of the event are defined as p/(1-p). Odds can be expressed as a number or as a ratio Examples: p = ½ = 0.5, odds = 0.5/0.5= 1 (odds 1:1) p = 1/5 = 0.2, odds = 0.2/0.8 = 1/4 = 0.25 (1:4) p = ¾ = 0.75, odds = 0.75/0.25 = 3 (3:1) NB! If odds=p/(1-p), then p = odds/(1 + odds) Image source: Wikipedia Example: A horse has run 100 races and has won 60 of them. What is its probability of winning a race? How about odds? BMS2043 – Statistics and Data Analysis, 2024 Further on probabilities in (bio)statistics Diagnostic tests: Sensitivity & Specificity Predictive values of tests: Positive predictive value & Negative predictive value Probability distributions: Most statistical methods assume an underlying distribution P-value is also a probability! BMS2043 – Statistics and Data Analysis, 2024 Example - diagnostic test for sensitivity Sensitivity: “If I have Disease X, what is the likelihood I will test positive for it?” Mathematically, this is expressed as: Sensitivity = True Positives / (True Positives + False Negatives) Sensitivity =TP / (TP + FN) = 134 / (134 + 11) = 134 / 145 = 0.924 BMS2043 – Statistics and Data Analysis, 2024 The Company’s blood test identified 92.4% of those WITH Disease X. https://uk.cochrane.org/news/sensitivity-and-specificity-explained-cochrane-uk-trainees-blog Example - diagnostic test for specificity specificity: “If I do not have Disease X, what is the likelihood I will test negative for it?” Mathematically, this is expressed as: specificity = True Negatives / (True Negatives + False Positives) Sensitivity =TN / (TN + FP) = 245 / (245+ 7) = 245 / 252 = 0.972 BMS2043 – Statistics and Data Analysis, 2024 The Company’s blood test identified 97.2% of those WITHOUT Disease X. https://uk.cochrane.org/news/sensitivity-and-specificity-explained-cochrane-uk-trainees-blog Example - Positive Predictive Value Positive Predictive Value (PPV) is the proportion of those with a POSITIVE blood test that have Disease X. "If I have a positive test, what is the likelihood I have Disease X?" PPV = True Positives / (True Positives + False Positives) =TP / (TP + FP) = 134 / (134 + 7) = 134 / 141 = 0.95 BMS2043 – Statistics and Data Analysis, 2024 The blood test identified 95% of those with a POSITIVE blood test, as having Disease X. https://uk.cochrane.org/news/sensitivity-and-specificity-explained-cochrane-uk-trainees-blog Example - Negative Predictive Value Negative Predictive Value (NPV) is the proportion of those with a NEGATIVE blood test that have Disease X. "If I have a negative test, what is the likelihood I do not have Disease X?" PPV = True Negatives / (True Negatives + False Negatives) =TN / (TN + FN) = 245 / (245 + 11) = 245 / 256 = 0.957 BMS2043 – Statistics and Data Analysis, 2024 The blood test identified 95.7% of those with a NEGATIVE blood test, as not having Disease X. https://uk.cochrane.org/news/sensitivity-and-specificity-explained-cochrane-uk-trainees-blog Study design, data types, and distributions Study designs Experimental study Randomised controlled trial (RCT) Intervention group (e.g. new drug) vs. placebo group Observational study No intervention, just observation Cohort study: A group of subjects linked in some way, e.g. geographical region Case-control study: e.g. people with a disease vs. those without Prospective (real time) vs. retrospective study (data collected about past) Cross-sectional (a point in time) vs. longitudinal study (collection of data on same participants over an extended period of time) Representative (random) sample BMS2043 – Statistics and Data Analysis, 2024 Types of data Qualitative Quantitative subjective characteristics and opinions things that cannot be expressed as a number Continuous: Can be measured Height Weight Age Temperature Discrete: Can be counted Nominal: Categories with no ordering Number of students Male, female, non-binary Ordinal: Ordered categories First, second, third 0 hours, 1-4 hours, 5+ hours Interval: Known, equal intervals BMS2043 – Statistics and Data Analysis, 2024 £0-10k, £10-20k, £20-30k Distribution of the data Most data can be described through a mathematical distribution, such as: Normal (also called the Gaussian distribution) Uniform Exponential Mean ± 1 SD includes 68,2% of cases Mean ± 2 SD includes 95,4% of cases Mean ± 3 SD includes 99,7% of cases Binomial Geometric Poisson BMS2043 – Statistics and Data Analysis, 2024 Standard normal distribution Examples of distributions BMS2043 – Statistics and Data Analysis, 2024 Descriptive statistics Descriptive statistics Purpose: to describe the distribution of a phenomenon E.g. Height in a population E.g. Proportion of highly educated in a population Cannot be used to make inference without a statistical test Measures of Location Dispersion (spread) of the data Association (for two variables) BMS2043 – Statistics and Data Analysis, 2024 Measures of location, dispersion and association Measure Purpose Arithmetic mean To describe a middle point or central tendency Median Mode To describe the most common value in data Fractile, e.g. quartiles To describe the cut-off point where the distribution reaches a certain probability, e.g. 25% of the sample Frequencies, percentages Standard deviation To describe how spread out the data is Range (min, max) Interquartile range Contingency tables (cross-tabulations) Correlation BMS2043 – Statistics and Data Analysis, 2024 To describe the association between two variables Kaakinen et al. Am J Epidemiol 2010:172:653-665 BMS2043 – Statistics and Data Analysis, 2024 BMI (Body Mass Index) = Weight (kg) / [Height (m)]2 Arithmetic mean The average of the observed values Example: time spent on screen/day in minutes N=10: 30, 120, 45, 10, 90, 80, 25, 40, 115, 100 Mean= (30+120+45+10+90+80+25+40+115+100)/10 = 655/10 = 65.5 (min) BMS2043 – Statistics and Data Analysis, 2024 Standard deviation (SD) The most commonly used measure of spread or variability in the sample Measures how spread the data are around the mean Note! Variance = squared SD = s2 = 𝜎2 A high SD indicates a very spread-out data (the subjects have different values, there is a lot of variability) A low SD means the data are tightly grouped (the subjects have very similar values, i.e. there is little variability) Example: time spent on screen/day in minutes N=10: 30, 120, 45, 10, 90, 80, 25, 40, 115, 100 mean=65.5 SD=sqrt(((30-65.5)2+(120-65.5)2+…+(100-65.5)2)/(10-1))=40.1 BMS2043 – Statistics and Data Analysis, 2024 Example: Mean and SD Mean = 80 kg SD = 5 kg BMS2043 – Statistics and Data Analysis, 2024 Mean = 80 kg SD = 3 kg Standard error (SE) of the mean, SEM If we repeat the data collection many times (i.e. take many samples from the population), there will be a different mean each time. With SEM, we can measure the variability across many samples in a population. Remember: SD measures variability within a single sample SEM = 𝜎x = 𝜎 ! ≈ " ! Where, 𝜎 = standard deviation (SD) of the population. Since population SD 𝜎 is seldom known, we use sample SD s, and n = sample size (number of observations). Example: time spent on screen/day in minutes --> N=10; mean=65.5; SD=40.1 SEM =40.1/sqrt(10) =12.7 BMS2043 – Statistics and Data Analysis, 2024 Skewness and kurtosis Symmetrical and skewed distributions Kurtosis in the normal curve Skewness is used to measure the degree of asymmetry of a distribution. In other words, it quantifies the degree of distortion from the normal distribution. For skewed distributions, better to report median rather than mean. Kurtosis is a measure that describes how heavily the tails of a distribution differ from the tails of a normal distribution. NB! With large sample sizes slight deviations not so serious, i.e. ok to have approximately normal distribution. Highway Safety Analytics and Modeling, Chapter 5, Exploratory analyses of safety data, https://doi.org/10.1016/B978-0-12-816818-9.00015-9 BMS2043 – Statistics and Data Analysis, 2024 Summary: How to describe your data Dependencies between two variables: Correlation Two commonly used measures: 1. Pearson correlation: quantitative traits linear relationship 2. Spearman correlation: Quantitative or ordinal data, e.g. Likert scale (1,2,3,4,5) Does not require a linear relationship; however, needs the data to follow a monotonic relationship Good for non-normal data Based on ranks of the data Both give us a correlation coefficient r, -1 ≤ r ≤ 1 BMS2043 – Statistics and Data Analysis, 2024 Spearman correlation Positive monotonic Negative monotonic Non-monotonic Pearson correlation Pearson correlation Pearson correlation Spearman correlation Spearman correlation Spearman correlation BMS2043 – Statistics and Data Analysis, 2024 Spearman vs. Pearson Figure by Skbkekas - Own work, CC BY-SA 3.0, https://commons.wikimedia.org/w/index.php?curid=8778554 BMS2043 – Statistics and Data Analysis, 2024 Pearson correlation: example Weight Height weight – mean(weight) height – mean(height) 60 160 -3.8 -5.2 19.76 65 163 1.2 -2.2 -2.64 55 164 -8.8 -1.2 10.56 74 182 10.2 16.8 171.36 65 157 1.2 -8.2 -9.84 Mean: 63.8 SD: 7.05 Mean: 165.2 SD: 9.78 BMS2043 – Statistics and Data Analysis, 2024 Sum: 189,2 Dependencies between two variables: Correlation Several sets of two variables plotted against each other, with the Pearson correlation coefficient for each set: If the two variables are not associated, their correlation is 0 but it is not true vice versa – correlation 0 does not necessarily imply no associations! https://en.wikipedia.org/wiki/Correlation_and_dependence BMS2043 – Statistics and Data Analysis, 2024 Dependencies between two categorical variables: cross-tabulation SEX Hypertension, no Hypertension, yes Row total Male 2035 0.437 509 0.775 2544 Female 2625 0.563 148 0.225 2773 Column total 4660 0.876 657 0.124 5317 BMS2043 – Statistics and Data Analysis, 2024 Graphical ways of describing data Bar chart Histogram Boxplot Outliers Max (or Q3+1.5*IQR) 75th percentile (Q3) median 25th percentile (Q1) Density plot Scatter plot Min (or Q1-1.5*IQR) IQR = interquartile range = Q3-Q1 BMS2043 – Statistics and Data Analysis, 2024 Test your knowledge 1. Name at least five different measures that you can use to describe your data. 2. Which of the following statements is TRUE: the standard error of the mean (SEM) is affected by: a. The size of the sample b. The sample mean d. The standard deviation of the sample e. All of the above f. None of the above 3. What measures are preferred to describe a skewed distribution? Why? BMS2043 – Statistics and Data Analysis, 2024