ANM150 AY2324 Week 6 Research 8 Statistics.pdf
Document Details
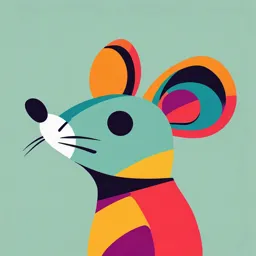
Uploaded by HandierMesa
Tags
Full Transcript
STATISTICS CASE 10 ANM 150 Dr. Monique Aucoin ND MSc OBJECTIVES Define terms like sample, population, incidence, prevalence Describe the process of hypothesis testing and assessing for statistical significance Define and interpret Relative and Absolute Risk, Odds ratio, number needed to tre...
STATISTICS CASE 10 ANM 150 Dr. Monique Aucoin ND MSc OBJECTIVES Define terms like sample, population, incidence, prevalence Describe the process of hypothesis testing and assessing for statistical significance Define and interpret Relative and Absolute Risk, Odds ratio, number needed to treat/harm, power Differentiate between clinical and surrogate outcomes and their respective strengths QUICK POLL Raise your hand if you have taken a course in statistics? Calculated statistics for a research project? Know what a p value is? INTERPRETING STUDY FINDINGS: STATISTICS TERMINOLOGY Population vs sample Population Sample TERMINOLOGY: PREVALENCE/INCIDENCE Prevalence: Represents the total number of cases suffering from a disorder E.g. In 2008, 26 630 persons in Ontario are HIV positive Incidence: Represents the number of new cases suffering from a disorder E.g. In 2008, 1620 persons were newly infected with HIV in Ontario 1620 / 12.8 million = ~ 0.0001 or 1 in 10 000 HYPOTHESIS TESTING The process of deciding, statistically, whether the findings of an investigation reflect chance or real effect STATISTICS Difference in group means (averages) Ex. Average blood pressure in the treatment vs control groups Difference in the likelihood of an outcome Ex. likelihood of a heart attack in the treatment vs control groups Statistical significance (real differences or chance?) STATISTICAL SIGNIFICANCE: P VALUE P-value -what are the odds that the difference is due to chance? -0.05 is generally the cut off (5% likelihood) -p greater than 0.05 not significant -p less than 0.05 significant STATISTICAL SIGNIFICANCE: CI 95% Confidence interval (CI) -we don’t know the exact result, 95% sure that it’s within this range -if these intervals overlap non-significant difference -if they don’t overall significantly different STATISTICAL SIGNIFICANCE Ex 1. Hemoglobin A1c in group 1 is 5.7 (95% CI 5.4-6.2), in control group 6.1 (95% CI 5.9-6.5) Significant? Ex 2. Hemoglobin A1c in group 1 is 5.7, in control group 6.1 p=0.055 Significant? STATISTICAL SIGNIFICANCE Based on: How big is the difference? How big is the sample size? Concept of “Power” Non-sig difference – may be because too few participants to detect a difference How did this relate to meta-analyses? RELATIVE RISK Probability of a bad outcome in RR = 1 No difference the intervention group divided RR > 1 Risk of bad outcome INCREASED by probability of a bad outcome with tx in the control group RR < 1 Risk of bad outcome DECREASED with tx Relative Risk = event rate (tx) Ex. Patients taking vitamin C, 12% got URTI, control group, 20% got event rate URTI (placebo) RR= 12/20 = 0.6 RELATIVE RISK REDUCTION How much did the treatment reduce the risk of the bad outcome? RRR= 1-relative risk Previous example: RR= 12/20 = 0.6 RRR= 1-0.6 = 0.4 (40% reduction in risk with the vitamin C) ABSOLUTE VS RELATIVE RISK REDUCTION Absolute RR: the absolute amount the intervention decreased the risk ARR= risk of outcome with placebo – risk with mediation Previous example: ARR = 20%-12%= 8% (RRR = 1-0.6 = 0.4 (40%)) ABSOLUTE VS RELATIVE RISK REDUCTION Ex. Intervention reduces risk from 4% to 3% RRR = 25% (This is misleading compared to ARR) ARR = 1% Ex 2. Pacifier use decreases risk of SIDS by 50%, risk of SIDS 4 per 100,000 (0.00004%) ***When outcomes occur infrequently, can see large difference between ARR and RRR ODDS RATIO – REFRESHER FROM THE SR LECTURE Looking at the relationship between an exposure (ex. Eating a food, taking a drug, exposure to a toxin) and an outcome (having a heart attack, a change in a blood value, being hospitalized) Often used in observational studies (but sometimes in clinical trials too) ODDS RATIOS OR = 1 exposure does not affect the likelihood of the outcome OR>1 exposure associated with increased likelihood of the outcome OR correct but misleading OUTCOMES CLINICAL OUTCOMES A clinical event Ex. Stroke, suicide, hip fracture SURROGATE OUTCOMES Not a clinical event An indicator that can be observed sooner, at a lower cost, or less invasively Allows inferences about effect on the true outcome AKA: biomarkers, indicators of disease progression OUTCOMES Stroke LDL-Cholesterol Suicide Columbia Suicide Severity Scale Hip Fracture Bone mineral density SURROGATE OUTCOME Must be validated (shown to predict the outcome) If not, may be a poor outcome Ex. PSA levels and prostate cancer STATISTICAL SIGNIFICANCE Not due to chance P value of less than 0.05 less than 5% likelihood that the difference was due to chance alone Heavily dependent on sample size -big enough sample small differences statistically significant -ex. 569 pt with advanced cancer, drug intervention, 6.24 months vs 5.91 months, p=0.038 CLINICAL SIGNIFICANCE Does the intervention have a genuine, measurable effect Related to extent of change, whether the change make a real difference in the lives of patients No broadly accepted standard for this Involves clinician and patient judgement POWER Ability of a study to detect a real difference between two groups (ex. People taking a treatment and people taking placebo) Depends on sample size, size of the effect, variability within a sample Lower power: may not detect a difference even if one exists BUT also: Small sample sizes may find a significant effect when one doesn’t exist (use caution when reading small pilot studies!) Button KS, Ioannidis J, Mokrysz C, Nosek BA, Flint J, Robinson ES, Munafò MR. Power failure: why small sample size undermines the reliability of neuroscience. Nature reviews neuroscience. 2013 May;14(5):365-76. QUESTIONS? COMMENTS? REFLECTIONS OR INSIGHTS?