A Guide for Assumptions Checks PDF
Document Details
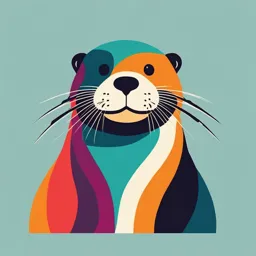
Uploaded by FavoriteHelium
Arianne E. Autrz, Rpm and Joshua Q. Cadayona, Rpm
Tags
Summary
This guide provides a step-by-step approach of how to check assumptions for different statistical tests. It details various methods to assess assumptions regarding normality, outliers, and homogeneity of variances. It offers clear examples of how to conduct these tests.
Full Transcript
PREPARED BY: ARIANNE E. AUTRIZ, RPm and JOSHUA Q. CADAYONA, RPm A GUIDE FOR ASSUMPTIONS CHECKS The following information can be considered as a guide when conducting assumptions checks to see whether the hypothesis-testing procedu...
PREPARED BY: ARIANNE E. AUTRIZ, RPm and JOSHUA Q. CADAYONA, RPm A GUIDE FOR ASSUMPTIONS CHECKS The following information can be considered as a guide when conducting assumptions checks to see whether the hypothesis-testing procedure is appropriate for the data, or the assumption(s) is/are violated. Various methods are indicated per assumption check, as well as when to use these methods. Normality Assumption Shapiro-Wilk Test (ideal if n < 50, because it can falsely report that a normally distributed data is non-normal if the sample size is large) o If p-value is <.05 = non-normal o If p-value is ≥.05 = normal Kolmogorov-Smirnov Test (if n ≥ 50) o Same decisions with Shapiro-Wilk Test Histogram (used if sample size is large, e.g., 300) o Visually check if the distribution follows the normal curve Q-Q Plot (used with histogram if sample size is large) o If the dots follow the straight line, the data is normally distributed No Significant Outliers Assumption Boxplot o If no dots are present, there are no outliers o If dots are present, there are outliers Scatterplot (applicable only for correlation) o Visually check if there is plotted data that seems to be far from the pattern; if a datum is far from the pattern, there is a significant outlier. Equal Variances Homogeneity of Variance (for t test and ANOVA) o Levene’s Test ▪ If p <.05 = unequal variance ▪ If p ≥.05 = equal variance Homoscedasticity (for correlation) o Scatterplot ▪ Visually check if the data “fans out”; if the data shows this pattern, the data is not homoscedastic (i.e., heteroscedastic). PREPARED BY: ARIANNE E. AUTRIZ, RPm and JOSHUA Q. CADAYONA, RPm Linearity of Relationship (for correlation) Scatterplot o Visually check if the pattern of the data is following a linear path (i.e., in a straight line). ▪ If yes, there is linearity of relationship. ▪ If the pattern curves, the relationship of the variables is non-linear. Independence of Observations To check if the observations are unrelated to each other, the respondents’ scores must not be duplicated. Expected Counts (for Chi-square statistic) For chi-square test of goodness of fit o Check expected frequencies: there must be at least 5 expected frequencies per group. For chi-square test of independence o Check expected frequencies: less than 20% of the cells have an expected frequency that is less than 5. Mutual Exclusivity (for Chi-square statistic) To check if each observation only contributes to one cell (i.e., a respondent can only be found in one group), the encoding of the data should be checked if every respondent belongs to only one group. CHOOSING THE RIGHT STATISTICS t-Test - Used when the study aims to compare or identify difference between two groups One-Sample t Test - if the population mean is present but there is no existing population variance Assumptions: 1. Variable is continuous 2. Observations are independent 3. Normal distribution 4. No outliers PREPARED BY: ARIANNE E. AUTRIZ, RPm and JOSHUA Q. CADAYONA, RPm t-Test for Dependent Samples (Paired t-Test) - comparing two different sets of data from a single set of respondents (i.e., 1 group of respondents, 2 scores each) Assumptions: 1. Dependent variable is continuous (i.e., interval or ratio) 2. Independent variable must be two, categorical related groups 3. Observations are independent 4. Normal distribution in difference scores 5. No significant outliers in difference scores ***Use Wilcoxon-Signed Rank Test (Nonparametric counterpart of Paired t-Test) if assumptions of parametric test is violated t-Test for Independent Samples - comparing two sets of data from two different sets of respondents (i.e., 2 different groups of respondents, 1 score each) Assumptions: 1. Dependent variable is continuous. 2. Independent variable should have two categorical independent groups (two different groups) 3. Observations are independent. 4. Normal distribution among two groups. 5. No outliers in the two groups. 6. There has to be homogeneity of variance (If this assumption is violated, use Welch’s t) ***Use Mann-Whitney U Test (Nonparametric counterpart of t-Test for Independent Samples) if assumptions of parametric test is violated One-Way ANOVA - used to compare three or more groups Assumptions: 1. Dependent variable is continuous 2. Independent variable consists of two or more categorical, independent groups. 3. Observations are independent. 4. No significant outliers. 5. Normal distribution is observed among all groups, or the residuals of the dependent variable is normally distributed. 6. There is homogeneity of variances. If all assumptions were met, Student’s ANOVA should be used. As for post hoc comparison (if ANOVA results indicate statistical significance): ○ Tukey’s HSD test = equal sample size PREPARED BY: ARIANNE E. AUTRIZ, RPm and JOSHUA Q. CADAYONA, RPm ○ Tukey-Kramer test = unequal sample size ○ Scheffé test = unequal sample size If all assumptions were met except homogeneity of variance, use Welch’s ANOVA and Games-Howell test as post hoc comparison. If assumptions were violated, Kruskal-Wallis H test should be used, then either Dunn’s test (or Bonferroni Procedure) or DSCF Pairwise Comparisons can be used as a post hoc test. Variations of ANOVA Repeated-Measures ANOVA = three or more sets of scores are coming from the same respondents Two-Way ANOVA = two factors are used (e.g., measuring the effect of coffee and music to memory) Analysis of Covariance (ANCOVA) = ANOVA where other variables that might affect the study are being controlled Multivariate Analysis of Variance (MANOVA) = ANOVA where more than one dependent variable is being studied at once (e.g., effect of coffee to hyperactivity and attention span) Multivariate Analysis of Covariance (MANCOVA) = like ANCOVA but more than one dependent variable is being studied at once. Pearson’s r Correlation - used to determine the relationship between two variables Assumptions: 1. Variables are both continuous. 2. Variables should be paired. 3. Observations are independent. 4. The relationship must be linear. 5. Bivariate normal distribution is present. 6. No univariate or multivariate outliers. 7. Homoscedasticity is present. If assumptions were violated, either use Kendall’s tau-B correlation or Spearman’s rho (but Kendall’s tau is more preferred due to its robustness compared to Spearman’s). Chi-Square Test for Goodness of Fit - used to determine if the actual proportions of the categories being studied (e.g., number of men vs. women who committed crimes) do not fit to an expected proportion (e.g., 50% of those who committed crimes are men while the other 50% are women). PREPARED BY: ARIANNE E. AUTRIZ, RPm and JOSHUA Q. CADAYONA, RPm Assumptions: 1. One categorical variable 2. Independence of observations 3. Mutually exclusive groups 4. At least 5 expected frequencies in each group Chi-Square Test for Independence - used to determine if various categories are not related to each other (e.g., if certain color preferences are associated with specific personality traits). Assumptions: 1. Two categorical variables with at least two or more groups. 2. Independence of observations. 3. Less than 20% of the cells have an expected frequency of less than 5.