Week 7 Descriptive Statistics PDF
Document Details
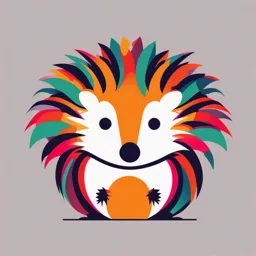
Uploaded by IndustriousRockCrystal
Tags
Summary
These notes cover descriptive statistics, focusing on averages, variability, spread, and common values like range. The document details the frequentist approach to data analysis and discusses its implications for data distribution, estimates, and confidence intervals.
Full Transcript
Week 7 Descriptive Statistics What is the best way to describe the sample of data collected Sample – what you collect, subset of individuals that represent a population Cannot provide datasheet Report things like o Averages o Variability o Spread...
Week 7 Descriptive Statistics What is the best way to describe the sample of data collected Sample – what you collect, subset of individuals that represent a population Cannot provide datasheet Report things like o Averages o Variability o Spread o Most common o Range Try to collect data to make generalizations of a population, try to have characteristics of population of interest Key aspects and principles (frequentist approach) Data distribution and assumptions o Many frequentist methods are based on certain assumptions about the data (e.g. normality) o Validity of the results often hinges on whether these assumptions hold Point estimation o Best guess for population parameters based on sample data Confidence intervals o Frequentists provide a range (interval) of values that likely contain the parameter o Confidence level indicates frequency the interval would contain the parameter if experiment were to be repeated Descriptive statistics Value representing the centre of a distribution is known as CENTRAL TENDENCY can be measured in 3 ways: o mean o median o mode Calculating mean in science, we must work with our observations in terms of probability, diTerence between 6 observations and 60 observations more samples, more errors that are going to cancel out, close the estimate to the population value Histograms – tell us how often each value of a variable occurs use thee to look at shape of distribution of scores in a single variable order the variable values on the x-axis from smallest to largest group data by bins of equal size Y axis is number (frequency) of data points in each bin Mode Score that most frequently occurs in dataset Easy to spot = tallest bar Median Middle score when scores are ranked in order of magnitude Important reminders Median o Relatively unaTected by extreme values o Relative unaTected by skewed distributions Mean o Influenced by extreme values o Influenced by skewed distributions Caution o Calculating mean/central tendency is not always cancelling out errors o “What am I trying to measure and what does value mean?” o Sometimes parameter estimation is an actual value that we can never know but still very useful to summarize data Range Very easy to calculate Take the largest score and subtract from smallest score **can be dramatically aTected by extreme scores Interquartile range IR addresses concern by of influenced of extreme values For IR we cut oT top and bottom 25% of the data and are left with the middle 50% Quartiles are the 3 values that sort the data into equal parts *Important things to remember o Is not aTected by extreme scores at either end o Only problem – you lose a lot of data! o Graphs referred to boxplots or box and whiskers Variance Important! Measure by finding diTerence of each value from the mean o Sum of Squares (SS) § Square the values because of negative data points § **total value not an average o Variance (V) § Don’t calculate the average like normal § Divide by N-1 (ex. Instead of 10/5=2, do 10/4-2.5) Standardization is a fundamental concept in statistics Standard deviations Z – scores Standard deviation puts variable back on its own scale When calculating variance, have to fix neg value problem o Put the values in “SS units” o Need to bring it back to the units the variable was collected in! Z-score: final boss of standardization Z-score – standardized value that tells you how far away a single value id from the mean of set of values o Must standardize scales before comparing diTerent groups o Relative vs. Absolute Z-score – gives the “standard normal distribution” Relative vs absolute Week 8 What is probability? Probability – measure of likelihood or chance that a particular event will occur Types of probability o Subjective probability – based on personal judgment or belief about how likely an event is, such as predicting the weather o Theoretical probability – based on mathematical reasoning, such as coin tosses or dice rolls o Experimental probability – based on actual experiments or observed data Probability is an extension of logic o True & false à corresponds to absolute belief o Almost some level of uncertainty Probability allows us to extend logic to work with uncertain values between true and false Classical answer from latin means “to test”, and related to several words in English: probe, proof, approve “that which happens generally but not invariability” o Aristotle viewed probability as related to events that happen frequently but are not guaranteed o Early interpretations were qualitative, focusing more on general tendencies that precise calculations Probability was observed as a natural tendency rather than calculated accurately Transition to quantitative probability – during renaissance, shift toward mathematical probability began more systematic calculations and regularized objects like standardized dice Renaissance period Gaming equipment more reliable Mathematics of chance started to become a topic Common example: o 0-0 to start, first to 3 gets $100 Key figures Pascal and Fermat initiated formal probability by working on problems related to gambling o Credited with early developments of probability theory o Idea to divide the stakes according to what we would now refer to as each player’s expected value Distribution = point/general mass in middle, frequency of data above of below? Expected value and probability distribution Expected value – weighted average of all possible outcomes Use of probability distribution to assign likelihood to each outcome Each player’s payout based on their probability of winning The classical answer – summary Advantages o Intuitive o Works well for relatively simple dice and card games Disadvantages o Doesn’t work well with anything else Frequentist approach “that which generally happens” Connection was assumed between probabilities of events and their occurrences in repeated observations o Relative frequency o By definition, long-run frequency of occurrence is the probability Bernoulli’s law of large numbers Able to show observed frequencies (e.g count estimate) would converge to the true probability as the number of trials got larger o Some “moral certainty” of an unknown probability Ex. Flipping a coin o Theoretically – 50% chance of getting heads or tails o Flip 10x, chance of 7 heads 3 tails, just by chance o Flip 10k times, likely to be much closer to a 50-50 split As sample size increases, sample estimate will get closer to expected value (or true estimate) of the entire population (side note: sample vs population) o The more data points you have, the more reliable your estimate becomes Galton Wisdom of Crowds “wisdom of the crowd” is an observation about aggregate judgement o Individual estimates very widely o Aggregated data cab converge on an accurate or true value Avg estimate of a large group can be surprisingly accurate, even more so than individual expert judgements Frequentist approach Developing the concept of probability as an objective fact, verifiable by observations of frequency OTers an appealing degree of objectivity, explains popularity among scientists Statistical distributions Normal distribution Bell-shaped (aka symmetry) Majority of scores lie around centre of distribution One peak (unimodal) Further away from centre, scores become less frequent Originally known as Gaussian distribution Normal distribution is one of the most fundamental and useful statistical tools Why normal distributions are “normal” Normal distributions reflect life’s complexity Things we study in the real world are often complicated and are the sum of many small factors When you sum many small things together, look “gaussian” or “normal” When you start adding fluctuations, they cancel each other out More fluctuations, better chance that fluctuation will be cancelled by one in the opposite direction Central limit theorem – eventually, most likely sum is one where all fluctuations are cancelled out, this would be a sum of zero (relative to the mean) Frequentist approach Views probability as long-rum frequency of events Inference approach – relies on concepts like p-values, confidence intervals, and null hypothesis significance testing Many standard methods in statistics are based on frequentist principles and the Gaussian distribution o Mean, median, mode o T-tests, ANOVA, etc. o Confidence intervals Biggest critique – reliance on p-values and null hypothesis significance testing has been criticized for various reasons, including potential misinterpretations and over-reliance on arbitrary significance levels Where the frequentist approach struggles o Extremely rare events o One time events o Identifying the appropriate reference class § Such situations require probability suitable for inference P-value – measures the probability using the area under the normal curve beyond the observed Z- score Bayesian approach Uses formula (Bayes’ theorem) combines prior belief, the new data you collected, and how likely that new data is, given prior belief Result of formula is updated belief or “posterior” Allows you to combine previous knowledge with new experimental data, oTering a more comprehensive understanding Bayesian statistics is like learning: start with what you know, encounter new info, update understanding based on new information Bayes theorem Prior, likelihood & posterior of bayes theorem Can use bayes theorem as a probability tool to logically Calculate and quantify how likely our belief is given # parts of the theorem o Posterior probability – probability of beliefs given we observed o Likelihood – probability of data given our beliefs o Prior probability – estimate of probability of belief Criticisms and limitations of Bayesian approach Subjectivity of priors – one primary critic of Bayesian stats is subjectivity involved in choosing prior distribution Computational intensity – Bayesian methods can be computationally intensive and. May only be feasible for some large datasets or complex models Historical momentum – frequentist stats has been dominant paradigm for a long time, textbooks, statistical software primarily designed around it Standardization – Bayesian stats, in contrast, oTers a lot of flexibility, can be both an advantage and a disadvantage. Procedures like hypothesis testing are standardized, making results easier to communicate and compare across studies Key aspect and principles (Frequentist statistics) Probability – long-run frequency of events Sample vs population – frequentist methods often focus on drawing inferences about population based on a sample; sample is used to make generalizations about the larger population from which it was drawn Data distribution and assumptions – frequentist methods are based on certain assumptions about the data (e.g. normality). Validity of the results often hinges on whether these assumptions holds Point estimation – provide single “best guesses” for population parameters based on sample data Confidence intervals – instead of providing a single estimate for a parameter, frequentists provide a range of values that likely contains the parameter. The confidence level (%) indicates the frequency with which the interval would contain the parameter if the experiment were repeated many times Hypothesis testing – central to frequentist stats, involves setting up null and alternative hypotheses Based on the data and the chosen significance level, one either rejects or fails to reject the null hypothesis P-values – key concept in hypothesis testing; is the probability of observing the given data (or something more extreme) assuming that the null hypothesis is true; small p-value (typically < 0.05) is evidence against the null hypothesis Week 9 Correlation – statistical measure of degree which 2 continuous variables change together (whether positive, or negatively related or unrelated) Measured using correlation coeTicient, quantifies the strength of relationship between the variables Useful for describing simple relationships among data Do not provide info about cause and eTect Can be used as a statistical test Negative correlation – one variable increases as the other decreases Positive correlation – one variable increases, so does the other; one variable decreases, so does the other Zero correlation – occurs when there is no relationship between 2 variables What we need to know to quantify a correlation Covariance Correlation co-eTicient Assumptions of Pearson’s correlation coeTicient Assumptions need to check first to ensure you can use the test: o Continuous variables o Equal variances between variables o No outliers o Related pairs (values come from the same subject) o Linearity (relationship between values is best described by a line) How to get variance to covariance Variance – calculated on ONE variable Covariance – calculated on TWO variables Are changes in one variable met with similar changes in the other variable? Covariance Covariance interpretation Positive covariance – as one variable deviates from mean, other variable deviates in same direction Negative covariance – as one variable deviates, the other variable deviates in opposite direction Cannot compare covariance in an objective way; is not standardized Cannot compare the covariance found in dataset to another dataset unless they are measured in units with similar range of values To Standardize covariance, need the correlation coeTicient CoeTicient gives covariance number meaning Known as the Pearson product-moment correlation coeTicient or Pearson correlation coeTicient Statistical Significance When employing inferential statistics, we are estimating parameters o Guessing at the true population parameter/statistic of interest Estimating parameters Guessing at the true population statistic of interest How to use stats to test hypothesis Hypothesis Alternative/Experimental hypothesis (H1) – prediction that comes from theory, typically states eTect/diTerence will be present (something is happening) Null hypothesis (H0) – prediction that states that an eTect is absent (nothing happening) Null Hypothesis Significance Testing Most commonly used method of inferential statistic that tests an observation using null hypothesis as default/starting position “Assume no eTect or diTerence until shown otherwise” NHST Default position is null; need strong evidence to reject the null hypothesis; not a 50/50 proposition NHST does not prove or disprove things; can either: o Support alternative hypothesis o State there isn’t enough evidence to reject null hypothesis Key point is that no question is definitively answered as true or not true How to tell null or alternative hypothesis is supported Statistical significance – based on probability that you would get same or more extreme results if null hypothesis is true (remember – frequentist approach) P(your results | there is no diTerence) = how likely is it that you get the results of experiment, if there was no diTerent or eTect o P value set at less than 5% - statistical significance occurs when probability of your result is