Investigation of Antalya Forest Fire's Impact on Air Quality PDF
Document Details
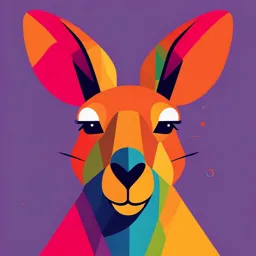
Uploaded by GratifyingMaclaurin
Konya Technical University
2023
Duygu Arikan and Ferruh Yildiz
Tags
Related
- Reglamento del Servicio de Bomberos Forestales de la Generalitat (PDF)
- Prevención de Incendios Forestales PDF
- Flames in the Forest Past Paper PDF
- Manual de Instrucciones y Procedimientos PDF
- Decreto 12/2011, de 17 de Febrero, Aprobación del Reglamento del Operativo de Lucha Contra Incendios Forestales (PDF)
- Test nº 4 - Incendio Forestal (30/04/2024) PDF
Summary
This study investigates the impact of the 2021 Antalya forest fire on air quality. It uses satellite imagery and Google Earth Engine to analyze burned areas, vegetation indices, carbon monoxide, and meteorological data. The study aims to assess the environmental effects of the fire and correlate findings with national air quality station data.
Full Transcript
Remote Sensing Applications: Society and Environment 29 (2023) 100922 Contents lists available at ScienceDirect Remote Sensing Applications: Society and Environment journal homepage: www.elsevier.com/locate/rsase Investigation of Antalya forest fire's impact on air quality by satellite images using...
Remote Sensing Applications: Society and Environment 29 (2023) 100922 Contents lists available at ScienceDirect Remote Sensing Applications: Society and Environment journal homepage: www.elsevier.com/locate/rsase Investigation of Antalya forest fire's impact on air quality by satellite images using Google earth engine Duygu Arikan *, Ferruh Yildiz Konya Technical University, Engineering and Science Faculty, Geomatics Engineering Department, Konya, Turkiye ARTICLE INFO ABSTRACT Keywords: Several fires occur in Türkiye every year for various reasons. Some of these fires are extinguished within a short period of time. But some of them get bigger due to various factors. Prolonged fires harm the biological ecosystem and nature to a great extent. Gases released into the atmosphere due to the fires negatively affect the life of living things. In this study, the number of fires in the Antalya-Manavgat region in 2021 was determined. For this aim, BAI (Burned Area Index), NDVI (Normalized Difference Vegetation Index), SAVI (Soil Adjusted Vegetation Index) plant indexes were used to determine the amount of fire by using high resolution satellite images. In addition, the carbon monoxide (CO) and ultraviolet aerosol index (UVAI) values during the fire were investigated using satellite images. The precipitation and temperature values of the region between the years 2015–2021 were discussed. The emitted gases were correlated with the national air quality station data and when the destroyed area after the fire was determined by satellite, it was determined that the CO gas released in the fire produced meaningful results with the national air quality station. Forest fire Air quality Carbon monoxide Ultraviolet aerosol index Google earth engine 1. Introduction Forests are natural ecosystems consisting of plant and animal communities, along with organisms that can't be seen with the naked eye. According to the report published by the General Directorate of Forestry, 29.4% of Türkiye is forested as of 2020 (URL 1, GDF). In our country Türkiye, forests are generally located on the Mediterranean, Aegean and Black Sea coastlines. The risk of forest fires is quite high since the summers are dry in the Mediterranean and Aegean regions of our country, which has different climatic diversity (Oğuz et al.2021). When the causes of forest fires between 2001 and 2020 are examined, it is unknown what the causes are for 50% of the fires, whilst the remaining 50% has been determined to be caused by negligence-carelessness, lightning strikes, intention or by accident (Mustafa and Korkmaz, 2021). Forest fires that start for different reasons can spread to a wide area depending on factors such as temperature, wind and precipitation. For example, in July 2002, a forest fire that started with a lightning strike in Quebec, Canada, grew under the influence of strong winds, causing more trees to disappear (URL 2). In addition to these, the occurrence of global warming and climate changes has turned forest fires into a global issue and also affects the ecological balance (Kollanus et al.2016). Because during the fire, certain chemical gases (methane, carbon monoxide, carbon dioxide, nitrogen dioxide, polycyclic aromatic hydrocarbons) emerge and the amount of particulate matter increases. Particulate matter and fine particles less than 2.5 μm in aerodynamic diameter are considered to be the most harmful pollutants for human health (Hänninen et al., 2009; Kollanus et al., 2017). Thus, with the occurrence of forest fires, the fire smoke and the resulting gases negatively affect the air quality. These pollutants emitted into the Corresponding author. E-mail addresses: [email protected] (D. Arikan), [email protected] (F. Yildiz). * https://doi.org/10.1016/j.rsase.2023.100922 Received 30 November 2022; Received in revised form 4 January 2023; Accepted 12 January 2023 Available online 23 January 2023 2352-9385/© 2023 Elsevier B.V. All rights reserved. Remote Sensing Applications: Society and Environment 29 (2023) 100922 D. Arikan and F. Yildiz atmosphere have a certain lifetime, and during this time it is possible for them to be transported long distance by the movement of the wind or air mass (Hänninen et al., 2009). In addition, the size of the fire and the frequency of burning are also factors affecting this. While the studies carried out for the evaluation of small-scale forest fires are efficient, it is difficult to evaluate the damage assessment in large-scale forest fires. It is also costly from an economic point of view (Badarinath et al., 2011). Considering that global warming and climate conditions are changing quickly, faster and more economical solutions must be found for possible forest fires. Thanks to the remote sensing technology, which is used to determine and evaluate the severity of forest fires, the post-fire situation and mapping, the mass detection of burned areas, and the density of gases emitted into the atmosphere (Kollanus et al., 2017; Reid et al., 2016). Remote sensing technology is used not only after at forest fire, but also for the detection of active forest fires (Coppo, 2015). When the studies conducted for this purpose were examined, different satellite images were used. For example, Wooster et al. (2012) used Sentinel-3 satellite data. Seydi et al. (2022), using Landsat satellite imagery, developed the Fire-Net deep learning algorithm for the detection of active fire areas and burning biomass. Higa et al. (2022), utilized the Convolutional Neural Network (CNN) algorithm for object detection and mapping the active fire area using MODIS (Aqua and Terra) satellite sensor data. Although the common purpose of the studies is to determine active forest fires, the data series sets used were different. In parallel with the development of technology, there are various satellites sent to space for different purposes. For example, for air quality analysis or to determine the density of gases emitted into the atmosphere, the 1970 Solar Backscatter Ultra Violet (SBUV) satellite (Tan, 2015), in 1995 the Global Ozone Monitoring Experiment (GOME) satellite, followed by GOME-2 satellite (X. Liu et al., 2005), in 2004 the Ozone Monitoring Instrument” (OMI), and in 2011 the Suomi National Polar-Orbiting Partnership (S-NPP) (Cao et al., 2013) satellite was launched. Finally, in 2017, ESA Copernicus launched the TROPOspheric Monitoring Instrument (TROPOMI), a device connected to the Sentinel5P satellite. Serving the same purpose, this satellite leads the air quality studies today. The device, which uses passive remote sensing technique, offers access to Ozone (O3), Nitrogen Dioxide (NO2), Sulfur Dioxide (SO2), Carbon Monoxide (CO), Methane (CH4), Formaldehyde (HCHO), Cloud, and UV Aerosol Index data in the atmosphere. In this way, it is beneficial for the studies which examine the gases emitted into the atmosphere after forest fires and in the assessment of environmental effects (Xulu et al.2021). Various air pollutants are evaluated from the satellite data used, free of charge, and used in different application fields. There are studies which examine the energy consumption of buildings by utilizing NO2, CO and SO2 pollutants (Ayoobi et al.2022) to detect methane emissions and landfills in mega cities (Sembhi et al., 2022), and to monitor air quality in the COVID-19 period (Velayarce et al., 2022). The purpose of this study is to determine the amount of burned forest area using high resolution Planet satellite images and compare it with different vegetation index. In the second phase, the long-term temperature data via Modis satellite and the CHIRPS meteorological satellite are used to examine the effect of precipitation amount on forest fire events. Finally, the carbon monoxide and ultraviolet aerosol index data were analyzed, and their relations were evaluated using the Google Earth Engine (GEE) platform, obtained from the Sentinel-5 satellite data and through national air quality stations. When the literature was revised, carbon monoxide gas was considered to examine the gas situation in the atmosphere in general during and after the fire. In this study, the ultraviolet index was also examined. Because it contains greenhouse gases and aerosol sources that affect air quality as a result of burning. This practice was carried out to quickly determine the severity of forest fires, and to accelerate decision-making to restore the burned areas and to raise awareness. 2. Material and methods 2.1. Study Area Antalya, located in the south of Türkiye, was chosen as the study area (Fig. 1). Manavgat, the region where the fire was seen, is the second biggest district of Antalya after Kepez. The Mediterranean climate is dominant in the region where it is mild and rainy in the winter and hot and dry in the summer (URL3, Municipality). While the average temperature values are between 28 and 40 in the summer season, it goes down to 10–20 in the winter season. The city, which has a surface area of 20.177 km2, is covered with forests covering an area of 11.460 km2 according to the 2020 Forest Presence report (URL 1, GDF). There are different types of trees (red pine, oak, beech, cedar, pine) according to the heights in the region where the vegetation is maquis. From 2001 to 2020 in Antalya, there was a 12% decrease in the tree cover (WRI, 2022). In Fig. 2, the number of trees lost between 2001 and 2020 in Antalya province is given. The workflow diagram of the study is presented in Fig. 3 and the information about the data used in this study is presented in Table 1. 2.2. The data used in the study In this study, images from the high-resolution Planet satellite, launched on October 31, 2017 were used to map the burn severity. The spatial resolution of the Planet satellite is 3 m, and it has 4 spectral bands: Blue (455–515 nm), Green (500–590 nm), Red (590–670 nm), NIR (780–860 nm). The temporal resolution of the satellite, which consists of 3 main systems, PlanetScope, Skysat and RapidEye constellations, is 1 day. In this way, the images of the same region are updated every day (Şallı, 2020). Images have been downloaded from the planet portal through membership. In the study, it was aimed to use the different spectral indices to determine the fire severity. For this purpose, images from July 19, 2021, before the fire and August 24, 2021, after the fire were used. Although the fire started on July 29 and ended on August 6, the images used were chosen from a wider time period. The main reason for this is the images with minimum cloud cover were preferred. To determine the area of land affected by the fire, the spectral bands of the satellite image were mathematically processed. For this purpose, RED and NIR bands were used to determine the spectral vegetation index of the image, and the indexes and mathematical 2 Remote Sensing Applications: Society and Environment 29 (2023) 100922 D. Arikan and F. Yildiz Fig. 1. Study area. Fig. 2. The amount of lost trees in Antalya between 2001 and 2020 (WRI, 2022). formulas used in Table 2 are given. The 3rd band of the planet satellite represents the red and the 4th band represents the near infrared (NIR). NDVI was developed by Rouse et al. (1974) and is crucial in vegetation monitoring and agricultural applications. Different studies have been carried out to examine vegetation with this index. Such as; predicting biomass productivity (Tucker et al., 1985; Wylie et al., 1995), monitoring and estimating crop productivity (Becker-Reshef et al., 2010), monitoring the growth of trees over a given time period (J. Wang et al., 2004), determining the yield and quality of pasture lands based on current status and seasonal variation (İleri and Koç, 2022), monitoring land cover use and change (Abdullah et al., 2022), and detecting burnt land cover (B. Yılmaz et al., 2022). NDVI takes a value of −1 to +1. A positive value means that the vegetation is greener and more abundant, whereas a negative value indicates that the vegetation is dry and on the brink of extinction (W. Wang et al., 2022). The BAI and SAVI indices are based on the NDVI vegetation model. BAI was developed by Martín in 1998 with the purpose of quickly and accurately identifying and mapping areas damaged by fire (Chuvieco and Martín, 1998; Chuvieco et al., 2002). The basis of BAI is the charcoal signal left over after at fire (Chuvieco et al., 2002). On the other hand, SAVI is an index which was developed to eliminate the change caused by soil types. Therefore, its difference from NDVI is the L coefficient used in the equation. The reflection value of L is a fixed value of 0.5 (Huete, 1988). In the second stage of the study, precipitation and temperature data were analyzed. CHIRPS (Climate Hazards Group Infrared Precipitation with Station) was used to estimate the precipitation time series. The system, co-funded by the U.S. Agency for International Development (USAID), the USAID Famine Early Warning Systems Network (FEWS NET), the National Aeronautics and Space Administration (NASA) and the National Oceanic and Atmospheric Administration (NOAA) was developed by scientists from the USGS Earth Resources Observation and Science (EROS) Center for drought analysis, precipitation determination and forecasting. With more than 30 years of precipitation data, CHIRPS combines 0.05° resolution satellite imagery and station data (Funk et al., 2015). In this study, daily data between 2015 and 2021 was downloaded and used with Version 2.0. 3 Remote Sensing Applications: Society and Environment 29 (2023) 100922 D. Arikan and F. Yildiz Fig. 3. The workflow diagram. Table 1 Information about the data used in the study. Purpose Data Dataset Availability Resolution/ Scale Source Burned Area Mapping and Visualization Pollution Mapping Pollution Mapping Pollution Monitoring Planet July 29, 2021 and Aug 07, 2021 29 July – 06 Aug 2, 0,211 29 July – Aug 06, 2021 26 July – Aug 10, 2021 3m www.planet.com 1113.2 m 1113.2 m 7 station Jan 2015–Dec 2021 Jan 2015–Dec 2021 5566 m 1000 m GEE GEE http:// www.havaizleme.gov.tr GEE GEE Rainfall Temperature Sentinel5P Carbon Monoxide Sentinel5P Aerosol Index National Weather MonitoringStation CHIRPS MODIS Terra LST Table 2 Vegetation indices and their mathematical formulas. Abbreviation Spectral Index Formula NDVI Normalized Difference Vegetation Index NDVI = BAI Burned Area Index BAI = SAVI Soil-adjusted Vegetation Index SAVI = NIR−RED NIR+RED 1 (0.1−RED)2 +(0.06−NIR)2 (1+L)(NIR−RED) (NIR+Red+L) Terra Land Surface Temperature (LST) data set of the MODIS satellite (MOD11A1.006) was used for temperature data. The spatial resolution of the satellite, which allows daily data collection, is 1 km. Whilst the v.6 model has been in use since 12/16/2012, it has been announced that v6.1 will be used in the near future (URL, 4). Temperature, precipitation, CO and UVAI data were acquired from the Google Earth Engine (GEE) platform. Since it is web-based, operations can be carried out independently from the computer (Yaman and Görmüş, 2022). The application is developed in Python and Javascript scripting languages (Arıkan and Yıldız, 2022). There is free access (O. S. Yılmaz et al., 2021). In the third stage of the study, the air quality in areas around forest fires was examined. Because the amount of air pollution is increasing in regions where severe forest fires are seen (Heil and Goldammer, 2001; J. C. Liu et al., 2015). The main reason for this situation is that with combustion, some chemical gases are released after compounds found in nature react with each other. These gases can be natural gases such as carbon dioxide, carbon monoxide, sulfur dioxide, nitrogen and derivatives, as well as toxic gases such as formaldehyde, methane or acetic acid. Carbon monoxide, which is released as a result of lack of oxygen or incomplete combustion of carbon dioxide, affects regional air quality (Singh et al., 2021). This is a situation which directly or indirectly affects human health and the environment. Fine particulate particles from smoke pose a threat to human health. According to a study by Johnston et al. (2011), it is stated that smoke from forest fires causes early death of approximately 300,000 people a year. Increasing air pollution due to fires cause health problems such as asthma, respiratory disorders such as the onset of chronic obstructive pulmonary disease (COPD) and eye irritation in individuals 4 Remote Sensing Applications: Society and Environment 29 (2023) 100922 D. Arikan and F. Yildiz (Reid et al., 2016). When the literature is scanned, forest fires and gases affecting air quality have been examined in various studies. The criteria used for the purpose of the study and the data with which it was conducted are presented in Table 3. Changes in CO, NO2 and particulate matter were mostly investigated in forest fires. In this study, in addition to carbon monoxide data and data obtained from air quality monitoring stations, ultraviolet aerosol index data was also examined. It contains greenhouse gases and the aerosol source, which plays an important role in the air quality of forest fires. This gas cloud, known as the Absorbent Aerosol Index, is mostly used in biomass combustion to track the evolution of volcanic ash that occurs following volcanic eruptions. The difference of the aerosol index from other gases, is that it can be calculated by deriving pixels even in a cloudy environment. The wavelength used in UVAI is calculated using the wavelength pair between 340 and 380 nm of the spectral band and is expressed as unitless (URL, 5). In order to analyze the effect of gases released as a result of the fire on air quality, Sentinel-5P satellite data was exported via GEE and maps were developed. The Sentinel-5P satellite was sent on October 13, 2017 by the European Space Agency to monitor air quality. Thanks to the TROPOMI sensor on the satellite, the values emitted by different gases can be measured. For this purpose, the data of carbon monoxide and the ultraviolet aerosol index gases emitted between the dates of July 29, 2021–August 6, 2021, when the fire occurred, were downloaded. The number of bands in the carbon monoxide data of Sentinel 5P satellite is 8, while the number of bands in the ultraviolet aerosol index data is 6. It is possible to access real-time and offline data of all the gases except for methane. The data used in the study is the offline data. Downloading and exporting of the data was done via GEE because it is a cloud platform that can make scientific analysis quickly and provides free access to users (Gorelick et al., 2017). The maps of the downloaded images are made in ArcGIS. In addition, data gathered from national air quality monitoring stations and images obtained from the GEE were compared and interpreted. The monitoring of air quality values in the country, the number of stations and their working status are carried out by the Turkish Ministry of Environment and Urbanization. The first measurements were the data obtained from Samsun and Erzurum in 2015. While these two stations collect only SO2 and Particulate matter data at first, later on, air quality index values are also calculated using the data of 5 air pollutants (particulate matter, CO, NO2, SO2, O3). As of 2022, the number of stations in Türkiye is 340 (URL4). The data is obtained instantaneously from the fields are divided into 6 classes as good (0–50), moderate (50–100), sensitive (100–150), unhealthy (150–200), bad (200–300) and dangerous (300–500) and are broadcasted hourly. 3. Results In July and August, forest fires occurred in several regions in Türkiye. In the study, satellite images were used to detect the forest fires in Antalya. The downloaded planet satellite images were processed by ESA via The Sentinel Application Platform (SNAP), which is free to use. Different index images (NDVI, BAI, and SAVI) were created with the Raster/Band Maths process. It was determined that Antalya Manavgat, was where the fire was mostly seen, and these images were then divided. Afterwards, maps were produced in ArcGIS and NDVI, BAI and SAVI are presented in Fig. 4, Fig. 5, Fig. 6, respectively. The NDVI value is directly related to the growth and health of the plants. Fading or even the death of vegetation reflects negatively on the NDVI profile. Pre-fire and post-fire NDVI values were calculated mathematically, and their maps are shown in Fig. 4. AccordTable 3 Pollutant gases used in air quality studies and for what purpose they are used. Reference The Criterion Used Used Data Purpose Dempsey (2013) Sannigrahi et al. (2022) CO Particulate matter (PM 2.5 and PM 10), NO2 MODIS Air quality data available for the study area Forecasting air pollution Examining the effects on health İban and Şahin (2021) CO, formaldehyde, SO2 and O3 MODIS, Sentinel 2A and Sentinel 5P Analyzing land cover and atmosphere data Hänninen et al. (2009) PM2.5 and PM10 Examining the effects on health, Yao and Henderson (2014) PM2.5 Carvalho et al. (2011) O3 and PM10 Available air quality data for the study area (Finland) PM2.5, Aerosol Optical Depth from MODIS satellite, The Hazard Mapping System Datasets, Fire Danger Rating values, Daily forecasts of the venting index from NOAA satellite Available air quality data for the study area Sannigrahi et al. (2020) C, CO2, CH4, NO2, NOx and particulate matter Assessing the impact on the environment Isaev et al. (2002) Carbon emissions MODIS satellite Land Surface Temperature dataset, Quantification of net primary productivity POT/HRVIR and RESURS-O/MSU-E images. Kasischke and Bruhwiler (2002) Singh and Kansal (2022) Reahard et al. (2010) CO2, CO and CH4 Analysis of the AVHRR imagery CO and NOx MODIS satellite land surface temperature, Sentinel-2 ASTER, Landsat 5 and MODIS Xulu et al. (2021) CO CO2, CO, CH4, NO2, SO2, Particulate matter (PM2.5, PM10), Pb Sentinel-2, Sentinle-5 Modeling the regions that cannot be measured Investigating the effects on emissions To be able to detect damage and predict carbon emissions Estimating the current state of carbon emissions Analyzing changes and estimating the effect of gases on air quality Assessing air quality and examining health risks to human populations Examining the CO status 5 Remote Sensing Applications: Society and Environment 29 (2023) 100922 D. Arikan and F. Yildiz Fig. 4. NDVI image before and after fire. Fig. 5. BAI image before and after fire. ing to the post-fire NDVI value, the ground was completely covered with dead vegetation in an area of 49,5344 km2, while the unhealthy vegetation affected by the fire corresponds to an area of 65,3281 km2. The total area of vegetation affected by the fire is 194,4382 km2. In addition, the obtained NDVI vegetation index map was evaluated with an error matrix (Ok et al., 2012). To do this, it is divided into 5 classes as High Severity Burned Area, Moderate High Severity Burned Area, Moderate Low Severity Burned Area, Low Severity Burned Area, Unburned area, and its overall accuracy, kappa accuracy, and producer accuracy of each class were calculated (Table 4). 500 points were used for testing. According to the result of the error matrix obtained, the overall accuracy of the classifications was determined as 85% and the kappa value as 81.25% (Table 4). When evaluated on an individual class basis, all classes except Moderate High Severity are over 85% accurate for user accuracies. On the other hand, accuracy is the lowest in High Severity at 61%. The reason for the low accuracy of High Severity and Moderate High Severity is thought to be due to the amount of burn intensities. BAI index was calculated using the mathematical model given in Table 2. The area of the High Severity burned area was 97.32 km2, the Moderate High Severity burned area was 24.50 km2 and the Moderate Low Severity burned area was 1.27 km2, with a total of 123.09 km2 destroyed. In the studies carried out, the SAVI vegetation index was used to determine the drought (Aziz and Nugraha, 2022). The fires that occur not only change the vegetation but also some of the chemical properties of the soil. For this reason, the SAVI index was calculated in order to reduce the soil effect in the burned areas and the area changes in the soil after the fire were determined. In the region 6 Remote Sensing Applications: Society and Environment 29 (2023) 100922 D. Arikan and F. Yildiz Fig. 6. SAVI image before and after fire. Table 4 Overall accuracy and kappa accuracy of NDVI vegetation. Burning Area High Severity Moderate High Severity Moderate Low Severity Low Severity Unburned Area Total User's Accuracy (%) High Severity Moderate High Severity Moderate Low Severity Low Severity Unburned Area Total Producer's Accuracy (%) Overall Accuracy (%) Kappa (%) 61 27 9 3 0 100 0.61 0.85 0.81 5 93 2 0 0 100 0.93 4 6 85 2 3 100 0.85 0 0 0 100 0 100 1 0 0 2 12 86 100 0.86 70 126 98 117 89 500 0.87 0.74 0.87 0.85 0.97 where the High Severity fire was experienced, an area of 37.31 km2 was affected, in the Moderate High Severity burned area this is 72.56 km2, and in the Moderate Low Severity burned area this is 84.17 km2. There is a total change of 194.04 km2. With the effect of global warming, there is a decrease in precipitation and an increase in the temperature. This situation leads to drought. Increasing droughts is one of the main factors that triggers forest fires. Singh et al. (2021) in their study conducted, they stated that the increase in dryness and temperature conditions causes an increase in forest fires and prolongs the duration of the fires. For this reason, precipitation and temperature conditions were examined from 2015 to the year of the fire. Rainfall data of CHIRPS satellite was analyzed via GEE and recorded in ‘csv’ format. The monthly precipitation for the Antalya region between the years of 2015 and 2021 is shown in Fig. 7. In general, the amount of precipitation increases in December and Janu- Fig. 7. Total amount of precipitation falling on Antalya between 2015 and 2021. 7 Remote Sensing Applications: Society and Environment 29 (2023) 100922 D. Arikan and F. Yildiz ary, while it decreases in July and August, which is the summer season. While the average precipitation amount in January is 185 mm, this amount decreases to as low as 17 mm in July. While the annual precipitation was 738 mm in 2015, it is 618 mm in 2021. In this direction, there was a decrease of 16.27% in the precipitation. There are changes in precipitation patterns due to global warming. The decrease in the amount of precipitation from year to year leads to drought. Average annual land surface temperature data from the MODIS satellite was downloaded via GEE. These data are daily and MOD11A1.006 Terra dataset was used. In line with these data, the monthly temperature situation for the Antalya region for the years 2015 and 2021 is shown in Fig. 8. When examined annually, the land surface temperature decreases in the winter months and this temperature value is observed as 35° on average in the summer months. From 2015 to 2021, the temperature rates increased. There were fluctuations in temperature changes only in 2016 and 2018. Moreover, it was determined that the land surface temperature in March 2021 was lower than the other years. When all years are compared, the month which has the highest land surface temperature is August. There is a significant positive correlation between MODIS LST values and the values obtained from the CHIRPS precipitation data. This situation leads to droughts as precipitation decreases and the temperature values increase. When the meteorological results are evaluated, situations such as droughts create a suitable environment for forest fires. The fire in Antalya started on July 29, 2021 and the fires were brought under control on August 06, 2021. But during this time, most of the forest areas were burned. Furthermore, gases that increase air pollution were also released. Satellite images of the Sentinel-5P satellite were downloaded to determine the density of CO and UVAI gases on the specified dates. CO maps are presented in Fig. 9 and UVAI maps are presented in Fig. 10. The date on which carbon monoxide is the most visible is 29 July, the day the fire started. The maximum density value measured on this date is 0.1556 mol/m2. The average value determined from the Carbon Monoxide Column Number Densities throughout the fires in Antalya was found to be 0.034–0.040 mol/m2. On the day of the fire, this value was on average 0.084 mol/m2 only in Manavgat. While the average value was 0.083 mol/m2 on 30 July, this value decreased by 20.49% as the fires were brought under control on 31 July. The carbon monoxide column number density for the Manavgat region decreased to 0.043 mol/m2 on August 5, the date the fire ended. Similarly, when UVAI maps are examined, it is more widely distributed than CO. The UV absorbing aerosol index value was calculated, with a maximum level of 5.6189 in the parts where the fire was seen. Whilst the aerosol index value for Antalya was at a minimum value of 0.383 on July 29, when the fire occurred, these values increased gradually in the following days. On July 30, this value increased by 40.46% to 0.538, and on July 31 to reach 1.034, which shown an increase of 170%. With the fires being brought under control on July 31, the aerosol index value dropped to 0.785 on August 1. By August 5, 2021, the aerosol index value had decreased back to the minimum levels. However, on August 5, this value showed a sudden increase, and the absorbing aerosol index value was calculated as 2.16. When the UVAI image of this date was examined, multiple fires were occurring in various parts of Muğla province in the western part. This is the main reason for the increase in the amount of UVAI in the western part. In addition to satellite data, quality index values for Antalya and its surroundings were downloaded from Türkiye's national air quality monitoring stations to analyze the changes in the air quality index over the same period. The air quality data presented in Fig. 11 have been downloaded to include 3 days before the fire (July 26, 2021) and 2 days after the fire (August 08, 2021). The main reason for this is to better reflect the air quality situation in Antalya and its surrounding districts. The value recorded in Manavgat on July 29, when the fire broke out, was 212.14 mg/m3. While the air quality values were approximately 35 mg/m3 for the same area before the fire, these values increased during and after the fire. Although the fire broke out in the Manavgat region, it was observed that there was an increase in the air quality values in the surrounding districts as well. Ac- Fig. 8. Antalya land surface temperature between 2015 and 2021. 8 Remote Sensing Applications: Society and Environment 29 (2023) 100922 D. Arikan and F. Yildiz Fig. 9. Maps produced from CO data from the date of fire start. cording to the nationally determined air quality indices, it was observed that the good situation before the fire increased to sensitive levels after the fire. In the table given in Fig. 11, it is seen that there is a value of 0 in some parts, which means that there is no calculated air quality index data for that day and the station. 4. Discussion Different vegetation indices were examined in this study. It was determined that NDVI and SAVI vegetation had more similar results compared to the values obtained from the BAI index. İban and Şahin (2021), Singh and Kansal (2022), Xulu et al. (2021) in their studies, they examined the CO data from satellite images. In this study, the CO data were obtained from the Sentinel satellite image and it was found to be compatible with the national air quality station. For this reason, UVAI data were also examined. The situation at the time of the fire was examined with carbon monoxide and ultraviolet aerosol index data from the Sentinel-5P satellite. As can be seen in Fig. 9, carbon monoxide started to disperse in the atmosphere during and after the fire because of its gas feature. A large part of the carbon monoxide gas moved in the North-West direction with the effect of the wind. Information on wind speed and direction was collected from URL 6. Accordingly, on 29, 30 and, 31 July, the wind speed was approximately 20–30 km/h and heavy breezes were experienced between 6:00–12:00. Between 6:00 and 12:00 on July 29 and 30, the wind blew to the north. Then it turned its direction first to the south and then to the west. On July 31, the wind direction was westward between 3:00 and 9:00, and it turned northward between 9:00–12:00. On August 1, the wind speed was measured around 40 km/h. While the direction of the wind, which was strong between 9:00 and 12:00, was towards the north, it turned southwards after 15.00. As of August 2, the 9 Remote Sensing Applications: Society and Environment 29 (2023) 100922 D. Arikan and F. Yildiz Fig. 10. Maps produced from UVAI data from the date of fire start. Fig. 11. Data from national air quality stations (URL 6). 10 Remote Sensing Applications: Society and Environment 29 (2023) 100922 D. Arikan and F. Yildiz speed of wind had decreased to 10 km per hour. The gases that emerged during the fire spread faster this way. But it is also a disadvantage. Because wind is one of the factors that increase the severity of the fire. When Fig. 10 is examined, the carbon monoxide images were also valid for UVAI. As of August 1, the index value has decreased by 0.785. However, on August 5, this value showed a sudden increase, and the absorbing aerosol index value was calculated as 2.16. When the UVAI image of this date was examined, it was seen that fires had occurred in various parts of Muğla province located towards the west. This is the main reason for the increase in the amount of UVAI in the western part. Besides, it is observed that the amount of pollutants increased in the north of Antalya on August 02, 2021. This can especially be seen in the ultraviolet aerosol index map. The increase in pollution here is due to the cement factory in the region. Because such factories cause an increase in the amount of nitrogen dioxide, carbon monoxide, dust and particulate matter. This causes harm to public health, agriculture, and the environment. The visuals given in Figs. 12 and 13 are detailed representations of Figs. 9 and 10. According to the map, the carbon monoxide density increased rapidly in the Manavgat region, where the fire started. The density of the carbon monoxide columns measured on this date is 0.1556 mol/m2. As can be seen from the map, it was observed that the pollutant density changed as Fig. 12. Detailed status of the carbon monoxide map of July 29, 2021. Fig. 13. Detailed status of aerosol index map of July 29, 2021. 11 Remote Sensing Applications: Society and Environment 29 (2023) 100922 D. Arikan and F. Yildiz 0.1359–0.1556 mol/m2 on the day of the fire. In addition, it has been observed that the carbon monoxide concentration in urban areas is higher than in rural areas. This is due to the incomplete combustion of carbon in the structure of fuels. In Fig. 13, detailed representation of the Aerosol index map of July 30, 2021 is given. The ongoing fire in Manavgat can be seen in Fig. 13.b. The carbon monoxide density value in this part specifically is 0.109 mol/m2, while the aerosol index value is 3.696. On the same date, a small fire started near the Manavgat region and was brought under control in about 5 days. The carbon monoxide concentration in Fig. 13.c. is 0.085 mol/m2 and the aerosol index value is 3.235. While the average carbon monoxide density of Antalya for today was 0.038 mol/m2, the aerosol index value was 0.538. In Fig. 13a, the information from URL6 is confirmed and it is clearly seen that air pollutants are moving in the southwest direction with the effect of the wind. 5. Conclusions One of the main sources of vegetation loss and increase in air pollution is forest fires. In this study, it is understood that it is possible to detect and monitor the CO gas and aerosol index that occurs in the event of a forest fire using remote sensing satellites. At the same time, it is seen that it is possible to make analyses such as whether the plant status is healthy or not, the area determination as a result of the fire, and the soil condition by using different indices. With the study, it is seen that forest fires do not only affect the ecosystem, but also directly affect the local flora and fauna and its environment. For this purpose, it is necessary to control the spread of forest fires for human health and the environment and to minimize the damage that may occur. It may be impossible for us to control nature, but it is possible to protect nature by taking precautions. Declarations All authors have read, understood, and have complied as applicable with the statement on “Ethical responsibilities of Authors” as found in the Instructions for Authors and are aware that with minor exceptions, no changes can be made to authorship once the paper is submitted. Funding statement The authors declare that they have no known competing financial interests or personal relationships that could have appeared to influence the work reported in this paper. Author contribution Duygu ARIKAN: Methodology and application, Writing- Original draft preparation, Visualization, Investigation, Ferruh YILDIZ: Writing- Reviewing and Editing. All authors discussed the results and contributed to the final manuscript. Ethical statement for remote sensing applications: society and environment 1) 2) 3) 4) 5) 6) This material is the authors' own original work, which has not been previously published elsewhere. The paper is not currently being considered for publication elsewhere. The paper reflects the authors' own research and analysis in a truthful and complete manner. The paper properly credits the meaningful contributions of co-authors and co-researchers. The results are appropriately placed in the context of prior and existing research. All sources used are properly disclosed (correct citation). Literally copying of text must be indicated as such by using quotation marks and giving proper reference. 7) All authors have been personally and actively involved in substantial work leading to the paper, and will take public responsibility for its content. Declaration of competing interest The authors declare that they have no known competing financial interests or personal relationships that could have appeared to influence the work reported in this paper Data availability Data will be made available on request. Acknowledgments The authors would like to thank Google Earth Engine platforms, Sentinel Data Hub and Planet Scopus for providing data. References Abdullah, S., Barua, D., Abdullah, S., Abubakar, M., Rabby, Y.W., 2022. Investigating the impact of land use/land cover change on present and future land surface temperature (LST) of Chittagong, Bangladesh. Earth Systems and Environment 6 (1), 221–235. Arıkan, D., Yıldız, F., 2022. Landsat-8 Uydusu Kullanılarak 2018-2021 Yılları Arasında İstanbul’daki Kentsel Isı Adasının İncelenmesi. pp. 362–372. Ayoobi, A.W., Ahmadi, H., Inceoglu, M., Pekkan, E., 2022. Seasonal impacts of buildings’ energy consumption on the variation and spatial distribution of air pollutant 12 Remote Sensing Applications: Society and Environment 29 (2023) 100922 D. Arikan and F. Yildiz over Kabul City: application of Sentinel—5P TROPOMI products. Air Quality, Atmosphere Health 15 (1), 73–83. Aziz, Y.A., Nugraha, A.S.A., 2022. Comparison of vegetation index method to detect drought in bondowoso regency, east java. Media Komunikasi FPIPS 21 (1), 93–98. Badarinath, K., Sharma, A., Kharol, S., 2011. Forest fire monitoring and burnt area mapping using satellite data: a study over the forest region of Kerala State, India. Int. J. Rem. Sens. 32 (1), 85–102. Becker-Reshef, I., Vermote, E., Lindeman, M., Justice, C., 2010. A generalized regression-based model for forecasting winter wheat yields in Kansas and Ukraine using MODIS data. Remote Sensing of Environ. 114 (6), 1312–1323. Cao, C., De Luccia, F.J., Xiong, X., Wolfe, R., Weng, F., 2013. Early on-orbit performance of the visible infrared imaging radiometer suite onboard the Suomi National Polar-Orbiting Partnership (S-NPP) satellite. IEEE Trans. Geosci. Rem. Sens. 52 (2), 1142–1156. Carvalho, A., Monteiro, A., Flannigan, M., Solman, S., Miranda, A.I., Borrego, C., 2011. Forest fires in a changing climate and their impacts on air quality. Atmos. Environ. 45 (31), 5545–5553. Chuvieco, E., Martín, M.P., 1998. Cartografía de grandes incendios forestales en la Península Ibérica a partir de imágenes NOAA-AVHRR. Chuvieco, E., Martin, M.P., Palacios, A., 2002. Assessment of different spectral indices in the red-near-infrared spectral domain for burned land discrimination. Int. J. Rem. Sens. 23 (23), 5103–5110. Coppo, P., 2015. Simulation of fire detection by infrared imagers from geostationary satellites. Remote Sensing of Environ. 162, 84–98. Dempsey, F., 2013. Forest fire effects on air quality in Ontario: evaluation of several recent examples. Bull. Am. Meteorol. Soc. 94 (7), 1059–1064. Funk, C., Peterson, P., Landsfeld, M., Pedreros, D., Verdin, J., Shukla, S., Hoell, A., 2015. The climate hazards infrared precipitation with stations—a new environmental record for monitoring extremes. Sci. Data 2 (1), 1–21. Gorelick, N., Hancher, M., Dixon, M., Ilyushchenko, S., Thau, D., Moore, R., 2017. Google earth engine: planetary-scale geospatial analysis for everyone. Remote Sensing of Environ. 202, 18–27. Hänninen, O.O., Salonen, R.O., Koistinen, K., Lanki, T., Barregard, L., Jantunen, M., 2009. Population exposure to fine particles and estimated excess mortality in Finland from an East European wildfire episode. J. Expo. Sci. Environ. Epidemiol. 19 (4), 414–422. Heil, A., Goldammer, J., 2001. Smoke-haze pollution: a review of the 1997 episode in Southeast Asia. Reg. Environ. Change 2 (1), 24–37. Higa, L., Marcato Junior, J., Rodrigues, T., Zamboni, P., Silva, R., Almeida, L., Gonçalves, W.N., 2022. Active fire mapping on Brazilian pantanal based on deep learning and CBERS 04A imagery. Rem. Sens. 14 (3), 688. Huete, A.R., 1988. A soil-adjusted vegetation index (SAVI). Remote Sensing of Environ. 25 (3), 295–309. İban, M.C., Şahin, E., 2021. Monitoring burn severity and air pollutants in wildfire events using remote sensing data: the case of Mersin wildfires in summer 2021. Gümüşhane Üniversitesi Fen Bilimleri Dergisi 12 (2), 487–497. İleri, O., Koç, A., 2022. Monitoring the available forage using Sentinel 2-derived NDVI data for sustainable rangeland management. J. Arid Environ. 200, 104727. Isaev, A., Korovin, G., Bartalev, S., Ershov, D., Janetos, A., Kasischke, E., Murphy, T., 2002. Using remote sensing to assess Russian forest fire carbon emissions. Climatic Change 55 (1), 235–249. Johnston, F., Hanigan, I., Henderson, S., Morgan, G., Bowman, D., 2011. Extreme air pollution events from bushfires and dust storms and their association with mortality in Sydney, Australia 1994–2007. Environ. Res. 111 (6), 811–816. Kasischke, E.S., Bruhwiler, L.P., 2002. Emissions of carbon dioxide, carbon monoxide, and methane from boreal forest fires in 1998. J. Geophys. Res. Atmos. 107 (D1).. FFR 2-1-FFR 2-14. Kollanus, V., Tiittanen, P., Niemi, J.V., Lanki, T., 2016. Effects of long-range transported air pollution from vegetation fires on daily mortality and hospital admissions in the Helsinki metropolitan area, Finland. Environ. Res. 151, 351–358. Kollanus, V., Prank, M., Gens, A., Soares, J., Vira, J., Kukkonen, J., Lanki, T., 2017. Mortality due to vegetation fire–originated PM2. 5 exposure in Europe—assessment for the years 2005 and 2008. Environ. Health Perspect. 125 (1), 30–37. Liu, X., Chance, K., Sioris, C., Spurr, R., Kurosu, T., Martin, R., Newchurch, M., 2005. Ozone profile and tropospheric ozone retrievals from the Global Ozone Monitoring Experiment: algorithm description and validation. J. Geophys. Res. Atmos. 110 (D20). Liu, J.C., Pereira, G., Uhl, S.A., Bravo, M.A., Bell, M.L., 2015. A systematic review of the physical health impacts from non-occupational exposure to wildfire smoke. Environ. Res. 136, 120–132. Mustafa, A., Korkmaz, M., 2021. Türkiye’de orman yangını sorunu: Güncel bazı konular üzerine değerlendirmeler. Turkish J. Forest. 22 (3), 229–240. Oğuz, K., Oğuz, E., Çamalan, G., 2021. İzmir-Tırazlı orman yangınının uydu ve model verileri ile analizi. Ulusal Çevre Bilimleri Araştırma Dergisi 4 (1), 1–12. Ok, A.O., Akar, O., Gungor, O., 2012. Evaluation of random forest method for agricultural crop classification. Europ. J. Rem. Sens. 45 (1), 421–432. Reahard, R., Clark, R., Robin, C., Zeringue, J., McCarty, J., 2010. Louisiana Air Quality-Using ASTER, Landsat 5, and MODIS to Assess the Impact of Sugarcane and Marsh Burning Practices on Local Air Quality. (Paper presented at the AGU Fall Meeting Abstracts). Reid, C.E., Jerrett, M., Tager, I.B., Petersen, M.L., Mann, J.K., Balmes, J.R., 2016. Differential respiratory health effects from the 2008 northern California wildfires: a spatiotemporal approach. Environ. Res. 150, 227–235. Rouse, Jr, J., Haas, R.H., Deering, D., Schell, J., Harlan, J.C., 1974. Monitoring the Vernal Advancement and Retrogradation (Green Wave Effect) of Natural Vegetation. (Retrieved from). Şallı, B., 2020. Random Forest Classification of Tomato Fields with Planet Satellite Image Data and Accuracy Assessment. Institute of Science And Technology. Sannigrahi, S., Pilla, F., Basu, B., Basu, A.S., Sarkar, K., Chakraborti, S., Bhatt, S., 2020. Examining the effects of forest fire on terrestrial carbon emission and ecosystem production in India using remote sensing approaches. Sci. Total Environ. 725, 138331. Sannigrahi, S., Pilla, F., Maiti, A., Bar, S., Bhatt, S., Zhang, Q., Cerda, A., 2022. Examining the status of forest fire emission in 2020 and its connection to COVID-19 incidents in West Coast regions of the United States. Environ. Res. 210, 112818. Sembhi, H., Boesch, H., Villena, C.R., Guillo, R.B., Trent, T., Kunchula, R.K., Schneising, O., 2022. Estimating Landfill Methane Emissions in Indian Megacities with Sentinel 5p TROPOMI. (Retrieved from). Seydi, S.T., Saeidi, V., Kalantar, B., Ueda, N., Halin, A.A., 2022. Fire-Net: a deep learning framework for active forest fire detection. J. Sens. vol. 2022, Article ID 8044390, 14 pages, 2022. https://doi.org/10.1155/2022/8044390. Singh, S., Kansal, M.L., 2022. Chamoli flash-flood mapping and evaluation with a supervised classifier and NDWI thresholding using Sentinel-2 optical data in Google earth engine. Earth Sci. Inform. 1–14. Singh, S., Singh, H., Sharma, V., Shrivastava, V., Kumar, P., Kanga, S., Singh, S.K., 2021. Impact of forest fires on air quality in Wolgan Valley, New South Wales, Australia—a mapping and monitoring study using Google Earth engine. Forests 13 (1), 4. Tan, S.-Y., 2015. NOAA satellites and solar backscatter Ultra violet (SBUV) subsystems national oceanic and atmospheric administration (NOAA) solar backscatter Ultra violet (SBUV). Handbook Cosmic Hazard. Planetary Defense 449–486. Tucker, C.J., Vanpraet, C.L., Sharman, M., Van Ittersum, G., 1985. Satellite remote sensing of total herbaceous biomass production in the Senegalese Sahel: 1980–1984. Remote Sensing of Environ. 17 (3), 233–249. URL 1, 2020. General Directorate of Forestry Report. Access 18.February.2022. https://www.ogm.gov.tr/tr. URL 2. Access 23.February.2022. https://cimss.ssec.wisc.edu/gWRIoes/misc/020628/020628.html. URL 3. Access 18.February.2022. https://www.antalya.bel.tr/. URL 4. Access 21.February.2022. https://lpdaac.usgs.gov/products/mod11a1v006/. URL 5. Access 04.April.2022. https://sentinel.esa.int/web/sentinel/technical-guides/sentinel-5p/level-2/aerosol-index. URL 6. Access 26.February.2022. http://www.havaizleme.gov.tr. Velayarce, D., Bustos, Q., García, M.P., Timaná, C., Carbajal, R., Salvatierra, N., Murray, V., 2022. Air quality analysis in Lima, Peru using the NO2 levels during the COVID-19 pandemic lockdown. Atmosphere 13 (3), 373. Wang, J., Rich, P., Price, K., Kettle, W., 2004. Relations between NDVI and tree productivity in the central Great Plains. Int. J. Rem. Sens. 25 (16), 3127–3138. Wang, W., Hu, P., Yang, Z., Wang, J., Zhao, J., Zeng, Q., Yang, Q., 2022. Prediction of NDVI dynamics under different ecological water supplementation scenarios based on a long short-term memory network in the Zhalong Wetland, China. J. Hydrol. 608, 127626. Wooster, M.J., Xu, W., Nightingale, T., 2012. Sentinel-3 SLSTR active fire detection and FRP product: pre-launch algorithm development and performance evaluation 13 Remote Sensing Applications: Society and Environment 29 (2023) 100922 D. Arikan and F. Yildiz using MODIS and ASTER datasets. Remote Sensing of Environ. 120, 236–254. Wylie, B.K., Denda, I., Pieper, R.D., Harrington, Jr, J.A., Reed, B.C., Southward, G.M., 1995. Satellite-based herbaceous biomass estimates in the pastoral zone of Niger. J. Range Manag. Xulu, S., Mbatha, N., Peerbhay, K., 2021. Burned area mapping over the southern cape Forestry region, South Africa using Sentinel data within GEE cloud platform. ISPRS Int. J. Geo-Inf. 10 (8), 511. Yaman, Ş., Görmüş, E.T., 2022. Orman zararlılarının verdiği zararın Google earth engine kullanılarak i?zlenmesi. Turkish J. Rem. Sens. GIS 3 (2), 139–149. Yao, J., Henderson, S.B., 2014. An empirical model to estimate daily forest fire smoke exposure over a large geographic area using air quality, meteorological, and remote sensing data. J. Expo. Sci. Environ. Epidemiol. 24 (3), 328–335. Yılmaz, O.S., Oruç, M.S., Ateş, A.M., Gülgen, F., 2021. Orman yangın siddetinin Google earth engine ve coğrafi bilgi sistemleri kullanarak analizi: hatay-belen örneği. J. Inst. Sci. Technol. 11 (2), 1519–1532. Yılmaz, B., Demirel, M., Balçık, F., 2022. Yanmış alanların sentinel-2 MSI ve landsat-8 OLI ile tespiti ve analizi: çanakkale/gelibolu orman yangını. Doğal Afetler ve Çevre Dergisi 8 (1), 76–86. 14