Quantitative Analyses - PSY260 Laboratory - PDF
Document Details
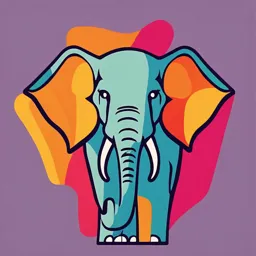
Uploaded by WellWishersConnemara2829
Klein Independent School District
Tags
Summary
This document is a laboratory workshop analyzing Quantitative Analyses. It covers topics such as descriptive and inferential statistics. It explores data collection methods, assumption checks, and statistical tests like t-tests, Pearson correlations, and Chi-Square tests. The session will also compare JASP and SPSS data analysis software.
Full Transcript
Quantitative Analyses So Far PSY260: Laboratory Workshop 2 24/25 In today’s session: – Revise the different analyses covered in PSY140 – Get to grips with data again! Comparing JASP and SPSS (for our portfolios) Getting to Know Your Data ...
Quantitative Analyses So Far PSY260: Laboratory Workshop 2 24/25 In today’s session: – Revise the different analyses covered in PSY140 – Get to grips with data again! Comparing JASP and SPSS (for our portfolios) Getting to Know Your Data A note from last year (and last week) – In PSY140, you covered observation studies, surveys, and experiments – People often think that each design has set analyses (e.g. you ran a Chi Square analysis for your observation study, so these must always go together) – But it is more the type of data that you collect, and whether you’re focused on differences or relationships, that guides what analyses can be conducted! – So, familiarising yourself with data (and the different terms and distinctions) should help with conducting the right analysis! Your Data – We collect raw data that we need to clean (i.e. data wrangling) before analysis – The analysis we can do depends on many factors (i.e. ‘Assumption Checks’): – the kind of study (e.g. were we studying differences or relationships?) – What type of data we have (is it continuous or discrete?) – And other parameters (e.g. is our data normal?) – These assumptions can determine what descriptive and inferential data we use too (more on these shortly) – But we should generally know which analysis we need before we’ve collected any data Collecting Data for our Study Before we collect any data, we should know what level our data will be Levels include: – Nominal; e.g. favourite ice cream – Ordinal; e.g. ranking of different ice creams – Interval; e.g. rating the temperatures of different ice creams – Ratio; e.g. rating how much we like different ice creams on a scale of 1-10 N.B. the level doesn’t just refer to the DV Our example from last week – Remember, we’re looking at whether different personality traits (light triad vs. dark triad) are linked to engagement with dark tourism (desire to visit) – We have the potential to collect discrete data: – categorise Pp as either scoring higher on the light triad or the dark – categorise Pp as either wanting to visit or not – But we can also collect continuous data – score people on the different personality traits – ask Pp how much (on a scale of 1-10) they’d like to visit a dark site Assumption Checks After Data Collection – We need to take some steps before analysing our cleaned data Skew is – we the more want to important of check whether our data is parametric (translation: does theour two, data satisfy but kurtosis different refers to the tails of a distribution requirements?) or non-parametric – We need to make sure our levels of data are correct (theLeptokurtic software usually = tall and thin assumes correctly) Platykurtic = short and wide – Whilst there are different assumptions to check (we’ll introduce new ones throughout the year), we’ve only really considered the ‘Assumption of Normality’ – Does our data represent a normal distribution? Is there any ? Is there any ? – We can check (or other graphs) or we can check the – Important to note that assumption checks shouldn’t reflect a major focus of your research – they are just checks and many tests are actually pretty robust! Annotation of a Normal Distribution Descriptive Statistics Measures of Central Tendency – As the term ‘descriptive statistics’ implies, these are used to describe aspects of our observed data (i.e. data collected from our sample) – The measures include: – The : the average of our data (i.e. adding everything together and dividing by the total number of participants) – The : the middle point of our data when each datum is lined up in ascending order (N.B. If there is no apparent midpoint, take the average of the two pieces of data either side) – The : the most common data point – If the mean, median, and mode are equal, your distribution should be normal Measures of Central Tendency – We also get descriptive stats for the distribution – With the mean, we report the – With the median, we report the – We carry out assumption checks to decide which of these we report – Mode is only really used for nominal data – Mean and Median depend on whether our data is skewed Central Tendency and Normality – Part of the Assumption of Normality is assessing whether we have any in our dataset – These are ‘extreme’ data points – what constitutes extreme? – We have to decide whether to remove these or not (why remove someone’s valid response?) – The Mean is affected by outliers, whilst the Median isn’t (so much) – For example, when we talk about average income increasing, that’s because of the rich getting richer (the median paints a more accurate picture) – So, if your data violates the Assumption of Normality, you probably want to report the median (and IQR) NOT the mean (and SD) Inferential Statistics Inferential Statistics – Whilst descriptive stats describe our sample, inferential stats are used to make assumptions (i.e. inferences) about the population – For every analysis, we get a specific statistic (e.g. χ2, r, t) - and we’ll look at three analyses shortly – But, we need to assess whether our obtained results were statistically significant or not (regardless of the analysis) – Something is statistically significant if the p-value is below the In psychology, we use an alpha level of.05 (i.e. 5%) (N.B. different fields use different alpha levels) – We reject the null hypothesis if the p-value is below.05 (or fail to reject if the p-value was greater than the alpha level) – Essentially, we’re saying that there’s less than a 5% chance of getting our observed results (or more extreme results) if there is truly nothing going on. Therefore, there must be something going on – This is as much ‘maths’ as you need to do really – is this number smaller than.05? Inferential – JASP and SPSS calculate these values for us, but we’d have to work these out by hand in ancient times! Statistics – To work out your rough p-value, for example, you’d use a critical values table, using your alpha level and the degrees of freedom – But even with more accurate p-values, we can still make mistakes: – Type I: sometimes referred to as ‘alpha’; rejecting the null hypothesis when we shouldn’t (i.e. thinking there’s something there when there isn’t: a false positive) – Type II: sometimes referred to as ‘beta’; failing to reject the null hypothesis when we should (i.e. not identifying something that is there: a false negative) Inferential Statistics – Our alpha level is actually our accepted rate of making a Type I error – If you increase this (say to.5), there’s a greater chance of incorrectly rejecting the null (Type I) – If you decrease this (say to.001), there’s a greater chance of failing to reject the null (Type II) – To help with this, we want to make sure our study has – Having an adequate sample size is one way to increase this (also things like the type of study, variance in our data) – We aim for.8 (this means there’s an 80% chance of finding a significant result, if there’s one to be found) – However, (e.g. Cohen’s d) are less affected by sample size than p-values – these are essentially a measure of the practical significance – Cohen’s d of 0 means your result is basically random – Cohen’s d of 0.8 (considered large) basically means that our results are correct c.2/3 of the time – Remember, if your p-value is greater than.05 (i.e. non-sig), still report effect sizes (might suggest need for replications) Inferential Statistics: TL;DR – So, we collect data from our sample (drawn from the population) – Work out whether our observed results are due to chance (is our p-value less than the alpha level?) – And use effect sizes to be more assured that our findings are meaningful – The aim is to infer that the sample mean is reflective of the population mean, but different samples will give different scores – We can also use Confidence Intervals to work out a legitimate range of means in which the true population mean is likely to fall – We use 95% in psychology: if we ran our study 100 times, the true population mean would appear 95 times Analysing our Data Testing Frequencies: Chi-Squares – The most basic analysis we did last year (Remember, just because we used this for an observational study, doesn’t mean it’s used exclusively for observational research or vice versa) – Essentially assessing whether our observed count (i.e. our collected data) is different from the expected count (row total*column total/total observations) – Whilst it is a non-parametric tests, there are still assumptions to be met: – Data should be nominal (i.e. categorical) – All observations should be independent (i.e. one value doesn’t affect another value) – Your cells in the frequency table (e.g. tally chart) should be mutually exclusive (i.e. individuals cannot belong to more than one cell) – There needs to be an expected value of at least 5 in 80% of the cells Chi-Square Test of Independence – A two-way test (two categorical variables with multiple levels, e.g. 2x2, 2x3) – Our study could be 2x2 (personality: dark vs. light; dark tourism: visit vs. avoid) – Used to test whether the variables are significantly independent from one another. The focus is on association (difficult to infer causality) – For example, is someone’s personality associated with their travel interests? – H1: People who score higher on the light triad will want to engage in DT more than people who score higher on the dark triad – H0: There will be no association between personality and travel interests – N.B. There are other kinds of Chi-Square tests (e.g. Goodness of Fit) Testing Relationships: Pearson’s – We used Pearson’s Correlation Coefficient for Practical 2 – Spearman’s Rho or Kendall’s Tau if Assumption of NormalityFun is violated Fact: The correlation – Assesses the relationship between two variables (takencoefficient from theis an example same participant) – H1 would be that there would be a of an effect size relationship between your variables – We get a correlation coefficient, which tells us: – The direction of the relationship: either positive (variables move in same direction) or negative (variables move in opposite directions) – The strength of the relationship: correlations can range from -1 to +1, the closer to either of these ends, the stronger the relationship Testing Differences: Student’s t-test – Higher Explanation: used to compare the standardised difference between two groups (e.g. extroverts vs. introverts) or two conditions (e.g. studying in silence vs. with music) – Essential Explanation: is the mean of one level significantly different from the mean of the other level? – There are (used for between-subjects designs; Student’s is the classic, but perhaps best to use Welch’s correction) and (used for within-subjects designs) – Mann-Whitney U Test is a non-parametric independent t-test (for between-subjects) – Wilcoxon Signed-Rank Test is a non-parametric paired t-test (for within-subjects) A quick note about data analysis software Last year, we used JASP in PSY140 However, SPSS has been the focus for many years SPSS is still said to be looked for by employers Your supervisors next year may prefer this too Therefore, we will demonstrate JASP AND SPSS for every analysis – but use which you prefer Activity – Using the guides on Canvas, practice the different analyses in both JASP AND SPSS! – This is the only time you’ll need to use both – For your portfolio, you’ll need to compare the two programmes (this will go on the ‘Data Analysis’ page) – Use the following pointers to guide your reflection: – Was one more intuitive than the other? Why? – Were any differences evident across different analyses? – Has your past experience informed your evaluation? If you were more familiar with SPSS, for example, would your view change? – Are you more likely to use one over the other? If so, which and why? – About a paragraph should suffice Let’s do the Chi Square together! – We want to assess whether there is an association between ice cream preference and level of intelligence. – I’ve sat down on Roker Beach and watched people order ice creams. They only have two choices (banana or strawberry) though! – When they walk away, I judge their levels of intelligence (I don’t know how either; that’s one of the flaws with observation research) – So, we have two variables: – Ice cream preference: banana or strawberry – Intelligence: smart or not so smart – What’s the design of our study? Activity – Using the guides on Canvas, practice the different analyses in both JASP AND SPSS! – This is the only time you’ll need to use both – For your portfolio, you’ll need to compare the two programmes (this will go on the ‘Data Analysis’ page) – Use the following pointers to guide your reflection: – Was one more intuitive than the other? Why? – Were any differences evident across different analyses? – Has your past experience informed your evaluation? If you were more familiar with SPSS, for example, would your view change? – Are you more likely to use one over the other? If so, which and why? – About a paragraph should suffice Making Use of Our Results Applications and Implications – Once we’ve ascertained whether to reject our null hypothesis or not, we need to think about the applications and implications of these findings – This is a big focus of the discussion sections of reports (and potential implications can help to rationalise a study in the intro) – We can see that the light triad is associated more with dark tourism than the dark triad – time to rethink dark tourism? Not as bad as the media makes out? Improving the Quality of Research – Formulate a specific, falsifiable hypothesis We are looking for patterns within data – Select the best design Controlling and analysis for testing this hypothesis for all the noise will help us find – Recruit a good sized sampleone if there’s one to be found – Control for confounding variables as much as possible – If comparing different samples, make sure they’re matched on other characteristics – If Pp are completing multiple tasks, make sure these are ordered logically (e.g. counterbalanced, ramping up) Next Week’s Lab Session – Qualitative Methods Revisited with Linda – The first time point for our in-class assessment