Week 12 S2 2024 BMET1961 Introduction to Biomedical Imaging PDF
Document Details
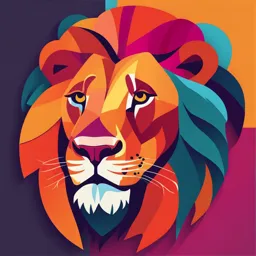
Uploaded by PermissibleMossAgate8517
The University of Sydney
2024
Sandhya Clement
Tags
Summary
This document is an introduction to biomedical imaging. It covers the week 11 lecture and introduces the basics of the topic.
Full Transcript
BMET1961 Introduction to Biomedical Engineering B Week 11: Introduction to Biomedical Imaging Dr Sandhya Clement Lecturer School of Biomedical Engine...
BMET1961 Introduction to Biomedical Engineering B Week 11: Introduction to Biomedical Imaging Dr Sandhya Clement Lecturer School of Biomedical Engineering The University of Sydney I would like to acknowledge the Traditional Owners of Australia and recognise their continuing connection to land, water and culture. I am currently on the land of the Cardigal people of the Eora Nation, and I pay my respects to their Elders, past, present and emerging. I further acknowledge the Traditional Owners of the country on which you are on and pay respects to their Elders, past, present and future. The University of Sydney Who am I ? # Lecturer, School of Biomedical Engineering, The University of Sydney (since 2021) Previously: #Lecturer, Graduate School of Biomedical Engineering –Research, UNSW (2018-2021) #Research Associate-Macquarie University (2017-2018) #Lecturer-Education focused-Department of Electronics and Communication Engineering, College of Engineering, Munnar, India. (2001-2012) Teaching units: Fundamentals of Electrical Engineering, Electronics Circuits, Digital Electronics, Biomedical instrumentation, Digital Signal Sandhya Clement Processing, Biomedical Design and Technology, Biomedical Image Lecturer, BME Analysis, Digital communication, Nanomedicine. Office: J07, 414 Research: Nanodrug formulation for cancer therapy, biomedical image analysis, device development and engineering education research. The University of Sydney This lecture – An overview of biomedical imaging and image analysis – Basics of biomedical imaging – Computerised image analysis – Standard image processing techniques – A sneak peak on the importance of imaging: Application examples – How to proceed further The University of Sydney Page 4 Biomedical imaging Where do we use it? Where does it come from? The University of Sydney Page 5 Motivation: Does biomedical imaging matter? Watson, Crick, and Wilkins Ernst Ruska Electron microscopy Nobel Prize 1962 Ruska, Binnig, Rohrer Nobel Prize 1986 Wilhelm Röntgen, Rosalind Franklin, “Photo 51” “left hand of Albert von Kölliker” X-ray diffraction of DNA Röntgen, Nobel Prize 1901 Computed Tomography Cormack, Hounsfield Nobel Prize 1979 Magnetic Resonance Imaging Lauterbur, Mansfield Nobel Prize 2003 The University of Sydney Page 6 What is biomedical imaging? Localised measurement of biophysical effect within a living system The University of Sydney Page 7 What is biomedical imaging? Localised measurement of biophysical effect within a living system – Localised: positioning (spatial) information is important – Measurement: varying ranges/values of information – Biophysical effect: physical effect of biological phenomenon – Living system: a system that is biological (is or was alive) – Within: can be inside – Implies minimal perturbation to the system The University of Sydney Page 8 Medical imaging – “medical imaging refers to the process involving specialized instrumentation and techniques to create images or relevant information about the internal biological structures and functions of the body” A P Dhawan et al. (2008) – Broadly, medical imaging can be considered a part of radiological science. – The field is multi-disciplinary in nature, requiring expertise from a wide range of disciplines: medicine (radiology), physics, chemistry, physiology, engineering, computer science. The University of Sydney Page 9 Clinical context – imaging departments Leica Biosystems Whole slide Scanner GE BrightSpeed CT Imaging Suite 3D ultrasound via the GE Voluson E8 system: Dr Wolfgang Moroder The University of Sydney Page 10 Scientific contexts – research labs – Studying biology – Testing new Cortical neuron Cell cycle (multi-nucleated) therapies – Examining disease mechanisms – Investigating drug interactions – Discovering functions (b) Treatment progress (nano drug is PLGA-VP-PFOB, and processes RDT is X-ray+nanodrug) Clement et al., Biomedicines, 2021 The University of Sydney Page 11 Modern day context – from the home Nokia 7650 iPhone Braun et al., “Telemedical wound care using a new generation of mobile telephones: a Gunter et al., “Feasibility of an image-based mobile health protocol feasibility study”, Archives of dermatology, 141(2): 254-258, 2005. for postoperative wound monitoring”. Journal of the American College of Surgeons, 226(3): 277-286, 2018. The University of Sydney Page 12 Stryker Mako Surgical Assistant Robot – Patient-specific pre-operative planning from CT data – Intra-operative adjustment of implant placement by surgeon – Robotic arm assists the operative plan https://www.stryker.com/us/en/joint-replacement/systems/mako-robotic-arm-assisted-surgery.html The TheUniversity Universityof of Sydney Sydney Page 13 Page 13 What is an image (as a concept)? – In our context – A representation – In visual form – Of some object or subject more definitions! https://www.merriam-webster.com/dictionary/image The University of Sydney Page 14 Acquisition: a diagrammatic view The 3 S Subject Signal Sensor (visible light) The University of Sydney Page 15 Acquisition: a diagrammatic view The 3 S The 4 S Subject Signal Sensor (visible light) Storage The University of Sydney Page 16 What is an image? – A matrix – Each “cell” of the matrix – Pixel – picture element – Pixel size – size of the area seen in pixel x – Numerical value of cell (x) determines its appearance The TheUniversity Universityof of Sydney Sydney Page 17 Page 17 3D Data: Pixels and voxels The University of Sydney Page 18 Videos as images over time Time The University of Sydney Page 19 Video data: frames … The University of Sydney Page 20 Intensity – The magnitude of the pixel/voxel value Increasing Intensity – Affects its appearance, usually through brightness or colour – High intensity bright – Low intensity dark The University of Sydney Page 21 Intensity – The magnitude of the pixel/voxel value Increasing Intensity – Affects its appearance, usually through brightness or colour – High intensity bright – Low intensity dark The University of Sydney Page 22 Binary Images – Binary pixel values – x can only be 0 (black) or 1 (white) 0 1 1 1 0 1 1 1 0 1 1 1 0 1 1 1 0 1 1 1 0 1 1 1 0 1 1 1 0 1 1 1 0 1 1 1 0 1 1 1 0 1 1 1 0 1 1 1 0 1 1 1 0 1 1 1 The TheUniversity Universityof of Sydney Sydney Page 23 Page 23 Binary Images The TheUniversity Universityof of Sydney Sydney Page 24 Page 24 Gray Scale images – Range of pixel values – From some minimum to some maximum – e.g. 0 (black) to 255 (white) 0 25 50 100 150 200 250 255 The TheUniversity Universityof of Sydney Sydney Page 25 Page 25 What about colour? Use greyscale to represent amount of one particular colour in the image, then composite Red channel Green channel Blue channel The University of Sydney Page 26 Biomedical Images The University of Sydney Page 27 Biomedical imaging CT PET MRI Electro-cardiogram (ECG) 1D 3D 2D 4D X-ray Cardiac MRI OCT Functional MRI US Dynamic PET The TheUniversity Universityof of Sydney Sydney Page Page27 28 Biomedical image modalities – Anatomy/Organ Ultrasound Magnetic X-Ray Resonance Radiography Computed The TheUniversity Universityof of Sydney Sydney Tomography Page Page28 29 Biomedical image modalities – Molecular/Function Positron Emission Functional Magnetic Resonance Single Photon Emission Tomography Computed Tomography The TheUniversity Universityof of Sydney Sydney Page 30 Page 30 Biomedical image modalities – Cellular/Tissue Phase-Contrast Microscopy Fluorescence Microscopy Fluorescence Microscopy Pathology Slide The University of Sydney Page 31 Page 31 Biomedical image modalities – “Regular” Light Photos Dermoscopy The TheUniversity Universityof of Sydney Sydney Page 32 X-rays – Wavelength: ~10pm-10nm (10 000pm) – Ionising radiation – Wavelengths >100 or 200 pm are absorbed completely by the body – Not ideal for medical applications (body absorbs all radiation, nothing to detect) – Can we measure how much radiation is absorbed by each body part? The University of Sydney Page 33 Examples physics behind X-ray imaging High Lower Object Detector X-ray source or Image High Lower Sensor Object Medium High The University of Sydney Page 34 X-rays and human tissues – Tissue density affects how much x-ray radiation is absorbed – Calcium (bone) absorbs quite a lot – Adipose tissue (fat), muscles and other soft tissues absorb less – Water absorbs even less – Air absorbs very little – Attenuation: The ‘stopping power’ of tissue The University of Sydney Page 35 The University of Sydney Page 36 Computerised Image Analysis The University of Sydney Page 37 Why use computers? Some tasks are: – Time-consuming – Repetitive – Tedious The University of Sydney Page 38 Why use computers? Count the number of cells in – a collection of videos – Count cells in first frame – Look for new cells in the – next frame – Repeat 1 and 2 for duration of video AND don’t lose track of old cells – Repeat 1,2, 3 for all videos The University of Sydney Page 39 Why use computers? Computers can: – Follow sets of instructions – Automation of tedious tasks – Process LOTS of data – High throughput analysis – Repeat tasks consistently – Don’t get distracted or bored – Be more objective – May not be as biased or subjective as humans – Scale up – More hardware as needed The University of Sydney Page 40 Computing Hardware – Processors – Rapid calculations – Intel Core i9 capable of trillion floating point operations per second – Sunway TaihuLight supercomputer: over 93,000 trillion operations per second – Distributed and parallel computation – Simultaneous processing across multiple hardware processors or cores – Often dividing one big problem into multiple smaller ones – Graphics Processing Units (GPUs) – Commonly used for video gaming – Design makes them excellent for parallel computation and image processing The University of Sydney Page 41 Computing Hardware – Random Access Memory (RAM) – The amount of data that can be directly accessed for processing – Different from storage: Data that is “saved” and has to be loaded into – memory for processing – Consumer PCs: 8 to 16 GB – Sunway TaihuLight Supercomputer: 1,310,000 GB For comparison One smart phone image: 0.02 to 0.03 GB (depends on camera) One CT image: 0.2 to 0.3 GB The University of Sydney Page 42 Standard Image Processing task The University of Sydney Page 43 Standard image processing tasks – Denoising – Noise is unwanted artifacts – Caused by sensors, environment (lack of light), or acquisition protocol – Change appearance of the image – Distortion – Blurriness – Spots – Disrupts analysis – Is what we are seeing information or just random noise? The University of Sydney Page 44 Standard image processing tasks – Denoising With noise Noise reduced The University of Sydney Page 45 Page 45 Standard image processing tasks – Denoising – Removal of noise Neighbourhood Median – Many different 1 1 0 1 techniques Neighbours: 0 1 1 1 1 1 1 1 1 0 1 1 Neighbours: 0 1 1 1 1 Median: 1 Median: 1 – Often based on 1 1 1 1 statistical analysis – Compare a pixel to other pixels 1 1 1 1 1 1 1 1 – Replace with an 1 1 1 1 adjusted value. The TheUniversity Universityof of Sydney Sydney Page 46 Page 46 Standard image processing tasks – Registration – Multiple images of same subject taken at different times (even a few seconds apart) can be misaligned – People move over short periods (limbs, heart, lungs) – People change over longer periods (gain/lose weight, grow older) – Multiple scans of the same patient from different machines or modalities (e.g., PET-CT): Fusion – Alignment is important for assessment – Location of disease in anatomy? – Tumour smaller/bigger? – Tissue regenerating? The University of Sydney Page 47 PET (acquired at small size) CT (large) The University of Sydney Page 48 Standard image processing tasks – Registration – Transform the images into the same coordinate space – Global methods transform the entire image – Scale, rotate, translate, affine (attempt to preserve straight lines) – Elastic or non-rigid methods transform only part of the image – Distort part of the image to make important landmarks match up E.g., lungs in two images that were moving due to breathing – This distorts parts of the images – Changes some pixel values, leaves others the same The University of Sydney Page 49 Standard image processing tasks – Segmentation – Often only need to consider a specific part of the image – The area with cancer, or the bone with the defect, etc. – Remaining parts of the image unnecessary – Segmentation – Process to identify and isolate the region of interest (ROI) – Delineate the boundary – Separate what is part of the ROI (inside) and what is not (outside) The University of Sydney Page 50 Standard image processing tasks – Segmentation – Group pixels that are similar – Similar pixel value or color – Part of the same object – Along the boundary of an object Liver Tumour – Example: Fill tool in painting programs Region Boundary The University of Sydney Page 51 Standard image processing tasks – Segmentation Select lowest (e.g. necrosis) Select same value, connected (e.g. border) 10 25 29 24 18 20 15 10 25 29 24 18 20 15 Image 17 11 11 11 11 18 28 17 11 11 11 11 18 28 10 17 15 15 25 11 11 11 29 24 11 11 12 25 0 11 18 11 11 11 20 15 18 28 12 19 12 17 15 11 12 25 11 12 19 15 11 12 25 11 12 19 17 11 0 0 11 12 19 15 11 0 12 11 12 17 15 11 0 12 11 12 17 25 11 0 0 11 17 12 29 11 11 11 11 30 20 17 11 0 0 11 12 19 17 11 0 0 11 12 19 25 11 0 0 11 17 12 25 11 0 0 11 17 12 29 11 11 11 11 30 20 29 11 11 11 11 30 20 10 25 29 24 18 20 15 Compare with values > average Expand border to similar values 17 11 11 11 11 18 28 10 25 29 24 18 20 15 10 25 29 24 18 20 15 15 11 12 25 11 12 19 17 11 11 11 11 18 28 17 11 11 11 11 18 28 15 11 0 11 11 12 17 15 11 12 25 11 12 19 15 11 12 25 11 12 19 17 11 0 0 11 12 19 15 11 0 11 11 12 17 15 11 0 12 11 12 17 25 11 0 0 11 17 12 17 11 0 0 11 12 19 17 11 0 0 11 12 19 29 11 11 11 11 30 20 25 11 0 0 11 17 12 25 11 0 0 11 17 12 Pixel Values 29 11 11 11 11 30 20 29 11 11 11 11 30 20 The University of Sydney Page 52 Standard image processing tasks – Segmentation The TheUniversity Universityof of Sydney Sydney Page 53 Page 53 Standard image processing tasks – Visualisation – Images are acquired to be viewed – Clinicians, scientists, and these days even patients – “A picture is worth a thousand words” – Lots of complex biological information – Can overload with redundant or irrelevant information – Visualisation – Process to improve cognition of humans – Creates illustrations, abstractions, or views of information – Summarises, focuses, or draws attention to important aspects – Assists with interpretation and analysis (communication) The University of Sydney Page 54 3D Rendering Slices Volume Data Rendering – This is a 2D projection of 3D volume data. – Remember that the display is screen is still 2D. – The rendering makes it look 3D. The University of Sydney Page 55 Standard image processing tasks – Visualisation PET Tumour Segmentation Registration GPU 3D CT Lung Segmentation Visualisation The University of Sydney Page 56 Standard image processing tasks – Feature Extraction – “A picture is worth a thousand words” – Lots of complex biological information – How do you calculate, quantify, and record important variables? – What is the volume of the tumour? – How long is the defect? – How quickly are the cells dividing? – Feature extraction – Obtaining informative data for use in analysis, machine learning, or to answer specific questions The University of Sydney Page 57 Standard image processing tasks – Feature Extraction Tumour Lung Size/volume Size/volume Roundness/sphericity Shape Position in lung Texture Glucose uptake Distance between lungs The TheUniversity Universityof of Sydney Sydney Page 58 Page 58 Why process and analyse? – Image acquisition is not perfect – Trade-offs – Adjusting contrast resolution may require adjusting radiation – Larger magnetic field strengths may increase spectral resolution but require more energy to power, and greater electromagnetic protection for the hospital – Patients have different cameras at home – Not every facility has the latest and best equipment – Not all image content is relevant to the task The University of Sydney Page 59 Metadata: DICOM, NifTI and TIFF The University of Sydney Page 60 What is metadata? – Data that provides information about other types of data – In the context of biomedical imaging: – What type of image (MR, CT, PET, etc.) is stored in the file? – What scanner was the image acquired with? – What are the dimensions and resolution? – How many grey levels (bit-depth, contrast resolution) used? – What radiotracer dose was administered? – Focus on a few types: DICOM, TIFF, NifTI The University of Sydney Page 61 DICOM – Digital Imaging and Communications in Medicine – Standard for communication and management of medical image data and related information – Used for storage, exchange, transmission, visualisation and results reporting – Defines data dictionary, data structures, file formats, etc. – Ensures image cannot be separated from relevant information by mistake (but can still be deliberately separated) The University of Sydney Page 62 DICOM tags http://dicom.nema.org/medical/dicom/current/output/html/part06.html The University of Sydney Page 63 NifTI – Neuroimaging Informatics Technology Initiative – Open file format used for neuroimaging data – Often magnetic resonance (MR) data – Files usually have a.nii or.nii.gz extension – Sometimes other extensions:.hdr.hdr.gz.img.img.gz – Image data and metadata often included in the same file The University of Sydney Page 64 TIFF (or TIF) Tagged Image File Format Flexible, adaptable file format V6.0 in 1992 around since 1986 Designed to reduce reliance on Has extensible components proprietary formats Can create tags for your own needs Information about image A label/property and its described in ‘tags’ corresponding value The University of Sydney Page 65 What use is metadata? – Obtaining information for processing – Resolution and slice thickness for resampling/resizing – Body weight and dose for 𝑆𝑈𝑉 calculation – Body weight and height and gender for 𝑆𝑈𝑉 – Analysis of processing outcomes – Older scanner may result in different noise characteristics – Different protocol may result in visual differences The University of Sydney Page 66 Biomedical imaging Applications A Sneak Peak The University of Sydney Page 67 Enhancing and processing Fuji Film Digital Radiography PET-CT fusion https://www.fujifilmusa.com/products/medical/digital-x-ray/image- By User:MBq, CC BY-SA 3.0, processing/dynamic-visualization/#images https://commons.wikimedia.org/w/index.php?curid=20682407 The TheUniversity Universityof of Sydney Sydney Page 68 Page 68 Decision making Simulating surgery as part of treatment planning Radiotherapy dose planning Oshiro and Ohkohchi. “Three-dimensional liver surgery simulation: computer-assisted https://medicine.umich.edu/dept/radonc/educ surgical planning with three-dimensional simulation software and three-dimensional ation-training/resources-references/contouring- printing.” Tissue engineering part A, 23(11-12), pp.474-480, 2017. atlas-gi-radiotherapy-planning/imrt- unresectable-pancreatic-cancer-case-2/case-2- supplemental The University of Sydney Page 69 Classification – Catagorising images or regions into different groups Pipeline: – regression, artificial neural 1. Segment images networks, support vector 2. Extract features from regions machines, etc. 3. Use features to classify – Example: type of disease, normal or abnormal structures, malignant or benign The University of Sydney Page 70 Research concept Image Data Prediction Image ML/AI methods that Classification Data analyse image data Delineation … Image Data I design this The University of Sydney Page 71 Covid 19 classification – Differentiate various lung infections based on segmentation-based classification. – Deep learning approach is used for segmentation and classification. Kuzinkovas, D., & Clement, S. (2023). The detection of covid-19 in chest x-rays using ensemble cnn techniques. Information, 14(7), 370. The University of Sydney Page 72 Learning More The University of Sydney Page 73 Units you can do – BMET2925: AI, Data, and Society in Health – Learn about different types of health data – Apply data analytics and AI to health problems – Hands-on projects – BMET5933: Biomedical Image Analysis – Learn about different image analysis techniques – Learn to develop your own image analysis applications – Apply cutting-edge deep learning techniques to image data The University of Sydney Page 74 Tools you can use Python Image Processing Toolbox MeVisLab Fiji OsiriX The University of Sydney Page 75 Build Your Own Machine Learning Model – Data – Kaggle, The Cancer Imaging Archive (TCIA), International Skin Imaging Collaboration (ISIC), … – GPUs – Nvidia Titan V (~$4500), 2080 Ti ($1800), 1080Ti (~$900),.. – Languages – Python, MATLAB, C++ – Programming Library – Caffe, Tensorflow, Torch, Theano, MatConvNet, MXNet, … – Architecture – AlexNet, VGG, GoogleNet, ResNet, U-Net, V-Net, … The University of Sydney Page 76 A way to approach an image analysis problem – Identify the objective – Identify the image property that helps you achieve the goal – Identify what part of the image you need to look at Work backwards – Register and denoise the image data if needed – Segment the part of the image you need – Compute the image features that are most relevant – Analyse the image features to achieve your objective The University of Sydney Page 77 Musculoskeletal analysis – Can combine information from multiple images – CT, x-ray, multiple types of MR – Register all relevant images – Segment each image – Extract features from each image and combine information – Analyse: – Detect bone defect – Characterise muscle inflammation The University of Sydney Page 78 End Please feel free to contact if you want to know more about this topic [email protected] The University of Sydney Page 79