BMEN 509 - Lecture Recaps PDF
Document Details
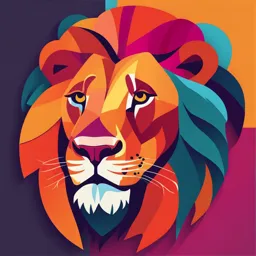
Uploaded by UndauntedLaplace7853
University of Calgary
Tags
Summary
These notes cover basic biomedical imaging concepts, discussing different types of imaging, their qualities, and the history behind them. It includes details on image acquisition and processing, alongside highlighting the importance of image quality factors.
Full Transcript
Jan 15 - Imaging Basics Expanding beyond visible light -โ Tech in biomedical imaging extends beyond the visible spectrum -โ Imaging allows us to observe and analyze internal structures and functions that the human eye cant see Electromagnetic Radiation Spectrum -โ The entire range o...
Jan 15 - Imaging Basics Expanding beyond visible light -โ Tech in biomedical imaging extends beyond the visible spectrum -โ Imaging allows us to observe and analyze internal structures and functions that the human eye cant see Electromagnetic Radiation Spectrum -โ The entire range of electromagnetic waves arranged by their wavelengths or frequencies Non-Ionizing Radiation vs Ionizing Radiation -โ Ionizing: high energy radiation capable of removing electrons from atoms or molecules, creating ions aka ionizing (most biomedical imaging techniques fit in here) -โ Non-ionizing: lower energy radiation that does not have enough energy to ionize atoms or molecules What is biomedical imaging used for? -โ Diagnostic imaging is essential for confirming, correctly assessing and documenting courses of many diseases -โ Assesing responses to treatement -โ Importnat for medical decision making and can reduce unencessary procedure (limit invasivness where possible) History -โ 1895 - Xray - Wilhelm Rontgen -โ 1923 - nuclear medicine -โ 1972 - CT scanner -โ After WW2 - Ulrasound and MRI Medical Image is like a matrix of numbers (pixels) -โ Medical images are often gray-scale (aka each pixel represents a shade of gray ranging from black to white) -โ Black = lowest intensity (no light) -โ White = highest intesity (max light) -โ The value of a pixel is denoted as x[n1,n2] which represents the light intensity or brightness at a specific location -โ The n1 and n2 are the coords of the pixel -โ Each pixel value provides info about the tissue or structure at that specific location in the body -โ E.G : in an x-ray, bone absorbs more radiation so it appears brighter, while soft tissue absorbs less and appears darker Imaging Classification 1.โ Classification by the process/energy used to form an image -โ Visible light: photography, endoscopy, light microscopy, near-infrared light -โ X-ray transmission: projection and computer tomographyof attenuation thru tissues -โ PET/SPECT: gamma-ray emission from radio-tracers within the body -โ Ultrasound: reflection of ultrasonic pressure waves off of tissues -โ MRI: induction of precession of spin systems in a magnetic fieldโฆmeasures teh energy released when nuclei return to original state 2.โ Classification according to physical phenomenon -โ Transmission imaging -โ Emission imaging -โ Reflection imaging -โ Nuclear resonance imaging Classification can be useful for selecting an image modality based on the medical question at hand Image Quality -โ Factors that make for a good image: 1.โ No acquisition issues 2.โ Good resolution, sharp image 3.โ No artifacts (e.g. no ring on finger when taking x-ray) 4.โ Good signal, low noise 5.โ Good contrast -โ Consider limitations of each system (aka choose the right system for the job) -โ CNR (contrast to noise ratio): measures ability to distinguish between two diff tissues or structures in an image based on diff in their signal intensities (contrast) -โ Low CNR = low contrast = bad image -โ SNR (signal to noise ratio): strength of signal relative to background noise (overall clarity of image) -โ low SNR = bad image -โ ROC (receiver operating characteristics): tool used to evaluate the diagnostic performance of a medical imaging system -โ Aka: can the system distinguish between true pos and true neg -โ TPR (true positive rate) also called sensitivity -โ FPR (false positive rate) -โ Graphs an ROC curve (TPR on x-axis and FPR on y-axis) -โ The Closer the ROC curve is to the top left corner the better the systems performance Jan 17 DATA ACQUISITION Analog detector -โ DEF: device used in imaging systems to detect and measure physical signals like light, sound, radiation and convert them into a continous electrical signal (analog signal) -โ Collects an analog signal and a analog signal processor converts it into readable output BUT signal is still in analog form โฆ can later involve additional processing to convert into digital form later (like an ADC) Digital detector: -โ DEF: device used to detect physical signals like X-rays, light, or sound and convert them directly into digital signalsโdiscrete numerical values that can be easily processed, stored, and analyzed by computers. -โ NOTE: modern imaging systems increasingly favor digital detectors due to their high precision, ease of integration, and ability to produce real-time images. Quantization Error โ When does it become a factor? -โ Whenever an analog signal is converted to a digital signal How does it work? -โ A continuous signal is sampled at discrete intervals determined by the system bit depth -โ High bit depth reduces quantization error by increasing number of discrete intensity levels -โ The continuous signal may not match a discrete level perfectly, leading to a small approximation error (called quantization error) โ โ โ More on bit depth -โ e.g. 8-bit depth means the image can have 256 possible values.. The signal intensity will be approximated to one of these 256 levels BUT if a system has a 16-bit depthโฆthe image can now have 65 536 possible values and the signal can be assigned to a more accurate discrete level (better representation of the actual signal) when being converted from analog to digital - leading to a smaller approximation and smaller quantization error Memorize -โ More bits = more discrete levels = less quantization error = better image Why does it matter in biomed imaging? -โ A big quantization error reduces the accuracy of the digital representation of the signal which = lower image quality + can amplify noise in the image -โ A small quantization error ensures better contrast = the image is better seen It's more than just bit-size that can affect limit image qualityโฆ Time Limitations on Signal Acquisition 1.โ Resolution vs time -โ High res images require more time to be captured (system needs to record more data points) 2.โ Patient movement and image quality -โ The more time signal acquisition takes, the greater the likelihood that the patient might move -โ Patient moevemnt can lead to motion artifacts ( blurriness or distortions) Key takeaway is that time is an important factor in the imaging process, and the system needs to balance acquisition speed and image quality based on the specific clinical application -โ Emergency situations: x-ray and CT scan is often used due to shorter acquisition time but image quality might not be as good -โ MRI takes a long time to acquire and can be a limitation in emergency situations or for patients who cant stay still well (kids) Nyquist Theorem DEF: to accurately sample a signalโฆ the signal must be sampled at least twice as fast as its bandwidth to avoid losing info when converting a continuous signal to a discrete one In other words: the sampling rate needs to be at least twice the highest frequency present in the signal Why? Ensures the capture of the entirety of the signal, preserving its integrity Aliasing: using a lower than required sampling frequency (at a lower rate than Nyquist rate) What does this result in? The high-frequency details of the signal that cant be captured during sampling are misinterpreted or folded back into lower frequencies leading to distortions, artifacts or missing info in the digital signal (or image) How does this show up in medical imaging? In medical images, if sampling rate is too low, fine structures or small variations may be missed or incorrectly represented + artifacts or unwanted patterns may appear SPATIAL RESOLUTION DEF: ability of an imaging system to resolve small independent objects in close proximity to one another (ability to tell small things apart) Dependent onโฆ the acquisition system being used and itsโฆ 1.โ Detector type 2.โ Sampling rate 3.โ Bit-depth etc. Imaging system with high spatial resolution = can detect smaller details or higher spatial frequencies Spatial Frequency: the rate at which structures or details in image appear/chnage in an area a)โ Has lowest spatial frequency c) has highest spatial frequency Cone test: used to evaluate the ability to distinguish between two objects or structures that are close together (i.e., the smallest spatial detail the eye can resolve). (this is a test performed on the human eyeโฆ for evaluating spatial resolution of technical imaging techniques we use other methodsโฆ.) Evaluation of Spatial Resolution in Imaging Tehcnologies 1.โ Line spread function (LSF) - Blurriness What is this? -โ describes how an imaging system responds to a line source of radiation (like a thin beam or line of light) -โ When you capture an image of a sharp line, the system will spread out the line into a broader, blurred region due to the limitations in resolutionโฆ The LSF quantifies how much the sharp line has spread out in the image. -โ Good spatial resolution = small and more concentrated spread โ Math -โ LSF is usually represented by Gaussian function (bc the line blur tends to be symmetric and its spreading pattern resembles a gaussian curve) -โ ฯ: Standard deviation, which controls the width of the Gaussian curve. -โ Smaller ฯ = narrower gaussian function = sharper resolution (in perfect world ฯ = 0) -โ Small FWHM = high res (cuz narrower LSF) 2.โ Point Spread function (PSF) - 3D blurriness -โ Ideally, a point source should appear as a tiny dot in the image. However, due to imperfections in the system (like blurring), the point will spread out into a regionโฆThe PSF measures the amount of spreading or blur around that point. -โ Good spatial resolution = small PSF -โ Resolution may vary depending on the plane -โ Why? Bc imaging systems often have diff performance characteristics along different spatial planes -โ NOTE: The image we actually see is not a perfect replica of the objects geometry but rather a blurred version. Aka it will be a convolution (combination) of the actual geometry of the object and the PSD to produce a third function = the image. 3.โ Modulation Transfer Function (MTF) - response in frequency -โ Mathematical representation of how well the system can capture the contrast of different spatial frequencies (finer or coarser details) in the image. -โ In easier termsโฆ quantifies how much detail at different scales (from very fine to very coarse) the system can accurately reproduce -โ MTF = 1 means system can perfectly repreoduce the contrast for a particular frequency (ideal) -โ AN IDEAL MTF RESPONDS EQUALLY TO ALL FREQUENCIES ( = 1 for every spatial frequency) -โ This means the imaging system perfectly reproduces all details.. 1.โ From Low- frequency (large, broad structures) 2.โ To High-frequency (fine, small details) โ โ โ โ Without loss in contrast or sharpness NOISE Def: any signal that is recorded but is unwanted info Types of noise: electronic, quantum, environmental, etc. Noise models -โ Used to mathematically represent how noise behaves + impacts the image -โ E.g.โs : Poisson noise, Guassian/Normal noise, etc. SNR (signal to noise ratio) Def: : strength of signal relative to background noise (overall clarity of image) -โ low SNR = bad image -โ a higher ฯ = bad image CNR (contrast to noise ratio) Def: measures ability to distinguish between two diff tissues or structures in an image based on diff in their signal intensities (contrast) -โ Low CNR = low contrast = bad image Jan 20 - Introduction ROC CURVE ROC (Receiver Operating Characteristic) curve What is it? -โ A graphical representation is used to evaluate the performance of a diagnostic test, imaging system, or classifier -โ Illustrates the trade-off between sensitivity (true pos rate) and specificity (false pos rate) Sensitivity (True Positive Rate) -โ The proportion of actual positives correctly identified by the test (aka: Was IDENTIFIED AS POSITIVE and IS ACTUALLY POSITIVE) Specificity (True Negative Rate) -โ The proportion of actual negatives correctly identified by the test (aka: Was IDENTIFIED AS NEGATIVE and IS ACTUALLY NEGATIVE) Accuracy -โ How well a test correctly identifies BOTH true positives (TP) and true negatives (TN) (aka the proportion of correct diagnoses) -โ Limitation: accuracy can be misleading if the dataset is imbalance (one class bigger than another) Confusion Matrix โ Provides the raw numbers (TP, FP, TN, FN) which can then be used to derive metrics used to evaluate the models performance โ Metrics Include: Jan 22 - Image Rendering 1st things 1stโฆ what is image rendering.. Image rendering: creating a visual representation of medical data which varies depending on the imaging modality (type of imaging used) โฆ so each modality has unique advantages and limitations Common approaches in image rendering to make medical images more informative and visually meaningfulโฆ 1.โ Gray scale representation -โ What it is: The most basic and widely used representation in medical imaging. Each pixel is assigned an intensity value that corresponds to the measured signal -โ Why it's used: Highlights differences in tissue density or composition.Simplicity allows for a clear visualization of structures. 2.โ Superimposing Information -โ What it is: Overlaying additional data onto the image to provide more context or functional information. -โ Why it's used: Combines multiple imaging modalities for better diagnosis. Adds functional or diagnostic data on top of anatomical images. 3.โ Enhancement of surface info -โ What it is: Techniques like surface rendering emphasize the external contours or surfaces of structures, often in 3D. -โ Why it's used: Provides a clearer view of anatomical boundaries or abnormalities. Useful for surgical planning or studying structural relationships Projection and Tomography (methods for acquiring and reconstructing the image data โฆ which is then used for rendering aka visually representing the data) Projection -โ Def: image formed by the passage on a single direction of energy -โ AKA: gives single 2D image of a 3D structure -โ When to use: when a quick overview is needed w out requiring 3D info - quick/borad diagnostic (faster, less expensive, lower radiation exposure) (ex: bone fractures) Tomography -โ Def: imaging by slicing or sectioning through an object to create a series of cross-sectional images (or slices) that can be reconstructed into a 3D representation. -โ AKA: gives 3D image of structure by using multiple 2D slices -โ When to use: when you need detailed 3D info (slower, more expensive, higher radiation exposure) (ex: brain imaging, cancer staging) Orientation + Coordinates Why? Define spatial position of structures in body + ensure consistent data interpretation. Coordinates and anatomical planes are important in medical imaging because they help describe where things are in the body and ensure images are consistent, accurate, and easy to interpret. 1.โ Anatomical Planes -โ Axial (Transverse) -โ Coronal (Frontal) -โ Sagittal 2.โ Coordinate systems: โ Patient Centric Coordinates (defined relative to patient) -โ X-axis: left to right -โ Y-axis: Anterior (front) to posterior (back) -โ Z-axis: Inferior (bottom) to superior (top) These work together to act like a map for the body, making sure everything is correctly oriented and positioned in the images. IMAGE CHARACTERISTICS Histogram What is it? Graphical representation of the pixel intensity level distribution in an image (every point in space in the image has a numerical value) -โ Pixel intensity represents the grayscale level: -โ Low intensity: Dark areas (e.g., air, soft tissue). -โ High intensity: Bright areas (e.g., bones, metal). -โ Peaks in certain intensity ranges may indicate the presence of specific tissues or abnormalities. (like in the brain pic on slide 45 - white spot has a peak) Fourier Transform What is it? A math tool that breaks down an image into its frequency components Why do we use it? -โ The imaging techniques give us an image in the spatial domain - which is the actual visual representation of data โthe image.โ -โ The fourier transform can then be used to convert the spatial domain into frequency domain -โ The frequency domain - is a representation of the images frequency components -โ The frequency domain can then be used for advanced image processing likeโฆ 1.โ Filtering noise -โ Remove noise like high-frequency noise -โ Emphasize edges or contrast (by focusing on high freq components) -โ Smooth images (by reducing high frequencies). 2.โ Reconstructing images -โ The raw data is collected in the frequency domain in modalities like CT or MRI. Transforming it back to the spatial domain gives us the visible image. 3.โ Analyzing patterns -โ Detect repetitive patterns or periodic features in an image, like textures or structures. โฆ NOTE: we can also use the inverse fourier transform to get spatial domain if the imaging modality collects data in the frequency domain (like an MRI - the raw data is collected in the frequency domain and then is converted to spatial domain using inverse fourier transform) How we can use this to actually manipulate imagesโฆ 1.โ Fourier Transform: Convert the spatial domain image into the frequency domain. 2.โ Manipulation: Apply filters or adjustments in the frequency domain to isolate or enhance desired components. 3.โ Inverse Fourier Transform: Convert the frequency-domain image back into the spatial domain for visualization. Remember!!! 1.โ Fourier Transform: spatial domain to frequency domain 2.โ Inverse Fourier Transform: frequency domain to spatial domain In Biomedical Imaging FFT is more often used (Fast Fourier Transfrom) โฆ why? -โ Faster algorithm -โ Biomedical data deals with discrete data and the FFT is designed specifically to compute the Discrete Fourier Transform efficiently. -โ Practicality: In biomedical imaging, you need to convert data between the spatial domain (images) and the frequency domain (k-space). FFT allows for quick and accurate both forward and inverse Fourier transforms, enabling fast image reconstruction and analysis. (Same exact concept as FT just faster and deals w/ discrete data - preferred for biomed img) Remember these basic Fourier Transforms: Jan 27 - DICOM and Histogram Manipulation DICOM (Digital Imaging and Communications in Medicine) -โ standard for storing, transmitting, and sharing medical images and related data -โ Enables medical info associated to the images to be in a single file and independent of proprietary formatting (aka it does not rely on a specific, closed, or proprietary format that is controlled or owned by a particular company or organization. Instead, it uses open, standardized, or universally accepted formats that can be easily accessed, read, or used by a variety of different systems or software from different vendors.) -โ Structure of a DICOM -โ Organized into image data (pixels) and associated metadata (like patient info, modality, etc) into datasets -โ Each data set is constructed from 3 components 1.โ Tag: An identifier or label used to define a particular piece of data within the set (e.g., patient name, date of the examination). -โ made up of an ordered pair of unsigned 16-bit integers. This means the tag is represented by two 16-bit numbers that are assigned to specific pieces of information in the dataset. -โ For example, one pair of numbers might represent the "patient's name" tag, and another pair might represent the "date of the examination" tag. 2.โ Value Length: Specifies the length (size) of the data associated with the tag. 3.โ Value: The actual data (e.g., the name of the patient or the date of the examination). Image Processing Popular image processing techniques 1.โ Enhancement: histogram manipulation, gray level manipulation, sharpening, smoothing 2.โ Features: edge detection, segmentation 3.โ Restoration: compression, noise removal, blur removal Histogram manipulation 1.โ Histogram Equalization -โ Contrast enhancement -โ Increasing the diff between light and dark regions in the image -โ How it's done? -โ expanding the pixel intensity range to use the full available range (ex: 0 to 255 in an 8-bit image) -โ This makes it so we can maximize the amounts of shades of grey availableโฆ if theres for example an image with lots of pixel values clustered around the same intensity range, stretching the histogram can redistribute these values across the full spectrum thus enhancing the contrast 2.โ Brightening the Image -โ Brightening an image that is too dark or has low visibility, helps to highlight structures that are hard to see -โ How it's done? -โ If you add a constant value to all pixel intensities, the entire histogram shifts to the right -โ As a result, dark pixels become lighter and the bright pixels are pushed toward the max intensity value 3.โ Histogram Compression -โ a form of contrast reduction -โ How it's done? -โ reducing the dynamic range of pixel intensities in an image, effectively "compressing" the histogram to use a smaller range of values -โ Narrower range of colour is now available -โ Usually, we dont want contrast reduction but sometimes this can be used in dynamic range management for exampleโฆ -โ If an image has a very wide dynamic range, meaning it contains both extremely dark and very bright areas, histogram compression can help to adjust the image so that it fits into a viewable range. This is useful when the image is too extreme for the display device or analysis tool to render effectively. -โ Compression reduces the difference between the lightest and darkest areas, making the entire image more balanced and easier to view, especially on devices that can only display a limited range of brightness. NOTE - Histograms can be adjusted in two different approaches 1.โ Global modifications (affect the whole image) -โ Adjusts the entire imageโs histogram uniformly -โ These mods treat the image as a whole and dont account for local variations or specific areas within the image -โ useful when you want to improve overall contrast or enhance features that are consistent across the image, like in cases where an image has a poor overall dynamic range -โ ex) histogram equalization 2.โ Local Modifications (Based on Neighbours) -โ Allows for more specific manipulation -โ making changes that are specific to small regions of the image -โ These modifications allow for more targeted enhancement or correction based on local intensity distributions, without affecting the entire image in the same way -โ Ex: smoothing, median filtering (noise reduction) Jan 29 - Filtering Filters -โ What is it? -โ modifies an image by changing/altering the contributions of pixels -โ Domains - filtering can be done in either 1.โ Spatial/Image Domain: directly manipulating pixel values 2.โ Frequency Domain: applying transformations to modify frequency components -โ Types 1.โ Low pass filters: -โ Frequency domain: lets low frequencies thru and gets rid of high frequencies -โ Spatial/Image domain: averages local pixel values - effectively reducing rapid intensity changes/variations (associated with high frequencies) therefore making the image smoother -โ Note: it doesn't actually increase the low frequencies but allows them to remain more visible by reducing the presence of high frequency noise and sharp transitions -โ It smooths images but blurs details 2.โ High pass filters: -โ Frequency domain: Let high frequencies thru and gets rid of low frequencies -โ Spatial/Image domain: looks for those rapid intensity changes between pixels (areas with sharp transitions) - the HPF highlights these sharp changes by making the differences more pronounced -โ Enhances edges but increases noise How LPF and HPF work in each domain and why to use them 1.โ Spatial/Image Domain Filtering โ Involves direct manipulation of pixel values (averaging pixel values using a convolution kernel/convolution mask) โ In LPFsโฆ โโ Taking an average of neighbouring pixel values to smooth the image โ In HPFs โโ Enhancing edges by emphasizing pixel intensity differences โ But how does this work? โโ By means of convolutionโฆ the filter (kernel) is moved across the image, and a weighted sum of neighbouring pixels replaces each pixel โ When to use this domain? โโ Works better for smaller filters โโ Best for localized changes โ Why? Convolution applies filters directly to pixels, making it useful for enhancing or modifying specific areas. 2.โ Frequency Domain Filtering โ Involves transforming the image into frequency space using Fourier Transform to then modify the frequencies โ In LPFsโฆ โโ We suppress high-frequency components by reducing amplitudes in the high frequency regions โ In HPFsโฆ โ We suppress low-frequency components by reducing amplitudes in the low-frequency region. โ But how does this work? โโ The Fourier Transform (FFT) transforms the image into its frequency representation. โโ A filter is applied to modify the frequency components. โโ The image is converted back using the Inverse Fourier Transform (IFFT). โ When to use this domain? โโ More efficient for larger filter sizes โ Best for global changes โโ Why? Fourier Transform breaks the image into frequency components, allowing you to modify the entire image more effectively. Okayโฆbut filters are more advanced than just letting some high frequencies (or low frequencies) through or notโฆ -โ Real-world signals and systems are more complex than that and require more sophisticated filtering techniques -โ From the basics of LPFs and HPFsโฆA classic low-pass filter (LPF) or high-pass filter (HPF) is very simple: you choose a cutoff frequency, and any signal below (for LPF) or above (for HPF) that frequency is allowed through. The filter either passes or blocks frequencies based on a sharp cutoff. โฆHowever, while this is simple and works in some cases, it has some downsides: -โ Abrupt cutoff: The transition from "allowed" to "blocked" frequencies is usually very sharp, which can cause distortion or ringing effects in the signal. For example, when you cut off a frequency, the signal can get "messy" near the cutoff point, which is often undesirable. -โ No smoothness: The classic filter doesn't smooth out transitions well, which can be an issue if you need a more natural or gradual change. โ โฆ So, we needed to make filters that could: 1.โ Provide smoother transitions (gradual roll-off) rather than sharp cutoffs. 2.โ Offer no distortion in the passband (the frequencies you want to keep). 3.โ Work in a practical way, without introducing artifacts like "ringing" or "ghosting." The following is how adjustments in size and filter domains can address these issues.. Size and Domain Considerations for LPFs (all in frequency domain) 1.โ Size - how much are we cutting out or letting thru (reffering to frequencies) -โ In the frequency domain, the size of the filter is referring to the cutoff frequency -โ If a smaller LPF is used, then lots of frequencies are getting removed and the image is likely blurrier -โ If a bigger LPF is used (letting more frequencies thru) then the image will be clearer 2.โ Domain - affects how we remove the unwanted frequencies (all referring to frequency domain) a.โ Circular LPF -โ Smooth frequency response with symmetry in all directions; periodic effects in the time domain. -โ AKA: Smooth, but can create repeating patterns. b.โ Rectangular LPF -โ Sharp cutoff with abrupt transitions; time-domain "boxcar" filtering, but with spectral leakage. -โ AKA: Sharp cutoff but causes distortion. c.โ Sinc (moving average) LPF -โ Perfect theoretical filter with an ideal cutoff; "ringing" artifacts due to truncation in time-domain. -โ AKA: Perfect but leads to ringing. d.โ Butterworth LPF -โ Smooth frequency response with no ripples in the passband; gradual and practical transition in both domains. -โ AKA: Smooth, gentle cutoff with no ripples. Examples of different types of smoothing and edge-enhancing masks (aka filters) - all used in spatial/image domain โ Smoothing -โ Neighbourhood average -โ This is the simplest type of smoothing. A pixel is replaced by the average value of the pixels in its neighborhood. It smooths out the image by reducing sudden changes. -โ Weighted average -โ This is similar to the neighbourhood average, but each pixel in the neighborhood is given a weight. So, some pixels have more influence on the result than others, which helps to preserve certain details while smoothing. -โ Gaussian mask -โ A more advanced form of weighted average, this uses a Gaussian distribution (bell curve) to assign weights. The pixels closer to the center of the neighborhood get higher weights, and the pixels further away get lower weights. It produces a smoother blur and is very commonly used in image processing. -โ Median -โ The median filter replaces each pixel with the median value of the pixels in its neighborhood (not the average). Itโs great for removing salt-and-pepper noise because it avoids the blurring effect of averaging. โ Edge enhancement -โ Prewitt -โ A simple edge-detection filter that uses gradient operators to calculate the change in intensity in both the horizontal and vertical directions. It's good for detecting edges but not as sensitive as some other methods. -โ Sobel -โ Similar to Prewitt, but with a slightly different kernel (mask). The Sobel filter gives more weight to the center pixel in its kernel, making it more sensitive and better at detecting edges compared to Prewitt. It's widely used for edge detection in image processing. -โ Laplacian -โ A second-order derivative filter that highlights regions of rapid intensity change (edges) but works differently from gradient-based methods like Prewitt and Sobel. It is used to detect areas of rapid change, but can be more sensitive to noise. Feb 3 - Segmentation and Registration Segmentation -โ Def: dividing images into meaningful parts -โ Examples of segmentation methods 1.โ Gray-levl techniques: uses intensity differences to separate regions 2.โ Edge detection: finds object boundaries by detecting sharp changes in intensity 3.โ Libraries of images: uses a reference dataset to classify pixels -โ The whole technique is based on finding masks that select different parts of the image Registration -โ Def: aligns images from different modalities or time points -โ Advantages: -โ can make for more complete medical images with more data -โ Can use it for tracking changes in stuff over time (like tumours)...u can put an image of the tumour now vs 3 months ago to see if its changed -โ Disadvantages/problems: -โ Images aren't always in exact same orientation -โ Adjustments must be made but then distortion can be an issue -โ Basic steps in registration: 1.โ Obtain image datasets 2.โ Transform the images (move, rotate, deform) 3.โ Compare the transformed image to the reference and adjust until they match 4.โ Optimize alignment to minimize differences 5.โ Render both images (overlap them with transparency for example) -โ Types of transformations used in registration: 1.โ Rigid displacement: moves the whole image without distortion T(x)=I(x)+D (shifts image by vector D) 2.โ Rotation: rotates image T(x)=RI(x)+D (where R is a rotation matrix) 3.โ Parametric transformation: more complex transformation (scaling and shear) 4.โ Non-parametric transformation: more complex transformations (splines, warping) -โ Ways to compare reference image to transformed imageโฆ 1.โ Distance between fixed landmarks (e.g. key anatomical points) 2.โ Intensity differences (measuring pixel mismatch) 3.โ Distance to curved points (aligning curved features) 4.โ Correlation between images (measuring similarity)