Hypothesis Testing: Null vs Alternative Hypothesis PDF
Document Details
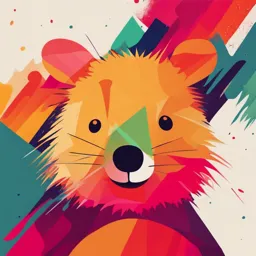
Uploaded by IrresistibleHurdyGurdy9110
Gordon College
Dante P. Sardina
Tags
Summary
This document provides an overview of hypothesis testing, a critical decision-making process for evaluating population claims. It covers the null hypothesis, alternative hypothesis, significance levels, and p-values. The document also discusses Type I and Type II errors, and includes information on parametric and non-parametric tests.
Full Transcript
Republic of the Philippines City of Olongapo GORDON COLLEGE Olongapo City Sports Complex, Donor St., East Tapinac, Olongapo City HYPOTHESIS TESTING According to Bluman (2009), hypothesis testing constitutes a critical decision-making process for evalu...
Republic of the Philippines City of Olongapo GORDON COLLEGE Olongapo City Sports Complex, Donor St., East Tapinac, Olongapo City HYPOTHESIS TESTING According to Bluman (2009), hypothesis testing constitutes a critical decision-making process for evaluating population claims. In this procedure, the researcher is required to define the population of interest, articulate the specific hypotheses to be assessed, establish the significance level, select a representative sample from the population, gather the relevant data, execute the necessary calculations for the statistical test, and ultimately derive a conclusion. Furthermore, hypothesis testing is an essential component of statistical inference, primarily utilized to ascertain the validity of a hypothesis based on sample data. The process commences with the formulation of two competing hypotheses: the null hypothesis (H₀), which asserts the absence of an effect or relationship between variables, and the alternative hypothesis (H₁), which posits the existence of such an effect or relationship. The primary objective of hypothesis testing is to evaluate the evidence presented by the data about the null hypothesis, culminating in a determination of whether to reject or fail to reject H₀, based on a predetermined significance level, commonly denoted as alpha (α) (Turner et al., 2020; Curran‐Everett, 2009; Newman, 2008). NULL HYPOTHESIS VS. ALTERNATIVE HYPOTHESIS In statistical hypothesis testing, the null hypothesis (H 0) and the alternative hypothesis (H1) serve as foundational concepts that guide the analysis of data. The null hypothesis typically posits that there is no effect or no difference between groups, while the alternative hypothesis suggests that there is an effect or a difference. This dichotomy is crucial for determining the validity of claims made based on empirical data. Null Hypothesis The null hypothesis is often viewed as a statement of no change or no effect, which is easier to test due to its specific nature. For instance, in clinical trials, the null hypothesis might state that a new treatment does not differ from a standard treatment, while the alternative hypothesis would assert that the new treatment is either better or worse than the standard treatment (Ratain & Karrison, 2007). This framework allows researchers to focus on the null hypothesis, using statistical tests to determine whether to reject it based on the evidence provided by the data (Huang et al., 2014). The rejection of the null hypothesis typically occurs when the p-value falls below a predetermined significance level, such as 0.05, indicating that the observed data is unlikely under the assumption that the null hypothesis is true (Graaf & Sack, 2018). Alternative Hypothesis Alternative hypothesis is crucial for understanding the implications of rejecting the null hypothesis. It represents the hypothesis that researchers aim to support through their analysis. The alternative hypothesis can be one-sided or two-sided, depending on whether the research question is directional (e.g., treatment A is better than treatment B) or non-directional (e.g., treatment A is different from treatment B) (Brereton, 2020). The formulation of the alternative hypothesis is essential, as it shapes the interpretation of the results. For example, if a study finds significant evidence against the null hypothesis, Dante P. Sardina Republic of the Philippines City of Olongapo GORDON COLLEGE Olongapo City Sports Complex, Donor St., East Tapinac, Olongapo City it can lead to the acceptance of the alternative hypothesis, thereby suggesting a meaningful effect or difference (Ratain & Karrison, 2007; Huang et al., 2014). Depending on the nature of the research question, these hypotheses can be tested using either one-tailed or two-tailed tests. 1. Two-tailed test hypotheses In a two-tailed test, the alternative hypothesis is non-directional, indicating that the parameter of interest can be either greater than or less than a certain value. For example, consider the following hypotheses regarding the mean of a population: Null Hypothesis (H0): μ = μ0 (the population mean is equal to a specified value) Alternative Hypothesis (H1): μ ≠ μ0 (the population mean is not equal to the specified value) This type of testing is commonly used in various fields, including psychology and medicine, where researchers are interested in detecting any significant difference from a known value, regardless of the direction of the difference (Montalbán et al., 2016; Turner et al., 2020). The two-tailed test is often preferred when there is no prior expectation about the direction of the effect, as it provides a more conservative approach to hypothesis testing (Prescott, 2019; Curran‐Everett, 2009). 2. One-tailed test hypotheses In contrast, a one-tailed test is used when the alternative hypothesis is directional, suggesting that the parameter of interest is either greater than or less than a certain value. For instance, the hypotheses might be formulated as follows: Null Hypothesis (H0): μ ≤ μ0 (the population mean is less than or equal to a specified value) Alternative Hypothesis (H1): μ > μ0 (the population mean is greater than the specified value) Left-tailed test ▪ Ho : parameter = specific value ▪ H1 : parameter < specific value Right-tailed test ▪ Ho : parameter = specific value ▪ Ha : parameter > specific value This testing approach is often applied in scenarios where a specific direction of effect is anticipated based on prior research or theoretical considerations (Ruxton & Neuhäuser, 2010; Lombardi & Hurlbert, 2009). However, the use of one-tailed tests has been a subject of debate, as it can lead to misinterpretation of results if the direction of the effect is not correctly predicted (Hurlbert & Lombardi, 2012; Serlin, 2000). Critics argue that one-tailed tests may inflate the Type I error rate if used inappropriately, emphasizing the need for careful justification of their use (Ruxton & Neuhäuser, 2010; Lombardi & Hurlbert, 2009). Note. Although the definitions of null and alternative hypotheses given here use the word parameter, these definitions can be extended to include other terms such as distributions and randomness. Dante P. Sardina Republic of the Philippines City of Olongapo GORDON COLLEGE Olongapo City Sports Complex, Donor St., East Tapinac, Olongapo City Relationship Between Signs in Hypothesis and the Tails of the Test Test Type Two-tailed test Right-tailed test Left-tailed test Signs in the Ho : µ = k Ho : µ = k Ho : µ = k Ho Signs in the H1 : µ ≠ k H1 : µ > k H1 : µ < k H1 Rejection In both tails In the right tail In the left tail region Clarifications: ▪ Two-tailed test: The null hypothesis (H₀) assumes the population mean (µ) equals some value (k). The alternative hypothesis (H₁) tests for any significant difference (i.e., µ ≠ k), and the rejection region is in both tails of the distribution (for values significantly higher or lower than the hypothesized mean). ▪ Right-tailed test: The null hypothesis (H₀) assumes µ = k, and the alternative hypothesis (H₁) suggests that µ is greater than the hypothesized value (µ > k). The rejection region is in the right tail (values significantly greater than the hypothesized mean). ▪ Left-tailed test: Similar to the right-tailed test, but the alternative hypothesis (H₁) suggests that µ is less than the hypothesized value (µ < k). The rejection region is in the left tail (values significantly smaller than the hypothesized mean). Common Phrases in Hypothesis Testing Common Phrases in Common Phrases in Symbol Symbol Hypothesis Testing Hypothesis Testing Is equal to Is not equal to = Is the same as ≠ Is not the same Is exactly the same as Is different from Is increased Is decreased Is greater than Is less than Is higher than Is lower than Is at least Is at most ≥ Is not less than ≤ Is not more than Is greater than or equal to Is less than or equal to Dante P. Sardina Republic of the Philippines City of Olongapo GORDON COLLEGE Olongapo City Sports Complex, Donor St., East Tapinac, Olongapo City Clarifications: ▪ =: Used in the null hypothesis (H₀) to indicate that the population parameter (e.g., mean, proportion) is exactly equal to a hypothesized value. ▪ ≠: Used in the alternative hypothesis (H₁) for two-tailed tests to indicate that the population parameter is not equal to the hypothesized value. ▪ >: Used in right-tailed tests for the alternative hypothesis (H₁) to indicate that the population parameter is greater than the hypothesized value. ▪ α: If the p-value is greater than the significance level, you fail to reject the null hypothesis. This means the observed data could likely have occurred by chance, and there is no significant effect. The P-value method for testing hypotheses differs from the traditional method (Critical Value Method using z-score). Dante P. Sardina Republic of the Philippines City of Olongapo GORDON COLLEGE Olongapo City Sports Complex, Donor St., East Tapinac, Olongapo City UNDERSTANDING THE CRITICAL-VALUE METHOD The Critical-Value Method is one of the most common approaches for hypothesis testing. It involves comparing the test statistic (calculated from sample data) to a critical value that represents the threshold for rejection, based on the significance level (α) and the type of test you are conducting (e.g., one-tailed or two-tailed). The observed value of the statistic (sample observation) is compared to critical values (population observation). These critical values are expressed as standard z values. For instance, if we use a level of significance of 0.05, the size of the rejection region is 0.05. ONE-TAIL Illustration Fig. 1 Finding the critical values for α (alpha) = 0.05 (right-tailed test) 0.95 Rejection region α = 0.05 0.45 Z C.V. Accepted region Fig. 2 Finding the critical values for α (alpha) = 0.05 (left-tailed test) 0.95 Rejection region α = 0.05 0.45 C.V. Z Accepted region Dante P. Sardina Republic of the Philippines City of Olongapo GORDON COLLEGE Olongapo City Sports Complex, Donor St., East Tapinac, Olongapo City If the test is two-tailed; the rejection region is divided into two equal parts (i.e. we divided 0.05 into two equal parts of 0.025 each). A rejection region of 0.025 in each tail of the normal distribution results in the cumulative area of 0.025 below the critical value in the left tail and a cumulative area of 0.025 above the upper critical value of the right tail. TWO-TAILED Illustration Fig. 3 Testing the Hypothesis about the Mean (σ Known) at 0.05 Significance Level 0.025 0.95 0.025 Accepted region Rejected Rejected region region - C.V. + C.V. Z Z PARAMETRIC VS NON-PARAMETRIC TESTS In statistics, parametric tests and non-parametric tests are two broad categories of hypothesis tests that are used to assess different types of data. The key difference between them lies in the assumptions they make about the underlying data distribution. Parametric Tests Parametric tests assume that the data follow a certain distribution, usually a normal distribution. They are based on parameters (e.g., mean, standard deviation) and rely on assumptions about the population from which the sample is drawn. Key Features of Parametric Tests: Assume normality: Parametric tests assume that the data is approximately normally distributed. This assumption is especially important for small sample sizes. Dante P. Sardina Republic of the Philippines City of Olongapo GORDON COLLEGE Olongapo City Sports Complex, Donor St., East Tapinac, Olongapo City Use of parameters: These tests typically rely on parameters such as the population mean and standard deviation. Require interval or ratio data: The data used in parametric tests must be continuous (interval or ratio scale). More powerful: Parametric tests are generally more powerful, meaning they are more likely to detect a significant effect if one exists. Common Parametric Tests: One-sample t-test: Compares the sample mean to a known value (e.g., hypothesized population mean). Independent samples t-test: Compares the means of two independent groups. Paired samples t-test: Compares the means of two related groups (e.g., before and after treatment). One-way ANOVA: Compares the means of three or more independent groups. Pearson’s Correlation: Measures the linear relationship between two continuous variables. When to Use Parametric Tests: Data is normally distributed or approximately so. Data is continuous (interval or ratio scale). Sample size is large enough to rely on the central limit theorem, which states that the sampling distribution of the sample mean will approximate a normal distribution. Non-Parametric Tests Non-parametric tests, also known as distribution-free tests, do not assume a specific distribution for the data. These tests are more flexible and can be used when the data does not meet the assumptions required by parametric tests. Key Features of Non-Parametric Tests: No assumptions about distribution: Non-parametric tests do not require the data to follow a normal distribution. They are useful for skewed, ordinal, or categorical data. Based on ranks or categories: These tests often work with ranks (for continuous data) or frequencies (for categorical data), rather than actual values. Less powerful: Non-parametric tests are generally less powerful than parametric tests because they use less information (e.g., ranks instead of actual data values). Common Non-Parametric Tests: Mann-Whitney U test: The non-parametric equivalent of the independent samples t-test; compares the distributions of two independent groups. Wilcoxon Signed-Rank Test: The non-parametric equivalent of the paired samples t-test; compares the differences between paired data points. Dante P. Sardina Republic of the Philippines City of Olongapo GORDON COLLEGE Olongapo City Sports Complex, Donor St., East Tapinac, Olongapo City Kruskal-Wallis H Test: The non-parametric equivalent of one-way ANOVA; compares the distributions of three or more independent groups. Spearman’s Rank Correlation: The non-parametric equivalent of Pearson’s correlation; measures the relationship between two variables based on ranks. Chi-square Test: A non-parametric test for categorical data that assesses the association between two variables. When to Use Non-Parametric Tests: Data is not normally distributed. Data is ordinal (ranked categories) or nominal (categorical). The sample size is small and the assumptions of parametric tests cannot be met. Key Differences Between Parametric and Non-Parametric Tests Feature Parametric Tests Non-Parametric Tests Assume normal distribution and known Assumptions No assumption about the distribution. parameters (mean, variance). Ordinal or nominal data; can be used for Data Type Continuous data (interval or ratio). continuous data with non-normal distribution. Mean, standard deviation, t-value, F- Ranks, frequencies, chi-square, U- Test Statistics value. statistic. Generally, more powerful when Less powerful; relies on ranks or Power assumptions are met. categories. Used when data is skewed, ordinal, or Used when data is approximately Use Case categorical, or when sample sizes are normal, and for larger sample sizes. small. One-sample t-test, ANOVA, Pearson Mann-Whitney U test, Wilcoxon Signed- Examples correlation. Rank test, Spearman correlation. When to Choose Parametric vs Non-Parametric Tests Normal Distribution: If your data is normally distributed and meets other assumptions (e.g., homogeneity of variance for t-tests or ANOVA), parametric tests are usually the preferred choice as they are more powerful and efficient. - If the data is not normally distributed, then non-parametric tests should be used. Dante P. Sardina Republic of the Philippines City of Olongapo GORDON COLLEGE Olongapo City Sports Complex, Donor St., East Tapinac, Olongapo City Data Type: If your data is continuous (e.g., test scores, heights, weights), you can generally use parametric tests, provided the distribution assumptions are met. If your data is ordinal (ranked, such as Likert scale) or nominal (categorical, such as gender or color), you should choose non-parametric tests. Sample Size: Parametric tests typically require larger sample sizes to achieve reliable results. Non-parametric tests can be useful for small sample sizes or when the data is not normally distributed. STEPS FOR HYPOTHESIS TESTING USING THE P-VALUE METHOD IN SPSS Step1. State the Hypotheses and identify the claim. Null Hypothesis (H₀): The population parameter (mean, proportion) is equal to a specified value. Alternative Hypothesis (H₁): The population parameter is different from the specified value. Step 2. Set the Significance level Arbitrary significance levels: 1%, 5%, and 10% Step 3. Select the Appropriate Test and Calculate the Test Statistic Test the normality Select appropriate test Calculate the test statistic Step 4. Compute the p-value You use statistical software to find that the p-value Step 5. Make a decision Reject or fail to reject the null hypothesis by comparing the p value to alpha () - If p ≤ α, reject H₀. - If p > α, fail to reject H₀. Example: Since the p-value > α, you fail to reject the null hypothesis. This means there is not enough evidence to suggest that the average score is different from 75. Step 6. Interpret the Result or make a conclusion Dante P. Sardina Republic of the Philippines City of Olongapo GORDON COLLEGE Olongapo City Sports Complex, Donor St., East Tapinac, Olongapo City REFERENCES Bluman, A. G. (2009). Elementary Statistics: A Step by Step Approach (Seven). McGraw-Hill Companies, Inc. Turner, D. P., Deng, H., & Houle, T. T. (2020). Statistical hypothesis testing: overview and application. Headache: The Journal of Head and Face Pain, 60(2), 302-308. https://doi.org/10.1111/head.13706 Curran‐Everett, D. (2009). Explorations in statistics: hypothesis tests and p values. Advances in Physiology Education, 33(2), 81-86. https://doi.org/10.1152/advan.90218.2008 Newman, M. C. (2008). “what exactly are you inferring?” a closer look at hypothesis testing. Environmental Toxicology and Chemistry, 27(5), 1013-1019. https://doi.org/10.1897/07-373.1 Ratain, M. J. and Karrison, T. (2007). Testing the wrong hypothesis in phase ii oncology trials: there is a better alternative. Clinical Cancer Research, 13(3), 781-782. https://doi.org/10.1158/1078-0432.ccr-06- 2533 Huang, P., Ou, A., Piantadosi, S., & Tan, M. (2014). Formulating appropriate statistical hypotheses for treatment comparison in clinical trial design and analysis. Contemporary Clinical Trials, 39(2), 294-302. https://doi.org/10.1016/j.cct.2014.09.005 Graaf, T. A. d. and Sack, A. T. (2018). When and how to interpret null results in nibs: a taxonomy based on prior expectations and experimental design. Frontiers in Neuroscience, 12. https://doi.org/10.3389/fnins.2018.00915 Brereton, R. G. (2020). Alpha, beta, type 1 and 2 errors, ergon pearson and jerzy neyman. Journal of Chemometrics, 35(3). https://doi.org/10.1002/cem.3240 Ratain, M. J. and Karrison, T. (2007). Testing the wrong hypothesis in phase ii oncology trials: there is a better alternative. Clinical Cancer Research, 13(3), 781-782. https://doi.org/10.1158/1078-0432.ccr-06- 2533 Huang, P., Ou, A., Piantadosi, S., & Tan, M. (2014). Formulating appropriate statistical hypotheses for treatment comparison in clinical trial design and analysis. Contemporary Clinical Trials, 39(2), 294-302. https://doi.org/10.1016/j.cct.2014.09.005 Montalbán, J. M. C., Olmos, R., & Pardo, A. (2016). Inconsistencies in reportedp-values in spanish journals of psychology. Methodology, 12(2), 44-51. https://doi.org/10.1027/1614-2241/a000107 Prescott, R. (2019). Two‐tailed significance tests for 2 × 2 contingency tables: what is the alternative?. Statistics in Medicine, 38(22), 4264-4269. https://doi.org/10.1002/sim.8294 Curran‐Everett, D. (2009). Explorations in statistics: hypothesis tests and p values. Advances in Physiology Education, 33(2), 81-86. https://doi.org/10.1152/advan.90218.2008 Ruxton, G. D. and Neuhäuser, M. (2010). When should we use one‐tailed hypothesis testing?. Methods in Ecology and Evolution, 1(2), 114-117. https://doi.org/10.1111/j.2041-210x.2010.00014.x Dante P. Sardina Republic of the Philippines City of Olongapo GORDON COLLEGE Olongapo City Sports Complex, Donor St., East Tapinac, Olongapo City Lombardi, C. M. and Hurlbert, S. H. (2009). Misprescription and misuse of one‐tailed tests. Austral Ecology, 34(4), 447-468. https://doi.org/10.1111/j.1442-9993.2009.01946.x Hurlbert, S. H. and Lombardi, C. M. (2012). Lopsided reasoning on lopsided tests and multiple comparisons. Australian &Amp; New Zealand Journal of Statistics, 54(1), 23-42. https://doi.org/10.1111/j.1467-842x.2012.00652.x Serlin, R. C. (2000). Answering two criticisms of hypothesis testing: a comment. Psychological Reports, 87(2), 579-581. https://doi.org/10.2466/pr0.2000.87.2.579 Vermeesch, P. (2011). Statistical significance does not equal geological significance: reply to comments on “lies, damned lies, and statistics (in geology)”. Eos, Transactions American Geophysical Union, 92(8), 66-66. https://doi.org/10.1029/2011eo080013 Christley, R. (2010). Power and error: increased risk of false positive results in underpowered studies. The Open Epidemiology Journal, 3(1), 16-19. https://doi.org/10.2174/1874297101003010016 Zerem, E. (2014). Farmakologija renin-angiotenzin sistema. Acta Medica Academica, 43(2), 174-175. https://doi.org/10.5644/ama2006-124.119 Jiménez-Gamero, I. and Analla, M. (2023). The importance of type ii error in hypothesis testing. International Journal of Statistics and Probability, 12(2), 42. https://doi.org/10.5539/ijsp.v12n2p42 Dash, B. and Ali, A. (2023). Importance of hypothesis testing, type i, and type ii errors – a study of statistical power. SSRN Electronic Journal. https://doi.org/10.2139/ssrn.4575635 Kelter, R. (2020). Analysis of type i and ii error rates of bayesian and frequentist parametric and nonparametric two-sample hypothesis tests under preliminary assessment of normality. Computational Statistics, 36(2), 1263-1288. https://doi.org/10.1007/s00180-020-01034-7 Mudge, J. F., Baker, L. F., Edge, C. B., & Houlahan, J. E. (2012). Setting an optimal α that minimizes errors in null hypothesis significance tests. PLoS ONE, 7(2), e32734. https://doi.org/10.1371/journal.pone.0032734 Zerem, E. (2014). Farmakologija renin-angiotenzin sistema. Acta Medica Academica, 43(2), 174-175. https://doi.org/10.5644/ama2006-124.119 Owusu-Ansah, E. d. J., Sampson, A., Samuel, A. K., & Robert, A. W. (2016). Sensitivity and specificity analysis relation to statistical hypothesis testing and its errors: application to cryptosporidium detection techniques. Open Journal of Applied Sciences, 06(04), 209-216. https://doi.org/10.4236/ojapps.2016.64022 Jamil, Muhammad Yasir. Hypothesis Testing in SPSS: Comprehensive Guide. 24 Oct. 2024, https://medicalbiochem.com/hypothesis-testing-in-spss/, https://medicalbiochem.com/hypothesis- testing-in-spss/. Tsushima, E. (2022). Interpreting results from statistical hypothesis testing: understanding the appropriate p-value. Physical Therapy Research, 25(2), 49-55. https://doi.org/10.1298/ptr.r0019 Dante P. Sardina Republic of the Philippines City of Olongapo GORDON COLLEGE Olongapo City Sports Complex, Donor St., East Tapinac, Olongapo City Castelnuovo, A. D. and Iacoviello, L. (2022). Moving beyond p-value. Bleeding, Thrombosis, and Vascular Biology, 1(1). https://doi.org/10.4081/btvb.2022.30 In, J. and Lee, D. K. (2024). Alternatives to the p value: connotations of significance. Korean Journal of Anesthesiology, 77(3), 316-325. https://doi.org/10.4097/kja.23630 Hopkins, W. G. (2022). Replacing statistical significance and non-significance with better approaches to sampling uncertainty. Frontiers in Physiology, 13. https://doi.org/10.3389/fphys.2022.962132 Lakens, D. and Delacre, M. (2020). Equivalence testing and the second generation p-value. Meta- Psychology, 4. https://doi.org/10.15626/mp.2018.933 Leo, G. D. and Sardanelli, F. (2020). Statistical significance: p value, 0.05 threshold, and applications to radiomics—reasons for a conservative approach. European Radiology Experimental, 4(1). https://doi.org/10.1186/s41747-020-0145-y Kwak, S. G. (2023). Are only p-values less than 0.05 significant? a p-value greater than 0.05 is also significant!. Journal of Lipid and Atherosclerosis, 12(2), 89. https://doi.org/10.12997/jla.2023.12.2.89 Cohen, J. (1988). Statistical Power Analysis for the Behavioral Sciences (2nd ed.). Hillsdale, NJ: Lawrence Erlbaum Associates. Fisher, R. A. (1925). Statistical Methods for Research Workers. Edinburgh: Oliver and Boyd. Neyman, J., & Pearson, E. S. (1933). On the Problem of the Most Efficient Tests of Statistical Hypotheses. Philosophical Transactions of the Royal Society of London. Series A, Mathematical and Physical Sciences, 231(694-706), 289-337. Wasserstein, R. L., & Lazar, N. A. (2016). The ASA's Statement on P-Values: Context, Process, and Purpose. The American Statistician, 70(1), 129-133. Dante P. Sardina