Week 7 - Mode Choice Part I PDF
Document Details
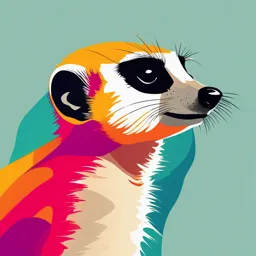
Uploaded by FinestPlatypus
UNSW Sydney
2022
null
Taha Rashidi
Tags
Related
- RATP Group Presentation PDF
- PTE 413 Urban Transport Planning in the Philippines (Module 2) - Don Honorio Ventura State University - PDF
- Bridging: Transportation - Chapter 8 - Urban Transportation - PDF
- L7 Transportation-2 PDF - Urban Mobility & Transport
- Gyroscopic Transportation - SVS English Medium School
- Module 1: Introduction to Transportation Planning and Engineering PDF
Summary
This document is a lecture/presentation on urban transport planning practice, specifically focusing on mode choice. The document covers various topics such as factors influencing mode choice, utility theory, and probabilistic choice modelling (including the multinomial logit model).
Full Transcript
CVEN9405: Urban Transport Planning Practice Mode Choice Part (I) Taha Rashidi 23th October 2022 Overview • What is Mode Choice? • Modelling Mode Choice • Utility Theory – Utility functions – Variable and parameter types • Probabilistic Choice modelling – Multinomial Logit Model • Estim...
CVEN9405: Urban Transport Planning Practice Mode Choice Part (I) Taha Rashidi 23th October 2022 Overview • What is Mode Choice? • Modelling Mode Choice • Utility Theory – Utility functions – Variable and parameter types • Probabilistic Choice modelling – Multinomial Logit Model • Estimation of Logit Models • Goodness of Fit Statistics CVEN 9405: Urban Transport Planning Practice 2 What is Mode Choice? The 4-step Transport Model • • • • CVEN 9405: Urban Transport Planning Practice Trip Generation (Weeks 2 and 3) – The number of trips generated by a land use (How many for travel?) Trip Distribution (Weeks 5 and 7) – Determination of the origins and destinations of the generated trips (Where people travel from?) Mode Split/Mode Choice (Weeks 8 and 9) – The distribution of travel across the available transport modes in a network. (How do they travel between origin and destination?) Traffic Assignment (Weeks 9 and 10) – For each mode, what links of the transport network are used to travel between origin and destination. (What routes do they select?) 3 What is Mode Choice? • The choice of a transport mode between two zones in a network. – Individuals make mode choices in a wide variety of decision contexts o Work or education, residential location, time of departure, etc. – Not all options (alternatives) are available to all individuals – Some alternatives will become available in the near future. E.g. Light Rail to UNSW – In real life, an analyst generally observes their final decision and characteristics of available/future options. CVEN 9405: Urban Transport Planning Practice 4 What is Mode Choice? • The importance of mode choice. – By collecting data on the choices individuals make, we can: o Estimate the mode share (or probability) for a given mode o Understand what factors affect mode choice o Suggest policies to improve key factors, thus increasing mode share – Estimate the travel demand for each mode (existing or proposed) • Implications – Compute the resulting traffic impact on the transport network for the travel demand – Suggest measures aimed at alleviating congestion Traffic Assignment Thus, mode choice feeds traffic assignment CVEN 9405: Urban Transport Planning Practice 5 What is Mode Choice? • Factors affecting mode choice – Characteristics of the journey: o Trip purpose: Commute, recreational, etc. o Trip timing: Peak versus off-peak o Number of people taking the trip: Individual or group – Characteristics of the traveller: (Individual characteristics) o Income. o Car availability/ownership o Household size o Age o and many more… CVEN 9405: Urban Transport Planning Practice 6 What is Mode Choice? • Factors affecting mode choice – Characteristics of the transport infrastructure: (attributes of alternatives) o Travel time: In-vehicle, waiting time, walking time o Monetary costs: Fares, tolls, fuel, operating costs o Accessibility: Can the mode take a traveller to the front door? o Reliability: Road versus Rail o Comfort and convenience: Private versus Public transport o Safety and security CVEN 9405: Urban Transport Planning Practice 7 Modelling Mode Choice • Why? – Need to forecast ridership on the proposed new or improved services o Identify the modes from which existing travellers will be diverted to new (or improved) services. – Determine the order of importance of attributes that affect mode choice • How? – Using Discrete Choice Modelling Framework o Goal: Develop probabilistic models to forecast individual choices using available information o Quite relevant in analysing choice data o Widely used in engineering, medicine and many more disciplines We discuss the Discrete Choice Modelling Framework in this lecture CVEN 9405: Urban Transport Planning Practice 8 Activity: Mobile Phone Choice Please respond to the following survey: Mobile Phone Choice Survey . The following options are available to you in the market. – iPhone 13 – Samsung Galaxy S21 Ultra – Google Pixel 3 CVEN 9405: Urban Transport Planning Practice 9 Which one of the following smartphones should you like to buy? ⓘ Start presenting to display the poll results on this slide. Activity: Mobile Phone Choice Answer the following: 1. What is the data type of a variable that represents mobile phone choice? A: Discrete/multinomial iPhone, Samsung Galaxy and Google Pixel 2. Is linear regression an appropriate model to predict the mobile phone choice? A: No 3. Give a reason why linear regression is not suitable in this problem. Let’s discuss why? CVEN 9405: Urban Transport Planning Practice 11 Limitation: Linear Regression on Multinomial variable For a new person aged 25 years: Linear regression predicts an outcome of 1.7 Which is unrealistic!! Thus, discrete choice modelling involve models where probability of observing an outcome is calculated and not the direct outcome itself CVEN 9405: Urban Transport Planning Practice 12 Utility Theory Utility: • The level of satisfaction (or dis-satisfaction) a consumer derives from an alternative • It is a latent quantity Hard to observe and measure (dimensionless) • Can be interpreted in two ways: – Positive utility which represents the level of satisfaction o E.g. The satisfaction one derives from using an iPhone – Negative utility (or disutility) which signifies the level of dis-satisfaction o E.g. As travel becomes more expensive, disutility increases Consumer Theory: A consumer will choose the types and quantities of goods that maximise their utility Higher the utility for an alternative, higher is the probability of it being selected by an individual CVEN 9405: Urban Transport Planning Practice 13 Utility Theory Random Utility Maximisation: • Originated in the field of economics from Consumer Theory • It states that a rational consumer will choose a product (alternative) which offers the maximum utility • • Consider that N individuals have a choice set comprising J alternatives. According to RUM, an individual 𝑛𝑛 will select option 𝑗𝑗 only if: 𝑼𝑼𝒏𝒏𝒏𝒏 > 𝑼𝑼𝒏𝒏𝒏𝒏 ∀ 𝒌𝒌 ∈ 𝟏𝟏. . 𝑱𝑱 − {𝒋𝒋} Example: If a person 𝑛𝑛 chose an iPhone then as per RUM 𝑈𝑈𝑛𝑛𝑖𝑖𝑖𝑖𝑖𝑖𝑖𝑖𝑖𝑖𝑖 > 𝑈𝑈𝑛𝑛𝑆𝑆𝑆𝑆𝑆𝑆𝑆𝑆𝑆𝑆𝑆𝑆𝑆𝑆 Challenge: How do we measure utility? 𝑎𝑎𝑎𝑎𝑎𝑎 𝑈𝑈𝑛𝑛𝑛𝑛𝑛𝑛𝑛𝑛𝑛𝑛𝑛𝑛𝑛 > 𝑈𝑈𝑛𝑛𝐺𝐺𝐺𝐺𝐺𝐺𝐺𝐺𝐺𝐺𝐺𝐺 The fact that utility cannot be directly measured is the main reason behind the existence of discrete choice modelling CVEN 9405: Urban Transport Planning Practice 14 Utility Theory • Utility Functions – The total utility of alternative 𝑗𝑗 for an individual 𝑛𝑛 can be expressed as a sum of observable utility and an error term. 𝑼𝑼𝒏𝒏𝒏𝒏 = 𝑽𝑽𝒏𝒏𝒏𝒏 + 𝜺𝜺𝒏𝒏𝒏𝒏 ∀ 𝒋𝒋 ∈ 𝑱𝑱; 𝒏𝒏 ∈ 𝑵𝑵 Where 𝑉𝑉𝑛𝑛𝑛𝑛 = Observed utility of alternative 𝑗𝑗 for individual 𝑛𝑛 𝜀𝜀𝑛𝑛𝑛𝑛 = An error term which corresponds to the unobserved utility of alternative 𝑗𝑗 for individual 𝑛𝑛 – The observed utility for each alternative can be expressed in terms of alternative specific attributes and person specific attributes (also called independent variables) o Alternative specific attributes: Travel time, travel cost of each mode of transport Cost and battery life of each mobile phone alternative Different for all alternatives o Generic (person specific) attributes: Age, Gender, Income Attitude CVEN 9405: Urban Transport Planning Practice Same across all alternatives 15 Utility Theory Observed Utility Function • Utility can be represented as a weighted sum of independent variables 𝑽𝑽𝒏𝒏𝒏𝒏 = 𝜶𝜶𝒋𝒋 + 𝜷𝜷𝟏𝟏 𝑿𝑿𝒏𝒏𝒏𝒏𝟏𝟏 + 𝜷𝜷𝟐𝟐 𝑿𝑿𝒏𝒏𝒏𝒏𝟐𝟐 + ⋯ + 𝜸𝜸𝟏𝟏𝟏𝟏 𝒁𝒁𝒏𝒏𝒏𝒏 + 𝜸𝜸𝟐𝟐𝒋𝒋 𝒁𝒁𝒏𝒏𝟐𝟐 + ⋯ Where ∀ 𝒋𝒋 ∈ 𝑱𝑱; 𝒏𝒏 ∈ 𝑵𝑵 𝑋𝑋𝑛𝑛𝑛𝑛𝑛𝑛 = The set of 𝑖𝑖 attributes specific to alternative 𝑗𝑗 for individual 𝑛𝑛 {𝑋𝑋𝑛𝑛𝑛𝑛1 , 𝑋𝑋𝑛𝑛𝑛𝑛2 , … } 𝑍𝑍𝑛𝑛𝑛𝑛 = The set of 𝑘𝑘 attributes specific to individual 𝑛𝑛 {𝑍𝑍𝑛𝑛𝑛 , 𝑍𝑍𝑛𝑛𝑛 , … } 𝛼𝛼, 𝛽𝛽, 𝛾𝛾 = The set of parameters to be calibrated Alternative specific variables Generic variables Lets looks at an example dataset CVEN 9405: Urban Transport Planning Practice 16 Utility Theory Mobile Phone Choice Survey 23 Dependent Variable Alternate specific variables (different across alternatives) CVEN 9405: Urban Transport Planning Practice Generic variables (same across alternatives) 17 Utility Theory Utility Specification Types of parameters There are three types of parameters: 1. Alternative specific constants (α) 𝑽𝑽𝒏𝒏𝒏𝒏 = 𝜶𝜶𝒋𝒋 + 𝜷𝜷𝟏𝟏 𝑿𝑿𝒏𝒏𝒏𝒏𝒏𝒏 + 𝜷𝜷𝟐𝟐 𝑿𝑿𝒏𝒏𝒏𝒏𝒏𝒏 + ⋯ + 𝜸𝜸𝟏𝟏𝒋𝒋 𝒁𝒁𝒏𝒏𝒏𝒏 + 𝜸𝜸𝟐𝟐𝒋𝒋 𝒁𝒁𝒏𝒏𝒏𝒏 + ⋯ CVEN 9405: Urban Transport Planning Practice 18 Utility Theory Utility Specification Types of parameters There are three types of parameters: 1. Alternative specific constants (α) 2. Generic parameters ( β) 𝑽𝑽𝒏𝒏𝒏𝒏 = 𝜶𝜶𝒋𝒋 + 𝜷𝜷𝟏𝟏 𝑿𝑿𝒏𝒏𝒏𝒏𝒏𝒏 + 𝜷𝜷𝟐𝟐 𝑿𝑿𝒏𝒏𝒏𝒏𝒏𝒏 + ⋯ + 𝜸𝜸𝟏𝟏𝒋𝒋 𝒁𝒁𝒏𝒏𝒏𝒏 + 𝜸𝜸𝟐𝟐𝒋𝒋 𝒁𝒁𝒏𝒏𝒏𝒏 + ⋯ CVEN 9405: Urban Transport Planning Practice 19 Utility Theory Utility Specification Types of parameters There are three types of parameters: 1. Alternative specific constants (α) 2. Generic parameters ( β) 3. Alternative specific parameters (γ) 𝑽𝑽𝒏𝒏𝒏𝒏 = 𝜶𝜶𝒋𝒋 + 𝜷𝜷𝟏𝟏 𝑿𝑿𝒏𝒏𝒏𝒏𝒏𝒏 + 𝜷𝜷𝟐𝟐 𝑿𝑿𝒏𝒏𝒏𝒏𝒏𝒏 + ⋯ + 𝜸𝜸𝟏𝟏𝒋𝒋 𝒁𝒁𝒏𝒏𝒏𝒏 + 𝜸𝜸𝟐𝟐𝒋𝒋 𝒁𝒁𝒏𝒏𝒏𝒏 + ⋯ General thumb rule: • Generic parameter X Alternative specific variable • Alternative specific parameter X Generic variable • For this course Sometimes it can be Alternative specific parameter X Alternative specific variable CVEN 9405: Urban Transport Planning Practice 20 Utility Theory 1. Alternative specific constants (ASC) • Accounts for the unobserved factors that were not captured by the analyst. E.g Camera resolution, RAM size as attributes • An ASC associates a non-zero probability to an alternative if all independent variables are set to zero • Also referred to as the brand effect 𝑽𝑽𝒏𝒏𝒏𝒏 = 𝜶𝜶𝒋𝒋 + 𝜷𝜷𝟏𝟏 𝑿𝑿𝒏𝒏𝒏𝒏𝒏𝒏 + 𝜷𝜷𝟐𝟐 𝑿𝑿𝒏𝒏𝒏𝒏𝒏𝒏 + ⋯ + 𝜸𝜸𝟏𝟏𝒋𝒋 𝒁𝒁𝒏𝒏𝒏𝒏 + 𝜸𝜸𝟐𝟐𝒋𝒋 𝒁𝒁𝒏𝒏𝒏𝒏 + ⋯ Thumb Rule • ASC for only J-1 alternatives can be determined. ASC for 1 alternative is fixed to ZERO and is the reference category CVEN 9405: Urban Transport Planning Practice 21 Utility Theory 1. Alternative specific constants (ASC) – Example 𝑽𝑽𝒏𝒏𝒏𝒏𝒏𝒏𝒏𝒏 = 𝟓𝟓. 𝟏𝟏 + … 𝑽𝑽𝒏𝒏𝒏𝒏𝒏𝒏 = 𝟐𝟐. 𝟓𝟓 + … 𝑽𝑽𝒏𝒏𝒏𝒏𝒏𝒏 = 𝟎𝟎 + ⋯ GP is the reference category. If all attributes are zero, still iPhone11 has a higher utility when compared to Google Pixel 𝑽𝑽𝒏𝒏𝒏𝒏𝒏𝒏𝒏𝒏 = 𝟓𝟓. 𝟏𝟏 + … 𝑽𝑽𝒏𝒏𝒏𝒏𝒏𝒏 = 𝟐𝟐. 𝟓𝟓 + … 𝑽𝑽𝒏𝒏𝒏𝒏𝒏𝒏 = 𝟒𝟒. 𝟏𝟏 + ⋯ 𝑽𝑽𝒏𝒏𝒏𝒏𝒏𝒏𝒏𝒏 = 𝟎𝟎 + … 𝑽𝑽𝒏𝒏𝒏𝒏𝒏𝒏 = −𝟏𝟏. 𝟕𝟕 + … 𝑽𝑽𝒏𝒏𝒏𝒏𝒏𝒏 = 𝟎𝟎 + ⋯ iPhone and GP are the reference categories. If all attributes are zero, still Samsung has a lower when compared to iPhone and Google Pixel CVEN 9405: Urban Transport Planning Practice 22 Utility Theory 2. Generic parameters • Assign a weight to alternative specific variables which are different across alternatives 𝑽𝑽𝒏𝒏𝒏𝒏 = 𝜶𝜶𝒋𝒋 + 𝜷𝜷𝟏𝟏 𝑿𝑿𝒏𝒏𝒏𝒏𝒏𝒏 + 𝜷𝜷𝟐𝟐 𝑿𝑿𝒏𝒏𝒏𝒏𝒏𝒏 + ⋯ + 𝜸𝜸𝟏𝟏𝒋𝒋 𝒁𝒁𝒏𝒏𝒏𝒏 + 𝜸𝜸𝟐𝟐𝒋𝒋 𝒁𝒁𝒏𝒏𝒏𝒏 + ⋯ Thumb Rule • Same parameter value for each alternative CVEN 9405: Urban Transport Planning Practice 23 Utility Theory 2. Generic parameters – Example 𝑽𝑽𝒏𝒏𝒏𝒏𝒏𝒏𝒏𝒏 = 𝟓𝟓. 𝟏𝟏 + 𝟎𝟎. 𝟓𝟓 ∗ 𝑪𝑪𝑪𝑪𝑪𝑪𝑪𝑪_𝒊𝒊𝒊𝒊𝒊𝒊 … 𝑽𝑽𝒏𝒏𝒏𝒏𝒏𝒏 = 𝟎𝟎 + 𝟏𝟏. 𝟕𝟕 ∗ 𝑪𝑪𝑪𝑪𝑪𝑪𝑪𝑪_𝑺𝑺𝑺𝑺 … 𝑽𝑽𝒏𝒏𝒏𝒏𝒏𝒏 = −𝟐𝟐. 𝟏𝟏 + 𝟐𝟐. 𝟖𝟖 ∗ 𝑪𝑪𝑪𝑪𝑪𝑪𝑪𝑪_𝑮𝑮𝑮𝑮 … SG is the reference category. If all attributes are zero, still iPhone11 has a higher utility when compared to Samsung If all attributes are zero, still Google has a lower utility when compared to Samsung An increase in the costs of iPhone, Samsung and Google phones increases the utility CVEN 9405: Urban Transport Planning Practice 24 Utility Theory 2. Generic parameters – Example 𝑽𝑽𝒏𝒏𝒏𝒏𝒏𝒏𝒏𝒏 = 𝟓𝟓. 𝟏𝟏 − 𝟎𝟎. 𝟓𝟓 ∗ 𝑪𝑪𝑪𝑪𝑪𝑪𝑪𝑪_𝒊𝒊𝒊𝒊𝒊𝒊 … 𝑽𝑽𝒏𝒏𝒏𝒏𝒏𝒏 = 𝟎𝟎 − 𝟏𝟏. 𝟕𝟕 ∗ 𝑪𝑪𝑪𝑪𝑪𝑪𝑪𝑪_𝑺𝑺𝑺𝑺 … 𝑽𝑽𝒏𝒏𝒏𝒏𝒏𝒏 = −𝟐𝟐. 𝟏𝟏 − 𝟐𝟐. 𝟖𝟖 ∗ 𝑪𝑪𝑪𝑪𝑪𝑪𝑪𝑪_𝑮𝑮𝑮𝑮 … SG is the reference category. If all attributes are zero, still iPhone11 has a higher utility when compared to Samsung If all attributes are zero, still Google has a lower utility when compared to Samsung An increase in the costs of iPhone, Samsung and Google phones decreases the utility CVEN 9405: Urban Transport Planning Practice 25 Utility Theory 3. Alternative specific parameters • Assign a weight to generic variables such as person specific information which remains the same across alternatives 𝑽𝑽𝒏𝒏𝒏𝒏 = 𝜶𝜶𝒋𝒋 + 𝜷𝜷𝟏𝟏 𝑿𝑿𝒏𝒏𝒏𝒏𝒏𝒏 + 𝜷𝜷𝟐𝟐 𝑿𝑿𝒏𝒏𝒏𝒏𝒏𝒏 + ⋯ + 𝜸𝜸𝟏𝟏𝒋𝒋 𝒁𝒁𝒏𝒏𝒏𝒏 + 𝜸𝜸𝟐𝟐𝒋𝒋 𝒁𝒁𝒏𝒏𝒏𝒏 + ⋯ Thumb Rule • Parameters for only J-1 alternatives can be determined. Value for 1 alternative is fixed to ZERO and is the reference category CVEN 9405: Urban Transport Planning Practice 26 Utility Theory 3. Alternative specific parameters - Example 𝑽𝑽𝒏𝒏𝒏𝒏𝒏𝒏𝒏𝒏 = 𝟓𝟓. 𝟏𝟏 − 𝟎𝟎. 𝟓𝟓 ∗ 𝑪𝑪𝑪𝑪𝑪𝑪𝑪𝑪_𝒊𝒊𝒊𝒊𝒊𝒊 + 𝟑𝟑. 𝟓𝟓 ∗ 𝑮𝑮𝑮𝑮𝑮𝑮𝑮𝑮𝑮𝑮𝑮𝑮 … 𝑽𝑽𝒏𝒏𝒏𝒏𝒏𝒏 = 𝟎𝟎 − 𝟏𝟏. 𝟕𝟕 ∗ 𝑪𝑪𝑪𝑪𝑪𝑪𝑪𝑪_𝑺𝑺𝑺𝑺 + 𝟎𝟎 ∗ 𝑮𝑮𝑮𝑮𝑮𝑮𝑮𝑮𝑮𝑮𝑮𝑮 … 𝑽𝑽𝒏𝒏𝒏𝒏𝒏𝒏 = −𝟐𝟐. 𝟏𝟏 − 𝟐𝟐. 𝟖𝟖 ∗ 𝑪𝑪𝑪𝑪𝑪𝑪𝑪𝑪_𝑮𝑮𝑮𝑮 − 𝟏𝟏. 𝟏𝟏 ∗ 𝑮𝑮𝑮𝑮𝑮𝑮𝑮𝑮𝑮𝑮𝑮𝑮 … SG is the reference category. If all attributes are zero, still iPhone11 has a higher utility when compared to Samsung If all attributes are zero, still Google has a lower utility when compared to Samsung An increase in the costs of iPhone, Samsung and Google phones decreases the utility Males get higher utility from iPhone when compared to Samsung Males get lower utility from Google when compared to Samsung 𝑽𝑽𝒏𝒏𝒏𝒏𝒏𝒏𝒏𝒏 = 𝟓𝟓. 𝟏𝟏 + 𝟎𝟎. 𝟓𝟓 ∗ 𝑪𝑪𝑪𝑪𝑪𝑪𝑪𝑪_𝒊𝒊𝒊𝒊𝒊𝒊 + 𝟑𝟑. 𝟓𝟓 ∗ 𝑮𝑮𝑮𝑮𝑮𝑮𝑮𝑮𝑮𝑮𝑮𝑮 … 𝑽𝑽𝒏𝒏𝒏𝒏𝒏𝒏 = 𝟎𝟎 + 𝟏𝟏. 𝟕𝟕 ∗ 𝑪𝑪𝑪𝑪𝑪𝑪𝒕𝒕𝑺𝑺𝑺𝑺 + 𝟐𝟐. 𝟏𝟏 ∗ 𝑮𝑮𝑮𝑮𝑮𝑮𝑮𝑮𝑮𝑮𝑮𝑮 … 𝑽𝑽𝒏𝒏𝒏𝒏𝒏𝒏 = −𝟐𝟐. 𝟏𝟏 + 𝟐𝟐. 𝟖𝟖 ∗ 𝑪𝑪𝑪𝑪𝑪𝑪𝑪𝑪_𝑮𝑮𝑮𝑮 − 𝟎𝟎 ∗ 𝑮𝑮𝑮𝑮𝑮𝑮𝑮𝑮𝑮𝑮𝑮𝑮 … How do we get these parameter values? Will be answered shortly AVOID such utility specifications CVEN 9405: Urban Transport Planning Practice 27 Utility Theory We saw earlier: • Consider that N individuals have a choice set comprising J alternatives. According to RUM, an individual 𝑛𝑛 will select option 𝑗𝑗 only if: 𝑼𝑼𝒏𝒏𝒏𝒏 > 𝑼𝑼𝒏𝒏𝒏𝒏 𝑼𝑼𝒏𝒏𝒏𝒏 = 𝑽𝑽𝒏𝒏𝒏𝒏 + 𝜺𝜺𝒏𝒏𝒏𝒏 ∀ 𝒌𝒌 ∈ 𝟏𝟏. . 𝑱𝑱 − {𝒋𝒋} ∀ 𝒋𝒋 ∈ 𝑱𝑱; 𝒏𝒏 ∈ 𝑵𝑵 Where 𝑉𝑉𝑛𝑛𝑛𝑛 = Observed utility of alternative 𝑗𝑗 for individual 𝑛𝑛 𝜀𝜀𝑛𝑛𝑛𝑛 = An error term which corresponds to the unobserved utility of alternative 𝑗𝑗 for individual 𝑛𝑛 We learnt how to determine 𝑉𝑉𝑛𝑛𝑛𝑛 𝜀𝜀𝑛𝑛𝑛𝑛 is assumed to follow some distribution which gives rise to probabilistic choice models CVEN 9405: Urban Transport Planning Practice 28 Probabilistic Choice Modelling • It is important to note that the error term is to differentiate between two otherwise identical observed utilities • The mathematical form of a probabilistic model is determined by the distribution of the error term: o 𝜀𝜀𝑛𝑛𝑛𝑛 follows Gumbel Distribution (EV-1) Logit Model o 𝜀𝜀𝑛𝑛𝑛𝑛 follows Normal Distribution Probit Model Out of scope Multinomial Logit Model (MNL) Probabilistic Model: Gives the probability that an individual with observed attributes selects an alternative CVEN 9405: Urban Transport Planning Practice 29 Derivation of Logit Model It is known that the CDF and PDF of Gumbel distribution is 𝑒𝑒 −𝑒𝑒 −𝑥𝑥 Assume that an individual 𝑛𝑛 has to select between 2 alternatives. According to RUM, individual 𝑛𝑛 will select alternative 1 only if: 𝑼𝑼𝒏𝒏𝟏𝟏 > 𝑼𝑼𝒏𝒏𝟐𝟐 => 𝑽𝑽𝒏𝒏𝟏𝟏 + 𝜺𝜺𝒏𝒏𝟏𝟏 > 𝑽𝑽𝒏𝒏𝟐𝟐 + 𝜺𝜺𝒏𝒏𝟐𝟐 ∞ −𝑥𝑥 respectively => 𝜺𝜺𝒏𝒏𝟐𝟐 < 𝑽𝑽𝒏𝒏𝟏𝟏 − 𝑽𝑽𝒏𝒏𝟐𝟐 + 𝜺𝜺𝒏𝒏𝟏𝟏 ∞ 𝑃𝑃𝑛𝑛 𝑗𝑗 = 1 = 𝑃𝑃𝑛𝑛 𝜀𝜀𝑛𝑛𝑛 < 𝑉𝑉𝑛𝑛𝑛 − 𝑉𝑉𝑛𝑛𝑛 + 𝜀𝜀𝑛𝑛𝑛 = � and 𝑒𝑒 −𝑥𝑥 . 𝑒𝑒 −𝑒𝑒 −∞ 𝑉𝑉𝑛𝑛𝑛 −𝑉𝑉𝑛𝑛𝑛 + 𝜀𝜀𝑛𝑛𝑛 � −∞ = � 𝐹𝐹𝑛𝑛 𝜀𝜀𝑛𝑛𝑛 < 𝑉𝑉𝑛𝑛𝑛 − 𝑉𝑉𝑛𝑛𝑛 + 𝜀𝜀𝑛𝑛𝑛 𝜀𝜀𝑛𝑛𝑛 ) . 𝑓𝑓 𝜀𝜀𝑛𝑛𝑛 . 𝑑𝑑𝜀𝜀𝑛𝑛𝑛 𝑓𝑓 𝜀𝜀𝑛𝑛𝑛 . 𝑑𝑑𝜀𝜀𝑛𝑛𝑛 . 𝑓𝑓 𝜀𝜀𝑛𝑛𝑛 . 𝑑𝑑𝜀𝜀𝑛𝑛𝑛 −∞ Where 𝑃𝑃𝑛𝑛 𝑗𝑗 = 1 Probability that individual 𝑛𝑛 selects alternative 1 𝐹𝐹𝑛𝑛 𝜀𝜀𝑛𝑛2 < 𝑉𝑉𝑛𝑛𝑛 − 𝑉𝑉𝑛𝑛𝑛 + 𝜀𝜀𝑛𝑛𝑛 𝜀𝜀𝑛𝑛𝑛 ) CDF of Gumbel distribution where 𝑥𝑥 = 𝑉𝑉𝑛𝑛𝑛 − 𝑉𝑉𝑛𝑛𝑛 + 𝜀𝜀𝑛𝑛𝑛 𝑓𝑓 𝜀𝜀𝑛𝑛𝑛 PDF of Gumbel distribution where 𝑥𝑥 = 𝜀𝜀𝑛𝑛𝑛 Continued… … CVEN 9405: Urban Transport Planning Practice 30 Derivation of Logit Model ∞ 𝑃𝑃𝑛𝑛 𝑗𝑗 = 1 = � 𝐹𝐹𝑛𝑛 𝜀𝜀𝑛𝑛𝑛 < 𝑉𝑉𝑛𝑛𝑛 − 𝑉𝑉𝑛𝑛𝑛 + 𝜀𝜀𝑛𝑛𝑛 𝜀𝜀𝑛𝑛𝑛 ) . 𝑓𝑓 𝜀𝜀𝑛𝑛𝑛 . 𝑑𝑑𝜀𝜀𝑛𝑛𝑛 ∞ −∞ = � exp(−𝑒𝑒 −(𝑉𝑉𝑛𝑛𝑛 − 𝑉𝑉𝑛𝑛𝑛+ 𝜀𝜀𝑛𝑛𝑛 ) ) . exp −𝜀𝜀𝑛𝑛𝑛 . exp(−𝑒𝑒 −𝜀𝜀𝑛𝑛𝑛 ). 𝑑𝑑𝜀𝜀𝑛𝑛𝑛 −∞ ∞ = � exp(−𝑒𝑒 −(𝑉𝑉𝑛𝑛𝑛 − 𝑉𝑉𝑛𝑛𝑛+ 𝜀𝜀𝑛𝑛𝑛 ) ) . exp −𝜀𝜀𝑛𝑛𝑛 . exp(−𝑒𝑒 −(𝑉𝑉𝑛𝑛𝑛 − 𝑉𝑉𝑛𝑛1+ 𝜀𝜀𝑛𝑛𝑛) ). 𝑑𝑑𝜀𝜀𝑛𝑛𝑛 −∞ ∞ = � exp(−𝑒𝑒 − −∞ ∞ 𝑉𝑉𝑛𝑛𝑛 − 𝑉𝑉𝑛𝑛𝑛 + 𝜀𝜀𝑛𝑛𝑛 2 + −𝑒𝑒 − = � exp(− � 𝑒𝑒 − 𝑉𝑉𝑛𝑛𝑛 − 𝑉𝑉𝑛𝑛𝑗𝑗 + 𝜀𝜀𝑛𝑛𝑛 = � exp(− � 𝑒𝑒 − 𝑉𝑉𝑛𝑛𝑛 − 𝑉𝑉𝑛𝑛𝑛𝑛 −∞ ∞ −∞ 𝑗𝑗=1 2 𝑗𝑗=1 𝑉𝑉𝑛𝑛𝑛 − 𝑉𝑉𝑛𝑛1 + 𝜀𝜀𝑛𝑛𝑛 ) . exp −𝜀𝜀𝑛𝑛𝑛 . 𝑑𝑑𝜀𝜀𝑛𝑛𝑛 ) . exp −𝜀𝜀𝑛𝑛𝑛 . 𝑑𝑑𝜀𝜀𝑛𝑛𝑛 . 𝑒𝑒 −𝜀𝜀𝑛𝑛𝑛 ) . exp −𝜀𝜀𝑛𝑛𝑛 . 𝑑𝑑𝜀𝜀𝑛𝑛𝑛 Integration by substitution, Let 𝑒𝑒 −𝜀𝜀𝑛𝑛𝑛 = 𝑡𝑡 CVEN 9405: Urban Transport Planning Practice => −𝑒𝑒 −𝜀𝜀𝑛𝑛𝑛 . 𝑑𝑑𝜀𝜀𝑛𝑛𝑛 = 𝑑𝑑𝑡𝑡 Continued… 31 Derivation of Logit Model 2 ∞ 𝑃𝑃𝑛𝑛 𝑗𝑗 = 1 = � exp(− � 𝑒𝑒 − 0 𝑃𝑃𝑛𝑛 𝑗𝑗 = 1 = 𝑗𝑗=1 𝑉𝑉𝑛𝑛𝑛 − 𝑉𝑉𝑛𝑛𝑛𝑛 exp(− ∑2𝑗𝑗=1 𝑒𝑒 − 𝑉𝑉𝑛𝑛𝑛 − 𝑉𝑉𝑛𝑛𝑛𝑛 𝑃𝑃𝑛𝑛 𝑗𝑗 = 1 = 0 + − ∑2𝑗𝑗=1 𝑒𝑒 − 1 𝑉𝑉𝑛𝑛𝑛 − 𝑉𝑉𝑛𝑛𝑛𝑛 1 + 𝑒𝑒 −(𝑉𝑉1 − 𝑉𝑉2) Similarly for MNL . 𝑡𝑡) . 𝑑𝑑𝑑𝑑 . 𝑡𝑡) ∞ 0 𝒆𝒆𝑽𝑽𝒏𝒏𝒏𝒏 𝑷𝑷𝒏𝒏 𝒋𝒋 = 𝟏𝟏 = 𝑽𝑽 𝒆𝒆 𝒏𝒏𝒏𝒏 + 𝒆𝒆𝑽𝑽𝒏𝒏𝟐𝟐 𝑷𝑷𝒏𝒏 𝒋𝒋 = 𝟏𝟏 = 𝒆𝒆𝑽𝑽𝒏𝒏𝒏𝒏 ∑𝑱𝑱𝒋𝒋=𝟏𝟏 𝒆𝒆𝑽𝑽𝒏𝒏𝒋𝒋 Source: http://jenni.uchicago.edu/econ312/Slides/topic2/probabilistic-choice_2006-03-29_all_tsa.pdf CVEN 9405: Urban Transport Planning Practice 32 Multinomial Logit (MNL) Model Mode split when travel times are equal Example 1: 2 modes Consider travellers need to choose between driving a private vehicle (auto) or catching a bus. The utilities of the two alternative models are represented relative to travel time. 𝑈𝑈𝑎𝑎𝑎𝑎𝑎𝑎𝑎𝑎 = 𝑉𝑉𝑎𝑎𝑎𝑎𝑎𝑎𝑎𝑎 + 𝜀𝜀𝑎𝑎𝑎𝑎𝑎𝑎𝑎𝑎 = 1 − 2𝑡𝑡𝑎𝑎𝑎𝑎𝑎𝑎𝑎𝑎 + 𝜀𝜀𝑎𝑎𝑎𝑎𝑎𝑎𝑎𝑎 Probability of choosing “auto” using the MNL model: 𝑒𝑒 𝑉𝑉𝑎𝑎𝑎𝑎𝑎𝑎𝑎𝑎 1 𝑃𝑃 𝑎𝑎𝑎𝑎𝑎𝑎𝑎𝑎 = 𝑉𝑉 = 𝑒𝑒 𝑎𝑎𝑎𝑎𝑎𝑎𝑎𝑎 + 𝑒𝑒 𝑉𝑉𝑏𝑏𝑏𝑏𝑏𝑏 1 + 𝑒𝑒 𝑉𝑉𝑏𝑏𝑏𝑏𝑏𝑏−𝑉𝑉𝑎𝑎𝑎𝑎𝑎𝑎𝑎𝑎 1 1 = = 1 + 𝑒𝑒 −2𝑡𝑡𝑏𝑏𝑏𝑏𝑏𝑏−1+2𝑡𝑡𝑎𝑎𝑎𝑎𝑎𝑎𝑎𝑎 1 + 𝑒𝑒 −1+2(𝑡𝑡𝑎𝑎𝑎𝑎𝑎𝑎𝑎𝑎−𝑡𝑡𝑏𝑏𝑏𝑏𝑏𝑏) CVEN 9405: Urban Transport Planning Practice 1 0.8 P(auto) 𝑈𝑈𝑏𝑏𝑏𝑏𝑏𝑏 = 𝑉𝑉𝑏𝑏𝑏𝑏𝑏𝑏 + 𝜀𝜀𝑏𝑏𝑏𝑏𝑏𝑏 = −3𝑡𝑡𝑏𝑏𝑏𝑏𝑏𝑏 + 𝜀𝜀𝑏𝑏𝑏𝑏𝑏𝑏 1.2 0.6 0.4 0.2 -10 0 -5 0 5 Difference in travel time (t_auto - t_bus) 33 10 Multinomial Logit (MNL) Model • • Equivalent Differences Property – Consider 2 options A and B which have utilities 𝑈𝑈𝐴𝐴 and 𝑈𝑈𝐵𝐵 respectively. Now assume that both utilities increase by ∆𝑈𝑈 𝑒𝑒 𝑈𝑈𝐴𝐴 +∆𝑈𝑈 𝑒𝑒 𝑈𝑈𝐴𝐴 𝑒𝑒 ∆𝑈𝑈 𝑒𝑒 𝑈𝑈𝐴𝐴 = 𝑈𝑈 = 𝑃𝑃 𝐴𝐴 = 𝑈𝑈 +∆𝑈𝑈 𝑒𝑒 𝐴𝐴 + 𝑒𝑒 𝑈𝑈𝐵𝐵 +∆𝑈𝑈 𝑒𝑒 𝐴𝐴 + 𝑒𝑒 𝑈𝑈𝐵𝐵 𝑒𝑒 ∆𝑈𝑈 𝑒𝑒 𝑈𝑈𝐴𝐴 + 𝑒𝑒 𝑈𝑈𝐵𝐵 Example of Equivalent Differences Property: – Consider utilities of -0.5, -1.5, and -3.0 for auto, rail and bus respectively. Then assume all utilities increase by 1. 𝑃𝑃 𝑎𝑎𝑎𝑎𝑎𝑎𝑎𝑎 = 𝑃𝑃 𝑟𝑟𝑟𝑟𝑟𝑟𝑟𝑟 = 𝑃𝑃 𝑏𝑏𝑏𝑏𝑏𝑏 = 𝑒𝑒 −0.5 𝑒𝑒 −0.5 +𝑒𝑒 −1.5 +𝑒𝑒 −3.0 𝑒𝑒 −1.5 𝑒𝑒 −0.5 +𝑒𝑒 −1.5 +𝑒𝑒 −3.0 𝑒𝑒 −1.5 𝑒𝑒 −0.5 +𝑒𝑒 −1.5 +𝑒𝑒 −3.0 Base Utilities = 0.690 = 0.254 = 0.057 CVEN 9405: Urban Transport Planning Practice 𝑃𝑃 𝑎𝑎𝑎𝑎𝑎𝑎𝑎𝑎 = 𝑃𝑃 𝑟𝑟𝑟𝑟𝑟𝑟𝑟𝑟 = 𝑃𝑃 𝑏𝑏𝑏𝑏𝑏𝑏 = 𝑒𝑒 0.5 𝑒𝑒 0.5 +𝑒𝑒 −0.5 +𝑒𝑒 −2.0 𝑒𝑒 −0.5 𝑒𝑒 0.5 +𝑒𝑒 −0.5 +𝑒𝑒 −2.0 𝑒𝑒 −2.0 𝑒𝑒 0.5 +𝑒𝑒 −0.5 +𝑒𝑒 −2.0 = 0.254 = 0.057 Increased Utilities 34 = 0.690 Estimation of Logit Models Objective: Estimate the parameters of logit model using a given dataset. This is done using Maximum Likelihood Estimation or Entropy Maximisation. Maximum Likelihood Estimation (MLE) • Finding the set of parameter values which maximise the probability of observing the given data points. • Likelihood function: Joint probability of all observed choices made by individuals in a dataset. Generally taken as the product of probabilities of the chosen alternatives (𝑌𝑌𝑛𝑛 ) across individuals 𝑵𝑵 𝑳𝑳 = � 𝑷𝑷𝒏𝒏 (𝒋𝒋 = 𝒀𝒀𝒏𝒏 ) 𝒏𝒏=𝟏𝟏 • 𝑵𝑵 𝒍𝒍(𝜶𝜶, 𝜷𝜷, 𝜸𝜸) = 𝒍𝒍𝒍𝒍 𝑳𝑳 = � 𝒍𝒍𝒍𝒍 𝑷𝑷𝒏𝒏 (𝒋𝒋 = 𝒀𝒀𝒏𝒏 ) 𝒏𝒏=𝟏𝟏 The log-likelihood function (𝑙𝑙) is maximised by changing the parameter values (𝛼𝛼, 𝛽𝛽, 𝛾𝛾) using a given dataset CVEN 9405: Urban Transport Planning Practice 35 MLE Example • • • Suppose a logit model of binary choice between car and bus is to be estimated. For simplicity, the observed utility for the two modes is defined as follows: 𝑽𝑽𝑎𝑎𝑎𝑎𝑎𝑎𝑎𝑎 = 𝛽𝛽 × 𝑇𝑇𝑇𝑇𝑎𝑎𝑎𝑎𝑎𝑎𝑎𝑎 𝑽𝑽𝑏𝑏𝑏𝑏𝑠𝑠 = 𝛽𝛽 × 𝑇𝑇𝑇𝑇𝑇𝑇𝑇𝑇𝑇𝑇 Suppose that the given dataset consists of observations of mode choice of only three individuals. The modal travel times and observed choice for each of the three individuals in the sample is as follows: Individual Auto Travel Time (mins) Bus Travel time (mins) Chosen Mode 1 30 50 Auto 2 20 10 Auto 3 40 30 Bus CVEN 9405: Urban Transport Planning Practice 36 MLE Example The probabilities for the observed mode for each individual are: Individual Auto Travel Time (mins) Bus Travel time (mins) 1 30 50 2 20 10 3 40 30 Probability 𝑃𝑃 𝐴𝐴𝐴𝐴𝐴𝐴𝐴𝐴, 1 = 𝑃𝑃 𝐴𝐴𝐴𝐴𝐴𝐴𝐴𝐴, 2 = 𝑃𝑃 𝐵𝐵𝐵𝐵𝐵𝐵, 3 = 𝑒𝑒 30𝛽𝛽 𝑒𝑒 30𝛽𝛽 +𝑒𝑒 50𝛽𝛽 𝑒𝑒 20𝛽𝛽 𝑒𝑒 20𝛽𝛽 +𝑒𝑒 10𝛽𝛽 𝑒𝑒 30𝛽𝛽 𝑒𝑒 40𝛽𝛽 +𝑒𝑒 30𝛽𝛽 = = = 1 1+𝑒𝑒 20𝛽𝛽 1 1+𝑒𝑒 −10𝛽𝛽 1 1+𝑒𝑒 10𝛽𝛽 The log-likelihood expression is as follows: 𝑁𝑁 𝑙𝑙 = � 𝑙𝑙𝑙𝑙 𝑃𝑃𝑛𝑛 (𝑗𝑗 = 𝑌𝑌𝑛𝑛 ) = 𝑙𝑙𝑙𝑙 𝑃𝑃1 𝑗𝑗 = 𝐴𝐴𝐴𝐴𝐴𝐴𝐴𝐴 𝑛𝑛=1 + 𝑙𝑙𝑙𝑙 𝑃𝑃2 𝑗𝑗 = 𝐴𝐴𝐴𝐴𝐴𝐴𝐴𝐴 + 𝑙𝑙𝑙𝑙 𝑃𝑃3 𝑗𝑗 = 𝐵𝐵𝐵𝐵𝐵𝐵 The optimisation algorithms maximise the log-likelihood value by changing the values of the parameters. CVEN 9405: Urban Transport Planning Practice 37 MLE Example Same β identified for likelihood and log-likelihood 𝛽𝛽 = −0.076 Same procedure when having multiple parameters Likelihood CVEN 9405: Urban Transport Planning Practice 38 Logit Model: Sample Result 𝑽𝑽𝒏𝒏𝒏𝒏 = 𝟎𝟎 − 𝟎𝟎. 𝟏𝟏𝟏𝟏 ∗ 𝑿𝑿𝒏𝒏𝒏𝒏𝒏𝒏 − 𝟏𝟏. 𝟐𝟐 ∗ 𝑿𝑿𝒏𝒏𝒏𝒏𝒏𝒏 + … 𝑽𝑽𝒏𝒏𝒏𝒏 = 𝟎𝟎. 𝟑𝟑𝟑𝟑 − 𝟎𝟎. 𝟏𝟏𝟏𝟏 ∗ 𝑿𝑿𝒏𝒏𝒏𝒏𝒏𝒏 − 𝟏𝟏. 𝟐𝟐 ∗ 𝑿𝑿𝒏𝒏𝟐𝟐𝟐𝟐 + … 𝑽𝑽𝒏𝒏𝒏𝒏 = 𝟎𝟎. 𝟔𝟔𝟔𝟔 − 𝟎𝟎. 𝟏𝟏𝟏𝟏 ∗ 𝑿𝑿𝒏𝒏𝒏𝒏𝒏𝒏 − 𝟏𝟏. 𝟐𝟐 ∗ 𝑿𝑿𝒏𝒏𝟑𝟑𝟑𝟑 + … Goodness of fit summary Results obtained from a software called Biogeme. Biogeme Demo in the next lecture Estimated parameters CVEN 9405: Urban Transport Planning Practice 39 Logit Models: Goodness of Fit 1. Rho-squared – Gives the overall goodness of the entire model (similar to R-squared in linear regression) 𝝆𝝆𝟐𝟐 = 𝟏𝟏 − 𝑳𝑳𝑳𝑳𝒇𝒇𝒇𝒇𝒇𝒇𝒇𝒇𝒇𝒇 𝑳𝑳𝑳𝑳𝒊𝒊𝒊𝒊𝒊𝒊𝒊𝒊𝒊𝒊𝒊𝒊𝒊𝒊 – A value closer to 1 is a better model as it is significantly different relative to the no-information model – Sometimes 𝐿𝐿𝐿𝐿𝑐𝑐𝑐𝑐𝑐𝑐𝑐𝑐𝑐𝑐𝑐𝑐𝑐𝑐𝑐𝑐 is used instead of 𝐿𝐿𝐿𝐿𝑖𝑖𝑖𝑖𝑖𝑖𝑖𝑖𝑖𝑖𝑖𝑖𝑖𝑖 . Use 𝐿𝐿𝐿𝐿𝑖𝑖𝑖𝑖𝑖𝑖𝑖𝑖𝑖𝑖𝑖𝑖𝑖𝑖 in this course For the sample result: 𝜌𝜌2 = 1 − −37.936 = 0.136 −43.944 Interpretation: The model is able to explain 13.6 percent unobserved variation in the dataset CVEN 9405: Urban Transport Planning Practice 40 Logit Models: Goodness of Fit 2. Adjusted Rho-squared – Penalises rho-squared for the number of estimated parameters (similar to adjusted R-squared in linear regression) For the sample result: 𝒂𝒂𝒂𝒂𝒂𝒂. 𝝆𝝆𝟐𝟐 = 𝟏𝟏 − 𝑎𝑎𝑎𝑎𝑎𝑎. 𝜌𝜌2 = 1 − 𝑳𝑳𝑳𝑳𝒇𝒇𝒇𝒇𝒇𝒇𝒇𝒇𝒇𝒇 −𝑲𝑲 𝑳𝑳𝑳𝑳𝒊𝒊𝒊𝒊𝒊𝒊𝒊𝒊𝒊𝒊𝒊𝒊𝒊𝒊 K is the number of estimated parameters −37.936 − 6 = −0.0001 → 0 −43.944 Interpretation: The model is not able to explain any unobserved variation in the dataset. Poor model CVEN 9405: Urban Transport Planning Practice 41 Logit Models: Goodness of Fit 3. Likelihood Ratio Test For the sample result: 𝑳𝑳𝑳𝑳 = −𝟐𝟐 ∗ (𝑳𝑳𝑳𝑳𝒊𝒊𝒊𝒊𝒊𝒊𝒊𝒊𝒊𝒊𝒊𝒊𝒊𝒊 − 𝑳𝑳𝑳𝑳𝒇𝒇𝒇𝒇𝒇𝒇𝒇𝒇𝒇𝒇 ) 𝐿𝐿𝐿𝐿 = −2 ∗ (−43.944 + 37.936) = 12.016 CVEN 9405: Urban Transport Planning Practice 42 Criteria for Model Selection • 3 criteria generally used: – Behavioural interpretation of parameters (sign and significance) – Goodness of fit statistics – Model parsimony • No exact hierarchy of criteria • Judgment is required when selecting the best among multiple models depending on the situation – Model for future forecasts can have goodness of fit as the top priority – Model explaining choice behaviour can emphasise on behavioural interpretation CVEN 9405: Urban Transport Planning Practice 43 Willingness to Pay (WTP) Estimates • • Represents the marginal rate of substitution which keeps the utility the same if one of the attribute increases in magnitude Defined as the ratio of two parameter values obtained from logit model • Value of Time (Value of Travel Time Savings) = • VoT represents how much money are individuals willing to spend to reduce their travel time by 1 hour VoT has important policy implications • For the sample result: 𝑉𝑉𝑉𝑉𝑉𝑉 = 𝜷𝜷𝑻𝑻𝑻𝑻 𝜷𝜷𝑻𝑻𝑻𝑻 × 𝟔𝟔𝟔𝟔 $/𝒉𝒉𝒉𝒉𝒉𝒉𝒉𝒉 −0.155 × 60 = $7.32/ℎ𝑜𝑜𝑜𝑜𝑜𝑜 −1.27 CVEN 9405: Urban Transport Planning Practice 44 If TT in minutes and TC in $ Practice Problem CVEN 9405: Urban Transport Planning Practice 45 MNL Model Example 2: Currently only 6% of the 5000 workers at a new office park in Macquarie Park use the express bus service from a specific white collar neighbourhood. The local planning commission identifies the utility function for a mode 𝑚𝑚 to be a function of out-of-pocket cost (OPC) and total travel time (TT), with a form: 𝑼𝑼𝒎𝒎 = 𝒂𝒂𝒎𝒎 – (𝟎𝟎. 𝟒𝟒𝟒𝟒 × 𝑶𝑶𝑶𝑶𝑶𝑶𝒎𝒎) – (𝟎𝟎. 𝟐𝟐𝟐𝟐 × 𝑻𝑻𝑻𝑻𝒎𝒎) – 𝑎𝑎𝑎𝑎𝑎𝑎𝑎𝑎𝑎𝑎 = 0.73, 𝑂𝑂𝑂𝑂𝑂𝑂𝑏𝑏𝑏𝑏𝑏𝑏 = $0.75, 𝑇𝑇𝑇𝑇𝑎𝑎𝑎𝑎𝑎𝑎𝑎𝑎 = 10.5 min, 𝑇𝑇𝑇𝑇𝑏𝑏𝑏𝑏𝑏𝑏 = 18 min – All other values are 0 • • • Does the MNL model replicate the actual bus mode share? According to the MNL model, what would be the bus mode share if the bus fare was reduced to $0? What does 𝑎𝑎𝑚𝑚 capture? CVEN 9405: Urban Transport Planning Practice 46 MNL Model Example: Currently only 6% of the 5000 workers at a new office park in Macquarie Park use the express bus service from a specific white collar neighbourhood. The local planning commission identifies the utility function for a mode 𝑚𝑚 to be a function of out-of-pocket cost (OPC) and total travel time (TT), with a form: – – 𝑼𝑼𝒎𝒎 = 𝒂𝒂𝒂𝒂 – (𝟎𝟎. 𝟒𝟒𝟒𝟒 × 𝑶𝑶𝑶𝑶𝑶𝑶𝒎𝒎) – (𝟎𝟎. 𝟐𝟐𝟐𝟐 × 𝑻𝑻𝑻𝑻𝒎𝒎) 𝑎𝑎𝑎𝑎𝑎𝑎𝑎𝑎𝑎𝑎 = 0.73, 𝑂𝑂𝑂𝑂𝑂𝑂𝑏𝑏𝑏𝑏𝑏𝑏 = $0.75, 𝑇𝑇𝑇𝑇𝑎𝑎𝑎𝑎𝑎𝑎𝑎𝑎 = 10.5 min, 𝑇𝑇𝑇𝑇𝑏𝑏𝑏𝑏𝑏𝑏 = 18 min All other values are 0 • Does the MNL model replicate the actual bus mode share? Determine Utilities: 𝑼𝑼𝑎𝑎𝑎𝑎𝑎𝑎𝑎𝑎 = 0.73 – (0.47 ∗ 0) – (0.22 ∗ 10.5) = −1.58 𝑼𝑼𝑏𝑏𝑏𝑏𝑏𝑏 = 0.00 – (0.47 ∗ 0.75) – (0.22 ∗ 18.0) = −4.31 Determine Mode Share: 𝑃𝑃 𝑎𝑎𝑎𝑎𝑎𝑎𝑎𝑎 = 𝑃𝑃 𝑏𝑏𝑏𝑏𝑏𝑏 = 𝑒𝑒 𝑈𝑈𝑎𝑎𝑎𝑎𝑎𝑎𝑎𝑎 𝑒𝑒 𝑈𝑈𝑎𝑎𝑎𝑎𝑎𝑎𝑎𝑎 +𝑒𝑒 𝑈𝑈𝑏𝑏𝑏𝑏𝑏𝑏 𝑒𝑒 𝑈𝑈𝑏𝑏𝑏𝑏𝑏𝑏 𝑒𝑒 𝑈𝑈𝑎𝑎𝑎𝑎𝑎𝑎𝑎𝑎 +𝑒𝑒 𝑈𝑈𝑏𝑏𝑏𝑏𝑏𝑏 = = 𝑒𝑒 −1.58 𝑒𝑒 −1.58 +𝑒𝑒 −4.31 𝑒𝑒 −4.31 𝑒𝑒 −1.58 +𝑒𝑒 −4.31 Yes the MNL model does reflect the actual bus mode share. CVEN 9405: Urban Transport Planning Practice = 0.939 = 0.061 = 6.1% 47 MNL Model Example 2: Currently only 6% of the 5000 workers at a new office park in Macquarie Park use the express bus service from a specific white collar neighbourhood. The local planning commission identifies the utility function for a mode 𝑚𝑚 to be a function of out-of-pocket cost (OPC) and total travel time (TT), with a form: – – 𝑼𝑼𝒎𝒎 = 𝒂𝒂𝒂𝒂 – (𝟎𝟎. 𝟒𝟒𝟒𝟒 × 𝑶𝑶𝑶𝑶𝑶𝑶𝒎𝒎) – (𝟎𝟎. 𝟐𝟐𝟐𝟐 × 𝑻𝑻𝑻𝑻𝒎𝒎) 𝑎𝑎𝑎𝑎𝑎𝑎𝑎𝑎𝑎𝑎 = 0.73, 𝑂𝑂𝑂𝑂𝑂𝑂𝑏𝑏𝑏𝑏𝑏𝑏 = $0.75, 𝑇𝑇𝑇𝑇𝑎𝑎𝑎𝑎𝑎𝑎𝑎𝑎 = 10.5 min, 𝑇𝑇𝑇𝑇𝑏𝑏𝑏𝑏𝑏𝑏 = 18 min All other values are 0 • According to the MNL model, what would be the bus mode share if the bus fare was reduced to $0? Existing Bus Passengers: 𝑃𝑃 𝑏𝑏𝑏𝑏𝑏𝑏 × 𝐷𝐷𝐷𝐷𝐷𝐷𝐷𝐷𝐷𝐷𝐷𝐷 = 0.061 × 5000 = 305 Existing Bus Revenue: 𝑃𝑃𝑃𝑃𝑃𝑃𝑃𝑃𝑃𝑃𝑃𝑃𝑃𝑃𝑃𝑃𝑃𝑃𝑃𝑃 × 𝐹𝐹𝐹𝐹𝐹𝐹𝐹𝐹 = 305 × $0.75 = $228.75 Utilities (Fare = 0): 𝑼𝑼𝑎𝑎𝑎𝑎𝑎𝑎𝑎𝑎 = 0.73 – (0.47 ∗ 0) – (0.22 ∗ 10.5) = −1.58 𝑼𝑼𝑏𝑏𝑏𝑏𝑏𝑏 = 0.00 – 0.47 ∗ 0 – 0.22 ∗ 18.0 = −3.96 Determine Mode Share: 𝑃𝑃 𝑎𝑎𝑎𝑎𝑎𝑎𝑎𝑎 = 𝑃𝑃 𝑏𝑏𝑏𝑏𝑏𝑏 = 𝑒𝑒 𝑈𝑈𝑎𝑎𝑎𝑎𝑎𝑎𝑎𝑎 𝑒𝑒 𝑈𝑈𝑎𝑎𝑎𝑎𝑎𝑎𝑎𝑎 +𝑒𝑒 𝑈𝑈𝑏𝑏𝑏𝑏𝑏𝑏 𝑒𝑒 𝑈𝑈𝑏𝑏𝑏𝑏𝑏𝑏 𝑒𝑒 𝑈𝑈𝑎𝑎𝑎𝑎𝑎𝑎𝑎𝑎 +𝑒𝑒 𝑈𝑈𝑏𝑏𝑏𝑏𝑏𝑏 The mode share increases from 6.1% to 8.5% CVEN 9405: Urban Transport Planning Practice = = 𝑒𝑒 −1.58 𝑒𝑒 −1.58 +𝑒𝑒 −3.96 𝑒𝑒 −3.96 𝑒𝑒 −1.58 +𝑒𝑒 −3.96 = 0.915 = 0.085 = 8.5% 48 Multinomial Logit (MNL) Model Example 2: Currently only 6% of the 5000 workers at a new office park in Macquarie Park use the express bus service from a specific white collar neighbourhood. The local planning commission identifies the utility function for a mode 𝑚𝑚 to be a function of out-of-pocket cost (OPC) and total travel time (TT), with a form: – – 𝑼𝑼𝒎𝒎 = 𝒂𝒂𝒂𝒂 – (𝟎𝟎. 𝟒𝟒𝟒𝟒 × 𝑶𝑶𝑶𝑶𝑶𝑶𝒎𝒎) – (𝟎𝟎. 𝟐𝟐𝟐𝟐 × 𝑻𝑻𝑻𝑻𝒎𝒎) 𝑎𝑎𝑎𝑎𝑎𝑎𝑎𝑎𝑎𝑎 = 0.73, 𝑂𝑂𝑂𝑂𝑂𝑂𝑏𝑏𝑏𝑏𝑏𝑏 = $0.75, 𝑇𝑇𝑇𝑇𝑎𝑎𝑎𝑎𝑎𝑎𝑎𝑎 = 10.5 min, 𝑇𝑇𝑇𝑇𝑏𝑏𝑏𝑏𝑏𝑏 = 18 min All other values are 0 • What does 𝑎𝑎𝑚𝑚 capture? Captures the mode bias towards auto and away from public transport. Existing Bus Passengers: 𝑃𝑃 𝑏𝑏𝑏𝑏𝑏𝑏 × 𝐷𝐷𝐷𝐷𝐷𝐷𝐷𝐷𝐷𝐷𝐷𝐷 = 0.061 × 5000 = 305 Existing Bus Revenue: 𝑃𝑃𝑃𝑃𝑃𝑃𝑃𝑃𝑃𝑃𝑃𝑃𝑃𝑃𝑃𝑃𝑃𝑃𝑃𝑃 × 𝐹𝐹𝐹𝐹𝐹𝐹𝐹𝐹 = 305 × $0.75 = $228.75 Bus Passengers (Fare = 0): 𝑃𝑃 𝑏𝑏𝑏𝑏𝑏𝑏 × 𝐷𝐷𝐷𝐷𝐷𝐷𝐷𝐷𝐷𝐷𝐷𝐷 = 0.085 × 5000 = 425 Bus Revenue (Fare = 0): 𝑃𝑃𝑃𝑃𝑃𝑃𝑃𝑃𝑃𝑃𝑃𝑃𝑃𝑃𝑃𝑃𝑃𝑃𝑃𝑃 × 𝐹𝐹𝐹𝐹𝐹𝐹𝐹𝐹 = 425 × $0.00 = $0 Why is the ridership still so low? The auto mode gains a massive modal bias advantage relative to the total utilities of both modes. The travel time of the bus is more than 1.5 times that of the auto mode. CVEN 9405: Urban Transport Planning Practice 49