Week 6 (ii) Lecture PDF
Document Details
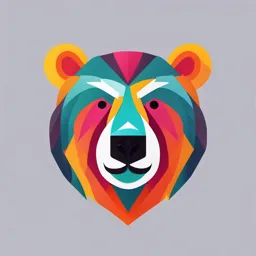
Uploaded by CleverNovaculite
Huddersfield Business School
Dr Artur Semeyutin
Tags
Summary
This is a lecture on violations of the CLRM assumptions in econometrics, covering essential reading from Chapter 5 of Brooks' textbook. The lecture discusses testing for and dealing with violations, including heteroscedasticity, autocorrelation, and functional form misspecification.
Full Transcript
Lecture 4: Violations of the CLRM Assumptions (Part II) Essential reading: Chapter 5 in Brooks. Dr Artur SemeyutinBIE0014 Econometrics Huddersfield Business School w/c 27/02/2023Dr Artur Semeyutin (BIE0014) VOA Business School1 / 36 Violation of the Assumptions of the CLRM Recall that we assumed of...
Lecture 4: Violations of the CLRM Assumptions (Part II) Essential reading: Chapter 5 in Brooks. Dr Artur SemeyutinBIE0014 Econometrics Huddersfield Business School w/c 27/02/2023Dr Artur Semeyutin (BIE0014) VOA Business School1 / 36 Violation of the Assumptions of the CLRM Recall that we assumed of the CLRM disturbance terms: 1 E (u t) = 0 2 var (u t) = σ2 < ∞ 3 cov (u i, u j) = 0 4 The X matrix is non-stochastic or fixed in repeated samples cov (u t, x t) = 0 5 u t ∼ N(0, σ2 ) Dr Artur Semeyutin (BIE0014) VOA Business School2 / 36 Investigating Violations of the Assumptions of the CLRM We will now study these assumptions further, and in particular look at: –How we test for violations –Causes –Consequences in general we could encounter any combination of 3 problems: –the coefficient estimates are wrong –the associated standard errors are wrong –the distribution that we assumed for the test statistics will be inappropriate –Solutions –the assumptions are no longer violated –we work around the problem so that we use alternative techniques which are still valid Dr Artur Semeyutin (BIE0014) VOA Business School3 / 36 Statistical Distributions for Diagnostic Tests Often, an F- and a χ2 - version of the test are available. The F-test version involves estimating a restricted and an unrestricted version of a test regression and comparing the RSS. The χ2 - version is sometimes called an “LM” test, and only has one degree of freedom parameter: the number of restrictions being tested, m . Asymptotically, the 2 tests are equivalent since the χ2 is a special case of the F-distribution: χ 2 (m ) m → F(m ,T −k) as (T −k) → ∞ For small samples, the F-version is preferable. Dr Artur Semeyutin (BIE0014) VOA Business School4 / 36 Assumption 1: E(u t) = 0 Assumption that the mean of the disturbances is zero. For all diagnostic tests, we cannot observe the disturbances and so perform the tests of the residuals. The mean of the residuals will always be zero provided that there is a constant term in the regression. Dr Artur Semeyutin (BIE0014) VOA Business School5 / 36 Assumption 2: var(u t) = σ2 < ∞ We have so far assumed that the variance of the errors is constant, σ2 - this is known as homoscedasticity. If the errors do not have a constant variance, we say that they are heteroscedastic e.g. say we estimate a regression and calculate the residuals, ˆ u t. Dr Artur Semeyutin (BIE0014) VOA Business School6 / 36 ût x2t + – Detection of Heteroscedasticity using White’s Test White’s general test for heteroscedasticity is one of the best approaches because it makes few assumptions about the form of the heteroscedasticity. The test is carried out as follows: 1 Assume that the regression we carried out is as follows yt = β 1 + β 2x 2t + β 3x 3t + u t And we want to test Var( u t) = σ2 . We estimate the model, obtaining the residuals, ˆ u t. 2 Then run the auxiliary regression ˆ u 2 t = α 1 + α 2x 2t + α 3x 3t + α 4x 2 2 t + α 5x 2 3 t + α 6x 2tx 3t + v t Dr Artur Semeyutin (BIE0014) VOA Business School7 / 36 Detection of Heteroscedasticity using White’s Test (Cont’d) 3 Obtain R2 from the auxiliary regression and multiply it by the number of observations, T. It can be shown that TR2 ∼ χ2 (m ) where mis the number of regressors in the auxiliary regression excluding the constant term. 4 If the χ2 test statistic from step 3 is greater than the corresponding value from the statistical table then reject the null hypothesis that the disturbances are homoscedastic. Dr Artur Semeyutin (BIE0014) VOA Business School8 / 36 Consequences of Using OLS in the Presence of Heteroscedasticity OLS estimation still gives unbiased coefficient estimates, but they are no longer BLUE. This implies that if we still use OLS in the presence of heteroscedasticity, our standard errors could be inappropriate and hence any inferences we make could be misleading. Whether the standard errors calculated using the usual formulae are too big or too small will depend upon the form of the heteroscedasticity. Dr Artur Semeyutin (BIE0014) VOA Business School9 / 36 How Do we Deal with Heteroscedasticity? If the form (i.e. the cause) of the heteroscedasticity is known, then we can use an estimation method which takes this into account (called generalised least squares, GLS). A simple illustration of GLS is as follows: Suppose that the error variance is related to another variable z t by var (u t) = σ2 z 2 t To remove the heteroscedasticity, divide the regression equation by z t y t z t = β 1 1 z t + β 2x 2t z t + β 3x 3t z t + v t where v t = u t z t is an error term. Now var(u t) = σ2 z 2 t , var( v t) = var ut z t =var( u t) z 2 t = σ 2 z 2 t z 2 t = σ2 for known z t. Dr Artur Semeyutin (BIE0014) VOA Business School10 / 36 Other Approaches to Dealing with Heteroscedasticity So the disturbances from the new regression equation will be homoscedastic. Other solutions include: 1 Transforming the variables into logs or reducing by some other measure of “size”. 2 Use White’s heteroscedasticity consistent standard error estimates. The effect of using White’s correction is that in general the standard errors for the slope coefficients are increased relative to the usual OLS standard errors. This makes us more “conservative” in hypothesis testing, so that we would need more evidence against the null hypothesis before we would reject it. Dr Artur Semeyutin (BIE0014) VOA Business School11 / 36 Background – The Concept of a Lagged Value t y ty t− 1 ∆ y t 2006 M09 0.8 − − 2006 M10 1.3 0.8 (1.3 −0.8) = 0.5 2006 M11 −0.9 1.3 ( −0.9 −1.3) = −2.2 2006 M12 0.2 −0.9 (0.2 − −0.9) = 1.1 2007 M01 −1.7 0.2 ( −1.7 −0.2) = −1.9 2007 M02 2.3 −1.7 (2.3 − −1.7) = 4.0 2007 M03 0.1 2.3 (0.1 −2.3) = −2.2 2007 M04 0.0 0.1 (0.0 −0.1) = −0.1 . . . . . . . . . . . . Dr Artur Semeyutin (BIE0014) VOA Business School12 / 36 Autocorrelation We assumed of the CLRM’s errors that Cov ( u i, u j) = 0 for i̸ = j, This is essentially the same as saying there is no pattern in the errors. Obviously we never have the actual u’s, so we use their sample counterpart, the residuals (the ˆ u t’s). If there are patterns in the residuals from a model, we say that they are autocorrelated. Some stereotypical patterns we may find in the residuals are given on the next 3 slides. Dr Artur Semeyutin (BIE0014) VOA Business School13 / 36 Positive Autocorrelation Positive Autocorrelation is indicated by a cyclical residual plot over time. Dr Artur Semeyutin (BIE0014) VOA Business School14 / 36ût ût–1 + – + – ût + – time Negative Autocorrelation Negative autocorrelation is indicated by an alternating pattern where the residuals cross the time axis more frequently than if they were distributed randomly Dr Artur Semeyutin (BIE0014) VOA Business School15 / 36ût ût–1 + – + – ût + – time No pattern in residuals – No autocorrelation No pattern in residuals at all: this is what we would like to see Dr Artur Semeyutin (BIE0014) VOA Business School16 / 36ût ût–1 + – + – ût + – time Detection of Autocorrelation: The Breusch-Godfrey Test It is a more general test for rth order autocorrelation: u t = ρ 1u t− 1 + ρ 2u t− 2 + ρ 3u t− 3 + · · · +ρ ru t− r + v t, v t ∼ N