IS4242 Intelligent Systems & Techniques L9 - Branding PDF
Document Details
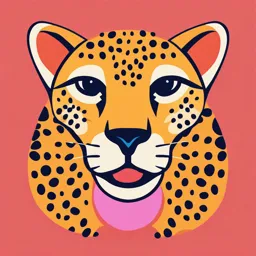
Uploaded by DexterousFern6890
National University of Singapore
NUS
Aditya Karanam
Tags
Summary
This document provides lecture notes for a course on intelligent systems and techniques, focusing specifically on branding. It explains concepts like brand culture, brand equity and strategies. The document covers visual analytics and convolution neural networks.
Full Transcript
IS4242 INTELLIGENT SYSTEMS & TECHNIQUES L9 – Branding Aditya Karanam © Copyright National University of Singapore. All Rights Reserved. ...
IS4242 INTELLIGENT SYSTEMS & TECHNIQUES L9 – Branding Aditya Karanam © Copyright National University of Singapore. All Rights Reserved. Announcements ▸ Quiz-1: Grades will be released by Friday ‣ Email: Simian (or me) regarding the grade if you find any discrepancies ▸ Midterm Feedback IS4242 (Aditya Karanam) 2 In this Class… ▸ What exactly is Brand? ▸ What is the Value of Brand to the Firm? ▸ How to derive Competitive Advantage through Branding? ▸ How to Create and Manage a Brand? ▸ Measuring Brand Image among consumers ‣ Convolution Neural Networks: Convolution and Pooling ‣ Hyperparameter Tuning IS4242 (Aditya Karanam) 3 What is brand? Traditional View ▸ The term “branding” comes from the practice of branding cattle ‣ Ranchers etched an identifying mark on animals to claim ownership ▸ Similarly, in the early days of market development, firms used brand marks to differentiate their products ‣ Some of these were merely the signature of the maker, while others were graphical representations and symbols ▸ Markers allowed consumers to differentiate between the products of one producer and another ‣ Identifying producers with whom they were familiar and trusted IS4242 (Aditya Karanam) 4 Brand: Culture of the Product ▸Contemporary view considers brand as the culture of the product. ‣Products are considered as cultural artifacts (borrowing the term from anthropology, sociology and history) ▸Products acquire meanings — connotations — as they circulate in society ▸Overtime, these meanings become conventional, widely accepted as “truths” about the product ‣Thus, creating a culture of the product, which is referred to as its brand IS4242 (Aditya Karanam) 5 Brand: Culture of the Product ▸ Consider a new product that has just been introduced by a new company. ▸ The product has a name and a trademarked logo, and other unique design features—all aspects that we intuitively think of as “the brand” ▸ However, the brand does not yet exist! ‣ Names, logos and designs are the material markers of the brand ‣ The product does not yet have a history – these markers are “empty” or devoid of meaning IS4242 (Aditya Karanam) 6 Brand: Culture of the Product ▸ Now think of some famous brands. ▸ These brands also have markers: Name, logo, distinctive product design feature, or any other design element that is uniquely associated with the product. ▸ These markers have been filled with customer experiences, advertisements, films and sporting events that displayed the brand in a popular culture, etc. ▸ These ideas of the product accumulate over time and “fill up” the brand markers with meaning. ‣ A brand culture is formed. IS4242 (Aditya Karanam) 7 Brand as Identity Markers of Consumers ▸ Earlier, brands played an informational role, adding value beyond its functional utility by serving as a heuristic, easing the decision-making process ‣ Thus, consumers pay extra for Heinz ketchup because they have learned to trust the Heinz brand and the company that stands behind it. ▸ In today’s culture, brands are the means to express personalities, lifestyles, ideologies, and a variety of other social identities. ‣ Brands are used by consumers as identity markers – props to construct and maintain their identities. ‣ This has created loyal customers following the culture of the product and forming communities – brand communities IS4242 (Aditya Karanam) 8 Measuring Brand Value: At a Product Level ▸ Brand equity is a measure of the brand’s value to the firm ‣ Sum of the intangible assets and liabilities linked to a brand. ▸ At a micro-level (product level), brand equity is the differential response consumers have to products when they are branded ▸ Offer consumers two identical products, one unbranded and one branded ‣ Brand equity is the consumer’s price premium: how much more consumers are willing to pay for the branded product over the unbranded product ‣ Value of the brand is the added value the brand brings during the purchase IS4242 (Aditya Karanam) 9 Brand Equity: At a Firm level ▸ On a macro-level (firm level), brand equity is measured as additional profits obtained from the sale of branded products over the sale of the same products unbranded ‣ At a firm level, brand equity drives not only a revenue premium but also cost savings ▸ Products with strong brands have lower marketing costs as consumers are more receptive and responsive to the brand’s messages IS4242 (Aditya Karanam) 10 Brand Equity: Dynamic and Difficult to Measure ▸ Brands, as assets, can both increase and decrease in value over time. ‣ Negative news about your brand can decrease its asset value ▸ Calculating the asset value of a brand is quite difficult ‣ Difficult to separate out the intangible value associated with their products from the tangible value derived from functional attributes of the product ▸ In most countries, brands do not appear on financial statements as assets unless they are purchased from another firm IS4242 (Aditya Karanam) 11 Branding as Competitive Advantage ▸ Companies can leverage brand equity in several ways ▸ The simplest is to create extensions ‣ New versions of the product within the same product category or extending the brand into other product categories. ‣ Example: Dyson has a good brand in vacuum cleaners and extended further into hair dryers IS4242 (Aditya Karanam) 12 Branding as Competitive Advantage ▸ Firms can also leverage their brand through co-branding, franchising, etc. ‣ Co-branding example: Prada and Adidas ‣ Several franchises in apparel, food, etc. IS4242 (Aditya Karanam) 13 Branding: Creation of Brand Culture ▸Brand cultures are created through stories from various “authors” ▸Companies: Shaped by all product-related activities of the firm that touch customers ‣ E.g.: All elements of the marketing mix: product, communication, pricing, etc. “tell stories” about the product. ▸Popular culture: Products are frequently used in media: films, television, books, etc. These representations can have a powerful influence on brands. ‣ E.g.: Ian Fleming - “Bond had been offered the Aston Martin (DB III) or a Jaguar 3.4. Either of the cars would have suited his cover – a well-to-do, adventurous young man with a taste for the good, fast things in life. But DB III had the advantage of an inconspicuous colour – battleship grey – and certain extras which might or might not come in handy.” ‣ Describes the image of Jaguar and Aston martin IS4242 (Aditya Karanam) 14 Branding: Creation of Brand Culture ▸Customers: Create consumption stories involving the product, which they often share with friends ‣Example: People share photos of the food they consume, pictures of themselves with brands, share their experience with the firm on social media, and so on ▸Influencers: In many categories, noncustomers’ opinions are influential. ‣Example: Product reviews on YouTube, Influencer Posts on TikTok, Instagram, etc. ▸Stories created by all of these “authors” interact in complex ways IS4242 (Aditya Karanam) 15 Branding: Creation of Brand Culture ▸ Customers watch ads and listen to influencers as they use the product. ▸ The media monitor how customers use the product and consider this in how they represent the product ▸ Quantity and complexity of these interactions makes it difficult to isolate the influence of each author The Firm Popular Culture Brand Stories Brand Culture Brand Shared, taken-for-granted brand stories, Brand Stories images, and associations Stories Brand Stories Influencers Customers IS4242 (Aditya Karanam) 16 How do you Manage Brand? ▸ Know your brand: The first step is always to understand your brand’s image in the minds of consumers ▸ Analyze what consumers have been hearing and interpreting about your brand (your received brand image) ‣ NOT what you have been saying in your brand communications (your intended brand identity) ▸ Understanding how your brand is currently perceived by consumers offers you insight into how to craft your brand messages going forward IS4242 (Aditya Karanam) 17 How do you Manage Brand? ▸ Brand management is image or personality management » ▸ Radically changing the meaning of a brand when consumers are using that meaning in their lives can be detrimental ‣ Ex: Twitter (X): Changes in name and logo ‣ Bird icon was friendly and welcoming, unclear about the meaning of ‘X’ – modern and edgy? ‣ This led to massive spike in Twitter Lite installs in the first week of the rebranding. IS4242 (Aditya Karanam) 18 How do you Manage Brand? ▸ Manage your brand’s authors: Meaning is authored by many people other than the brand’s managers ‣ Authors include consumers, media, influencers, etc. ▸ As a result, managers need to consider who the prominent authors of their brands are ▸ Work to positively influence those authors to compose and deliver desired brand messages IS4242 (Aditya Karanam) 19 Image-Based Brand Image: Measuring Brand from Images Technique: Convolution Neural Networks © Copyright National University of Singapore. All Rights Reserved. 20 How to Know Brand Image? ▸ Simply conduct survey of the customers ▸ More accurate: Listen to consumer experiences through social media such as Instagram, Twitter, etc. ▸ Consumers share photos and often tag brands in their posts ‣ Consumers communicate about brands with each other using these images ‣ For example, a search of #nike on Instagram returns over 92 million photos tagged with the brand name Nike (in 2023). IS4242 (Aditya Karanam) 21 Consumer Experiences: Example ▸ In the images posted on social media, consumers often link brands with usage context, feelings, and consumption experiences. ▸ Understand the brand image by calculating average consumption experiences in the consumer images IS4242 (Aditya Karanam) 22 Data Mining Task 1. Identify the consumption experience of apparel and beverage brands ‣ Classes: Glamor, Fun, Healthy, and Rugged ‣ Supervised learning problem ‣ Consider the task as image classification: 4 Class Classification problem 2. Calculate the average experience of all the consumers in a given brand ▸ How to obtain the data? ‣ Data with class labels for training the model ‣ Consumer data related to the brands IS4242 (Aditya Karanam) 23 Data ▸ Data for model building: ‣ Obtain tags from mTurk Annotators ‣ Build an image classifier to predict the experience ▸ Data on apparel and beverage brands: ‣ Identify brand images using the brand hashtags in the post on Instagram ‣ Brands: Adidas, Nike, Gucci, Levi's, Coca-Cola, Pepsi, Fanta, etc. IS4242 (Aditya Karanam) 24 Image Classification ▸ Can you identify the consumer experiences in these images? ▸ The human visual system is incredibly good: the hallmark of intelligence ▸ It is extremely difficult to make a rule-based computational system that recognizes experiences in the images. IS4242 (Aditya Karanam) 25 Image Classification: ML Approach ▸ Use a large number of labeled images to train a classifier Fun Rugged IS4242 (Aditya Karanam) Glamor Healthy 26 Image Representation ▸ Each image is a matrix of pixel values. ▸ Greyscale image: single value per pixel, 0.0 represents black, 255 represents white, and intermediate values have darkening shades of grey. ‣ E.g.: MNIST data: 28 x 28 pixel images ▸ RGB image: Each pixel is represented by three integers ranging from 0 to 255, reflecting the contribution of each of the three (red, green, blue) color channels ‣ Our dataset: 227 pixels wide and 227 pixels tall in each dimension: 227 x 227 x 3 ▸ Represent each image by a single dimensional vector of RGB values, i.e., a vector of 154,587 (=227 X 227 x 3) numbers. IS4242 (Aditya Karanam) 27 Neural Networks ▸ Example: Multi-label classifier Fun 154,587 vector Rugged Healthy Glamour IS4242 (Aditya Karanam) 28 Deep Neural Networks In the recent decade 1980s (deep: number of hidden layers) ▸ Increasing number of hidden layers ⇒ Increasing number of weights to be learnt ⇒ Optimization (Training) becomes harder IS4242 (Aditya Karanam) 29 Recap: Feed Forward Neural Network ▸ Neural Network Architecture ‣ Different layers of neurons ‣ Different types of activation functions ▸ Loss Function: Determined by the task ▸ Training: Backpropagation using gradient descent ‣ Learning rate and initialization matter ▸ CNN: Major change is in the architecture, especially feature mappings IS4242 (Aditya Karanam) 30 Convolution Neural Networks (CNN) ▸ Brief overview with intuition on the salient aspects of CNN ▸ Similar to Neural Networks but utilizes spatial correlations in grid-like data (e.g. images) ▸ Reduces the number of weights through convolution and pooling operations and parameter sharing (tied weights) IS4242 (Aditya Karanam) 31 Convolutional Neural Networks History: Alexnet ▸ Alexnet: CNN designed by Krizhevsky, Sutskever and Hinton., won the ImageNet Large Scale Visual Recognition Challenge, 2012. ‣ Marks the rise of deep neural networks IS4242 (Aditya Karanam) 32 Image Net Challenge ▸ Other architectures have improved performance further. IS4242 (Aditya Karanam) 33 Convolutional Neural Networks ▸ CNN can be considered to have two components: Feature Mappings and Classifier layers ▸ Feature mappings: Convolution, Activation functions (ReLU (max(0, 𝑥))) and Pooling ▸ Classifier: Fully connected layers (neurons) with some activation function IS4242 (Aditya Karanam) 34 Convolution ▸ A convolution operation uses a filter/kernel that ‘slides’ along the input volume and transforms it. ‣ The same weights are used for the entire input volume ▸ Stride: the step-size as the filter moves through the image (default value is 1) IS4242 (Aditya Karanam) 35 Convolution ▸ Padding: We may utilize pixels less on the perimeter of images compared to the one in the middle ▸ Padding the image with 0’s in the border ‣ Performed especially in the final layers IS4242 (Aditya Karanam) 36 Pooling ▸ Pooling transforms the input volume by resizing it using operations such as min, max, avg., etc. ‣ E.g., Max pooling takes the maximum of every block IS4242 (Aditya Karanam) 37 Convolutional Neural Networks ▸ Several convolutions are applied to the same image to extract different types of features ▸ Flatten the final layer of convolution ▸ Provide it as an input to the fully connected layer to obtain class IS4242 (Aditya Karanam) 38 Application: Consumer Experience ▸ 5 convolution layers and Max Pooling ‣ Activation function: ReLU ▸ Two fully connected layers for classification ▸ Efficiently implement using PyTorch library IS4242 (Aditya Karanam) 39 Application: Consumer Experience ▸ ConvNet with 5 layers and two fully connected layers Feature mapping Classifier IS4242 (Aditya Karanam) Included to highlight the architecture exact dimensions are in the code (prev. slide) 40 Calculating Image-Based Brand Image ▸ Obtain average probability of each class across all the images of a brand shared by users and sponsors ▸ Compare the probabilities to understand the brand image among consumers and the brand image that the firm wishes to portray Users’ posts Sponsored posts IS4242 (Aditya Karanam) 41 Hyper-Parameter Tuning ▸ Identify the optimal values of the parameter for the model ▸ 1. Create 3 splits of your data: Train(70%), Validation(10%) & Test Sets (20%) IS4242 (Aditya Karanam) 42 Hyper-Parameter Tuning ▸ 2. Use different hyperparameter values and train different models using Training Data IS4242 (Aditya Karanam) 43 Hyper-Parameter Tuning ▸ 3. Evaluate performance of each model on Validation Set and choose the best hyper parameters ▸ One validation set may not be representative of the data ‣ Combine training and validation data, and partition them into ‘k’ folds IS4242 (Aditya Karanam) 44 Hyper-Parameter Tuning ▸ K-Fold Cross Validation ▸ Divide the entire data into K folds ▸ In each fold, train on the training set and evaluate on the validation set ▸ Average the performance over all the folds Estimate of the “true” test error IS4242 (Aditya Karanam) 45 Hyper-Parameter Tuning ▸ Using the best Hyperparameter values, train the model on Training + Validation Set to learn a new model IS4242 (Aditya Karanam) 46 Hyper-Parameter Tuning ▸ Grid SearchCV ‣ Evaluates every possible combination of hyper-parameter values along with cross validation ▸ Randomized search CV ‣ Random samples of hyperparameter values are evaluated ‣ Optimizes for time ▸ Existing packages (sklearn) perform these efficiently IS4242 (Aditya Karanam) 47 References ▸ Churn management: ‣ https://hbsp.harvard.edu/product/509041-PDF-ENG ‣ Liu et al. 2022, Visual Listening In: Extracting Brand Image Portrayed on Social Media, Marketing Science ▸ Neural Networks: ‣ http://cs231n.github.io ‣ http://neuralnetworksanddeeplearning.com/index.html ‣ http://www.deeplearningbook.org ▸ Hyper-Parameter Tuning: ‣ https://sebastianraschka.com/blog/2016/model-evaluation-selection- part1.html IS4242 (Aditya Karanam) 48 Thank You © Copyright National University of Singapore. All Rights Reserved.