Applied Microeconometric Methods - Randomization - PDF
Document Details
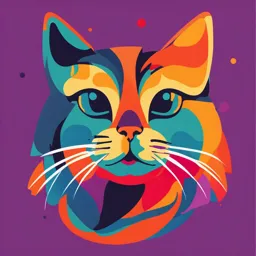
Uploaded by MerryCentaur
Utrecht University
Jacopo Mazza
Tags
Summary
These slides provide an overview of randomization in applied microeconometrics, focusing on Randomized Controlled Trials (RCTs), their limitations, and alternative designs, such as lotteries of overbooked programs, phase-in designs, and encouragement designs. The slides also discuss ethical concerns, external and internal validity, and potential violations like incomplete randomization.
Full Transcript
ECB3AMT Applied Microeconometric Methods 2. Randomization Dr. Jacopo Mazza Randomization 1. Randomized Controlled Trials (RCT) 2. Limitations of RCTs 3. Alternative designs Topic 2 Randomization 2 Randomization solves the evaluation problem Randomization is the Gold-Standard...
ECB3AMT Applied Microeconometric Methods 2. Randomization Dr. Jacopo Mazza Randomization 1. Randomized Controlled Trials (RCT) 2. Limitations of RCTs 3. Alternative designs Topic 2 Randomization 2 Randomization solves the evaluation problem Randomization is the Gold-Standard for solving the evaluation problem recall: the evaluation problem rests in the correlation of the remaining error term with the treatment effect If we randomly assign the treatment status, then and Topic 2 Randomization 3 Randomization solves the evaluation problem Ideally one draws two random samples of the population control group C and treatment group T Both samples are statistically indistinguishable from the population Note: mathematically, the expected value of a variable is just the mean of the variable,. Only the treatment group T is treated, the control group remains untreated Topic 2 Randomization 4 Randomization solves the evaluation problem We randomly assign the treatment status , so that This implies that there is no self-selection into the treatment If the treatment status is random, we receive the causal effect by comparing the means Randomization does not eliminate individual heterogeneity. But it ensures that on average the two groups are identical. Topic 2 Randomization 5 Randomization bias Randomization bias occurs if the selection into the experiment ( into treatment) was not random Presumption: those who are more likely to profit from the treatment are more likely to sign in for the experiment Consequence: program effects are not representative for the population General problem in social experiments Often it is impossible to hide the aim of the experiment self- selection into the experiment Comparison to agricultural experiments: “plots of ground do not respond to anticipated treatments of fertilizer, nor can they excuse themselves from being treated.” (Heckman, 1992) Topic 2 Randomization 6 Illustration of treatment effects Δ Δ 𝐴𝑇𝐶Δ+¿ ¿ Δ 𝐴𝑇𝐸 Δ 𝐴𝑇𝑇 Selection into the experiment Topic 2 Randomization 7 Treatment effects (selection into experiment) Average Treatment Effect (ATE) Population of the experiment is a random sample of the population The effect is representative for a randomly drawn unit of the population Average Treatment Effect on the Treated (ATT) The population of the experiment has self-selected into the experiment Note: this is often the policy relevant parameter! Average Treatment Effect on the Untreated (ATC) The population of the experiment has not selected into The population of the experiment has not self-selected into the experiment. Topic 2 Randomization 8 Treatment effects (compliance) Intention-to-treat Effect (ITT) Effect on those who were selected into treatment, irrespective of whether they received the treatment If participation in treatment is voluntary, ATT and ITT can differ ITT is informative about the potential effect of the treatment in the tested population, but not so much about the strength of the effect Local Average Treatment Effect (LATE) Effect on the compliers (i.e. on those who were selected into treatment and who actually picked up the treatment) Represents the effect of the treatment on the population of those who are responsive to the treatment This is the ITT effect, corrected by non-participation Topic 2 Randomization 9 Next Topic 1. Randomized Controlled Trials (RCT) Example for an RCT 2. Limitations of RCTs 3. Alternative designs Topic 2 Randomization 10 The RAND Health Insurance Experiment (HIE) 1974-1982, 6 US states, 3,958 people aged 14-61 Random assignment to 14 insurance plans Participants paid no premium, but cost sharing depending on plan “Catastrophic”: pay 95% yourself, but cap on total costs depending on income “deductible”: pay 95% yourself, caps at 150€/individual or 450€/family “coinsurance”: pay 25%-50% yourself, capped “free”: free comprehensive healthcare Aim: estimate price elasticity for demand for healthcare does generous healthcare improve health? Problem: Small treatment groups (researchers typically aggregate) Topic 2 Randomization 11 Balance Checks Topic 2 Randomization 12 Health Outcomes Topic 2 Randomization 13 The Oregon Trail HIE Experiment: Focus on middle-class, insured people no uninsured people, i.e. no real control group – contrast to U.S. reality Does Medicaid (basic universal healthcare) improve health? Very different question! State of Oregon randomly offered Medicaid to ineligible people Lottery among applicants, not all winners actually received aid Uninsured U.S. citizens or legal immigrants aged 19-64 Topic 2 Lottery for fairness considerations, Randomization not initially intended as 14 Topic 2 Randomization 15 Topic 2 Randomization 16 Next Topic 1. Randomized Controlled Trials (RCT) Example for an RCT 2. Limitations of RCTs 3. Alternative designs Topic 2 Randomization 17 External and internal validity External validity Are the results generalizable to other situations and populations i.e. are the results informative for other situations than the experiment itself? Internal validity Are the results internally consistent, do they represent causal effects? RCTs are the gold-standard to construct counterfactual situations high internal validity i.e. does the analysis represent the causal effect of the treatment? We will discuss these questions also with the other methods. Topic 2 Randomization 18 Violations of external validity Non-representative sample Results can only be generalized if the sample population is representative for the population at question Often violated if participation in the experiment is voluntary (and one only randomizes among the volunteers) Non-representative treatment i.e. the treatment cannot be implemented in reality General equilibrium effects Scaling up the treatment may have general equilibrium effects (=> see SUTVA) If external validity is violated, the results cannot be generalized beyond the experiment itself. Topic 2 Randomization 19 Violations of internal validity Incomplete randomization through self-selection of participants into the treatment E.g. if one deviates from the experimental protocol Þ Make balance checks (i.e. compare covariates between groups)! Selection into treatment was random, but participation in the treatment was not (incomplete treatment) Partial compliance (some individuals did not participate in the treatment) Change treatment status through the individuals (non- compliance) Attrition Topic 2 Randomization 20 Violations of internal validity The experiment affects the behavior of the participants Hawthorne effect: change in behavior of the treatment group because individuals know that they are evaluated John-Henry effect: change in behavior of the control group because they know that they are evaluated Insufficient sample size results in imprecise estimates Violations of internal validity imply that the estimates effects cannot be interpreted as causal effects! Topic 2 Randomization 21 How to read an RCT Study What is the research question? What is the identification problem, why do we need an experiment? What is the design of the experiment (internal validity)? How do the authors ensure randomization (is randomization complete)? Is treatment complete? (compliance, attrition) Are there behavioral effects? Balance checks – i.e. comparing relevant covariates What type of treatment effect(s) are measured (ATE, ATT, ITT, LATE)? Topic 2 Randomization 22 Randomized controlled trials Experiments tell us whether something works, but not why! Randomized studies are not always feasible 1. Randomization impossible in theory 2. Too expensive 3. Ethical objections against randomization RCTs are on the rise in business and economics Social experiments in policy evaluation Lab experiments in behavioral economics Field experiments, e.g. in development economics Topic 2 Randomization 23 Fundamentally unidentified question The concept of experiments helps to design research questions Is an ideal experiment imaginable for answering a research question? If the answer is “no”, it is a FUQ (fundamentally unidentified question). Examples The effect of carbon dioxide on the world climate Effect of inborn personal characteristics, such as e.g. sex or ethnicity E.g. what is the effect of sex on income? Problem: we cannot randomize the sex of a person Topic 2 Randomization 24 Ethical concerns Can we ethically justify to withhold the treatment from the control group? Obvious in medicine or in animal experiments By now most universities have ethic committees who have to approve experiments in advance Objections are particularly valid if the positive effects of a treatment are already proven or generally accepted Potential ways out of ethical dilemmas: Lotteries of overbooked programs Phase-in designs Nudging / encouragement designs Topic 2 Randomization 25 Randomization 1. Randomized Controlled Trials (RCT) 2. Limitations of RCTs 3. Alternative designs Topic 2 Randomization 26 Alternative designs Alternatives if ethical concerns preclude classical RCTs 1. Lotteries of overbooked programs 2. Phase-in designs 3. Encouragement designs Topic 2 Randomization 27 1. Lotteries of overbooked programs Possible if there is insufficient capacity to treat all applicants to a program Often assignment into treatment of overbooked programs is random Fair way of assignment Prevents the use of improper selection criteria Often occurs in governmental pilot projects or funding projects of foundations Simple organization of assignment possible Randomize treatment status among those who apply for the program – enables to estimate ATT by comparing treatment and control group Topic 2 Randomization 28 2. Phase-in designs Simultaneously implementing a program in several regions or for several groups of individuals can be difficult Ideally, on randomizes the introduction of the treatment across regions, individuals, … Addresses fairness concerns: at some point, everybody will be treated Topic 2 Randomization 29 Drawbacks of phase-in designs 1. Prevents estimation of long-term effects At some point, everybody is treated 2. Treatment effect cannot be estimated when sequence of phases is too quick The treatment effect needs sufficient time to unfold 3. Future treatment group anticipates the treatment Change in behavior due to anticipation of the treatment can bias the results, e.g. if firms expect to receive investment subsidies they might postpone investment decisions Topic 2 Randomization 30 3. Encouragement designs Instead of randomly assigning individuals into treatment, randomly encourage them into treatment – individuals have free choice Goes back to Richard H. Thaler (nudging) – 2017 Nobel price in economics Nudging examples from the real world Pictures on packs of cigarettes Snacks close to checkout counters Etc. Nudging raises the probability of treatment Typically estimate the intention-to-treat (ITT) effect Identify ATE / LATE via instrumental variable (IV) estimation (later in the course!) Topic 2 Randomization 31 Encouragement designs Populatio n Randomization encourage Not encouraged d participati No on participation ITT: compare „encouraged“ to „not encouraged“ Comparing „participation“ vs. „no participation“ does not provide the causal effect of participation! How to get effect (ATE/LATE) of participation? => Instrumental Variable Method (next topic) Topic 2 Randomization 32 Randomization 1. Randomized Controlled Trials (RCT) 2. Limitations of RCTs 3. Alternative designs Next Topic: Instrumental Variables (IV) Topic 2 Randomization 33