MMSM Video Notes on Factor Analysis PDF
Document Details
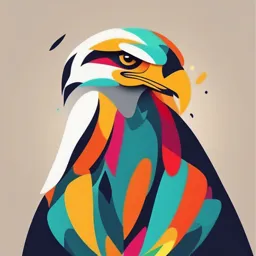
Uploaded by PoisedMeter7273
Radboud University
Vincent
Tags
Summary
These video notes cover factor analysis, a statistical method used to understand the interplay between multiple variables. The notes discuss how factor analysis simplifies complex data, finds hidden patterns, and focuses on the interrelationships between variables. The document covers measurement models, including both formative and reflective models.
Full Transcript
MMSM Video notes Week 37/39, factor analysis Factor analysis Purpose: estimate a model which explains variance/covariance between a set of observed variables (in a population) by a set of (fewer) unobserved factors and weighting Observed variables = the collected data You want to understand how u...
MMSM Video notes Week 37/39, factor analysis Factor analysis Purpose: estimate a model which explains variance/covariance between a set of observed variables (in a population) by a set of (fewer) unobserved factors and weighting Observed variables = the collected data You want to understand how unobserved factors play a role in this data set Easy explanation: simplify complex data, find hidden patterns and set the stage for deeper, more focused analysis. - Factor analysis is an interdependence technique: you are interested in how these different items interrelate with each other, you are not interested in predictions yet. - You want to define structure among variables - Interrelationships among large number of variables to identify underlying dimensions o The underlying dimensions are called factors - Factor analysis’ purpose is to summarize and reduce data Measurement model You have a construct, known as Xi ξ You have the underlying items called X11 X12 X13, they form the perception of the construct You are also interested in the measurement error, known as Epsilon or ε are there any systematic biases that influence how we measure these items. With factor analysis, you can asses this type of measurement error. Four constructs with different items. Multi item measurement Multi-item measurement uses several questions to measure a single concept to increase reliability and validity. This method provides a more accurate and consistent way to assess complex concepts in research and surveys. - Increases reliability and validity of measures - You can also allow you to asses measurements o Measurement error o Reliability o Validity - Two forms of measurement models o Formative (emerging) ▪ You have the items and they emerge towards a construct o Reflective (latent), typical ones in mkt and strtgy research and most used ▪ Latent means that there is a construct, and the items reflect this construct Reflective measurement models - Direction of causality is from construct to measure - Correlated indicators - Takes measurement error into account at the item level - Validity of items is usually tested with factor analysis You can see Xi, the construct, and we want the asses the factor loading by each item (X) which is denoted by lambda (λ) and we are also interested in the measurement error, Epsilon. Reliability and validity Or anything where you would like to asses higher order dimensions!!! The process of factor analysis 1. Formulate the problem What is it, what are we trying to do - The objectives of the analysis should be identified o Is it data summarization o Or is it data reduction - Which variables are we going to measure, this is based on past research, theory, judgement of the researcher - Measurement properties, should be ratio or interval - Sample size, how big does the sample need to be in order to conduct factor analysis (4-5 items per number of respondents per variable) Distinguish between exploratory factor analysis and confirmatory factor analysis Exploratory factor analysis - Is about the exploration of the data, finding an underlying structure of the data/ higher order dimensions - Assumptions that superior factors cause correlations between variables, but we do not yet know what these superior factors are - Reveal interrelationships will be revealed by factor analysis Confirmatory factor analysis - We have a priori ideas of underlying factors, derived from a theory - The relationships are assumed before conducting the factor analysis - Testing of the hypothesis 2. Construct the correlation matrix of the data we have conducted - Kaiser-Meyer Olkin (KMO) measure of sampling adequacy o It tells you if the sample adequately represents the population o Should be above.5, the closer to 1 the better - Barlett’s test of sphericity o Tests the null hypothesis that the variables are uncorrelated in the population o Must be significant, smaller than.05 3. Selecting the extraction method Very important step in factor analysis, usually we have two major types of extraction methods (extraction method: technique to identify the underlying factors): - Principal components analysis - Common factor analysis Principal component analysis - Looks at total variance in the data - Diagonal of the correlation matrix consist of unities o In the correlation matrix, you look at the diagonal, this is considered as a unity - Full variance is brought into the factor matrix - Primary concern: minimum number of factors that accounts for maximum explainable variance - The factors are called: principal components Principal components model - Mathematically, each variable is expressed as a linear combination of these components - Covariation among these variables is described in terms of a small number of principal components - If the variables are standardized, the principal component model may be represented as: o o - Example - - They all have a value of 1, initially and after extraction total variance has been considered - - When looking at the variance explained, the first factor has a high value of explaining variance, principal component model tries to maximize the variance Common factor analysis - Factors are estimated based only on the common variance, not the total variance - We don’t speak of unities, but on communalities who are inserted in the diagonal of the correlation matrix - Primary concern is to identify the underlying dimensions and their common variance - The extraction method is also known as: o Principal axis factoring - Mathematically, each variable is expressed as a linear combination of underlying factors - The covariation among the variables is described in terms of a small number of common factors plus a unique factor for each variable - If the variables are standardized, the factor model may be represented as o o o Example o o Initially they don’t have a value of one, and also after extraction they still don’t have a value of one. You can see there is a difference because this is about common and unique variance o o At the variance explained, you can see that the first factor is also a high proportion of explained variance, but it is not as high as the principal components model, even though it is the same date used. - - What can be seen, is that V1 and V3 have nice factor loadings with factor one - For factor 2, it can be seen that V2, V4 and V6 have nice and high significant factor loadings with factor 2 - Variable 5 has a significant loading on factor 1, but negative Important difference!!! The diagonal value of the correlation matrix can be distinguished into unity and communality In terms of variance, unity looks at the total variance (principal component analysis) In the communality we have a part of the common variance and the unique variance (common factor analysis) Total variance and common variance are extracted variances Unique variance is excluded variance 4. Determining the number of factors we have There are several methods to do this - A priori determination o You as a researcher have a very good idea of how many factors you might expect due to replication of existing studies, you a priori know of how many factors you expect. You can ask the programme how many factors you expect - Based on the eigenvalues (>1) o Should be above 1 - Based on the scree plot o You can have the eigenvalues plotted in a Scree Plot and it shows you the number of factors - Based on percentage of variance o Basically, once the factors explain more than 0.6 (60%) - Based on Split-Half-Reliability o Split the data set in two different sets and check the robustness of the number of factors. Based on this criteria you would chose the number of factors The most chosen method is: Eigenvalues above 1 Look at the eigenvalues above 1, so the first two components in this case Scree plot Use the cut-off point, in this case you would chose two factors Determine the number of factors: percentage of variance Above.60 would be a cut off, you would determine two factors 5. Rotating factors Rotating factors happens for interpretation reasons In rotating factors: each factor should have nonzero, or significant, loadings or coefficients for only some of the variables. You want to achieve that each factor has some variables that load on it, but not all variables, you cannot reduce or summarize data. At the same time, we would like each variable to have nonzero or significant loadings with only a few factors, if possible, with only one. The best outcome is that each variable loads significantly on one factor and has a zero loading on the other factors. This is because we want to achieve convergent and discriminant validity. Convergent validity: each the variable that would be expected to load significantly on the factor, loads significantly on the factor. Discriminant validity: At the same time this variable should not have any significant loading on all the other factors. It should discriminate the other factors. Example: An example of an unrotated variable matrix with factor plot Imagine that each factor that has been identifies is an axis What we do then is kind of rotate the axe, factors become more easily interpretable Factor rotation distinguishes between - Orthogonal rotation o When the axes are maintained at right angles (90 degrees) o Typical: Varimax o Used when it assumes that factors are not correlated - Oblique rotation o If the axes are not maintained at right angles o Typical: Oblimin o Used when it assumes that factors to be correlated Decisions should be based on theoretical considerations!!! If the factors correlate with each other, it doesn’t mean that you have a clean measurement Most of the time in our type of thesis research, we would use orthogonal rotation The fit after rotation can be increased 6. Interpreting factors When this happens, check if v5 was a reverse coded item and reverse code it Factor interpretation - A factor can be interpreted in terms of the variables that load high on it - If plotted, variables at the end of an axis are those that have high loadings on only that factor, and hence describe the factor. 7. Using factors in other analysis - Factor scores o Composite measures of each factor for each respondent o Based on factor loadings of all variables o Disadvantage, usually are not easily replicated across studies o Program can compute and save them in the data sheet - Surrogate variables o Examining the factor matrix -> select for each factor the variable with the highest loading -> use as surrogate (surrogate: iemand of iets dat iemand of iets anders vervangt of in plaats daarvan wordt gebruikt; een substituut voor een ander) o You select the variable that represents the best the factor o If you have similar loadings, use theoretical or other measurement considerations - Summated scores o Variables loading high on one factor are summed up or averaged. This sum score is used instead of the factor score o Advantages: ▪ It represents the multiple aspects in one variable ▪ Easier to use for prediction oriented research ▪ Reducing measurement error Take a good look at the advantages and disadvantages!! 8. Determine the model fit Determine the model fit via residuals - 1. Reproduce the correlations between variables from estimated correlations between the variables and the factors - 2. Compare differences between observed correlations (as given in the input correlation matrix) and reproduce correlations (as estimated from the factor matrix) o So basically, you are looking at what are my real observations and what are the predictions of my model and compare the differences o If the differences are small, you have a good model fit o The differences are called residuals, the smaller the residuals the better. - 3. Reliability analysis, sum scores consists of useful variance and error variance o If the error is purely random error, one can use reliability coefficients such as Chronebach’s alpha to determine the amount of useful variance in the scores Reliability analysis: Chronebach’s alpha - Usually, a value of 0,7 is accepted - In line with scientific progress higher thresholds should be used - Assessment of reliability is an iterative process In SPSS: Analyze->Data Reduction>Factor analysis Typical exam question: Week 40/42, ANOVA, ANCOVA, and MANOVA ANOVA: ANalysis Of VAriance Statistically method used to compare means of two or more groups Example: - Interest: reactions of call center customers to waiting time - Experiment: randomly group respondents into 3 groups: o Below average waiting time o Average waiting time o Above average waiting time - > survey: about their service perceptions and purchase intentions - Collect data: Waiting time is called factor, grouping variable or treatment - 1 corresponds to below waiting time - 2 corresponds to average waiting time - 3 corresponds to above waiting time Here you need ANOVA ANOVA defined: - To compare mean scores of two or more populations - The null hypothesis, typically, is that all population means are equal o This means that you will not find differences between the populations between the different groups o If you are interested in finding differences you want to reject H0 - To test for statistically significant differences between these populations Important measurement requirements - At least one IV needs to be categorical - Each possible outcome is called level or category - DV must be metrically scaled - Likert scale can be perceived as a metrical scale Types of ANOVA: One-way ANOVA: one factor with at least two levels, levels are independent N-Way ANOVA: two or more factors with at least two levels; levels are independent - We speak of the n factors where n is the number of factors ANCOVA: if independent variables contain both categorical and metric variables Categorical -> still factors Metric IV -> covariates (don’t confuse them with control variables, it just means that you have a metrically IV) - Analysis of COVERIANCE Repeated-measures ANOVA: one factor with at least two levels; levels are dependent - You have a repeated measurement, a before and an after Typical applications: Understanding the logic: Example ANOVA: You want to test the reaction time of drinks: First you can look at the variation within the group, not a lot going on Then you can look at the variation between the different groups, still not a lot going on New data: still not a lot of variation within the groups But a lot of variation between the groups Calcualte the ratio: F = between groups / within groups F ratio: The larger the ratio, the more likely it is that the groups have different means Statistics associated with one-way ANOVA - Sum of squares SSx o The variation Y related to variation in the means of the categories of X o This represents variation between the categories of X, or the proportion of the sum of squares in Y related to X - Sum of square error SSerror o Variation Y due to the variation within each of the categories of X o This variation is not accounted for by X - Sum of squares Y SSy o Total variation in Y - Eta^2 (η^2) o Measures the strength of the effect of X on Y (IV on DV) o Value varies between 0-1, the closer to 1, the stronger the effect. - F statistic o Tests the null hypothesis that the category means are equal in the population o If you accept the F, the population means are equal and find no difference in groups Research process and application Example of an application: First you can plot it https://kaf.brightspace.ru.nl/browseandembed/index/media- redirect/entryid/0_f77qs8pk/showDescription/false/showTitle/false/showTags/false/showDuration/ false/showOwner/false/showUploadDate/false/playerSize/400x285/playerSkin/23454705/thumbEm bed//autoPlay//startTime//endTime/ Second, you van look at the deviation of the grand mean (of the entire student population) First: calculate the SSy Total variance of bear consumption from the grand mean for each student Than you take the deviation points and square them, because sum of squares, add them all and you have SSy=1512 Next step is looking at the deviations from the group means We look at the deviations from each student to the group mean Next, we calculate the SSerror, which is the variance within these groups So you calculate the deviation from the group mean, square them and add them SSerror=462 Next: you look at the deviations of the group means from the grand mean: For each student the group mean, depending on which group the student is from The we look at the deviation from the group mean to the grand mean, and square them SSx: 1050 Calculating the statistics with one-way ANOVA We end up with an F of 2.08 which is smaller than 5.14 which is the critical value of F at an alpha level of.05, it is a non-significant effect. Route in SPSS N-way ANOVA: - Is for two or more factors with at least two levels; levels are independent - In marketing and strategy research, one is often concerned with the effect of more than one factors simultaneously` Main effect vs interaction effect Both of these effects are direct and independent: main effect Advertising level * price level: interaction effect (advertising level combined with price level) N-way analysis of variance - Consider the simple case of two factors X1(advertising levels) and X2 (price level) having categories c1 (the categories of advertising level) and c2 (categories of price levels) - The total variation in this case is portioned as follows: o SSy = SS due to X1 + SS due to X2 + SS due to the interaction of X1 and X2 + SSerror - The strength of the joint effects of two factors, called the overall effect, or multiple η^2, is measured as follows o Multiple η^2 = (SS due to X1 + SS due to X2 + SS due to interaction of X1 and X2)/SSy SPSS Route The significance of the overall effect may be tested by an F test: The significance of the main effect of each factor may be tested as follows for X1: If the overall effect is significant, the next step is to examin the significance of the interaction effect. Under the null hypothesis of no interaction, the appropriate F test is: N-way ANOVA vs ANCOVA - Both considered two or more IVs - N-way ANOVA is only categorical IVs o For example, advertising levels, countries, industries - ANCOVA categorical and metric IVs o Always need at least one categorical, but than you can enrich it with metrically skilled variables ANCOVA - To include statistical control variables ANCOVA mainly for two purposes - In quasi-experimental (observations) designs, to remove effects of variables which modify the relationship of categorical IVs to DVs, so controlling for them - In experimental designs, to control for factors which cannot be randomized but which can be measured on an interval scale - Hence: o Reducing the error term in the model o Procedures of statistical control (e.g. ‘’what if’’ analysis) Repeated measures ANOVA: within subject design - Sometimes you want to asses the difference in a particular variable due to treatment over time o You need a within subjects design or repeated measures analysis of variance for this - You need to repeatedly measure this variable before and after the treatment Interpreting the results is very important here!!! Assumptions before analyzing and conducting an ANOVA - Normality of sampling distribution of means -> usually not a problem if N for each group is > 30 - Independence of errors, no systematic biases in the data o Eror term is normally distributed o Error terms are uncorrelated - Independent scores - Sample size, you need a certain power to be able to conduct these analysis o 30 absolute minimum - Homogeneity of variance o Most important o Testing equal variance across groups o Assumption is important because it strongly affects the F test o Solution is Levene’s test Levene’s test Hypothesis: - H0: the variance of the dependent variable is the same for every group - H1; the variance of the dependent variable is different between groups You would like the Levene’s test to be non-significant that means you will have equal vriances. In case of significant Levene’s test: - When groups have equal size, this is usually not harmful - If the groups doe not have equal size, you should use the Welch statistic. (parametric adaptation of the F test) instead of the F test. It accounts for the differences in the variances in de DV. Issues in interpretation: - When conducting ANOVA on two or more factors, different interactions can arise. - Relative importance of factors o Experimental designs should be balanced => equal sample size for each factor (or category level) No interaction in case 1 - Both slopes develop along the same way Variable 1 has simply two categories, variable 2 has three categories. Other issues in interpretation – between subjects model - So here we need to calculation partioal eta2, given by SPSS - Omega squared indicates what proportion of the variation in DV is relation to a particular IV or factor - Normally, partial eta2 and omega2 are interpreted only for statistically significant effects Issues in interpretation – multiple comparisons - To examine differences among groups (means), we use contrasts - Contrasts are comparisons used to determine which of the means are statically different Different types of contrasts - A priori contrast o Are determined before the analysis, based on researcher’s framework o Deviation ▪ Group means vs. grand mean o Simple ▪ Group mean 1vs group mean 2 - Post hoc contrasts are made after the analysis, they all have little differences, depend on the group size and if the Levene’s test was significant or insignificant, such as: o LSD = least significant difference o Duncan’s multiple range test o Tukey’s altermate procedure o Scheffe’s test Week 45/47, Regression analysis Purpose: to estimate a model to analyse the relationship between (an) independent variable(s) and a dependent variable. - It is one of the most frequently used data analysis methods - Linear dependency between variables Distinguish between variables (all metrically scaled): - Simple regression o 1 metric IV and 1 metric DV - Multiple regression o Several metric IV and 1 metric DV Applications: - Analysis of causes - Forecasting the impact of something - Time-series analysis (prediction of trends) General form of a multiple regression model is as follows (population formula for the general form) DEZE MOET JE KENNEN e is random error General form is estimated by: Y hat, because it is a sample and not population What is the regression variate? It is a linear combination of weighted independent variables used collectively to predict the dependent variable. - It is all from b0 to bk, because it collectively predicts the dependent variable - In multiple regression, the variate represents the highest correlation between IV and DV Process of conducting a multiple regression analysis (MRA) All the methods follow a similar process: from problem formulation to model fit: 1. Objectives of multiple regression - Prediction and explanation o Prediction: concerned about trying to maximize the predicted value of the DV, what set of predictors has the highest prediction power o Explanation: concerned about the linear dependency between the different IVs and DVs. The magnitude, the significance of each IV and the impact on the DV. - Appropriateness of the research problem o Research problem that we are trying to investigate is appropriate for MRA - Specification of a statistical relationship o If we already know that a relationship doesn’t have a linear forms for example, we need to be aware of these relationships - Selection of the IVs and DVs o Theory supports the variables!! o Measurement- and specification error, especially in de DV Rules of thumb: model specification - Measurement error: Structural Equation Modelling (SEM) vs summated scales in multiple regression o SEM can directly asses the error o Summated scales - Irrelevant variables vs omitted variable bias o Irrelevant variables: try out irrelevant variables that you can get out o Omitted variables: forget to measure and include a very crucial variable that could explain a lot of variance to the DV ▪ Rather incorporate an irrelevant variables than a half omitted variable bias - Curvilinear relationships: quadratic and cubic polynomials are generally sufficient o When having a curvilinear relationship you can include quadratic and cubic polynomials, which are usually sufficient 2. Research design of MRA - Sample size, important criteria, with sample size you have a certain power - Unique elements of the dependence relationship o Can use dummy variables as independents to get more power - Nature of the independent variables o Can be both fixed and random parameters Rule of thumb: sample size considerations Simple regression: effective with a sample size of 20 MRA: effective with a sample size of 50, preferably 100 for most research situations Minimum ratio of observations to variables is 5 to 1 Preferred ratio is 15 or 20 to 1 More degrees of freedom - Improves generalizability - Addresses model parsimony and sample size concerns, find the right balance between model parsimony and sample size concerns 3. Assumptions of MRA - Linearity of the phenomenon measured o Does the theory support this assumption? - Constant variables of the residuals - Independence of the residuals - Normality of the residuals’ distribution - Not only hold for each DV and IV, but for the variate as well o Use graphical analysis (i.e., partial regression plots, residual plots, and normal probability plots) Remember from the first clip: variate - Variate is the linear combination of weighted independent variables used collectively to predict the dependent variable - In MRA: variate represents the highest correlation between all the IV and the DV Rules of thumb: assumptions - Remedies for problems in the variate via modifying independent variables o If the variate is not linear, you usually modify the IV, you cannot directly address the variate because it is a collection of IVs, therefore you need to modify the IVs or the set of IVs to address problems in the variate - Linearity: critical issue in MRA o To assure if linearity is there, use the descriptive statistics as we did before ▪ Look at the skewness, kurtosis, how they are distributed and so on o Bivariate relationships can be accessed via residual plot (a) is something you would like to have, there is no particular pattern, unbiased and homoscedastic, variance stays the same (b) is still homoscedastic, it stays the same across the entire residuals, but you can see it is biased (c) it is homoscedastic, but also biased. Normally it is an overlooked non-linear relationship, try out quadratic or cubic terms. (d) unbiased, there is no specific pattern, but they are heteroscedastic, variance is not constant (e) biased and heteroscedastic, almost the same in b but in heteroscedasticity (f) biased and heteroscedastic, some linear term going on but at the same time heteroscedastic Residual plots tell a lot about what might have gone wrong in the model specification Fitted model that is appropriate, no patterns and no heteroscedasticity Potential remedies when you find residual plots - Transform data and make it linear - Including polynomial terms 4. And 5. Estimating regression model and determining the fit 3 Basic tasks 1. We need to select method for specifying the regression model - Confirmatory (simultaneous) o Simply include IVs at the same time, simultaneously - Sequential search methods o Stepwise (step by step), variables are not removed once included in regression equation o Forward inclusion and backward elimination o Hierarchical, you have a certain set of variables that we first want to add to the analysis and so on - Combinatorial (all possible subsets), not used very often 2. Assess statistical significance of overall model is predicting the DV - First of the overall model o Ensure practical significance when using large sample sizes, it is important that you are theory driven o Use the adjuster R2 as you measure of overall model predictive accuracy o You need theoretical support as well - We look at the statistical significance of any regression coefficient, of any IV and its impact on the DV o Was statistical significance established? (is it a significant relationship?) o How does the sample size come into play? o Does it have practical significance (substantiality) in addition to statistical significance? 3. Determine whether any of the observations exert an undue influence on the results 5. Interpretation of dummy models Product placement has 3 categories: standard shelf, head of aisle, mega display Product placement needs to be a dummy variable Two models that were predicted, model a has the constant, price and shoppers Model b has all, plus the dummy model - R Square, which is the coefficient of the determination o How much variance in the DV is explained by all the IVs - Adjusted R2: takes into account complexity of the model o The more variables you add, the more variance you can explain, it correct for the complexity of the model o Therefore, we always look at the adjusted R2 - R Square change o Explained by adding other variables, so the dummy variable explains an additional 14,7 percent - F change statistics o Whether there is something going on in the model o We always want the F and the F change to be significant Sales = 113.575 + 0.019 – 119.05 + 45.095 Head of aisle is not taken into the calculation because it is not significant You cannot asses the e because it is the random error component, you cannot estimate it. - t values o Individual relationships of each IV with the DV o For example, 3.027, which leads to a significant p value of.005 o When t is above 1.96, it is significant at alpha of.05 o All is significant except for head of aisle in reference to standard shelf - Unstandardized coefficients o Relationship of each IV with the DV o Impact that one unit change which the IV has on the variable Sales (DV) - Standardized coefficients o Beta, which you look into when you want to look into relative importance of the IV in the model Issues - Always impact of each IV relative to other variables - Important rule: regression coefficients describe changes in DV due change in an IV if all other IV remain constant o This can be difficult when response formats of IVs vary (different scales) o If this is the case, use beta weights (standardized coefficients) when comparing relative importance among IVs - Multicollinearity o It happens when IV strongly intercorrelate with each other o You can assess degrees of multicollinearity ▪ Variance inflation factors (VIF) (should be at least one assigned indicator o Otherwise the structure model can’t run, it needs information - Each indicator can only be assigned once In de indicator to variable relationship distinguishes between: - Composite models o With composite the indicators form the latent variable - Reflective models o With reflective the arrow is always from the latent variable to the indicator o It causes the indicator - Direction is always from the construct to measure - Indicators are expected to be corrected - Dropping an indicator -> does not alter meaning of construct, because it is still reflected by the others - It considers measurement error at item level, so each item has an error - Similar to factor analysis - Typical for consumer research constructs o Attitudes for example - Direction is unspecified - Correlations between indicators are common, but not required - Dropping an indicator -> alters meaning of construct - Weights are predefined or estimated o If estimated by means of multiple regression, beware of multicollinearity - Typical for design research 3. Ensuring requirements and assumptions Sample size requirements in PLS - Ten times the number of maximum arrowheads pointing on a latent variable - Arrows belong to either o Structural model o Measurement model - ξ 1 with 3 observed variables, composed construct - ξ 2 with 2 reflective observables, reflective construct - η1 , which is reflected by Y1 to 4 The highest construct of arrowheads is 3, at ξ 1, therefore, minimum N=30 PLS is very nice to use with small sample sizes Even with complex models like this, you can observe with N=30 Adequate sample size - Technically, possible o N at least one other correlated construct 4. Assessing the measurement model First you have to assess the measurement model, secondly the structural model!!! Validation of the measurement model is a requirement for assessing the structural model Model fit compares theory to reality = assessing similarity of estimates covariance matrix (theory) to observed covariance matrix (reality) Saturated model in PLS: Tests of exact model fit - Rely on inference statistics (e.g. bootstrap) - What you need to know now is what are we testing? - Focal question: is the difference between estimated and empirical correlation matrix so small that it can be purely attributed to sampling error? Recommended threshold: difference should be non-significant (p-value > 0.05) Saturated model in PLS: test of approximate model fit - SRMR = measure of approximate model fit - Focal question: is the correlation matrix implied by our model sufficiently similar to empirical correlation matrix? Recommended threshold: the difference should be non-significant (p-value indicator reliability -> squared indicator loading Convergence validity: Average variance extracted (AVE) - Comparable to proportion of explained variance in factor analysis o Between 0 and 1 o AVE > 0.5 sign for unidimensionality ▪ That means that the indicators and thereby the variance you extract out of these indicators into this construct are unidimensional in the sense that this factor is the only factor that they can form, so no other factors. Discriminant validity: heterotrait-monotrait ratio of correlations (HTMT) You want to make sure that these indicators that have converged to one particular factor or construct do not load on any of the other factors, so no cross loading, they should discriminate the other factors. - Derived from multitrait-multimethod matrix (MTMM) - Estimate of construct correlation o Tells you something of these intercorrelations of these indicators between constructs - Threshold: HTMT.85: HTMT