Inferential Statistics PDF
Document Details
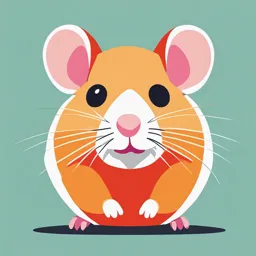
Uploaded by HandsDownAntigorite4242
Tags
Summary
This document provides an overview of inferential statistics, covering topics such as hypothesis testing, different types of statistical tests and how to interpret results.
Full Transcript
Inferential statistics allow us to infer the characteristic(s) of a population from sample data. Inferential statistics requires the performance of statistical tests to see if a conclusion is correct compared with the probability that conclusion is due to chance. These tests...
Inferential statistics allow us to infer the characteristic(s) of a population from sample data. Inferential statistics requires the performance of statistical tests to see if a conclusion is correct compared with the probability that conclusion is due to chance. These tests calculate a P-value that is then compared with the probability that the results are due to chance. www.statstutor.ac.uk An objective method of making decisions or inferences from sample data (evidence) Sample data used to choose between two choices i.e. hypotheses or statements about a population We typically do this by comparing what we have observed to what we expected if one of the statements (Null Hypothesis) was true www.statstutor.ac.uk Always two hypotheses: HA: Research (Alternative) Hypothesis What we aim to gather evidence of Typically that there is a difference/effect/relationship etc. H0: Null Hypothesis What we assume is true to begin with Typically that there is no difference/effect/relationship etc. www.statstutor.ac.uk Members of a jury have to decide whether a person is guilty or innocent based on evidence Null: The person is innocent Alternative: The person is not innocent (i.e. guilty) The null can only be rejected if there is enough evidence to doubt it i.e. the jury can only convict if there is beyond reasonable doubt for the null of innocence They do not know whether the person is really guilty or innocent so they may make a mistake www.statstutor.ac.uk Controlled via sample Typically restrict to a 5% Risk size (=1-Power of test) = level of significance Study reports Study reports NO difference IS a difference (Do not reject H0) (Reject H0) H0 is true Difference Does NOT exist in X Type I Error population HA is true Difference DOES exist in population X Type II Error Prob of this = Power of test www.statstutor.ac.uk Define study question Set null and alternative hypothesis Choose a suitable test Calculate a test statistic Calculate a p-value Make a decision and interpret your conclusions www.statstutor.ac.uk The chi-squared test is used when we want to see if two categorical variables are related The test statistic for the Chi-squared test uses the sum of the squared differences between each pair of observed (O) and expected values (E) = 2 n (Oi − Ei ) 2 i =1 Ei www.statstutor.ac.uk Analyse → Descriptive Statistics → Crosstabs Click on ‘Statistics’ button & select Chi-squared Test Statistic = 127.859 p- value p < 0.001 Note: Double clicking on the output will display the p-value to more decimal places www.statstutor.ac.uk We can use statistical software to undertake a hypothesis test e.g. SPSS One part of the output is the p-value (P) If P < 0.05 reject H0 => Evidence of HA being true (i.e. IS association) If P > 0.05 do not reject H0 (i.e. NO association) www.statstutor.ac.uk www.statstutor.ac.uk Summarizing Means Calculate summary statistics by group Look for outliers/ errors Use a box-plot or confidence interval plot www.statstutor.ac.uk T-tests are used to compare two population means. ₋ Paired data: same individuals studied at two different times or under two conditions PAIRED T-TEST ₋ Independent: data collected from two separate groups INDEPENDENT SAMPLES T-TEST www.statstutor.ac.uk Paired or unpaired? If the same people have reported their hours for 1988 and 2021 have PAIRED measurements of the same variable (hours) Paired Null hypothesis: The mean of the paired differences = 0 If different people are used in 1988 and 2021 have independent measurements Independent Null hypothesis: The mean hours worked in 1988 is equal to the mean for 2021 H 0 : 1988 = 2014 www.statstutor.ac.uk The t-distribution is similar to the standard normal distribution but has an additional parameter called degrees of freedom (df or v) For a paired t-test, v = number of pairs – 1 For an independent t-test, v = ngroup1 + ngroup 2 − 2 Used for small samples and when the population standard deviation is not known Small sample sizes have heavier tails www.statstutor.ac.uk As the sample size gets big, the t-distribution matches the normal distribution Normal curve www.statstutor.ac.uk Normality: Plot histograms ◦ One plot of the paired differences for any paired data ◦ Two (One for each group) for independent samples ◦ Don’t have to be perfect, just roughly symmetric Equal Population variances: Compare sample standard deviations ◦ As a rough estimate, one should be no more than twice the other ◦ Do an F-test (Levene’s in SPSS) to formally test for differences However the t-test is very robust to violations of the assumptions of Normality and equal variances, particularly for moderate (i.e. >30) and larger sample sizes www.statstutor.ac.uk There are alternative tests which do not have these assumptions Test Check Equivalent non-parametric test Independent t-test Histograms of data by Mann-Whitney group Paired t-test Histogram of paired Wilcoxon signed rank differences www.statstutor.ac.uk Every sample taken from a population, will contain different Sample A numbers so the mean n=20 Mean = 277 varies. 𝑥ҧ Sample B Which estimate is most Population Mean = ? n=50 reliable? SD =? Mean = 274 𝑥ҧ How certain or Sample C uncertain are we? n=300 Mean = 275 𝑥ҧ www.statstutor.ac.uk A range of values within which we are confident (in terms of probability) that the true value of a pop parameter lies A 95% CI is interpreted as 95% of the time the CI would contain the true value of the pop parameter i.e. 5% of the time the CI would fail to contain the true value of the pop parameter www.statstutor.ac.uk www.statstutor.ac.uk Are boys more likely to prefer maths and science than girls? Variables: Favorite subject (Nominal) Gender (Binary/ Nominal) Summarise using %’s/ stacked or multiple bar charts Test: Chi-squared Tests for a relationship between two categorical variables www.statstutor.ac.uk Relationship between two scale variables: Explores the way the two co-vary: (correlate) ₋ Positive / negative ₋ Linear / non-linear Outlier ₋ Strong / weak Presence of outliers Statistic used: r = correlation coefficient Linear www.statstutor.ac.uk Correlation Coefficient r Measures strength of a relationship between two continuous variables -1 ≤ r ≤ 1 r = 0.9 r = 0.01 r = -0.9 www.statstutor.ac.uk An interpretation of the size of the coefficient has been described by Cohen (1992) as: Correlation coefficient value Relationship -0.3 to +0.3 Weak -0.5 to -0.3 or 0.3 to 0.5 Moderate -0.9 to -0.5 or 0.5 to 0.9 Strong -1.0 to -0.9 or 0.9 to 1.0 Very strong Cohen, L. (1992). Power Primer. Psychological Bulletin, 112(1) 155-159 www.statstutor.ac.uk Regression is useful when we want to a) look for significant relationships between two variables b) predict a value of one variable for a given value of the other It involves estimating the line of best fit through the data which minimises the sum of the squared residuals. Residuals are the differences between the observed and predicted weights www.statstutor.ac.uk Simple linear regression looks at the relationship between two Scale variables by producing an equation for a straight line of the form Independent Dependent variable variable y = a + x Intercept Slope which uses the independent variable to predict the dependent variable www.statstutor.ac.uk We are often interested in how likely we are to obtain our estimated value of if there is actually no relationship between x and y in the population One way to do this is to do a test of significance for the slope H0 : = 0 www.statstutor.ac.uk Key regression table: Y = -6.66 + 0.36x P – value < 0.001 As p < 0.05, gestational age is a significant predictor of birth weight. Weight increases by 0.36 lbs for each week of gestation. www.statstutor.ac.uk How much of the variation in birth weight is explained by the model including Gestational age? Proportion of the variation in birth weight explained by the model R2 = 0.499 = 50% Predictions using the model are fairly reliable. Which variables may help improve the fit of the model? Compare models using Adjusted R2 www.statstutor.ac.uk Assumption Plot to check The relationship between the independent and Original scatter plot of the dependent variables is linear. independent and dependent variables Homoscedasticity: The variance of the residuals Scatterplot of standardised about predicted responses should be the same predicted values and residuals for all predicted responses. The residuals are independently normally Plot the residuals in a distributed Look for patterns. histogram www.statstutor.ac.uk Histogram of the residuals looks approximately normally distributed When writing up, just say ‘normality checks were carried out on the residuals and the assumption of normality was met’ Outliers are outside 3 www.statstutor.ac.uk Are there any patterns as the predicted values increases? There is a problem with Homoscedasticity if the scatter is not random. A “funnelling” shape such as this suggests problems. www.statstutor.ac.uk If the residuals are heavily skewed or the residuals show different variances as predicted values increase, the data needs to be transformed Try taking the natural log (ln) of the dependent variable. Then repeat the analysis and check the assumptions www.statstutor.ac.uk Multiple regression Multiple regression has several binary or Scale independent variables y = a + 1 x1 + 2 x2 + 3 x3 Categorical variables need to be recoded as binary dummy variables Effect of other variables is removed (controlled for) when assessing relationships www.statstutor.ac.uk In addition to the standard linear regression checks, relationships BETWEEN independent variables should be assessed Multicollinearity is a problem where continuous independent variables are too correlated (r > 0.8) Relationships can be assessed using scatterplots and correlation for scale variables SPSS can also report collinearity statistics on request. The VIF should be close to 1 but under 5 is fine whereas 10 + needs checking www.statstutor.ac.uk www.statstutor.ac.uk Choosing the right test 1) A clearly defined research question 2) What is the dependent variable and what type of variable is it? 3) How many independent variables are there and what data types are they? 4) Are you interested in comparing means or investigating relationships? 5) Do you have repeated measurements of the same variable for each subject? www.statstutor.ac.uk Clear questions with measurable quantities Which variables will help answer these questions Think about what test is needed before carrying out a study so that the right type of variables are collected www.statstutor.ac.uk Dependent variables INDEPENDENT DEPENDENT (explanatory/ affects (outcome) predictor) variable variable Does attendance have an association with exam score? Do women do more housework than men? www.statstutor.ac.uk Dependent Scale Categorical Ordinal Nominal www.statstutor.ac.uk How can ‘better’ be measured and what type of variable is it? Exam score (Scale) Do boys think they are better at maths?? I consider myself to be good at maths (ordinal) www.statstutor.ac.uk How many variables are involved? Two – interested in the relationship One dependent and one independent One dependent and several independent variables: some may be controls Relationships between more than two: multivariate techniques (not covered here) www.statstutor.ac.uk Data types Research question Dependent/ Independent/ outcome variable explanatory variable Does attendance have an Exam score (scale) Attendance (Scale) association with exam score? Do women do more Hours of Gender (binary) housework than men? housework per week (Scale) www.statstutor.ac.uk Dependent = Scale Independent = Categorical How many means are you comparing? Do you have independent groups or repeated measurements on each person? www.statstutor.ac.uk Comparing measurements on the same people Also known as within group comparisons or repeated measures. Can be used to look at differences in mean score: (1) over 2 or more time points e.g. 1988 vs 2014 (2) under 2 or more conditions e.g. taste scores Participants are asked to taste 2 types of cola and give each scores out of 100. Dependent = taste score Independent = type of cola www.statstutor.ac.uk Comparing means Independent t-test 2 Comparing BETWEEN groups 3+ Comparing means Comparing 2 Paired t-test measurements WITHIN the same subject 3+ www.statstutor.ac.uk Comparing means Independent t-test 2 Comparing BETWEEN groups One way 3+ ANOVA Comparing means Comparing 2 Paired t-test measurements WITHIN the same subject 3+ Repeated measures ANOVA ANOVA = Analysis of variance www.statstutor.ac.uk Investigating relationships Dependent Independent Test between variable variable 2 categorical variables Categorical Categorical Chi-squared test 2 Scale variables Scale Scale Pearson’s correlation Predicting the value of an Scale Scale/binary Simple Linear Regression dependent variable from the value of a independent Binary Scale/ binary Logistic regression variable Note: Multiple linear regression is when there are several independent variables www.statstutor.ac.uk