ANOVA Analysis of Variance PDF
Document Details
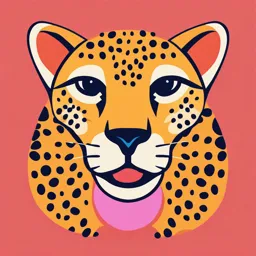
Uploaded by PrizeMeteor7340
Universiti Malaysia Sarawak
2024
Tags
Related
Summary
These notes provide a comprehensive explanation of Analysis of Variance (ANOVA), a statistical method used to compare means across multiple groups. The document explains the concept of ANOVA, its application in hypothesis testing, and detailed calculations. The example provided demonstrates the application of ANOVA to assess whether temperature levels significantly influence production rates.
Full Transcript
ANOVA Analysis of Variance Why Analyse the Variance? What are we interested in? We are interested to compare means (> than 2 means) We use variation (variance, s2) of data set as a tool to help us achieve our objectives (to compare means). Previously, we have tested hypothesis about two means. Bu...
ANOVA Analysis of Variance Why Analyse the Variance? What are we interested in? We are interested to compare means (> than 2 means) We use variation (variance, s2) of data set as a tool to help us achieve our objectives (to compare means). Previously, we have tested hypothesis about two means. But, if we’re interested to test hypothesis involve several means, what should we do. H0 : 1 = 2 = 3 = 4 = 5 Applying what we have learnt so far, we could test 2 the means at any one time. H1 : 1 = 2 H2 : 1 = 3 H3 : 1 = 4 H4 : 1 = 5 H5 : 2 = 3 H6 : 2 = 4 H7 : 2 = 5 H8 : 3 = 4 H9 : 3 = 5 H10 : 4 = 5 So, using what we have learnt about testing of hypothesis involving difference b/w means, to test the hypothesis that all 5 means are equal, we would have to test each of these 10 hypothesis. Rejecting any ONE of the 10 hypothesis If we fail to reject all about 2 means would 10 hypothesis about cause us to reject the the means, we would null that all 5 means fail to reject the null! are equal. One/Two Ways ANOVA? For our purpose we are only looking at one way. What does that mean? There is only one independent variable vs one dependent variable. ANOVA technique allows us to test the null hypothesis (all means are equal) against the alternative hypothesis ( AT LEAST one mean value is different) Example The temperature at which a plant is maintained is believed to affect the rate of production in the plant. Data in the following table are the number, x of unit produced in one hour for randomly selected one-hour period when the production process in the plant was operating at each of three temperature levels. Temperature Levels Sample from 68°F (k=1) 72 °F (k=2) 76 °F (k=3) 10 7 3 12 6 5 10 7 4 9 8 3 7 ∑(Column) C1 = 41 C2 = 35 C3 = 15 Total n1 = 4 n2 = 5 n3 = 4 Xi indicates the observed production mean at level i. K= 1, 2, & 3 corresponds to temperatures of 68°F, 72°F, 76°F. You observe there is variation among the sample mean! The question is: Do these variation indicate that the population mean is different? they came from different population. i.e. if they come from different population temperature has effect < 1 2 3 > If they come from the same population temperature has no effect < 1 = 2 = 3 > effect on output level. Note: ANOVA analysis of variance We are employing the variation in the data set to help us make decision! Within group variation Between group variation Question : Do these data suggest that temperature has a significant effect on the production level at 0.05 level of significance? Null hypothesis H0: 68 = 72 = 76 < the temperature does not have a significant effect on the production rate> H1: Not all temp. level means are equal or : At least two of the are not equal or : H0 is false We will make the decision to reject H0 or fail to reject H0 by using the F-distribution & F- statistics. ANOVA separates the variation among the entire set of data into 2 categories. To accomplish this separation, we first work with the numerator of the fraction used to define sample variance (this is also of course variation). The numerator of this fraction is called the sum of squares (SS) Sum of squares = From calculation, SS(total) = 94 SS(total) (94) Dibahagikan SS (temperature) SS(error) Variation due to Variation due to error temperature level when we take sample (between group) (within) SSBG SSWG SSBG + SSWG = SSTotal = 94 Formula for SSBG SSBG = (c21/n1 + c22/n2 + c2/n3 + …)- (x)2/N Ci represents column total Ni represents sample size for each column N represents sample size i.e. N= ni SSBG = (412/4+ 352/5+ 152/4) – (91)2/13 = (420.25+245+ 56.25) – 637 = 721.5 – 637 = 84.5 SSWG measures variation within the row SSWG = (x2) - (c21/n1 + c22/n2 + c2/n3 + …) We know (x2)= 731 (found previously) c21/n1 + c22/n2 + c2/n3 = 721.5 (found previously) Hence, SS(error) = 731-721.5 = 9.5 Note SS(total) = SSBG + SSWG We could verify this we found that SS(total) = 94 And, SSBG = 84.5 SSWG = 9.5 SSTotal = 84.5 + 9.5 = 94 (validated) For convenience, we would use an ANOVA table to record the sum of squares &to organize the rest of the calculations. Of what? Variation Source ss df ms Between group (MEMORISE) Within group Total Degree of Freedom We have calculated the three sums of squares for our illustration. The degree of freedom, df, associated with each of the three source are determined as follows: 1. df(between group) is one less than the number of jewels (column) for which the factor is tested. dfBG = K – 1 = 3-1 =2 dfToT = N – 1 = 13 – 1 = 12 dfwg = N – K = 13 – 3 = 10 So, just like sum of squares (ss), df also must balance up. < dfBG + dfwg = dfToT > 2 + 10 = 12 SSBW + SSWG = SS(total) dfBW + dfWG = dftotal When we test hypothesis, we have to use mean squares (MS). Mean of Squares MSquares for the factor being tested, MS BG, MSWG are obtained by dividing the sum of square value by corresponding number of freedom. MSBG = SSBG dfBG MSWG = SSWG dfWG Mean squares for our illustrations are MSBG = SSBG = 84.5 dfBG 2 = 42.25 Msquares for the factor being tested, MSBG, MSWG are obtained by dividing the sum of square value by corresponding number of freedom. MSBG = SSBG dfBG MSWG = SSWG dfWG Mean squares for our illustrations are MSBG = SSBG = 84.5 dfBG 2 = 42.25 MSWG = SSWG = 9.5 = 0.95 dfWG 10 The completed ANOVA is as follows. The hypothesis test is now completed using the two mean squares as measures of variance. Source SS df MS F ___ BG 84.5 2 42.25 44.47 WG 9.5 10 0.95 Total 94 12 The calculated value of the test statistic F is found by dividing MSBG by MSWG I.e. F = MSBG MSWG The decision to reject H0 or fail to reject H0 will be made by comparing the F value to a one-tailed critical value of F obtained from table. F = 42.25 0.95 = 44.47 The critical value is F(2,10,0.05) = 4.10 = 0.05 Rejection Region 4.10 (critical value) Hint: to find critical value from table, = 0.05, df for numerator (dfBG) = 2 df for denumerator (dfWG) = 10 We reject H0 because the value of F (test statistic) falls in the rejection region. Conclusion We therefore conclude that: At least one of the room temperatures does have significant effect on the production rate. OR There is a significant difference of production date for at least any two of the room temperatures. Multiple Comparison Procedure In ANOVA, we test the difference of > than 2 means When we reject H0, we accept H1 H1 Says not all means are equal / There is a significant difference of mean for at least any two of the groups. H0 : 1 = 3 = 4 = 2 = 5 If H1 is true, exactly where the differences lie multiple comparison procedure Multiple Comparison Procedure In the example below which test the difference in the electricity consumptions of 4 cities in Australia It was found that there are differences Multiple Comparison Procedure is carried out Can you tell where lies the difference (p < 0.05) Country Country Sig. (p- value) Adelaide Hobart 0.723 Melbourne 0.231 Perth 0.008 Hobart Adelaide 0.723 Melbourne 0.823 Perth 0.127 Melbourne Adelaide 0.231 Hobart 0.823 Perth 0.535 Perth Adelaide 0.008 Hobart 0.127 Melbourne 0.535 SPSS Questions A sleep reseacher hypothesized that children with different personality are different in terms of duration of time spent on deep sleep (Delta sleep). Test if the null hypothesis is true