Text Chapters 12-13 PDF
Document Details
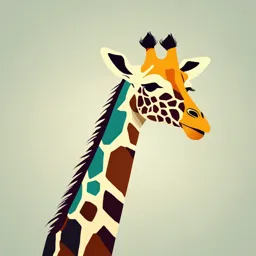
Uploaded by TrustworthyMimosa
University of Sharjah
Tags
Summary
This textbook chapter, titled 'Sampling', explains the importance of targeted data collection in research. It covers defining key terms like population and samples, the sampling process, different types of sampling designs, and the connection between sample statistics and population parameters. It's a valuable resource for understanding research methods in the context of data analysis.
Full Transcript
CHAPTER 13 Sampling LEARNING OBJECTIVES After completing Chapter 13, you should be able to: 1. Define sampling, sample, population, element, sampling unit, and subject. 2. Discuss statistical terms in sampling. 3. Describe and discuss the sampling process. 4. Compare and contr...
CHAPTER 13 Sampling LEARNING OBJECTIVES After completing Chapter 13, you should be able to: 1. Define sampling, sample, population, element, sampling unit, and subject. 2. Discuss statistical terms in sampling. 3. Describe and discuss the sampling process. 4. Compare and contrast specific probability sampling designs. 5. Compare and contrast specific nonprobability sampling designs. 6. Discuss precision and confidence and the trade-off between precision and confidence. 7. Discuss how hypotheses can be tested with sample data. 8. Discuss the factors to be taken into consideration for determining sample size and determine the sample size for any given research project. 9. Discuss sampling in qualitative research. 10. Discuss the role of the manager in sampling. INTRODUCTION Experimental designs and surveys are useful and powerful in finding answers to research questions through data collection and subsequent analyses, but they can do more harm than good if the population is not correctly tar- geted. That is, if data are not collected from the people, events, or objects that can provide the correct answers to solve the problem, the research will be in vain. The process of selecting the right individuals, objects, or events as representatives for the entire population is known as sampling, which we will examine in some detail in this chapter (see shaded portion in Figure 13.1). The reasons for using a sample, rather than collecting data from the entire population, are self‐evident. In research investigations involving several hundreds and even thousands of elements, it would be practically impossible to collect data from, or test, or examine, every element. Even if it were possible, it would be prohibitive in terms of time, cost, and other human resources. Study of a sample rather than the entire population is also sometimes likely to produce more reliable results. This is mostly because fatigue is reduced and fewer errors 235 236 research methods for business DETAILS OF STUDY MEASUREMENT Purpose of the Extent of researcher Measurement Study setting Research strategies study interference and measures DATA Experiment Operational ANALYSIS Survey research Minimal: Studying events definition Exploration Contrived Observation as they normally occur Items (measure) 1. Feel for Description Case studies Manipulation and/or Noncontrived Scaling data Hypothesis testing Grounded theory control and/or simulation Action research Categorizing Mixed methods Coding PROBLEM STATEMENT 2. Goodness of data Unit of analysis Sampling Time Data collection (population to design horizon method be studied) 3. Hypothesis Individuals Probability/ Interviews testing One-shot Dyads nonprobability (cross-sectional) Observation Groups Sample Longitudinal Questionnaires size (n) Physical measurement Organizations Machines Unobtrusive etc. FIGURE 13.1 The research process and where this chapter fits in therefore result in collecting data, especially when a large number of elements is involved. In a few cases, it would also be impossible to use the entire population to gain knowledge about, or test, something. Consider, for instance, the case of electric light bulbs. In testing the life of bulbs, if we were to burn every single bulb produced, there would be none left to sell! This is known as destructive sampling. POPULATION, ELEMENT, SAMPLE, SAMPLING UNIT, AND SUBJECT In learning how representative data (i.e., as reflected in the universe) can be collected, a few terms, as described below, have first to be understood. Population The population refers to the entire group of people, events, or things of interest that the researcher wishes to investigate. It is the group of people, events, or things of interest for which the researcher wants to make infer- ences (based on sample statistics). For instance, if the CEO of a computer firm wants to know the kinds of adver- tising strategies adopted by computer firms in the Silicon Valley, then all computer firms situated there will be the population. If an organizational consultant is interested in studying the effects of a four‐day work week on the white‐collar workers in a telephone company in Ireland, then all white‐collar workers in that company will make up the population. If regulators want to know how patients in nursing homes run by a company in France chapter sampling 237 are cared for, then all the patients in all the nursing homes run by them will form the population. If, however, the regulators are interested only in one particular nursing home run by that company, then only the patients in that specific nursing home will form the population. Element An element is a single member of the population. If 1000 blue‐collar workers in a particular organization happen to be the population of interest to a researcher, each blue‐collar worker therein is an element. If 500 pieces of machinery are to be approved after inspecting a few, there will be 500 elements in this population. Incidentally, the census is a count of all elements in the human population. Sample A sample is a subset of the population. It comprises some members selected from it. In other words, some, but not all, elements of the population form the sample. If 200 members are drawn from a population of 1000 blue‐ collar workers, these 200 members form the sample for the study. That is, from a study of these 200 members, the researcher will draw conclusions about the entire population of 1000 blue‐collar workers. Likewise, if there are 145 in‐patients in a hospital and 40 of them are to be surveyed by the hospital administrator to assess their level of satisfaction with the treatment received, then these 40 members will be the sample. A sample is thus a subgroup or subset of the population. By studying the sample, the researcher should be able to draw conclusions that are generalizable to the population of interest. Sampling unit The sampling unit is the element or set of elements that is available for selection in some stage of the sampling process. Examples of sampling units in a multistage sample are city blocks, households, and individuals within the households. Subject A subject is a single member of the sample, just as an element is a single member of the population. If 200 mem- bers from the total population of 1000 blue‐collar workers form the sample for the study, then each blue‐collar worker in the sample is a subject. As another example, if a sample of 50 machines from a total of 500 machines is to be inspected, then every one of the 50 machines is a subject, just as every single machine in the total popula- tion of 500 machines is an element. SAMPLE DATA AND POPULATION VALUES When we sample, the sampling units (employees, consumers, and the like) provide us with responses. For instance, a consumer responding to a survey question may give a response of “3”. When we examine the responses that we get for our entire sample, we make use of statistics. In Chapter 14 we will explain that there is a wide variety of statistics we can use, such as the mean, the median, or the mode. The reason we sample, however, is that we are interested in the characteristics of the population we sample from. If we study the entire population and calculate the mean or the standard deviation, then we don’t refer to this as a statistic. Instead, we call it a parameter of the population. 238 research methods for business Sample Population Statistics Parameters (X, S, S2) Estimate FIGURE 13.2 The relationship between sample and population Parameters The characteristics of the population such as μ (the population mean), σ (the population standard deviation), and σ2 (the population variance) are referred to as its parameters. The central tendencies, the dispersions, and other statistics in the sample of interest to the research are treated as approximations of the central tendencies, dispersions, and other parameters of the population. As such, all conclusions drawn about the sample under study are generalized to the population. In other words, the sample statistics – X (the sample mean), S (the standard deviation), and S2 (the variation in the sample) – are used as estimates of the population parameters μ, σ, and σ2. Figure 13.2 shows the relationship between the sample and the population. Representativeness of Samples Visit the companion website at www.wiley.com/college/sekaran for Author Video: Representativeness of samples. The need to choose the right sample for a research investigation cannot be overemphasized. We know that rarely will the sample be an exact replica of the population from which it is drawn. For instance, very few sample means (X) are likely to be exactly equal to the population means (μ). Nor is the standard deviation of the sample (S) likely to be the same as the standard deviation of the population (σ). However, if we choose the sample in a scientific way, we can be reasonably sure that the sample statistic (e.g., X, S, or S2) is fairly close to the population parameter (i.e., μ, σ, or σ2). To put it differently, it is possible to choose the sample in such a way that it is representative of the popu- lation. There is always a slight probability, however, that sample values might fall outside the population parameters. Normality of Distributions Attributes or characteristics of the population are generally normally distributed. For instance, when attributes such as height and weight are considered, most people will be clustered around the mean, leaving only a small number at the extremes who are either very tall or very short, very heavy or very light, and so on, as indicated in Figure 13.3. If we are to estimate the population characteristics from those represented in a sample with reason- able accuracy, the sample has to be chosen so that the distribution of the characteristics of interest follows the same pattern of normal distribution in the sample as it does in the population. From the central limit theorem, we know that the sampling distribution of the sample mean is normally distributed. As the sample size n increases, the means of the random samples taken from practically any population approach a normal distribution with chapter sampling 239 Low 𝜇 High FIGURE 13.3 Normal distribution in a population mean μ and standard deviation σ. In sum, irrespective of whether or not the attributes of the population are normally distributed, if we take a sufficiently large number of samples and choose them with care, we will have a sampling distribution of the means that has normality. This is the reason why two important issues in sampling are the sample size (n) and the sampling design, as discussed later. When the properties of the population are not overrepresented or underrepresented in the sample, we have a representative sample. When a sample consists of elements in the population that have extremely high values on the variable we are studying, the sample mean X will be far higher than the population mean μ. If, in contrast, the sample subjects consist of elements in the population with extremely low values on the variable of interest, the sample mean will be much lower than the true population mean μ. If our sampling design and sample size are right, however, the sample mean X will be within close range of the true population mean μ. Thus, through appropriate sampling design, we can ensure that the sample subjects are not chosen from the extremes, but are truly representative of the properties of the population. The more representative of the population the sample is, the more generalizable are the findings of the research. Recall that generalizability is one of the hallmarks of scientific research, as we saw in Chapter 2. While, in view of our concern about generalizability, we may be particular about choosing representative samples for most research, some cases may not call for such regard to generalizability. For instance, at the explor- atory stages of fact finding, we may be interested only in “getting a handle” on the situation, and therefore limit the interview to only the most conveniently available people. The same is true when time is of the essence, and urgency in getting information overrides a high level of accuracy in terms of priority. For instance, a film agency might want to find out quickly the impact on the viewers of a newly released film shown the previous evening. The interviewer might question the first 20 people leaving the theater after seeing the film and obtain their reac- tions. On the basis of their replies, she may form an opinion as to the likely success of the film. As another exam- ple, a restaurant manager might want to find the reactions of customers to a new item added to the menu to determine whether or not it has been a popular and worth while addition. For this purpose, the first 15 people who chose the special item might be interviewed, and their reactions obtained. In such cases, having instant information may be more gainful than obtaining the most representative facts. It should, however, be noted that the results of such convenient samples are not reliable and can never be generalized to the population. THE SAMPLING PROCESS Sampling is the process of selecting a sufficient number of the right elements from the population, so that a study of the sample and an understanding of its properties or characteristics make it possible for us to generalize such properties or characteristics to the population elements. The major steps in sampling include: 240 research methods for business 1. Define the population. 2. Determine the sample frame. 3. Determine the sampling design. 4. Determine the appropriate sample size. 5. Execute the sampling process. Defining the population Sampling begins with precisely defining the target population. The target population must be defined in terms of elements, geographical boundaries, and time. For instance, for a banker interested in saving habits of blue‐collar workers in the mining industry in the United States, the target population might be all blue‐collar workers in that industry throughout the country. For an advertising agency interested in reading habits of elderly people, the target population might be the German population aged 50 and over. These examples illustrate that the research objective and the scope of the study play a crucial role in defining the target population. Determining the sample frame The sampling frame is a (physical) representation of all the elements in the population from which the sample is drawn. The payroll of an organization would serve as the sampling frame if its members are to be studied. Likewise, the university registry containing a listing of all students, faculty, administrators, and support staff in the university during a particular academic year or semester could serve as the sampling frame for a study of the university population. A roster of class students could be the sampling frame for the study of students in a class. The telephone directory is also frequently used as a sampling frame for some types of study, even though it has an inherent bias inasmuch as some numbers are unlisted and certain others may have become obsolete. Although the sampling frame is useful in providing a listing of each element in the population, it may not always be a current, up‐to‐date document. For instance, the names of members who have recently left the organization or dropped out of the university, as well as members who have only recently joined the organiza- tion or the university may not appear in the organization's payroll or the university registers on a given day. The most recently installed or disconnected telephones will not, likewise, be included in the current telephone directory. Hence, though the sampling frame may be available in many cases, it may not always be entirely cor- rect or complete. When the sampling frame does not exactly match the population coverage error occurs. In some cases, the researcher might recognize this problem and not be too concerned about it, because the dis- crepancy between the target population and the sampling frame is small enough to ignore. However, in most cases, the researcher should deal with this error by either redefining the target population in terms of the sam- pling frame, screening the respondents with respect to important characteristics to ensure that they meet the criteria for the target population, or adjusting the collected data by a weighting scheme to counterbalance the coverage error. Determining the sampling design There are two major types of sampling design: probability and nonprobability sampling. In probability sampling, the elements in the population have some known, nonzero chance or probability of being selected as sample subjects. In nonprobability sampling, the elements do not have a known or predetermined chance of being selected as subjects. Probability sampling designs are used when the representativeness of the sample is of impor- tance in the interests of wider generalizability. When time or other factors, rather than generalizability, become critical, nonprobability sampling is generally used. Each of these two major designs has different sampling chapter sampling 241 strategies. Depending on the extent of generalizability desired, the demands of time and other resources, and the purpose of the study, different types of probability and nonprobability sampling design are chosen. The choice of the sampling procedure is a very important one. Therefore, this chapter will elaborately discuss the different types of sampling designs, bearing in mind the following points in the determination of the choice: What is the relevant target population of focus to the study? What exactly are the parameters we are interested in investigating? What kind of a sampling frame is available? What costs are attached to the sampling design? How much time is available to collect the data from the sample? Determining the sample size Is a sample size of 40 large enough? Or do you need a sample size of 75, 180, 384, or 500? Is a large sample better than a small sample; that is, is it more representative? The decision about how large the sample size should be can be a very difficult one. We can summarize the factors affecting decisions on sample size as: 1. The research objective. 2. The extent of precision desired (the confidence interval). 3. The acceptable risk in predicting that level of precision (confidence level). 4. The amount of variability in the population itself. 5. The cost and time constraints. 6. In some cases, the size of the population itself. Thus, how large your sample should be is a function of these six factors. We will have more to say about sample size later on in this chapter, after we have discussed sampling designs. Executing the sampling process The following two examples illustrate how, in the final stage of the sampling process, decisions with respect to the target population, the sampling frame, the sample technique, and the sample size have to be implemented. EXAMPLE A satisfaction survey was conducted for a computer person, leaving one out of ten randomly selected stores, retailer in New Zealand. The objective of this survey in randomly selected cities, in randomly selected was to improve internal operations and thus to retain regions, was approached during a one‐week period (the more customers. The survey was transactional in sampling technique). Trained interviewers that were nature; service satisfaction and several related variables sent out with standardized questionnaires approached were measured following a service encounter (i.e., a 732 customers leaving the stores (the sample size). visit to the retailer). Hence, customer feedback was A young researcher was investigating the anteced- obtained while the service experience was still fresh. To ents of salesperson performance. To examine his obtain a representative sample of customers of the hypotheses, data were collected from chief sales exe- computer retailer (the target population), every tenth cutives in the United Kingdom (the target population) 242 research methods for business via mail questionnaires. The sample was initially drawn were subsequently distributed to chief sales executives from a published business register (the sampling frame), of 450 companies (the sample size). To enhance the but supplemented with respondent recommendations response rate, pre‐addressed and stamped envelopes and other additions, in a judgment sampling methodol- were provided, anonymity was assured, and a summary ogy. Before distributing the questionnaires, the young of the research findings as an incentive to the partici- researcher called each selected company to obtain the pants was offered. Several follow‐up procedures, such name of the chief sales executive, who was contacted as telephone calls and new mailings, were planned in and asked to participate in the study. The questionnaires order to receive as many responses as possible. BOX 13 13.11 NONRESPONSE AND NONRESPONSE ERROR A failure to obtain information from a number of subjects included in the sample (nonresponse) may lead to nonresponse error. Nonresponse error exists to the extent that those who did respond to your survey are different from those who did not on (one of the) characteristics of interest in your study. Two important sources of nonresponse are not‐at‐homes and refusals. An effective way to reduce the incidence of not‐ at‐homes is to call back at another time, preferably at a different time of day. The rate of refusals depends, among other things, on the length of the survey, the data collection method, and the patronage of the research. Hence, a decrease in survey length, in the data collection method (personal interviews instead of mail questionnaires), and the auspices of the research often improve the overall return rate. Personalized cover letters, a small incentive for participating in the study, and an advance notice that the survey is tak- ing place may also help you to increase the response rate. Nonetheless, it is almost impossible to entirely avoid nonresponse in surveys. In these cases you may have to turn to methods to deal with nonresponse error, such as generalizing the results to the respondents only or statistical adjustment (weighting the data by observable variables). PROBABILITY SAMPLING When elements in the population have a known, nonzero chance of being chosen as subjects in the sample, we resort to a probability sampling design. Probability sampling can be either unrestricted (simple random sampling) or restricted (complex probability sampling) in nature. Unrestricted or simple random sampling In the unrestricted probability sampling design, more commonly known as simple random sampling, every element in the population has a known and equal chance of being selected as a subject. Let us say there are 1000 elements in the population, and we need a sample of 100. Suppose we were to drop pieces of paper in a hat, each bearing the name of one of the elements, and draw 100 of those from the hat with our eyes closed. We know that chapter sampling 243 the first piece drawn will have a 1/1000 chance of being drawn, the next one a 1/999 chance of being drawn, and so on. In other words, we know that the probability of any one of them being chosen is 1 in the number of the population, and we also know that each single element in the hat has the same or equal probability of being chosen. We certainly know that computers can generate random numbers and one does not have to go through the tedious process of pulling out names from a hat! When we thus draw the elements from the population, it is most likely that the distribution patterns of the characteristics we are interested in investigating in the population are also likewise distributed in the subjects we draw for our sample. This sampling design, known as simple random sampling, has the least bias and offers the most generalizability. However, this sampling process could become cumbersome and expensive; in addition, an entirely updated listing of the population may not always be available. For these and other reasons, other prob- ability sampling designs are often chosen instead. Restricted or complex probability sampling As an alternative to the simple random sampling design, several complex probability sampling (restricted probability) designs can be used. These probability sampling procedures offer a viable, and sometimes more efficient, alternative to the unrestricted design we just discussed. Efficiency is improved in that more information can be obtained for a given sample size using some of the complex probability sampling procedures than the simple random sampling design. The five most common complex probability sampling designs – systematic sampling, stratified random sampling, cluster sampling, area sampling, and double sampling – will now be discussed. Systematic sampling The systematic sampling design involves drawing every nth element in the population starting with a randomly chosen element between 1 and n. The procedure is exemplified below. EXAMPLE If we wanted a sample of 35 households from a total materials, then the residents of corner houses may not population of 260 houses in a particular locality, then be exposed to as much noise as the houses that are in we could sample every seventh house starting from a between. Information on noise levels gathered from random number from 1 to 7. Let us say that the ran- corner house dwellers might therefore bias the research- dom number was 7, then houses numbered 7, 14, 21, 28, er's data. The likelihood of drawing incorrect con- and so on, would be sampled until the 35 houses were clusions from such data is thus high. In view of the selected. The one problem to be borne in mind in the scope for such systematic bias, the researcher must systematic sampling design is the probability of a sys- consider the plans carefully and make sure that the sys- tematic bias creeping into the sample. In the above tematic sampling design is appropriate for the study, example, for instance, let us say that every seventh before deciding on it. For market surveys, consumer house happened to be a corner house. If the focus of attitude surveys, and the like, the systematic sampling the research study conducted by the construction design is often used, and the telephone directory industry was to control “noise pollution” experienced frequently serves as the sampling frame for this by residents through the use of appropriate filtering sampling design. 244 research methods for business Stratified random sampling While sampling helps to estimate population parameters, there may be identifiable subgroups of elements within the population that may be expected to have different parameters on a variable of interest to the researcher. For example, to the human resources management director interested in assessing the extent of training that the employees in the system feel they need, the entire organization will form the population for study. But the extent, quality, and intensity of training desired by middle‐level managers, lower‐level managers, first‐line supervisors, computer analysts, clerical workers, and so on will be different for each group. Knowledge of the kinds of differ- ences in needs that exist for the different groups will help the director to develop useful and meaningful training programs for each group in the organization. Data will therefore have to be collected in a manner that will help the assessment of needs at each subgroup level in the population. The unit of analysis then will be at the group level and the stratified random sampling process will come in handy. Stratified random sampling, as its name implies, involves a process of stratification or segregation, followed by random selection of subjects from each stratum. The population is first divided into mutually exclusive groups that are relevant, appropriate, and meaningful in the context of the study. For instance, if the president of a com- pany is concerned about low motivational levels or high absenteeism rates among the employees, it makes sense to stratify the population of organizational members according to their job levels. When the data are collected and the analysis is done, we may find that, contrary to expectations, it is the middle‐level managers that are not motivated. This information will help the president to focus on action at the right level and devise better methods to motivate this group. Tracing the differences in the parameters of the subgroups within a population would not be possible without the stratified random sampling procedure. If either the simple random sampling or the sys- tematic sampling procedure were used in a case like this, then the high motivation at some job levels and the low motivation at other levels would cancel each other out, thus masking the real problems that exist at a particular level or levels. Stratification also helps when research questions such as the following are to be answered: 1. Are the machinists more accident prone than clerical workers? 2. Are Hispanics more loyal to the organization than Native Americans? Stratifying customers on the basis of life stages, income levels, and the like to study buying patterns and stratifying companies according to size, industry, profits, and so forth to study stock market reactions are com- mon examples of the use of stratification as a sampling design technique. Stratification is an efficient research sampling design; that is, it provides more information with a given sample size. Stratification should follow the lines appropriate to the research question. If we are studying con- sumer preferences for a product, stratification of the population could be by geographical area, market segment, consumers' age, consumers' gender, or various combinations of these. If an organization contemplates budget cuts, the effects of these cuts on employee attitudes can be studied with stratification by department, function, or region. Stratification ensures homogeneity within each stratum (i.e., very few differences or dispersions on the variable of interest within each stratum), but heterogeneity (variability) between strata. In other words, there will be more between‐group differences than within‐group differences. Proportionate and disproportionate stratified random sampling Once the population has been stratified in some meaningful way, a sample of members from each stratum can be drawn using either a simple random sampling or a systematic sampling procedure. The subjects drawn from each stratum can be either proportionate or disproportionate to the number of elements in the stratum. For instance, if an organization chapter sampling 245 TA B L E 1 3. 1 Proportionate and disproportionate stratified random sampling Number of subjects in the sample Proportionate sampling Job level Number of elements (20% of the elements) Disproportionate sampling Top management 10 2 7 Middle‐level management 30 6 15 Lower‐level management 50 10 20 Supervisors 100 20 30 Clerks 500 100 60 Secretaries 20 4 10 Total 710 142 142 employs 10 top managers, 30 middle managers, 50 lower‐level managers, 100 supervisors, 500 clerks, and 20 secretaries, and a stratified sample of about 140 people is needed for some specific survey, the researcher might decide to include in the sample 20% of members from each stratum. That is, members represented in the sam- ple from each stratum will be proportionate to the total number of elements in the respective strata. This would mean that two from the top, six from the middle, and ten from the lower levels of management would be included in the sample. In addition, 20 supervisors, 100 clerks, and four secretaries would be represented in the sample, as shown in the third column of Table 13.1. This type of sampling is called a proportionate stratified random sampling design. In situations like the one above, researchers might sometimes be concerned that information from only two members at the top and six from the middle levels would not truly reflect how all members at those levels would respond. Therefore, a researcher might decide, instead, to use a disproportionate stratified random sampling procedure. The number of subjects from each stratum would now be altered, while keeping the sample size unchanged. Such a sampling design is illustrated in the far right‐hand column in Table 13.1. The idea here is that the 60 clerks might be considered adequate to represent the population of 500 clerks; seven out of ten managers at the top level might also be considered representative of the top managers, and likewise 15 out of the 30 manag- ers at the middle level. This redistribution of the numbers in the strata might be considered more appropriate and representative for the study than the previous proportionate sampling design. Disproportionate sampling decisions are made either when some stratum or strata are too small or too large, or when there is more variability suspected within a particular stratum. As an example, the educational levels among supervisors, which may be considered to influence perceptions, may range from elementary school to master's degrees. Here, more people will be sampled at the supervisory level. Disproportionate sampling is also sometimes done when it is easier, simpler, and less expensive to collect data from one or more strata than from others. In summary, stratified random sampling involves stratifying the elements along meaningful levels and taking proportionate or disproportionate samples from the strata. This sampling design is more efficient than the simple random sampling design because, for the same sample size, each important segment of the popula- tion is better represented, and more valuable and differentiated information is obtained with respect to each group. 246 research methods for business Cluster sampling Cluster samples are samples gathered in groups or chunks of elements that, ideally, are natural aggregates of ele- ments in the population. In cluster sampling, the target population is first divided into clusters. Then, a random sample of clusters is drawn and for each selected cluster either all the elements or a sample of elements are included in the sample. Cluster samples offer more heterogeneity within groups and more homogeneity among groups – the reverse of what we find in stratified random sampling, where there is homogeneity within each group and heterogeneity across groups. A specific type of cluster sampling is area sampling. In this case, clusters consist of geographic areas such as counties, city blocks, or particular boundaries within a locality. If you wanted to survey the residents of a city, you would get a city map, take a sample of city blocks and select respondents within each city block. Sampling the needs of consumers before opening a 24‐hour convenience store in a particular part of town would involve area sampling. Location plans for retail stores, advertisements focused specifically on local populations, and TV and radio programs beamed at specific areas could all use an area sampling design to gather information on the inter- ests, attitudes, predispositions, and behaviors of the local area people. Area sampling is less expensive than most other probability sampling designs, and it is not dependent on a sampling frame. A city map showing the blocks of the city is adequate information to allow a researcher to take a sample of the blocks and obtain data from the residents therein. Indeed, the key motivation for cluster sampling is cost reduction. The unit costs of cluster sampling are much lower than those of other probability sampling designs of simple or stratified random sampling or systematic sampling. However, clus- ter sampling exposes itself to greater bias and is the least generalizable of all the probability sampling designs, because most naturally occurring clusters in the organizational context do not contain heterogeneous ele- ments. In other words, the conditions of intracluster heterogeneity and intercluster homogeneity are often not met. For these reasons, the cluster sampling technique is not very common in organizational research. Moreover, for marketing research activities, naturally occurring clusters, such as clusters of residents, buyers, students, or shops, do not have much heterogeneity among the elements. As stated earlier, there is more intra- cluster homogeneity than heterogeneity in such clusters. Hence, cluster sampling, though less costly, does not offer much efficiency in terms of precision or confidence in the results. However, cluster sampling offers con- venience. For example, it is easier to inspect an assortment of units packed inside, say, four boxes (i.e., all the elements in the four clusters) than to open 30 boxes in a shipment in order to inspect a few units from each at random. Single-stage and multistage cluster sampling We have thus far discussed single‐stage cluster sam- pling, which involves the division of the population into convenient clusters, randomly choosing the required number of clusters as sample subjects, and investigating all the elements in each of the randomly chosen clusters. Cluster sampling can also be done in several stages and is then known as multistage cluster sam- pling. For instance, if we were to do a national survey of the average monthly bank deposits, cluster sampling would first be used to select the urban, semi‐urban, and rural geographical locations for study. At the next stage, particular areas in each of these locations would be chosen. At the third stage, banks within each area would be chosen. In other words, multistage cluster sampling involves a probability sampling of the primary sampling units; from each of these primary units, a probability sample of the secondary sampling units is then drawn; a third level of probability sampling is done from each of these secondary units, and so on, until we have reached the final stage of breakdown for the sample units, when we sample every member in those units. chapter sampling 247 Double sampling This plan is resorted to when further information is needed from a subset of the group from which some infor- mation has already been collected for the same study. A sampling design where initially a sample is used in a study to collect some preliminary information of interest, and later a subsample of this primary sample is used to examine the matter in more detail, is called double sampling. For example, a structured interview might indicate that a subgroup of the respondents has more insight into the problems of the organization. These respondents might be interviewed again and asked additional questions. This research adopts a double sampling procedure. Review of probability sampling designs There are two basic probability sampling plans: the unrestricted or simple random sampling, and the restricted or complex probability sampling plans. In the simple random sampling design, every element in the population has a known and equal chance of being selected as a subject. The complex probability plan consists of five dif- ferent sampling designs. Of these five, the cluster sampling design is probably the least expensive as well as the least dependable, but is used when no list of the population elements is available. The stratified random sam- pling design is probably the most efficient, in the sense that for the same number of sample subjects, it offers precise and detailed information. The systematic sampling design has the built‐in hazard of possible systematic bias. Area sampling is a popular form of cluster sampling, and double sampling is resorted to when information in addition to that already obtained by using a primary sample has to be collected using a subgroup of the sample. NONPROBABILITY SAMPLING In nonprobability sampling designs, the elements in the population do not have any probabilities attached to their being chosen as sample subjects. This means that the findings from the study of the sample cannot be confidently generalized to the population. As stated earlier, however, researchers may, at times, be less concerned about gener- alizability than obtaining some preliminary information in a quick and inexpensive way. They might then resort to nonprobability sampling. Sometimes nonprobability sampling is the only way to obtain data, as discussed later. Some of the nonprobability sampling plans are more dependable than others and could offer some impor- tant leads to potentially useful information with regard to the population. Nonprobability sampling designs, which fit into the broad categories of convenience sampling and purposive sampling, are discussed next. Convenience sampling As its name implies, convenience sampling refers to the collection of information from members of the popula- tion who are conveniently available to provide it. One would expect the “Pepsi Challenge” contest to have been administered on a convenience sampling basis. Such a contest, with the purpose of determining whether people prefer one product to another, might be held at a shopping mall visited by many shoppers. Those inclined to take the test might form the sample for the study of how many people prefer Pepsi over Coke or product X to product Y. Such a sample is a convenience sample. Consider another example. A convenience sample of five officers who attended a competitor's showcase demonstration at the county fair the previous evening offered the vice president of the company information on the “new” products of the competitor and their pricing strategies, which helped the VP to formulate some ideas on the next steps to be taken by the company. Convenience sampling is most often used during the exploratory phase of a research project and is perhaps the best way of getting some basic information quickly and efficiently. 248 research methods for business Purposive sampling Instead of obtaining information from those who are most readily or conveniently available, it might some- times become necessary to obtain information from specific target groups. The sampling here is confined to specific types of people who can provide the desired information, either because they are the only ones who have it, or they conform to some criteria set by the researcher. This type of sampling design is called purposive sampling, and the two major types of purposive sampling – judgment sampling and quota sampling – will now be explained. Judgment sampling Judgment sampling involves the choice of subjects who are most advantageously placed or in the best position to provide the information required. For instance, if a researcher wants to find out what it takes for women managers to make it to the top, the only people who can give first‐hand information are the women who have risen to the positions of presidents, vice presidents, and important top‐level executives in work organizations. They could reasonably be expected to have expert knowledge by virtue of having gone through the experiences and processes themselves, and might perhaps be able to provide good data or information to the researcher. Thus, the judgment sampling design is used when a limited number or category of people have the informa- tion that is sought. In such cases, any type of probability sampling across a cross‐section of the entire population is purposeless and not useful. Judgment sampling may curtail the generalizability of the findings, due to the fact that we are using a sample of experts who are conveniently available to us. However, it is the only viable sampling method for obtaining the type of information that is required from very specific pockets of people who alone possess the needed facts and can give the information sought. In organizational settings, and particularly for market research, opinion leaders who are very knowledgeable are included in the sample. Enlightened opinions, views, and knowledge constitute a rich data source. Judgment sampling calls for special efforts to locate and gain access to the individuals who do have the req- uisite information. As already stated, this sampling design may be the only useful one for answering certain types of research question. Quota sampling Quota sampling, a second type of purposive sampling, ensures that certain groups are adequately represented in the study through the assignment of a quota. Generally, the quota fixed for each subgroup is based on the total numbers of each group in the population. However, since this is a nonprobability sampling plan, the results are not generalizable to the population. Quota sampling can be considered a form of proportionate stratified sampling, in which a predetermined proportion of people are sampled from different groups, but on a convenience basis. For instance, it may be surmised that the work attitude of blue‐collar workers in an organization is quite different from that of white‐ collar workers. If there are 60% blue‐collar workers and 40% white‐collar workers in this organization, and if a total of 30 people are to be interviewed to find the answer to the research question, then a quota of 18 blue‐ collar workers and 12 white‐collar workers will form the sample, because these numbers represent 60% and 40% of the sample size. The first 18 conveniently available blue‐collar workers and 12 white‐collar workers will be sampled according to this quota. Needless to say, the sample may not be totally representative of the popu- lation; hence the generalizability of the findings will be restricted. However, the convenience it offers in terms of effort, cost, and time makes quota sampling attractive for some research efforts. Quota sampling also becomes a necessity when a subset of the population is underrepresented in the organization – for example, minority groups, foremen, and so on. In other words, quota sampling ensures that all the subgroups in the chapter sampling 249 population are adequately represented in the sample. Quota samples are basically stratified samples from which subjects are selected nonrandomly. In a workplace (and society) that is becoming increasingly heterogeneous because of the changing demo- graphics, quota sampling can be expected to be used more frequently in the future. For example, quota sampling can be used to gain some idea of the buying predispositions of various ethnic groups, to get a feel of how employees from different nationalities perceive the organizational culture, and so on. Although quota sampling is not generalizable like stratified random sampling, it does offer some informa- tion, based on which further investigation, if necessary, can proceed. That is, it is possible that the first stage of research will use the nonprobability design of quota sampling, and once some useful information has been obtained, a probability design will follow. The converse is also entirely possible. A probability sampling design might indicate new areas for research, and nonprobability sampling designs might be used to explore their feasibility. Review of nonprobability sampling designs There are two main types of nonprobability sampling design: convenience sampling and purposive sam- pling. Convenience sampling is the least reliable of all sampling designs in terms of generalizability, but sometimes it may be the only viable alternative when quick and timely information is needed, or for exploratory research purposes. Purposive sampling plans fall into two categories: judgment and quota sam- pling designs. Judgment sampling, though restricted in generalizability, may sometimes be the best sam- pling design choice, especially when there is a limited population that can supply the information needed. Quota sampling is often used on considerations of cost and time and the need to adequately represent minority elements in the population. Although the generalizability of all nonprobability sampling designs is very restricted, they have certain advantages and are sometimes the only viable alternative for the researcher. Table 13.2 summarizes the probability and nonprobability sampling designs discussed thus far, and their advantages and disadvantages. Figure 13.4 offers some decision choice points as to which design might be useful for specific research goals. TA B L E 1 3. 2 Probability and nonprobability sampling designs Sampling design Description Advantages Disadvantages Probability sampling 1. Simple random All elements in the population High generalizability of Not as efficient as sampling are considered and each findings. stratified sampling. element has an equal chance of being chosen as the subject. 2. Systematic Every nth element in the Easy to use if sampling Systematic biases are sampling population is chosen starting frame is available. possible. from a random point in the sampling frame. 250 research methods for business Sampling design Description Advantages Disadvantages 3. Stratified random Population is first divided into Most efficient among all Stratification must be sampling (Str.R.S.) meaningful segments; probability designs. meaningful. More Proportionate thereafter subjects are All groups are adequately time consuming Str.R.S. drawn in proportion to sampled and than simple Disproportionate their original numbers in comparisons among random sampling Str.R.S. the population. groups are possible. or systematic Based on criteria other than sampling. their original population Sampling frame for numbers. each stratum is essential. 4. Cluster sampling Groups that have heterogeneous In geographic clusters, The least reliable and members are first identified; costs of data collection efficient among all then some are chosen at are low. probability random; all the members in sampling designs each of the randomly since subsets of chosen groups are studied. clusters are more homogeneous than heterogeneous. 5. Area sampling Cluster sampling within a Cost‐effective. Useful for Takes time to collect particular area or locality. decisions relating to a data from an area. particular location. 6. Double sampling The same sample or a subset of Offers more detailed Original biases, if any, the sample is studied twice. information on the will be carried over. topic of study. Individuals may not be happy responding a second time. Nonprobability sampling 7. Convenience The most easily accessible Quick, convenient, less Not generalizable sampling members are chosen as expensive. at all. subjects. 8. Judgment sampling Subjects selected on the basis of Sometimes, the only Generalizability is their expertise in the subject meaningful way to questionable; investigated. investigate. not generalizable to entire population. 9. Quota sampling Subjects are conveniently Very useful where Not easily chosen from targeted minority participation generalizable. groups according to some in a study is critical. predetermined number or quota. chapter sampling 251 Is REPRESENTATIVENESS of sample critical for the study? Yes No Choose one of Choose one of the PROBABILITY the NONPROBABILITY sampling designs. sampling designs. If purpose of If purpose of study mainly is for: study mainly is: Generalizability. Assessing Collecting Gathering more To obtain quick, To obtain informa- differential information information from even if unreliable, tion relevant to and parameters in in a localized a subset of information. available only with subgroups of area. the sample. certain groups. population. Choose Choose Choose Choose area Choose double Choose simple systematic cluster sampling. sampling. convenience random sampling. sampling sampling. sampling. if not enough $. All subgroups Looking for Need responses have equal number information that of special interest of elements? only a few "experts" minority groups? can provide? Choose Choose Yes No judgment quota sampling. sampling. Choose Choose proportionate disproportionate stratified random stratified random sampling. sampling. FIGURE 13.4 Choice points in sampling design 252 research methods for business INTERMEZZO: EXAMPLES OF WHEN CERTAIN SAMPLING DESIGNS WOULD BE APPROPRIATE Simple random sampling This sampling design is best when the generalizability of the findings to the whole population is the main objective of the study. Consider the following two examples. EXAMPLE The human resources director of a company with 82 its four geographical regions of operation, wants to people on its payroll has been asked by the vice presi- know what types of sales gimmicks worked best for dent to consider formulating an implementable flex- the company overall during the past year. This is to time policy. The director feels that such a policy is not help formulate some general policies for the company necessary since everyone seems happy with the 9‐to‐ as a whole and prioritize sales promotion strategies 5 hours, and no one has complained. Formulating such for the coming year. Instead of studying each of the a policy now, in the opinion of the director, runs the 80 stores, some dependable (i.e., representative and risk of creating domestic problems for the staff and generalizable) information can be had, based on the scheduling problems for the company. She wants, how- study of a few stores drawn through a simple random ever, to resort to a simple random sampling procedure sampling procedure. That is, each one of the 80 stores to do an initial survey, and, with the results, convince would have an equal chance of being included in the the VP that there is no need for flextime, and urge him sample, and the results of the study would be the to drop the matter. Since simple random sampling most generalizable. A simple random sampling offers the greatest generalizability of the results to the procedure is recommended in this case since the pol- entire population, and the VP needs to be convinced, it icy is to be formulated for the company as a whole. is important to resort to this sampling design. This implies that the most representative information has to be obtained that can be generalized to the The regional director of sales operations of a medium‐ entire company. This is best accomplished through sized company, which has 20 retail stores in each of this design. It has to be noted that in some cases, where cost is a primary consideration (i.e., resources are limited), and the number of elements in the population is very large and/or geographically dispersed, the simple random sampling design may not be the most desirable, because it could become quite expensive. Thus, both the criticality of generalizability and considerations of cost come into play in the choice of this sam- pling design. Stratified random sampling This sampling design, which is the most efficient, is a good choice when differentiated information is needed regarding various strata within the population, which are known to differ in their parameters. See the examples on the following page. chapter sampling 253 EXAMPLE The director of human resources of a manufacturing (according to regional preferences) could be devel- firm wants to offer stress management seminars to the oped, then the 80 stores would first be stratified on the personnel who experience high levels of stress. He con- basis of the geographical region, and then a represent- jectures that three groups are most prone to stress: the ative sample of stores would be drawn from each of the workmen who constantly handle dangerous chemicals, geographical regions (strata) through a simple random the foremen who are held responsible for production sampling procedure. In this case, since each of the quotas, and the counselors who, day in and day out, lis- regions has 20 stores, a proportionate stratified ran- ten to the problems of the employees, internalize them, dom sampling process (say, five stores from each and offer them counsel, with no idea of how much they region) would be appropriate. If, however, the north- have really helped the clients. To get a feel for the expe- ern region had only three stores, the southern had 15, rienced level of stress within each of the three groups and the eastern and western regions had 24 and 38 and the rest of the firm, the director might stratify the stores, respectively, then a disproportionate stratified sample into four distinct categories: (1) the workmen random sampling procedure would be the right choice, handling the dangerous chemicals, (2) the foremen, (3) with all three stores in the northern region being stud- the counselors, and (4) all the rest. He might then ied, because of the small number of elements in that choose a disproportionate random sampling procedure population. If the sample size was retained at 20, then (since group (3) can be expected to be very small, and the north, south, east, and west regions would proba- groups (2) and (1) are much smaller than group (4)). bly have samples respectively of three, four, five and This is the only sampling design that would allow eight. It is interesting to note that sometimes when the designing of stress management seminars in a stratified random sampling might seem logical, it meaningful way, targeted at the right groups. might not really be necessary. For example, when test‐ marketing results show that Cubans, Puerto Ricans, If, in the earlier example, the regional director had and Mexicans perceive and consume a particular wanted to know which sales promotion gimmick product the same way, there is no need to segment the offered the best results for each of the geographical market and study each of the three groups using a areas, so that different sales promotion strategies stratified sampling procedure. Systematic sampling If the sampling frame is large, and a listing of the elements is conveniently available in one place (as in the telephone directory, company payroll, chamber of commerce listings, etc.), then a systematic sampling procedure will offer the advantages of ease and quickness in developing the sample, as illustrated by the following example. EXAMPLE An administrator wants to assess the reactions of scheme by using a systematic sampling design. The employees to a new and improved health benefits company's records will provide the sampling frame, scheme that requires a modest increase in the premi- and every nth employee can be sampled. A stratified ums to be paid by the employees for their families. The plan is not called for here since the policy is for the administrator can assess the enthusiasm for the new entire company. 254 research methods for business BOX 13.2 13 2 NOTE Systematic sampling is inadvisable where systematic bias can be anticipated to be present. For example, systematic sampling from the personnel directory of a company (especially when it has an equal number of employees in each department), which lists the names of the individuals department‐wise, with the head of the department listed first, and the secretary listed next, has inherent bias. The possibility of systematic bias creeping into the data cannot be ruled out in this case, since the selection process may end up pick- ing each of the heads of the department or the departmental secretaries as the sample subjects. The results from such a sample will clearly be biased and not generalizable, despite the use of a probability sampling procedure. Systematic sampling will have to be scrupulously avoided in cases where known systematic biases are possible. Cluster sampling This sampling design is most useful when a heterogeneous group is to be studied at one time. Two examples are offered below. EXAMPLE A human resources director is interested in knowing heterogeneous group of individuals (i.e., from various why staff resign. Cluster sampling will be useful in this departments), and the study can be conducted at case for conducting exit interviews of all members a low cost. completing their final papers in the human resources department on the same day (cluster), before resign- A financial analyst wishes to study the lending prac- ing. The clusters chosen for interview will be based on tices of banks in the Netherlands. All the banks in each a simple random sampling of the various clusters of city will form a cluster. By randomly sampling the clus- personnel resigning on different days. The interviews ters, the analyst will be able to draw conclusions on the will help to understand the reasons for turnover of a lending practices. Area sampling Area sampling is best suited when the goal of the research is confined to a particular locality or area, as per the example below. EXAMPLE A telephone company wants to install a public tele- the crime statistics and interviewing the residents in a phone outlet in a locality where crime is most rampant, particular area will help to choose the right location so that victims can have access to a telephone. Studying for installation of the phone. chapter sampling 255 Double sampling This design provides added information at minimal additional expenditure. See the example below. EXAMPLE In the previous exit interview example, some individu- philosophical differences, and why these particular als (i.e., a subset of the original cluster sample) might issues were central to the individuals' value systems. have indicated that they were resigning because of Such additional detailed information from the target philosophical differences with the company's policies. group through the double sampling design could help The researcher might want to do an in‐depth interview the company to look for ways of retaining employees with these individuals to obtain further information in the future. regarding the nature of the policies disliked, the actual Convenience sampling This nonprobability design, which is not generalizable at all, is used at times to obtain some “quick” information to get a “feel” for the phenomenon or variables of interest. See the example below. EXAMPLE The accounts executive has established a new account- new system without making it seem that he has doubts ing system that maximally utilizes computer technol- about its acceptability. He may then “casually” talk to ogy. Before making further changes, he would like to the first five accounting personnel that walk into his get a feel for how the accounting clerks react to the office, trying to gauge their reactions. BOX 13.3 13 3 NOTE Convenience sampling should be resorted to in the interests of expediency, with the full knowledge that the results are not generalizable at all. Judgment sampling: one type of purposive sampling A judgment sampling design is used where the collection of “specialized informed inputs” on the topic area researched is vital, and the use of any other sampling design would not offer opportunities to obtain the special- ized information, as per the example that follows. EXAMPLE A pharmaceutical company wants to trace the effects with a group of voluntarily consenting patients, tests of a new drug on patients with specific health problems the drug. This is a judgment sample because data are (muscular dystrophy, sickle cell anemia, rheumatoid collected from appropriate special groups. arthritis, etc.). It then contacts such individuals and, 256 research methods for business Quota sampling: a second type of purposive sampling This sampling design allows for the inclusion of all groups in the system researched. Thus, groups who are small in number are not neglected, as per the example below. EXAMPLE A company is considering operating an on‐site kinder- of the home, (3) single parents with kindergarten‐ garten facility. But before taking further steps, it wants age children, and (4) all those without children of to get the reactions of four groups to the idea: (1) kindergarten age. If the four groups are expected to employees who are parents of kindergarten‐age chil- represent 60%, 7%, 23%, and 10%, respectively, in the dren, and where both are working outside of the home, population of 420 employees in the company, then a (2) employees who are parents of kindergarten‐age quota sampling will be appropriate to represent the children, but where one of them is not working outside four groups. BOX 13.4 13 4 NOTE The last group in the above example should also be included in the sample since there is a possibility that they may perceive this as a facility that favors only the parents of kindergarten children, and therefore resent the idea. It is easy to see that resorting to quota sampling would be important in a case such as this. In effect, as can be seen from the discussions on sampling designs thus far, decisions on which design to use depend on many factors, including the following: 1. Extent of prior knowledge in the area of research undertaken. 2. The main objective of the study – generalizability, efficiency, knowing more about subgroups within a population, obtaining some quick (even if unreliable) information, etc. 3. Cost considerations – is exactitude and generalizability worth the extra investment of time, cost, and other resources in resorting to a more, rather than less, sophisticated sampling design? Even if it is, is suboptimization because of cost or time constraints called for? (See also Figure 13.4.) The advantages and disadvantages of the different probability and nonprobability sampling designs are listed in Table 13.2. In sum, choosing the appropriate sampling plan is one of the more important research design decisions the researcher has to make. The choice of a specific design will depend broadly on the goal of research, the charac- teristics of the population, and considerations of cost. chapter sampling 257 ISSUES OF PRECISION AND CONFIDENCE IN DETERMINING SAMPLE SIZE Having discussed the various probability and nonprobability sampling designs, we now need to focus attention on the second aspect of the sampling design issue – the sample size. Suppose we select 30 people from a popula- tion of 3000 through a simple random sampling procedure. Will we be able to generalize our findings to the population with confidence, since we have chosen a probability design that has the most generalizability? What is the sample size required to make reasonably precise generalizations with confidence? What do precision and confidence mean? These issues will be considered now. A reliable and valid sample should enable us to generalize the findings from the sample to the population under investigation. In other words, the sample statistics should be reliable estimates and reflect the population parameters as closely as possible within a narrow margin of error. No sample statistic (X, for instance) is going to be exactly the same as the population parameter ( μ), no matter how sophisticated the probability sampling design is. Remember that the very reason for a probability design is to increase the probability that the sample statistics will be as close as possible to the population parameters. Though the point estimate X may not accurately reflect the population mean, μ, an interval estimate can be made within which μ will lie, with probabilities attached – that is, at particular confidence levels. The issues of confidence interval and confidence level are addressed in the following discussions on precision and confidence. Precision Precision refers to how close our estimate is to the true population characteristic. Usually, we estimate the popu- lation parameter to fall within a range, based on the sample estimate. For example, let us say that from a study of a simple random sample of 50 of the total 300 employees in a workshop, we find that the average daily production rate per person is 50 pieces of a particular product ( X 50). We might then (by doing certain calculations, as we shall see later) be able to say that the true average daily production of the product (μ) lies anywhere between 40 and 60 for the population of employees in the workshop. In saying this, we offer an interval estimate, within which we expect the true population mean production to be ( 50 10). The narrower this interval, the greater the precision. For instance, if we are able to estimate that the population mean will fall anywhere between 45 and 55 pieces of production ( 50 5) rather than 40 and 60 ( 50 10), then we have more precision. That is, we now estimate the mean to lie within a narrower range, which in turn means that we estimate with greater exacti- tude or precision. Precision is a function of the range of variability in the sampling distribution of the sample mean. That is, if we take a number of different samples from a population, and take the mean of each of these, we will usually find that they are all different, are normally distributed, and have a dispersion associated with them. The smaller this dispersion or variability, the greater the probability that the sample mean will be closer to the population mean. We need not necessarily take several different samples to estimate this variability. Even if we take only one sample of 30 subjects from the population, we will still be able to estimate the variability of the sampling distribution of the sample mean. This variability is called the standard error, denoted by SX. The standard error is calculated by the following formula: S SX n where S is the standard deviation of the sample, n is the sample size, and SX indicates the standard error or the extent of precision offered by the sample. Note that the standard error varies inversely with the square root of the sample size. Hence, if we want to reduce the standard error given a particular standard deviation in the sample, we need to increase the sample size. 258 research methods for business Another noteworthy point is that the smaller the variation in the population, the smaller the standard error, which in turn implies that the sample size need not be large. Thus, low variability in the population requires a smaller sample size. In sum, the closer we want our sample results to reflect the population characteristics, the greater the preci- sion we should aim at. The greater the precision required, the larger the sample size needed, especially when the variability in the population itself is large. Confidence Whereas precision denotes how close we estimate the population parameter based on the sample statistic, confi- dence denotes how certain we are that our estimates will really hold true for the population. In the previous example of production rate, we know we are more precise when we estimate the true mean production (μ) to fall somewhere between 45 and 55 pieces than somewhere between 40 and 60. However, we may have more confi- dence in the latter estimation than in the former. After all, anyone can say with 100% certainty or confidence that the mean production (μ) will fall anywhere between zero and infinity! Other things being equal, the narrower the range, the lower the confidence. In other words, there is a trade‐off between precision and confidence for any given sample size, as we shall see later in this chapter. In essence, confidence reflects the level of certainty with which we can state that our estimates of the popula- tion parameters, based on our sample statistics, will hold true. The level of confidence can range from 0 to 100%. A 95% confidence is the conventionally accepted level for most business research, most commonly expressed by denoting the significance level as p ≤ 0.05. In other words, we say that at least 95 times out of 100 our estimate will reflect the true population characteristic. Sample Data, Precision, and Confidence in Estimation Precision and confidence are important issues in sampling because when we use sample data to draw inferences about the population, we hope to be fairly “on target,” and have some idea of the extent of possible error. Because a point estimate provides no measure of possible error, we do an interval estimation to ensure a relatively accurate estimation of the population parameter. Statistics that have the same distribution as the sampling distribution of the mean are used in this procedure, usually a z or a t statistic. For example, we may want to estimate the mean dollar value of purchases made by customers when they shop at department stores. From a sample of 64 customers sampled through a systematic sampling design proce- dure, we may find that the sample mean X 105, and the sample standard deviation S 10. X , the sample mean, is a point estimate of μ, the population mean. We could construct a confidence interval around X to estimate the range within which μ will fall. The standard error SX and the percentage or level of confidence we require will determine the width of the interval, which can be represented by the following formula, where K is the t statistic for the level of confidence desired. X KS We already know that: S SX n Here, 10 SX 1.25 64 chapter sampling 259 From the table of critical values for t in any statistics book (see Table II, columns 5, 6, and 8, in the statistical tables given toward the end of this book), we know that: For a 90% confidence level, the K value is 1.645. For a 95% confidence level, the K value is 1.96. For a 99% confidence level, the K value is 2.576. If we desire a 90% confidence level in the above case, then 105 1.645 (1.25) (i.e., 105 2.056). μ thus falls between 102.944 and 107.056. These results indicate that using a sample size of 64, we could state with 90% confidence that the true population mean value of purchases for all customers would fall between $102.94 and $107.06. If we now want to be 99% confident of our results without increasing the sample size, we necessarily have to sacrifice precision, as may be seen from the following calculation: 105 2.576 (1.25). The value of μ now falls between 101.78 and 108.22. In other words, the width of the interval has increased and we are now less precise in estimating the population mean, though we are a lot more confident about our estimation. It is not difficult to see that if we want to maintain our original precision while increasing the confidence, or maintain the confidence level while increasing precision, or we want to increase both the confidence and the precision, we need a larger sample size. In sum, the sample size, n, is a function of: 1. the variability in the population; 2. precision or accuracy needed; 3. confidence level desired; and 4. type of sampling plan used – for example, simple random sampling versus stratified random sampling. Trade-Off Between Confidence and Precision We have noted that if we want more precision, or more confidence, or both, the sample size needs to be increased – unless, of course, there is very little variability in the population itself. However, if the sample size (n) cannot be increased, for whatever reason – say, we cannot afford the costs of increased sampling – then, with the same n, the only way to maintain the same level of precision is to forsake the confidence with which we can predict our estimates. That is, we reduce the confidence level or the certainty of our estimate. This trade‐off between preci- sion and confidence is illustrated in Figures 13.5 (a) and (b). Figure 13.5 (a) indicates that 50% of the time the true mean will fall within the narrow range indicated in the figure, the 0.25 in each tail representing the 25% nonconfidence, or the probability of making errors, in our estimation on either side. Figure 13.5 (b) indicates that.50.99.25.25.005.005 X X (a) (b) FIGURE 13.5 Illustration of the trade-off between precision and confidence. (a) More precision but less confidence; (b) more confidence but less precision. 260 research methods for business 99% of the time we expect the true mean μ to fall within the much wider range indicated in the figure and there is only a 0.005% chance that we are making an error in this estimation. That is, in Figure 13.5 (a), we have more precision but less confidence (our confidence level is only 50%). In Figure 13.5 (b), we have high confidence (99%), but then we are far from being precise – that is, our estimate falls within a broad interval range. It thus becomes necessary for researchers to consider at least four aspects while making decisions on the sample size needed to do the research: 1. How much precision is really needed in estimating the population characteristics of interest – that is, what is the margin of allowable error? 2. How much confidence is really needed – that is, how much chance can we take of making errors in estimating the population parameters? 3. To what extent is there variability in the population on the characteristics investigated? 4. What is the cost–benefit analysis of increasing the sample size? SAMPLE DATA AND HYPOTHESIS TESTING So far we have discussed sample data as a means of estimating the population parameters, but sample data can also be used to test hypotheses about population values rather than simply to estimate population values. The procedure for this testing incorporates the same information as in interval estimation, but the goals behind the two methods are somewhat different. Referring to the earlier example of the average dollar value purchases of customers in a department store, instead of trying to estimate the average purchase value of the store's customers with a certain degree of accuracy, let us say that we now wish to determine whether or not customers expend the same average amount in pur- chases in Department Store A as in Department Store B. From Chapter 5, we know that we should first set the null hypothesis, which will state that there is no difference in the dollar values expended by customers shopping at the two different stores. This is expressed as: H0 : A B 0 The alternate hypothesis of differences will be stated nondirectionally (since we have no idea whether customers buy more at Store A or Store B) as: HA : A B 0 If we take a sample of 20 customers from each of the two stores and find that the mean dollar value purchases of customers in Store A is 105 with a standard deviation of 10, and the corresponding figures for Store B are 100 and 15, respectively, we see that: XA XB 105 100 5 whereas our null hypothesis had postulated no difference (difference = 0). Should we then conclude that our alternate hypothesis is to be accepted? We cannot say! To determine this we must first find the probability or likelihood of the two group means having a difference of 5 in the context of the null hypothesis or a difference of 0. This can be done by converting the difference in the sample means to a t statistic and seeing what the probability chapter sampling 261 is of finding a t of that value. The t distribution has known probabilities attached to it (see Table II (t distribution) in the statistical tables given toward the end of the book). Looking at the t distribution table, we find that, with two samples of 20 each (the degrees of freedom become n1 n2 2 38), for the t value to be significant at the 0.05 level, the critical value should be around 2.021 (see t distribution table column 6 against v40). We need to use the two‐tailed test since we do not know whether the difference between Store A and Store B will be positive or negative. For even a 90% probability, it should be at least 1.684 (see the number to the left of 2.021). The t statistic can be calculated for testing our hypothesis as follows: ( X1 X2 ) ( 1 2 ) t SX1 X2 n1 s12 n2 s22 1 1 SX1 X2 (n1 n2 2) n1 n2 (20 102 ) (20 152 ) 1 1 20 20 2 20 20 (XA XB ) ( A B ) t 4.136 We already know that XA XB 5 (the difference in the means of the two stores) and A B 0 (from our null hypothesis) Then 5 0 t 1.209 4.136 This t value of 1.209 is way below the value of 2.021 (for 40 degrees of freedom for a two‐population t‐test, the closest to the actual 38 degrees of freedom [(20 20) 2]) required for the conventional 95% probability, and even for the 90% probability, which requires a value of 1.684. We can thus say that the difference of 5 that we found between the two stores is not significantly different from 0. The conclusion, then, is that there is no signifi- cant difference between how much customers buy (dollars expended) at Department Store A and Department Store B. We will thus accept the null hypothesis and reject the alternative. Sample data can thus be used not only for estimating the population parameters, but also for testing hypoth- eses about population values, population correlations, and so forth, as we will see more fully in Chapter 15. THE SAMPLE SIZE Both sampling design and the sample size are important to establish the representativeness of the sample for generalizability. If the appropriate sampling design is not used, a large sample size will not, in itself, allow the findings to be generalized to the population. Likewise, unless the sample size is adequate for the desired level of 262 research methods for business precision and confidence, no sampling design, however sophisticated, will be useful to the researcher in meeting the objectives of the study. Hence, sampling decisions should consider both the sampling design and the sample size. Determining the Sample Size Now that we are aware of the fact that the sample size is governed by the extent of precision and confidence desired, how do we determine the sample size required for our research? The procedure can be illustrated through an example. EXAMPLE Suppose a manager wants to be 95% confident that the of ±$500 will have to encompass a dispersion of expected monthly withdrawals in a bank will be within (1.96 × standard error). That is, a confidence interval of $500. Let us say that a study of a sample of clients indicates that the average with- 500 1.96 SX X drawals made by them have a standard deviation of $3500. What would be the sample size needed in We already know that: this case? We noted earlier that the population mean can be S SX estimated by using the formula: n 3500 X KSX 255.10 n Since the confidence level needed here is 95%, the n 188 applicable K value is 1.96 (t table). The interval estimate The sample size indicated above is 188. However, let us say that this bank has a total clientele of only 185. This means we cannot sample 188 clients. We can, in this case, apply a correction formula and see what sample size would be needed to have the same level of precision and confidence given the fact that we have a total of only 185 clients. The correction formula is as follows: S N n SX n N 1 where N is the total number of elements in the population, n is the sample size to be estimated, SX is the standard error of the estimate of the mean, and S is the standard deviation of the sample mean. Applying the correction formula, we find that: 3500 185 n 255.10 n 184 n 94 We would now sample 94 of the total 185 clients. chapter sampling 263 To understand the impact of precision and/or confidence on the sample size, let us try changing the confi- dence level required in the bank withdrawal example, which needed a sample size of 188 for a confidence level of 95%. Let us say that the bank manager now wants to be 99% sure that the expected monthly withdrawals will be within the interval of $500. What will be the sample size now needed? SX will now be: 500 194.099 2.576 3500 194.099 n n 325 The sample has now to be increased 1.73 times (from 188 to 325) to increase the confidence level from 95% to 99%! Try calculating the sample size if the precision has to be narrowed down from $500 to $300 for a 95% and a 99% confidence level! Your answers should show the sample sizes needed as 523 and 902, respectively. These results dramatically highlight the costs of increased precision, confidence, or both. It is hence a good idea to think through how much precision and confidence one really needs, before determining the sample size for the research project. So far we have discussed sample size in the context of precision and confidence with respect to one variable only. However, in research, the theoretical framework has several variables of inte