Task 2 - Boxed-in: Essentials (Strange Fruit) PDF
Document Details
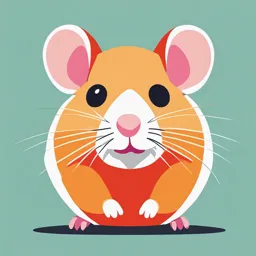
Uploaded by VerifiableMolybdenum2293
Maastricht University
Tags
Summary
This document discusses different approaches and theories of categorization, such as classical, prototype, and exemplar-based theories. It outlines the learning goals and introduces the concept of categorization, touching on natural, artifact, and nominal categories.
Full Transcript
Task 2 - Boxed-in: Essentials (Strange Fruit) Learning goals What is categorization? What are different approaches/theories of categorization? What are their strengths and weaknesses? Why do we categorize? Concept formation and categorization (Gobet chapter) Introduction Concept - the b...
Task 2 - Boxed-in: Essentials (Strange Fruit) Learning goals What is categorization? What are different approaches/theories of categorization? What are their strengths and weaknesses? Why do we categorize? Concept formation and categorization (Gobet chapter) Introduction Concept - the building block of semantic knowledge (e.g. 'dog'). Knowledge of concepts is essential and enables us to interact with the environment: by categorizing organisms and objects, we can be aware of their properties even though they may not be clearly visible at the moment, we can make inferences about them, and we can predict their behavior. Category - a class of concepts that share common properties (e.g. 'animal' = all organisms capable of voluntary movement). Natural category - a group of entities that exist in the natural world. Artefact category - a group of man-made objects that are designed with a specific goal or function in mind (e.g. 'computer'). Nominal category - a group of objects or ideas that are put together based on an arbitrary characteristic (e.g. 'positive numbers' or 'things that can be found in someone's office'). Classical theory of concepts Definition - a rule containing a set of defining features that are together individually necessary and jointly sufficient for category membership. Individually necessary - all members of the category have each of these features. Jointly sufficient - if all of these features are present in a given instance, it is a member of the category. Example: a bachelor is defined as an adult male who is not married. In the classical theory of categorization, categories are definitions. Category membership is binary: an object is either a member or not a member of a category. Through its definition, a concept will select all members of the category and only its members. Classification is not affected by context, the only thing that matters is the set of rules in the definition. Concepts can be organized using a hierarchy of inclusion relations (e.g. in biological taxonomy). Empirical data supporting classical theory Study: geometrical shapes of various colors, size & number are used to study how people attain concepts. Simple concepts - defined by a simple attribute (e.g. 'objects have to be triangles to belong to category X') Conjunctive concepts - defined by several attributes that have to be present together (e.g. 'objects have to be square and black to belong to category X') Disjunctive concepts - require that at least 1 attribute is present (e.g. 'objects have to be square or black to belong to category X'). Disjunctive concepts, which were the rarest in real life, were the hardest to learn. People found it difficult to identify the categories when information about previous trials had to be kept in short -term memory. Limitations in attention and short-term memory led to the development of 3 main strategies: ○ Successive scanning - keeping a single hypothesis in mind (e.g. 'square and blue') and limiting attention to cases that offer a direct test of the hypothesis. ○ Conservative focusing - the first positive example is taken as the hypothesis, and then only one feature is changed when positive instances do not match the hypothesis (does not impose much load on short -term memory, only the last hypothesis needs to be memorized). ○ Focus gambling - a similar strategy to conservative focusing, except that more features can be changed. Problems with classical theory It is not clear how the sometimes incorrect information we receive from the environment could lead to clear -cut concepts and perfect hierarchies of concepts. It is impossible to identify the necessary & sufficient conditions for many, if not most concepts. Different members of a category share family resemblances: members of a category resemble each other in different ways, and the Complex Cognition Page 1 Different members of a category share family resemblances: members of a category resemble each other in different ways, and the features that mostly determine resemblance for a pair of members may not be the same as features that characterize resemblanc e for another pair. Study: participants are asked to list the attributes of members of categories (e.g. fruit, vegetables and vehicles). They pro duced non-necessary attributes and their responses tended to have the structure of family resemblance. Follow-up study: the phrase 'necessary and sufficient properties' was explained to participants. Then they were asked to provide necessary and sufficient properties for deciding whether objects were members of a given category. In spite of these specific instructions, responses again corresponded to family -resemblance structures. People disagree when categorizing objects and classification is not stable over time. The strict hierarchy predicted by classical theory is not always true. Study: participants agree that car seats were chairs and chairs are furniture, but they disagree that car seats are furniture. In summary, while classical theories can explain data from concept attainment studies with artificial material, natural categ ories rarely satisfy the predictions of classical theory. This led research away from experiments with artificial categories and spurred the development of prototype theories. Prototype theories Prototype - the most typical member of a category (e.g. German shepherd for the category of dogs) or a set of such members of the category (e.g. golden retriever, German shepherd and collie). During classification, the features of the concept are matched against the properties of the object being classified, and an overall measure of similarity is obtained. Every matching feature increases similarity, and every mismatching feature decreases similarity. If the object and the concep t are similar enough - then the object is classified as a member. Prototypes are represented as a set of characteristic features - features that best describe the prototype, but are not necessary. Characteristic features are not necessary as in classical theory (they just correlate with category membership). They are fou nd by averaging the description of all the members of a given category that have been seen, and taking the features that recur most often (e.g. a characteristic feature of the concept of 'bird' is the ability to fly, but there are birds that do not fly - e.g. ostriches and penguins). Evidence supporting prototype theory People tend to list characteristic features of categories, and not defining features. Concepts have a typicality gradient (some members of the category are seen closer to the prototype than others). Study: when asking participants to rate typicality of items, ratings change from highly typical members, through atypical members, to non-members. Results are replicated for colors, natural categories, artefacts, social categories etc. Study: Participants need to answer 'yes' or 'no' to statements such as 'tomato is a fruit'. High - and low- typicality items produced rapid verification times. Borderline cases were slower. Study: When people have to enumerate as many members from a category as they can, they mention prototypes first. Study: numbers such as 22 and 4 are seen as better examples of even numbers than 18 or 26, and take less time to answer => typicality gradients exist even within artificial categories. The studies that support the typicality gradient hypothesis also support the idea of fuzzy (ill -defined) boundaries between categories. If some items receive no high rating for any category and low rating for several categories, then there is uncertainty about category boundaries. Concepts can be unstable. Typicality ratings are affected by context. Study: participants rate cows and goats as better examples of animals than horses and mules after reading a text about milking. The opposite is true when the context and the passage is about riding. Prototype theory accounts for the context-dependence by e.g. increasing the weights of one or more attributes. Evidence against prototype theory Prototype theories assume that people use only fairly superficial attributes for categorizing objects. They cannot include so-called central attributes, which people are also using during categorization. Typicality might be an end product of other classification processes that do not use typicality. The fact that participants can judge the typicality of items does not necessarily imply that it is important in the mental representation of these items. While some abstract concepts (e.g. work of art, science and crime) do show a prototypical structure, others do not (e.g. beli ef or instinct). Hierarchical structure and basic level For many concepts, the intermediate level of abstraction (basic level) plays a cognitively critical role, because it maximizes Complex Cognition Page 2 For many concepts, the intermediate level of abstraction (basic level) plays a cognitively critical role, because it maximizes dissimilarity between categories (=> it's possible to discriminate between categories )and similarity within a given category (=> the category can be used efficiently, because at the basic level members of a category can be interacted with in a similar way). When asked to list features common to superordinate, basic and subordinate level categories, participants listed 3, 9 and 10. 3 features, respectively. There is a large gain from when one goes from the superordinate level to the basic level, but only a small gain when one goes from the basic level to the subordinate level. When asked to say whether the picture of an object was a member of a category, people were faster with basic -level categories than with superordinate categories. When asked to name pictures of objects, people are more likely to choose names at the basic level. What counts as a basic level varies as a function of expertise and culture. American (non-expert) students use 'bird' to name pictures of birds, while bird experts name them using terms such as 'sparrow' and 'robin' => for experts, the subordinate level becomes the basic level. Individuals in non-industrialized societies use the biological genus to name plants (e.g. 'maple'), while individuals in Western countries use the level above (e.g. 'tree'). Exemplar-based theories Exemplar-based theories of categorization assume that we hold a large number of memory representations called exemplars/instances. We store a representation of an external object in memory every time we see it or interact with it => for each category, we have millions of instances stored in our long-term memory. When an object is presented, all instances are matched in parallel against it using some measure of similarity, and the insta nce closely matching it is retrieved. While it seems wasteful in resources, several computational models have implemented this idea, showing that it is theoretically possible. Evidence for the exemplar view Typicality gradients can be explained with the fact that typical instances have more exemplars similar to them. Exemplar-based theories can also account for the instability of concepts: matching objects in different contexts will retrieve differe nt instances, and thus different concepts. Exemplar-based theories assume storage of variability (because all instances are stored in long -term memory), while prototype theories assume that that information is lost (because prototypes are averages of instances). Study: participants are asked if an unknown 18-inches long object can be a ruler or a pizza. People choose pizza more often. The assumed reason is that people take variability into account when categorizing objects. While pizzas and rulers share the same size on average (around 12 inches), pizzas vary in size and rulers do not. Weaknesses Both prototype and exemplar-based theories assume that category membership judgment and typicality should co -vary. However, in the case of typicality judgments for even numbers, they do not (because typicality varies for numbers that are even). The theory assumes that each instance is fully stored in long-term memory when seen, but this is quite debatable. It fails to explain how concepts are organized and possibly linked to each other. Some concepts are described using arbitrary definitions. Some members of such categories share little similarity with each ot her => any theory based only on similarity cannot explain how humans use such concepts. Explanation-based theories Explanation-based theories - people use common-sense explanations to categorize objects. These common-sense explanations, motivated by intuitive theories people have about the world, specify what attributes should be used for categorizing objects. For example, when classifying abstract drawings, participants might remember that eyes are important in identifying people and thus the size of the eyes is very likely to be a predictive feature. Conversely, they will not hair length as a feature b ecause it can easily be changed. In this theory, concepts do not exist independently of our knowledge of the world in long -term memory, but they are linked. Evidence for explanation-based theories Explains why classification times vary depending on the task: In tasks where a rapid categorization is needed, a measure of similarity based on superficial features is used. In tasks where time is not essential but the quality of categorization is, people will rely more on central features and on t he type of explanations they provide. Explains why some concepts seem more natural and coherent than others. If an explanatory theory can be found, coherence will increase. Explains why classification can vary between contexts - different attributes (superficial or central) were used. Complex Cognition Page 3 Explains why classification can vary between contexts - different attributes (superficial or central) were used. Weaknesses of explanation-based theories Explanation-based theories lack specificity: it is not clear what a 'theory' is and how it relates to other parts of semantic memory. There is a danger of circularity of definition: concepts are based on explanations, but explanations themselves depend on con cepts. The only way to avoid this circularity is to specify exactly the relations between concepts and explanations, which is not do ne in current theories. They should explain when cognitive processing stops (e.g. when seeing a picture of a dog, many common -sense pieces of knowledge are activated: dogs are hungry because they need food for survival; food can be bought in supermarkets; the owner of the supermarket wants to make a profit, etc.) Role of strategies, attention and short-term memory The different theories of categorization may account for different aspects of the data. In real life, people probably use all 4 mechanisms. People might use alternatively definitions, prototypes, exemplars and explanations in different situations or domains. In artificial domains, people use the type of definitions and rules proposed by the classical approach. People tend to use prototypes at the beginning of learning a domain and exemplars later. Exemplar models provide a good account of the data when the number of instances in a category is small (as it is possible to memorize individual stimuli). However, prototype models seem more suitable to explain how people deal with categories that contain a large number of instances, where learning all stimuli is impossible. Human cognition is flexible and study participants tend to use the strategy suggested by the experimenter. The way instances are processed determines how they are going to be learned. Learning by classifying instances (e.g. 'Is a mushroom a vegetable?') will lead to a different type of categorization than ma king inferences about these instances (e.g. 'Will this mushroom poison me?'). Goals and attention are also important when learning categories. Focusing one's attention on different aspects of the stimuli will lead to the learning of different categories. Article Summary - Essentially biased: why are people fatalistic about genes Article summary here. Article Summary - Valuing differences and reinforcing them: multiculturalism increases race essentialism Article summary here. Article Summary - On the epidemic of food waste: idealized prototypes and the aversion to misshapen fruits and vegetables Article summary here. Complex Cognition Page 4