Tackling Data Lecture Notes PDF
Document Details
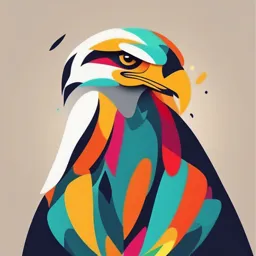
Uploaded by UnconditionalJasmine
Radboud University
2024
Peter Klaren
Tags
Summary
These are lecture notes for Statistics 1, part of the Bachelor Programme Biology at Radboud University, covering data handling and statistical analysis in the context of biology. The notes cover topics from data validation and organization to common statistical procedures. A key skill for quantitative approaches within medical biology.
Full Transcript
PETER KLAREN TA C K L I N G D ATA L E C T U R E N O T E S T O A C C O M PA N Y S T A T I S T I C S 1 , C O U R S E C O D E N W I - B P 0 1 2 C BACHELOR PROGRAMME BIOLOGY RADBOUD UNIVERSITY 2024 – 2025, Q1 Copyright © 2024 Peter Klaren Front picture: England hooker Tom Youngs confidently t...
PETER KLAREN TA C K L I N G D ATA L E C T U R E N O T E S T O A C C O M PA N Y S T A T I S T I C S 1 , C O U R S E C O D E N W I - B P 0 1 2 C BACHELOR PROGRAMME BIOLOGY RADBOUD UNIVERSITY 2024 – 2025, Q1 Copyright © 2024 Peter Klaren Front picture: England hooker Tom Youngs confidently takes on Wales fly-half Dan Biggar at the Wales v England rugby match (Cardiff, 16 March 2013) in the Rugby Six Nations Championship. Picture your- self, dear reader, tackling data and statistics with as much gusto as Tom Youngs does. This work is licensed under a Creative Commons “Attribution- NonCommercial-ShareAlike 4.0 International” license. Eighth printing, August 2024 Contents 1 About the course 9 1.1 Welcome! 9 1.2 Course objectives 9 1.3 Course software and hardware 10 1.4 Course prerequisites 11 1.5 How to read/use Tackling Data 11 1.6 About the lectures and practical sessions 13 1.7 Team-based learning 13 1.8 Examination 14 1.9 Contact and communication 15 2 Refresh your math 17 2.1 Introduction 18 2.2 Learning to read – Symbols and abbreviations 18 2.3 Learning to write – From phrases to mathematical expressions 19 2.4 Quantities have dimensions and units 21 2.5 Manipulating expressions 24 2.6 Some other mathematical notations 26 2.7 Further reading 28 2.8 Exercises 29 2.9 Answers to the exercises 31 I Validating, organising, and summarising data 33 3 Preventing errors – Data validation 35 3.1 Shit happens 36 4 Tackling Data 3.2 Controlling data entry 36 3.3 Validating data from others 40 3.4 Exercises 43 4 Variable types and data organization 47 4.1 You are not a robot - your computer is 48 4.2 A dataset contains values of variables 48 4.3 Get to know your variables 51 4.4 Importing observations for analysis 52 4.5 Dealing with missing and mixed-up data 53 4.6 Dealing with even more variables 54 4.7 Tidy data 56 4.8 Exercises 60 5 Summarizing data 63 5.1 A sample is mini-population 64 5.2 Descriptive statistics 65 5.3 Summarizing a sample – descriptive statistics 65 5.4 Data display – show me the data 69 5.5 Exercises 73 II Effect sizes and some theory 75 6 Variation and distributions 77 6.1 Introduction 78 6.2 All data vary, but biological data vary most 78 6.3 From frequency to probability density 80 6.4 The normal distribution is a bell-shaped probability density 81 6.5 Normal distribution: what is it good for? 83 6.6 How to use a probability density function 83 6.7 One size to fit all normal distributions 85 6.8 The standard normal distribution – what is it good for? 87 6.9 “Not normal” distributions 89 6.10 Exercises 89 CONTENTS 5 7 Effect sizes 93 7.1 A sample is miniature population 94 7.2 You never take the same sample twice. 1 95 7.3 You never take the same sample twice. 2 96 7.4 Samples, effect sizes, confidence intervals 97 7.5 Effect sizes for categorical data (counts) 99 7.6 Effect sizes for continuous (numeric) data 106 7.7 Effect sizes for associations or correlational data 108 7.8 Conclusion 110 7.9 Exercises 110 8 Null hypothesis significance testing 111 8.1 Introduction 112 8.2 Fisher, Neyman-Pearson, and NHST 113 8.3 What a p-value and statistical significance are not 114 8.4 NHST and the effectiveness of IQ pills 114 8.5 p( D | H ) ̸= p( H | D ) 118 III Analyzing numeric differences between sample means 121 9 Analyzing differences between two independent samples 123 9.1 Comparing two independent groups – an example 124 9.2 Attempting a z-transformation 125 9.3 Student and his t-distribution 125 9.4 Student’s t-test and the t-distribution 126 9.5 Can I use a t-test in the first place? 128 9.6 My data do not pass the homoskedasticity test! 129 9.7 Wilcoxon/Mann-Whitney U-test – A nonparametric alternative 130 9.8 Effect sizes 132 9.9 Back to our example 133 9.10 Reporting the analysis results 133 9.11 Reporting the analysis results 134 9.12 Exercises 135 6 Tackling Data 10 Analyzing differences between two paired samples 137 10.1 Comparing two paired samples – an example 137 10.2 Paired t-test 138 10.3 Wilcoxon’s signed rank test for paired observations 139 10.4 Reporting the analysis results 140 10.5 Exercises 141 11 Analysis of variance (Anova) 143 11.1 A family of errors 144 11.2 Anova – Comparing group means by analysis of variance 145 11.3 Kruskal-Wallis test – A nonparametric alternative for Anova 149 11.4 Anova has a significant outcome! Now what? 151 11.5 Effect size measures 153 11.6 Reporting the analysis results 154 11.7 Exercises 154 IV Analyzing differences between counts (categorical variables) 157 12 Analysing counts and frequencies 159 12.1 The χ2 -test comes in two variations 160 12.2 Calculation of the test statistic χ2 160 12.3 Chi-squared goodness-of-fit test 161 12.4 Chi-squared – Test of independence 163 12.5 Effect size 167 12.6 Reporting the analysis results 168 12.7 Excercises 168 13 Draw the line - Linear regression and correlation 173 13.1 Are two variables, x and y, associated? 178 13.2 The regression equation 180 13.3 Regression lines and confidence intervals 181 13.4 Reporting the analysis results 184 13.5 Exercises 184 CONTENTS 7 V Flow charts and tables 189 14 Choose your effect size 191 15 Choosing a statistical procedure 195 15.1 Know your data 195 15.2 Decision trees 196 16 Tables 199 16.1 The standard normal distribution or z-distribution 200 16.2 Student’s t-distribution 203 16.3 Chi-squared distribution 205 16.4 F distribution 207 16.5 Wilcoxon/Mann-Whitney’s U-statistic 210 16.6 Wilcoxon’s signed rank 213 16.7 Correlation 214 16.8 Random numbers 216 17 Bibliography 221 18 Index 229 1 About the course If there is effort, there is always accomplishment. Jigoro Kano (1860 – 1938), Japanese educator, founder of judo This chapter explains what you can expect of me, and what I expect of you in our course Statistics 1. 1.1 Welcome! Welcome to Statistics 1! This course aims to lay a first foundation in data handling and statistical analysis that will help you in your bachelor and master programme, and perhaps beyond as well. You can learn how to validate and organise data for statistical analysis, how some very common statistical procedures work, and how to choose and use them. These lecture notes contain quite a number of literature refer- ences. I encourage you to browse the bibliography. Many of the listed items are very well accessible for the curious and motivated young academic. You might want to read literature in addition (or instead of) my lecture notes. Pick and read literature that you find appealing. You might start to find statistics more interesting than you can possibly believe right now. (That’s what happened to me, to my surprise.) There is more, much more to learn about data analysis and statistics than can be conveyed in an introductory course. 1.2 Course objectives I intend not to “teach to the test”, and I do hope that you will not “study to the test.” Quantitative analysis and statistical literacy are important academic competences that require not only knowledge, 10 Tackling Data but also skill, a positive attitude and motivation, and a bit of perse- verance. This course will help you to reach the following goals: 1. You can correctly describe the basic statistical jargon used in this course in your own words. For example: p-value, z, t, degrees of freedom, mean, median, mode, standard deviation, standard error, normal distribution, confidence interval, effect size, etc., etc. 2. Given a research question and dataset, you can: (a) Name and characterise the variables in a data set; (b) Organize the data set for statistical analysis; (c) Choose an appropriate analytical procedure; (d) Perform the analysis (using the software JASP); (e) Interpret and report the outcome (effect size, p-value, signifi- cance). 3. You can correctly apply these procedures: (a) z-test; (b) Student’s t-test for independent observations and paired observations, respectively; (c) Wilcoxon-Mann-Whitney U-test and Wilcoxon’s signed rank test (non-parametric alternatives for the independent and paired t-test, respectively); (d) One-way analysis of variance (Anova); (e) Kruskal-Wallis test (non-parametric alternative for Anova); (f) χ2 -test of independence and χ2 goodness-of-fit test; (g) Linear regression and correlation. You can construct a cal- ibration curve and use it to calculate the value of a sample’s analyte from an instrument’s readings. 4. You feel more comfortable in a quantitative approach of (medi- cal) biology. This objective is not formulated in terms of observ- able student behaviour. Still, I do hope that this will also be an outcome of the course. You will train for course objectives 1, 2, 3 and 4 during self-study, lectures and practical sessions. Objectives 1, 2 and 3 will be tested in the final written examination. 1.3 Course software and hardware We will use the free statistics software JASP.1 JASP has a user- 1 https://jasp-stats.org/. JASP friendly graphical user interface and is updated frequently, offering stands for Jeffreys’s Amazing Statistics Program, in recognition of British more and more state-of-the-art statistical procedures and learning mathematician and statistician Harold modules with each new version. Jeffreys (1891 – 1989). JASP does not require specific hardware, and will run smoothly on a run-of-the-mill laptop. JASP is also installed on our faculty’s Chapter 1. About the course 11 servers, and is accessible in our terminal rooms via the Windows Start menu. I strongly encourage you to install JASP on your per- sonal device to practice with the software when you are not in class, and to analyse data that you will gather in other courses. When you have JASP installed, adjust one program setting. Find Preferences, select Results, and check the box “Display exact p- values” under Table options. Microsoft’s spreadsheet software Excel will be used for data en- try and data validation only, not for statistical analysis. Excel is not serious statistical software. Excel runs on our faculty servers, and is preinstalled on many Windows- and Mac-operated machines. A free alternative is LibreOffice’s spreadsheet software.2 2 https://www.libreoffice.org/ 1.4 Course prerequisites I assume you have some experience in working with spreadsheets. I hope you took the introductory workshop on data processing in Excel in the orientation week.3 If you made serious efforts in work- 3 A guide “Data processing in Ex- ing through the workshop’s assignments, you will be adequately cel” is available in the “Skills Portal Biosciences”: www.science.ru.nl/ equipped to use Excel in this course. biologyskills. I also assume you are not suffering from numerophobia or arith- mophobia (if you have to look up what this is you probably diag- nose negative). If you are, please contact me before or after class or in my office. Everyone has at least some mathematical abilities. And every- one can improve those abilities through study and practice. The University of Sheffield (UK) maintains a mathematics and statistics support centre; some of the materials offered there could be useful for you.4 Finally, Barbara Oakley, a professor in systems engineer- 4 https://www.sheffield.ac.uk/mash/ level ing at Oakland University, offers easy-to-follow learning strategies for mathematics and science.5 5 B. Oakley. A Mind for Numbers: How Please remind yourself that to enroll as a Biology student in to Excel at Math and Science (Even If You Flunked Algebra). Penguin-Random our university you had to take mathematics in secondary school House, 2014 and pass a final examination. You have managed years of math in school, you will manage this course in our university! 1.5 How to read/use Tackling Data These lecture notes are the English translation and edited version of materials that were compiled during the course’s 14-years old his- tory. It is not a verbatim transcript of the lectures’ content. Indeed, I will treat some topics that are not in these notes, and some topics in these notes will not be treated in class. It is wise to study these notes and to attend the lectures and practical sessions. If you want to brush up your secondary-school mathematics, you might want to read Chapter 2 as self-study. We will not treat this chapter explicitly during the course. Any quantitative analysis starts with gathering, validating, and 12 Tackling Data organizing data in a format that allows computerized analyses. Chapters 3 and 4 will treat these topics. Chapter 5 introduces our first statistical jargon and how to use it to summarize a data set. Chapter 6 is a theoretical one and de- scribes how the famous bell curve of the normal distribution is derived. Chapter 7 discusses sampling a bit more, and presents methods to describe effect sizes. Once you understand some of the consequences of sampling, it is time for a short Chapter 8 on null hypothesis significance testing (NHST). This outlines some basic philosophies behind classical statistics. NHST procedures for the analysis of differences between sam- ples are described in Chapters 9 to 12, treating different variable types and experimental designs. Chapter 13 is on the analysis of an association between two variables. Perhaps the most difficult task in Statistics is not how to do a procedure (we have software to do that for us), but how to select an appropriate one. Chapters 14 and 15 offer some guidelines to help you choose an appropriate effect size measure and statistical procedure, respectively. Finally, Chapter 16 contains statistical tables. Formally we don’t need these because our statistics software will produce exact values for us. Still, I think it serves an educational purpose when you can check computer output with your own calculations. That is also one reason why these lecture notes contain the formulas with which statistical procedures are performed. We will not worry about how they are derived, we trust our mathematicians in this. But: they are an invitation to you to manually check computer output. When you experience that you can reproduce a statistical calculation you trigger the reward centre in your brain, releasing the neurotransmitter dopamine (Fig. 1.1). Kicks for free! More importantly: you have opened the black box of statistics a bit. Figure 1.1: The ventral tegmental area (orange region) releases dopamine into the nucleus accumbens (pur- ple region) via the mesolimbic pathway and releases dopamine into the prefrontal cortex (blue re- gion) via the mesocortical pathway. From: https://openbooks.lib. msu.edu/neuroscience/chapter/ motivation-and-reward/. And: if you spot an error, please let me know! A dedicated dis- cussion forum is open in Brightspace. Chapter 1. About the course 13 1.6 About the lectures and practical sessions No need to copy text from presentations word by word (Fig. 1.2). I will make handouts of the lectures available after class. Lec- tures will be recorded and recordings will be made available via Brightspace. Stay focused during lectures and take notes, preferably using pen and paper, not a laptop. Indeed, you will need to sketch curves or write down formulas, which is easier done by hand than via a keyboard. Also, laptops can be a source of distraction, not only for Figure 1.2: Don’t be a copy zombie. you but also for those sitting close to you. They are poor in-class study aides. I’d rather see that you don’t use them during lectures. Talking about sources of distraction: please put away your mo- bile phone during a lecture or when you are working on an exer- cise. You will encounter lots of new concepts during the course, and it is best to be as dedicated in class as you can. Now and then I will ask you to use your phone as a learning tool to vote in a quiz, but that will be the only allowable educational use of your phone during class, as far as I am concerned. Please come well-prepared to class. Read the assigned litera- ture, have a first look at exercises, and watch the online instruction materials on JASP’s website.6 Collaborate in the TBL team that you 6 https://jasp-stats.org/ are assigned to. Discuss how to tackle an exercise, jointly decide on a best approach, and help each other out when stuck. Every class day there is a question-and-answer session on the topics of the day, a discussion of the practical exercises and other pertinent matters. I expect to see all of you there. 1.7 Team-based learning In this course, as well as in other courses you will take, the educa- tional concept of team-based learning (TBL), a flipped classroom method, is employed. TBL has been introduced to you in the orien- tation week. Make sure that you know what is expected from you, and reach out to your team members. On TBL-days, after self-study you will take readiness assurance tests individually (iRAT) and then as a team (tRAT) to assess your understanding of the content you prepared for. Application exer- cises are team activities in which you work on the resolution of an authentic “real world” statistical issue. In Statistics 1, the applica- tion consists of 2 to 3 statistical questions in context, each followed by 4 different solutions that, in principle, are all correct. You discuss and reach a consensus on the best solution within your team, and then simultaneously share your team’s choice with the other teams. Since not all teams might have chosen the same option, a discus- sion between teams will follow in which the reasoning behind the selected option is shared. An excellent way to test and share your understanding! 14 Tackling Data 1.8 Examination Your course grade will be determined by five components: A practical test (20% of the final grade); The results of your iRATs (20%); The results of your team’s tRATs (12%); A peer evaluation (8%); A theoretical exam (40%). The relative contribution of these components is based on the importance of the different learning objectives as well as the work loads. You require a grade of at least 50.0% for the combined TBL com- ponent (iRAT, tRAT, peer evaluation), and at least 50.0% for the written exam to earn the course credits. The final overall grade must be at least 55.0% to pass the exam and earn 3 credits. 1.8.1 Practical test In week 7 of the course you take a practical test in which you will have to organise and import two small datasets in JASP, perform an appropriate statistical analysis, and interpret and report the outcome. You can use these lecture notes during the test, but you are not allowed to use a calculator. 1.8.2 Individual Readiness Assurance Test (iRAT) The iRAT is a multiple-choice test of 3 to 5 questions about the pre- class preparation. You will take a number of iRATs, you can discard the lowest score. 1.8.3 Team Readiness Assurance Test (tRAT) The tRAT has the same questions as the iRAT, but the answers are now chosen by team consensus. Online scratch cards will be used to give the answers with your team. If your team only needs one attempt to select the correct answer you will receive 4 points. If you need to scratch twice to select the correct answer, you will receive 2 points. Three times is 1 point and four times is 0 points. 1.8.4 Peer evaluation In the peer evaluation you constructively and anonymously evalu- ate your team members on their contribution and participation in the team activities (tRAT, applications). There will be a formative peer evaluation mid-way through the course, and a summative one in the last week that counts for 10% in the final grade. A peer evaluation consists of two parts: Chapter 1. About the course 15 Qualitative feedback on each team member, and Quantitative grading of your team members. You perform these peer evaluations via a link in Brightspace. To pass this course, it is required that you fill in the full peer evalua- tion in time for all team members in the end-term peer evaluation. If you do not perform the peer evaluations, you automatically get a deduction of 50% for the peer evaluation part of this course. Af- ter each peer evaluation you receive the anonymous qualitative feedback from your team members. The mid-term peer evaluation is formative and will not be part of your grade. It will help you to gain insight in your team per- formance. The grade that you get from your team members in the end-term peer evaluation is your grade for the peer evaluation part of this course. Both qualitative feedback and grading are com- pletely anonymous. 1.8.5 Theoretical examination The course is concluded with a written online exam. All course components and learning materials are part of the examination material. The exam will test whether you have achieved the course objectives (see Section 1.2). The exam will consist of 3 open questions and 10 multiple choice questions. Computers or laptops are not allowed, you can bring: 1 A4 formula sheet written on both sides; 1 A4 sheet with flow charts to select a statistical procedure; A calculator. You are not allowed to use a graphical calculator. 1.9 Contact and communication Please discuss any questions relating to the content of the course in an appropriate discussion forum in Brightspace, our university’s learning management system. English is the lingua franca here. https://brightspace.ru.nl You will find that you and your colleagues often are very well capa- ble of explaining statistical concepts to each other. I will check the discussions at least twice a day during the course and contribute when necessary. You can contact me before or after class for anything you don’t want to bring up in Brightspace or during a class meeting. I work in the department of Plant & Animal Biology, my office is in room hg02.011 in the Huygens building of the Faculty of Science. Tele- phone: 024 3653245, e-mail: [email protected]. You can correspond with me in Dutch and English. Let’s get started! 2 Refresh your math Equations are more important to me because politics is for the present but an equation is something for eternity. Albert Einstein (1879 – 1955), German-born physicist Non notationes sed notiones. Carl F. Gauss (1777 – 1855), German mathematician and astronomer. Statistics is a quantitative discipline, with a firm basis in mathematics. You will encounter formulas and symbols in these lecture notes that are probably new to you. Still, the formulas that are presented do not go beyond addition and subtraction, multiplication and division, and squaring and rooting: arithmetic operations that you almost certainly have covered in primary school and the first years of secondary school. Mathematical language and technical jargon do not neces- sarily confuse. On the contrary, a basic understanding will help your intuition of how statistical procedures work. This chapter is an effort to demystify statistics and to open the black box a bit. After studying this chapter, you should know how to read, interpret, and manipulate simple mathematical and statisti- cal notation. Key terms and concepts: formula, symbol, prefix, constant, parameter, variable, quantity, unit, dimension. 18 Tackling Data 2.1 Introduction You might already have noticed, leafing through these pages, that there are quite some mathematical notations. I do take a risk, here. Indeed, it was found that a high number of mathematical expres- sions per page negatively affects a paper’s citation rate.1 Appar- 1 T. W. Fawcett and A. D. Higginson. ently a “heavy use of equations” does not improve the accessibility Heavy use of equations impedes communication among biologists. and legibility of a text. Not a very good thing, it seems, when writ- Proceedings of the National Academy of ing lecture notes that I want you to read and understand. Sciences of the United States of America, 109:11735–11739, 2012 Interestingly, Fawcett & Higginson’s study itself sparked a dis- cussion on the statistical analyses they used, and whether other scientific disciplines (i.e., physics) suffered from the same phe- nomenon as well.2 This chapter, still, is intended to help you read 2 J. E. Kollmer, T. Pöschel, and J. A. C. Gallas. Are physicists afraid of and understand the mathematical statistical notations and the no- mathematics? New Journal of Physics, tions or concepts they contain. 17:013036, 2015; and A. D. Higginson and T. W. Fawcett. Comment on ’Are physicists afraid of mathematics?’. New Journal of Physics, 18:118003, 2016 2.2 Learning to read – Symbols and abbreviations To study life sciences and enroll in college or university you had to take math in secondary school for a couple of years. Perhaps you even took (and passed) a final examination. You probably also took chemistry and physics next to biology. So you already have been exposed to symbols, such as F for force, N for nitrogen, Asn as the three-letter code for the amino acid asparagine and N as its single- letter code, λ for wavelength, m for mass, R for the universal gas constant, ρ for density, A for alanine. Et cetera, et cetera, et cetera. There are many more quantities that require a symbol than there are letters in the Greek (see Table 2.1) and Roman alphabet. So we often have to use the same symbol more than once: A for alanine, A for adenine, A for adenosine, A for ampere, A for argon, A for absorbance, A for amplitudo, A for surface area. But still: the use of symbols and abbreviations makes life easier: they often are better understood than the expansion. Some examples: Writing the sequence: “adenine uracil guanine,” you perhaps wouldn’t immediately recognize the mRNA (Hey! Another ab- breviation!) start codon AUG, coding for the amino acid M. Since 1996, the euro symbol (€) is the sign for the official cur- rency of the Eurozone. You all use it and know its meaning. Figure 2.1: Skeletal for- T3 is a useful shorthand notation for the thyroid hormone 3,5,3’- mula of (2S)-2-amino-3-[4-(4- l-triiodothyronine, which itself is a trivial notation of the offi- hydroxy-3-iodophenoxy)-3,5- diiodophenyl]propanoic acid. Or cial name: (2S)-2-amino-3-[4-(4-hydroxy-3-iodophenoxy)-3,5- T3. diiodophenyl]propanoic acid (Fig. 2.1). The formidable official name doesn’t tell you straight away that this thyroid hormone contains 3 iodine atoms, not 4 as in the prohormone T4. In that respect the abbreviation T3 is more informative than its official full name. Chapter 2. Refresh your math 19 Table 2.1: Greek alphabet list. Names, Name Letters and lower and upper case letters. Not alpha α, A iota ι, I rho ρ, ϱ, P all symbols are used in these lecture notes. beta β, B kappa κ, K sigma σ, ς, Σ gamma γ, Γ lambda λ, Λ tau τ, T delta δ, ∆ mu µ, M upsilon υ, Υ epsilon ϵ, ε, E nu ν, N phi ϕ, φ, Φ zeta ζ, Z xi ξ, Ξ chi χ, X eta η, H omicron o, O psi ψ, Ψ theta θ, Θ pi π, Π omega ω, Ω Some German cars are better known as BMW than as an auto- mobile rolling off the Bayerische Motoren Werke AG production lines. I think these examples nicely illustrate the usefulness of short- hand notation. We often are more familiar with abbreviations than with full names. Let’s have a look at an important biological process: water + carbon dioxide → glucose + oxygen It is the net reaction of carbon fixation during photosynthesis. You will recognize “carbon” in carbon dioxide, and “oxygen” in, well, oxygen. But that seems to be about it. If you don’t know what glucose is, this reaction scheme is not as informative as it could be. And where does water go in the reaction?3 3 “Water” in itself doesn’t tell you Things become much more informative when you use chemical that this is dihydrogen monoxide, or H2 O. The offical systematic name symbols and notation to describe the same reaction: of glucose is (2R,3S,4R,5R)-2,3,4,5,6- pentahydroxyhexanal, but that most 6H2 O + 6CO2 → C6 H12 O6 + 6O2 probably would not increase your insight in the chemical reaction that takes place in photosynthesis. Now you can see that water’s hydrogen atoms and carbon diox- ide’s C-atoms end up in glucose, and that oxygen can be seen as a waste product. The stoichiometry of the reaction, i.e., the propor- tions in which the molecules react, becomes clear: six molecules of water and six of carbon dioxide form one glucose molecule with the release of six oxygen molecules. In this course you will learn quite some new symbols: x̄ for sample mean, σ for population standard deviation, r for correlation coefficient, and many more. Look at them with an open mind, and try to understand what they represent. 2.3 Learning to write – From phrases to mathematical expres- sions If short-hand notation was all there is to it in using symbols, that wouldn’t be much. In the previous paragraph we have seen how to use a chemical expression that describes how the different atoms in water and carbon dioxide recombine to form glucose. Symbols 20 Tackling Data Table 2.2: Some statistical symbols Measurement Statistic Parameter and their meaning. x̄ is pronounced “x-bar”. Many textbooks write “sd” Mean x̄ µ for standard deviation, and “Var” or Standard deviation s σ “var” for variance. The correlation Variance s2 σ2 coefficient is sometimes written with a capital “R”. Number of observations, sample size n Population size N Correlation coefficient r ρ (i.e., the quantity they represent) can be grouped in an expression that shows the relationship between them. In statistical science we use mathematical expressions to describe relationships between constants, variables, statistics and parameters. A constant can only have one particular value, such as 4, π, 0 K or −273.15 ◦ C for absolute zero, g = 9.81 m s−2 for Earth’s gravitational acceleration. A variable can take multiple values that can be measured. Body height, eye colour, tomato yield in a greenhouse, bacteria count in a stool sample, serum T3 concentration, etc., are all variables. A statistic is typical for statistical science. It is a number that is calculated from a sample using some mathematical formula. Exam- ples are a sample mean (x̄) and standard deviation (s). A parameter (in a statistical context) is a number that tells you something about a population. Examples are a population’s mean (µ) and standard deviation (σ). A population’s parameter value most often is unknown and has to be estimated from a sample’s statistic. To distinguish between the two, statistics are denoted in Roman, parameters in Greek symbols (Table 2.2). How to write a relationship between constants, variables, statistics and parameters as a mathematical expression is well de- scribed by college mathematics teacher Nancy Myers (1939 – 2011): “Solving an applied problem is very much like translating a para- graph from one language to another. We must translate a paragraph written in English into another language called “Mathematics.” This process involves learning the meaning of English words in terms of mathematical symbols, and then translating English phrases into mathematical phrases.” 4 4 N. Myers. Algebra for College Students. D. Van Nostrand Company, New York, So it seems to be a matter of learning a new language and transla- 1979 tion! Compare the following statement and its translation: Verbatim Mathematical notation There is a number x such that seven added to three times this number is equal to the product of eight and the 3x + 7 = 8(2x − 5) quantity of five subtracted from twice this number. Both are equivalent, but I think you will agree with me that it is difficult to immediately grasp the verbatim, wordy notation on the Chapter 2. Refresh your math 21 left and understand what it all means. The mathematical notation is much clearer, and also easiest to solve for x. (Can you solve the equation in the box above, and show that x = 47 8 13 = 3 13 ?) Finding x and other abstract symbols is not all there is in learn- ing to write and understand mathematical notation. The relation- ship between constants, variables and statistics comes to life when Figure 2.2: The famous “Find x” symbols represent biological, chemical, physical, and statistical meme. Obviously the Pythagorean the- orem will help you find the numerical quantities. Let’s talk a bit about this. value of x. Now try this: write down the theorem first in mathematical, and then in verbatim notation. Which one 2.4 Quantities have dimensions and units is easiest to write? A quantity is an amount or number that can be measured, quanti- fied. It has a dimension: “that what is measured”, and is expressed as the product of a numerical value and a unit. A quantity X can be formally written as: X = { X }[ X ] where { X } represents the numerical value, and [ X ] an appropriate unit. For instance, you can have: { Body height } = 1.80 [ Body height ] = m Body height = 1.80 m Or: { Concentration } = 128 [ Concentration ] = mol L − 1 Concentration = 128 mol L − 1 The unit is the scale on which the quantity is expressed. Quot- ing a quantity without its unit makes the numerical value of the quantity meaningless. The same quantity can be measured in different units, but it always has the same dimension. For example for a typical body height: 1.80 m = 180 cm = 1.80 × 10 − 3 km ≈ 70.866 inch ≈ 5.905 ft But: no matter what unit is used for body height, the dimension is always the same, here: length (L). Don’t confuse the dimension L (length) with the unit L (liter)! The International System of Units (Système Internationale d’Unités, SI) recognizes seven dimensions with their associated base quantities, units, and symbols (Table 2.3). From the base quantities and dimensions all other physical, chemical and biological ones are derived. The base quantities most used in biology have the dimensions L, M, T, and N, and are com- bined to produce an almost infinite number of derived dimensions. 22 Tackling Data Table 2.3: Base quantities, dimensions, Base quantity and symbol Dimension Unit symbol units and symbols in the SI. By con- vention, units are written without a Length (l), width, height L m (meter) prefix. The unit of mass, kg (103 g), is Mass (m) M kg (kilogram) the only exception. Time, duration (t) T s (second) Electric current (I, i) I A (ampere) Thermodynamic temperature (T) Θ K (kelvin) Luminous intensity (I v ) J cd (candela) Amount of substance (n) N mol (mole) Let’s start with the base quantity Length and go from there. Body length (height) is simply expressed in the base dimension L. A surface area has the derived dimension L2 (length × width: L × L = L2 ). When you consider a volume to be a three-dimensional space, the dimension of volume is L3 (length × width × height). Velocity, v, is a quantity that indicates the distance moved by an object in a certain time period. Its dimension is: L/T or L T−1 , and can be expressed in the units km h−1 , or mm s−1 , or even in “miles per hour” that includes a non-SI unit. Velocity’s dimension still is the same, though. A derived unit that you will encounter often in the laboratory is that of molar concentration, expressed in the number of moles per liter. The dimension is: N/L3 or N L−3 , the unit is: mol L−1. Note the different use of the letter L in designating the dimension length (L) and unit liter (L)! (One liter is defined as 1 dm3.) Units can be preceded by a prefix or multiplication factor (see Table 2.4) which circumvents the use of an impractical number of trailing and leading zeros. To illustrate, the yearly total emission in 2020 of carbon dioxide by power plants, industry, households, traffic and agriculture in the Netherlands was 138 300 000 000 kg, or 138.3 Mt (1 tonne is defined as 103 kg).5 5 Source: RIVM/Emissieregistratie An example with very small numbers: the molar concentration (https://www.emissieregistratie. nl/data/overzichtstabellen-lucht/ of the thyroid hormone T3 circulating in your blood typically is broeikasgassen, accessed July 2022). about 2 nmol L−1 , or 2 × 10−9 = 0.000 000 002 mol L−1. Using Avogadro’s constant (NA = 6.022 × 1023 mol−1 ) you can calculate that this amounts to a bit more than 1.2 × 1015 T3 molecules per liter blood. 2.4.1 Rules for handling dimensions and units You cannot add apples and oranges. This may be stating the obvi- ous, but, to quote biochemist Athel Cornish-Bowden: “Consideration of units and dimensions is sometimes regarded as a pedantic nuisance, but this is a pity, because it is one of the most powerful tools that scientists have for detecting mistakes in algebra, not only their own but also other people’s.” 6 6 A. Cornish-Bowden. Basic Mathematics for Biochemists. Oxford University Press, 2nd edition, 1999 Proper consideration of units and dimensions helps you do arith- metic and (chemical) calculations correctly, and will in the end help Chapter 2. Refresh your math 23 Table 2.4: Prefixes used in scientific Symbol Name Factor communications. In biology and medicine prefixes k, d, c, m, µ, n, and T tera 1012 1 000 000 000 000 p are the most common. G giga 109 1 000 000 000 M mega 106 1 000 000 k kilo 103 1000 da deca 101 10 100 1 d deci 10−1 0.1 c centi 10−2 0.01 m milli 10−3 0.001 µ micro 10−6 0.000 001 n nano 10−9 0.000 000 001 p pico 10−12 0.000 000 000 001 f femto 10−15 0.000 000 000 000 001 a atto 10−18 0.000 000 000 000 000 001 you to start a statistical procedure with bona fide data. Cornish- Bowden formulated some simple rules on how to work with di- mensions and units. I have modified them a bit and present them below: Rule 1. Don’t equate quantities with different dimensions. Writing “3 cm = 3 kg” is nonsense and not allowed. Writing “3 cm > 1 kg” also. Indeed: cm is a unit of the dimension length (L), kg is a unit of a different dimension: mass (M). Figure 2.3: Don’t equate different Rule 2. Only quantities with the same unit can be added or sub- quantities. Don’t add apples and tracted. oranges. Don’t add population number, feet above sea level and Don’t try to add six meters to two hours (6 m + 2 h = ?). The year of establishment. Sign in the terms have different dimensions and thus different units. Don’t village of New Cuyama, Califor- try to subtract one hour from 3600 seconds (3600 s − 1 h = ?). nia, US. Image by Mike Gogulski, https://commons.wikimedia.org/w/ Here the terms have different units despite having the same index.php?curid=2513523. dimension. Where you see an expression in which quantities are added or subtracted, they must have the same unit (and dimension). Rule 3. When multiplying or dividing quantities, multiply or divide the units as well as the numbers. You can divide six meters by two hours to obtain a new quantity: velocity, with the dimension length (or distance) per time: 6m 6 m m velocity = = = 3 = 3 m h−1 = L T − 1 2h 2 h h Rule 4. Exponents are dimensionless. Don’t use a quantity with units as an exponent. You can raise 2 to the power 5 (25 = 32), you cannot raise 2 to the power 5 km (25 km = ?). Where you see some complicated exponent, for instance an exponent of the number e: ( x − µ )2 − e 2σ2 24 Tackling Data you know that the factors in the numerator and denominator of the exponent: ( x − µ)2 and 2σ2 , respectively. must have the same dimension. The pure number 2 is dimensionless. Since in the numerator µ is subtracted from x, it follows from Rule 2 that x and µ also must have the same unit. So: x, µ, ( x − µ), and σ all have the same unit and dimension. The numerator and denominator cancel out, the exponent is dimensionless, and only a (negative) number remains. (You will meet this exponent again when we discuss the normal distribution.) Rule 5. A logarithm is dimensionless. Don’t take the logarithm of a quantity with units. This is for the same reason that an exponent is dimensionless. Quite often you will find it necessary to log-transform measure- ments for the purpose of some statistical procedure. For instance: the log-transform of body height = 1.80 m is: log10 1.80 = 0.2553. The unit m is ignored. You cannot state that the log-transform of body length is “0.2553 log10 m.” A log-transformation you will surely know is: pH = −log10 [H+ ], with [H+ ] in mol L−1. If you want to know what mathe- matical trick is performed to make log-transformations of numbers with When you encounter an expression that only uses symbols and units a valid procedure, you will have algebra: check for dimensional consistency. When you see an ex- to read Cornish-Bowden’s book! The pression that contains numbers: check the units. answer is on p. 74. 2.5 Manipulating expressions Here are some simple manipulations you can perform. 2.5.1 Fractions In Equation 2.1, A is the numerator, B is the denominator: Attention readers from the Low Lands: Don’t mix up the term numerator with A the Dutch “noemer”! Numerator is (2.1) “teller” in Dutch, denominator is B “noemer”. The following expressions with fractions are equal: B A A× = ×B C C A×B = C 1 = A×B× C In the latter notation, the fraction 1/C, written with a solidus (/) or obelus (÷) can also be written in a linear, exponential form: C −1. As in: 1 A × B × = A × B × C −1 C (You have seen this linear notation already a few times on the pre- vious pages.) So, for example, we can express the quantity velocity in the unit kilometres per hour, and write it as km/h, or as km h−1. Chapter 2. Refresh your math 25 Sometimes the multiplication sign (×) is written as a centered dot (·), and sometimes a multiplication sign is omitted altogether: P × Q × R = P · Q · R = PQR Writing divisions with a solidus (·/·), obelus ( ·· ), or exponent , and multiplications with ×, ·, or no sign at all often is a matter of legi- bility and what an author thinks looks aesthetically nice on a page. Writing fractions in exponential notation has clear advantages, though. See the next section. 2.5.2 Exponentional notation of fractions and roots Writing fractions with a solidus can be ambiguous. Consider the following complex fraction: X = A/B/C How is this to be interpreted? A/B A X= or X= ? C B/C These two notations will give two different numerical outcomes. Just check for yourself: 6/3 6 ̸= 2 3/2 Writing fractions as exponents is exact. When you write the value of the universal gas constant as R = 8.314 J/mol/K, you might be confused between 8.314 J/mol 8.314 J R= and R= K mol/K The first notation is the correct one, and differs dimensionally from the second one. I here prefer to write R’s unit in a linear exponen- tial format. It also fits nicely on one line: R = 8.314 J mol−1 K−1 Formally we have to write the unit as: J1 mol−1 K−1 , but 1 is “the exponent we never write.” Indeed, A1 = A. In some cases, however, for example when simplifying expressions, it is wise to incorporate “1” explicitly to keep track of all exponents. In the final result the exponent 1 can then be omitted. 2.5.3 Multiplying and dividing exponents Using an exponential notation you can apply the following rules for working with powers. Multiplying two powers to obtain the product is done by summing the exponents: Am × An = Am+n 42 × 43 = 42+3 = 45 = 1024 26 Tackling Data Similarly, by subtracting exponents you can divide two powers: Now you can also “prove” that any number raised to the power 0 equals 1: Am x a−a = x a /x a = 1 = x0. = Am × A−n = Am−n An 42 1 1 5 = 42 × 4 − 5 = 42 − 5 = 4 − 3 = 3 = 4 4 64 The product rule and quotient rule only apply to expressions with the same base! Am × Bn ̸= ABm+n 43 × 52 ̸= 205 2.5.4 Power rule To raise a power to another power you will have to multiply the exponents: n Am = Am×n 3 42 = 42×3 = 46 = 4096 It is also possible to write roots as exponents. The square root of A can be written as √ A = A1/2 which notation is easier to manipulate when rearranging expres- sions containing roots and powers: √ 1 2 1 42 = 42 = 42× 2 = 41 = 4 2.6 Some other mathematical notations Throughout these lecture notes you will meet mathematical sym- bols other than those mentioned above. You will perhaps remember their meaning from your high school days, but still: to make sure I here give a short overview. 2.6.1 Larger, smaller... a < b, a is smaller than b a > b, a is greater than b a ≤ b, a ⩽ b, a is smaller than or equal to b a ≥ b, a ⩾ b, a is greater than or equal to b a ≪ b, a is much smaller than b a ≫ b, a is much greater than b a = b, a is equal to b a ̸= b, a b, a is not equal to b a ≈ b, a is approximately equal to b a ∼ b, a ∝ b, a is proportional to b Chapter 2. Refresh your math 27 The magnitude of “much smaller” and “much greater”, and how approximate “approximately” is, depends on the context in which the symbols are used. 2.6.2 A glossary e Euler’s number is named after the Swiss mathematician Leon- hard Euler (1707 – 1783). Base of the natural logarithm. The value of e is the sum of the infinite (see Infinity) series ∞ 1 1 1 1 1 1 e= + + + + +··· = ∑ 0! 1! 2! 3! 4! n =0 n! Something to evaluate on a rainy day. Keep in mind that 0! = 1. For mere mortals the rounded approximation e = 2.7183 is sufficient. See also Factorial and Summation sign. E- or e-notation Not to be confused with Euler’s number! Scientific or exponential notation to write a number, in particular very large or very small numbers. The letter E or e indicates the po- sition of the decimal point. It can be read as “times 10 to the power of...”. For example, 1.234E+2 means 1.234 × 102 , which is 123.4. A small number such as 0.000 005 can be written as 5e-6, which is 5 × 10−6. On your calculator you can use the EXP, E or EE (enter exponent) key to use scientific notation. See also Figure 2.4: Microsoft Excel and some statistics software use the E- or e- Fig. 2.4 for how Microsoft Excel interprets an E. notation to write numbers. Compare the number as it is entered in cell A1, Ellipsis, “...” A series of dots that has the meaning “and so forth” and the value in the formula bar, and or “continuing indefinitely” in mathematics. It can indicate an note how convenient the E-notation is in writing numbers with many leading infinite sequence of values: or trailing zeros. 1, 2, 3,... Or it can indicate the value of an irrational number such as e and π that have an indefinite continuation of digits after the decimal point: e = 2.7182... or e = 2.7182818284... π = 3.1415... or π = 3.1415926535... It can also indicate the omission of values when a mathematical operation (such as addition, or multiplication) is repeated. See Summation sign. Factorial, n! The factorial of a positive integer n is the product of all numbers from n down to 1. For example: 5! = 5 × 4 × 3 × 2 × 1 = 120 “Today I know that there is no limit Infinity, ∞ Mathematical infinity, such as the number of points on to human stupidity – it is infinite.” Gustav Flaubert (1821 – 1880), French a continuous line, or the size of an endless sequence of numbers: novelist. 1, 2, 3,... 28 Tackling Data Logarithm, common The logarithm with base 10 of a number. The common logarithm of 100, log 100, equals 2 because 102 = 100. In some countries and statistics software (such as JASP and R) log x is denoted by log10 x, lg x, or 10 log x. Logarithm, natural The logarithm with base e of a number. The nat- ural logarithm of 10, ln 10, equals 2.30258... because e2.30258... = 2.71828...2.30258... = 10. Note that in some countries and statistics software (such as JASP and R) ln x is denoted by log x. See also e. Summation sign, ∑ Sum of a set of n values. The subscript of the summation sign indicates the index of the starting value. This often is the first value in the set with index i = 1. The index is often indicated by the letter i, but it can be any other letter. The index increases in steps of 1. The superscript indicates the end value of the index, most often n. The end value of the index can also be indicated by some other letter than n. When the summation sign has no sub- and superscripts you can assume that the summation starts at i = 1 and includes the complete set. Examples: n ∑ a i = a1 + a2 + a3 + · · · + a n i =1 n ∑ x j = x k + x k +1 + x k +2 + · · · + x n −1 + x n j=k The summation of the set F = {1, 1, 2, 3, 5, 8, 13} is: ∑ F = 1 + 1 + 2 + 3 + 5 + 8 + 13 = 33 The summation of the fifth to seventh value in F is: 7 ∑ F = 5 + 8 + 13 = 26 i =5 The summation of squared values in F is: ∑ F2 = 12 + 12 + 22 + 32 + 52 + 82 + 132 = 273 2.7 Further reading Mathematical notation is a compact and to-the-point description of complex relationships. Appreciate them, and use them to practice and experiment with. Below I suggest some books (some non- technical) for further reading to help you refresh your numeracy. Mathematician and popular-science writer Ian Stewart is very fond of mathematical expressions. In his book on important equa- tions he writes: “...words are too imprecise, and too limited, to provide an effective route to the deeper aspects of reality. Words are too coloured by human-level assumptions. Words alone can’t provide the essential insights. Equations can.”7 7 I. Stewart. Seventeen Equations that Changed the World. Profile Books Ltd., 2012 Chapter 2. Refresh your math 29 Clearly a fan! He discusses the history and implications of some famous equations, but not without first presenting the mathemati- cal notation, dissecting a formula and explaining what it all means in plain language. Well worth a read. Dedicated to “everyone who has ever been made to feel bad at mathematics”, mathematician and concert pianist Eugenia Cheng wrote Is Maths Real? Cheng asks herself “dumb questions” and gives non-technical insightful answers, all in an effort to rid the world of “math phobia“.8 8 E. Cheng. Is Maths Real? Profile Biochemist Athel Cornish-Bowden wrote a no-nonsense textbook Books Ltd., 2023 that treats the basic arithmetic and mathematics that should be in the biologist’s toolbox.9 It starts out with fractions and logarithms, 9 A. Cornish-Bowden. Basic Mathematics and ends with simple calculus. Although my lecture notes do not for Biochemists. Oxford University Press, 2nd edition, 1999 contain any calculus, Cornish-Bowden’s book reintroduces your secondary-school math (including differential and integral calcu- lus). If you have worked through Basic Mathematics for Biochemists, or if you already are pretty confident in your mathematical skills, you might want to try Stephen Scott’s highly structured Beginning Mathematics for Chemistry.10 It starts off with algebra, and then 10 S. K. Scott. Beginning Mathematics for guides you through basic calculus and statistics. Chemistry. Oxford University Press, 1995 On a more general biological level, Richard Burton published two books as “an encouragement to quantitative thinking.”11 The 11 R. F. Burton. Biology by Numbers. An two editions are crammed with numerical biological examples, Encouragement to Quantitative Thinking. Cambridge University Press, 1998; and from Darwin’s estimations of the number of earth worms in a R. F. Burton. Physiology by Numbers: An field, to topics on kidney function and acid-base balance. Work- Encouragement to Quantitative Thinking. Cambridge University Press, 2000 ing through Burton’s examples is a great exercise, and you will learn more than just mathematics in the process. Personally, I found it very insightful to read (and check) the assumptions and calcula- tions William Harvey (1578 – 1657) made that lead him to describe the closed circulation of blood. 2.8 Exercises 1. Can you handle fractions and exponents? (a) Calculate 74 − 53. Give your answer as a fraction, simplify as much as possible. (b) Calculate 67 ÷ 32. Give your answer as a fraction, simplify as much as possible. 1 1 (c) Simplify 1+1 + 1+1. 2 3 3 4 2a 3 (d) Simplify 10a 4. 3 −2 n n (e) Write 103 × 65 as a fraction. Hint: ba = ban. 2. Can you read mathematical and scientific notation? (a) Use your calculator to calculate 31.2 · 30.6. 30 Tackling Data (b) Use your calculator to calculate 8.6−0.75. (c) The mean value of the set S = {10, 12, 14, 16, a} is x̄ = 100. What is the value of a? (d) Consider the following set: A = {2, 4, 6, 8, 10, 12, 14}. What is the value of ∑ib= a (3A2 ), with a = 1 and b = 3? (e) Why is ln 1000 = 6.9077... a correct statement? 3. Can you work with equations? (a) Solve for x: 3x2 = 75. (b) Use your calculator to solve for b: log10 b = 0.95. (c) Calculate the number of carbon (C) atoms in 5.0 g pure car- bon, when the molar mass of C is 12.01 g mol−1. You will need Avogadro’s number (NA = 6.022 × 1023 mol−1 ) to answer this question. Give your answer in 2 decimals. (d) The radioactive iodide isotope 131 I is used in medicine to treat tumours of the thyroid gland. The isotope desintegrates (“decays”) with a half-life (t1/2 ) of 8.02 days. The activity At of the isotope that remains after a certain period of time is given by the function: − ln 2 ·t t1/2 A t = A0 · e where A0 is the initial activity at time point t = 0, and t the time period in days. i. Show that at t = t1/2 , At = 21 A0. ii. What percentage of A0 remains after 15 days? iii. How long does it take for the activity of 131 I to fall to 1% of the initial activity? (e) Spectrophotometry is a much-used analytical technique in bi- ological and clinical laboratories. It is based on the absorption of light of a specific wavelength through a sample solution in a cuvette (a small test tube with straight sides). See Fig. 2.5. Figure 2.5: Basic design of a sim- Measurements are expressed as “absorbance” (A), defined as ple single beam spectrophotometer. the logarithm of the ratio of the intensity of incident light (I0 ) ©Yassine Mrabet / Wikimedia Com- mons / CC BY-SA 4.0. to that of transmitted light (It ) through a sample. Lambert- Beer’s law relates A to the concentration c of a dissolved com- pound: I0 A = log10 = ϵ·c·l It Here, c is the compound’s concentration, and l is the path length that the beam of light travels through the cuvette. In a regular standard cuvette l = 1 cm. i. What is the relationship between It and c? ii. You measure A = 0.260 in a sample. What is the relative amount of light that was absorbed by the sample? Chapter 2. Refresh your math 31 iii. 15 % of the 340 nm radiation incident on a solution of NADH is transmitted. Given ϵ N ADH = 6.22 × 106 cm2 mol−1 , what is the NADH concentration in mol L−1 ? The path length l = 1 cm. 2.9 Answers to the exercises 1. Can you handle fractions and exponents? 1 (a) 12. 7 (b) 9. (c) 2 32 35. 1 (d) 5a. (e) 25 175 243. 2. Can you read mathematical and scientific notation? (a) 7.2247... (b) 0.1991... (c) a = 448. (d) ∑31 (3A2 ) = 168. (e) Because e6.9077... = 1000. 3. Can you work with equations? (a) x = 5 or x = −5. (b) b = 8.9125.... (c) 2.51 × 1023. − ln 2 ·t1/2 t1/2 (d) i. At = A0 · e = A0 · e−ln 2 = A0 · 1 2 ii. 27.4 %. iii. 53 days. (e) i. c is inversely proportional to It : the higher the com- pound’s concentration, the lower It (and the higher A). ii. 45 % (It = 55 %). iii. c = 1.32 × 10−7 mol cm−3 = 1.32 × 10−4 mol L−1. Part I Validating, organising, and summarising data 3 Preventing errors – Data validation On two occasions I have been asked, “Pray, Mr. Babbage, if you put into the machine wrong figures, will the right answers come out?” Charles Babbage (1791 – 1871), English mathematician and philosopher, inventor of the first programmable computer When you learn statistics you almost invariably are given nice, clean datasets, ready-to-go for analysis. The real scien- tific world in the laboratory, clinic, and in the field, however is ugly. The datasets you will be working with will, or could, contain errors. It is important that you can check a dataset for suspicious data before you perform an analysis. Indeed: “Garbage in, garbage out”, as the conventional wisdom goes. This chap- ter presents a number of tools, mostly based on common spreadsheet functionalities, to detect and correct errors. In particular conditional formatting, and controlling user in- put by setting limits is useful. When you are dealing with a dataset compiled by others, plotting and sorting data are two simple methods to detect typos, roque values and sus- pected outliers. After studying this chapter, you should know how to do the following: 1. Use software tools (spreadsheet, statistics software) to prevent erratic data input in a spreadsheet. 2. Use software tools to check and validate a dataset for suspicious data. 36 Tackling Data Key terms and concepts: data validation, controlled input, cleaning data, descriptive statistics. 3.1 Shit happens “Human data entry can result in errors that ruin statistical results and conclusions. A single data entry error can make a moderate correlation turn to zero and a significant t-test non-significant.” 1 1 K. Barchard and L. Pace. Preventing human error: the impact of data Handling data is subject to errors. I have seen a colleague measure entry methods on data accuracy and statistical results. Computers in Human the same sample and enter the following triplicate absorbance Behavior, 27:1834–1839, 2011 readings in a spreadsheet: 0.301, 0.305 and 302. He then calculated a mean value of (0.301 + 0.305 + 302)/3 = 100.9, and happily Absorbance (A), also known as optical proceeded with the subsequent analyses, oblivious to the missing density (OD), is the quantity of light absorbed by a solution. It is used in leading decimal point in his last entry (which of course should have spectrophotometry. read 0.302) and to the fact that measuring an absorbance value of 100.9, let alone 302 is physically virtually impossible. Correct data analysis starts with correct data entry. Microsoft Excel and other spreadsheet software offer tools to help you to validate and clean data, but also some functionalities that are a bit hazardous to use. I will introduce some of these briefly while making a neat Body Mass Index calculator. 3.2 Controlling data entry To calculare a body mass index (BMI): enter someone’s body height (in m) and body weight (in kg) in two (separate) cells in a blank worksheet. In a third cell BMI can then be calculated using the simple formula: kg BMI = 2 = kg m−2 (3.1) m Figure 3.1: Minimal input to calculate a body mass index (BMI). Try to maintain overview of your calculations by clearly in- dicating what cell contains what variable and in what unit it is expressed (see Fig. 3.1). When the cell containing the formula is selected and function key F2 is pressed, Excel shows the typed formula and, in colours, the cells that the formula takes as input (Fig. 3.2). Mistakes can now be easily checked and corrected. Below you will find some hints and tips on how to control and validate your input, and remain vigilant in preventing errors. Chapter 3. Preventing errors – Data validation 37 Figure 3.2: Colour-coded information on the arguments of the function in cell C5 appear when pressing function key F2. 3.2.1 Beware of autocorrect and autocomplete functions Many spreadsheet packages, and Excel is no exception, have an autocorrect functionality that automatically converts input to what the software thinks is best. It is supposed to be a time-saver, but it can lead to errors. For example, when a bioinformatician opens a spreadsheet and types the gene code APR1, which is short for the enzyme“adenosine 5’-phosphosulphate reductase 1” in Arabidopsis thaliana,2 Excel converts the gene code to a date: 1 April, or April 2 A. thaliana is thale cress, a popular Fool’s Day (see Fig. 3.3). How appropriate. model organism in plant biology. Figure 3.3: In cell A1 the literal text APR1 was entered, which is automat- ically converted to the date of April Fools’ Day. Excel even adds the year 2001 although it was not given as input. Already almost two decades ago a study found that autocor- rection affected at least 30 gene names in public bioinformatics databases.3 More recently, Mark Ziemann and colleagues inspected 3 B. R. Zeeberg, J. Riss, D. W. Kane, circa 7500 gene lists attached as supplementary files to almost 3600 et al. Mistaken Identifiers: gene name errors can be introduced inadvertently research papers published in 18 different journals.4 Approximately when using Excel in bioinformatics. 20% of published articles with gene lists in Excel files were marred BMC Bioinformatics, 5:80, 2004 by (automatically) misspelled gene names. The problem not only 4 M. Ziemann, Y. Eren, and A. El-Osta. Gene name errors are widespread in occurs with gene names, but also with other entries that contain the scientific literature. Genome Biology, numbers. For example, suppose you wish to enter mouse number 17:177, 2016 2 from strain 1 as “1-2” or “1/2” in an Excel spreadsheet, this will be converted to “1 February” or to “January 2”, depending on your regional format settings. Figure 3.4: In cell A1 the literal text ‘APR1 (note the apostrophe preceding the text) was entered, which is now correctly displayed as intended. The apostrophe forces Excel to interpret the cell’s content as text. Unfortunately, there is no way to turn this annoying service off. However, you can manually change the cell’s format to “text”. You can also precede the cell’s input with an apostrophe. Both actions will force Excel to interpret the input as text (see Fig. 3.4). When you are not alert, you can also fall in the trap of Excel’s 38 Tackling Data autocomplete. Once one cell contains some text, Excel will remem- ber this. When you start typing in the next cell, Excel will use the first characters that appear and the contents of the top cell to sug- gest the new cell’s input (see Fig. 3.5). You will have to find and 5 Navigate to File > Options. In the Options window, open Advanced. In use Excel’s advanced option settings to disable the autocomplete the Editing Options section, uncheck feature.5 the Enable AutoComplete for cell values tick box. Figure 3.5: In cell A1 the text Homo sapiens was entered. My intention was to enter Homo erectus in cell A2, but immediately after typing the first letter “H”, Excel offers to complete the entry with “omo sapiens”. Not what I intended. All in all, when you are unaware of how your spreadsheet soft- ware receives and handles your input, you are bound to make errors. 3.2.2 An eye on the data – Conditional formatting One way of making data pop up, and also to detect possible mis- takes in data entry (on which more in the next section), is by letting the make-up of your cell reflect its content. This can be achieved by selecting and using a conditional formatting style in the Home tab (Fig. 3.6). Returning to our BMI: a BMI ≤ 18.5 is generally considered to be too light. Someone with a BMI between 18.5 and 25 has a healthy body weight, a BMI between 25 and 30 is classified as overweight, Figure 3.6: Use conditional formatting to define font and fill of a cell depend- and a BMI > 30 defines obese persons. It would be nice when the ing on the value it contains. colour of the cell holding the BMI-value would reflect this. To do so, you have to select Conditional Formatting in the Home tab different New Rule and Use a formula to determine which cells to format. You can have multiple rules per cell, make one for every BMI class. Choose informative colours for each one (think traffic lights), see Fig. 3.7 for an example. When a BMI class is defined by a lower and upper value a logical function =AND(...) can be used to set these values. For instance: =AND(C5>=18.5; C55 eggs. in a bird’s nest For sure, a book receiving 4 stars on Goodreads can considered Goodreads is an internet database to be a better read than a 2-star book, but it is silly to state that of books and reviews: https://www. goodreads.com/book. it is exactly twice as good. Similarly, someone who ticks the box “1 – Strongly disagree” when asked “Do you love statistics” in a questionnaire using a Likert scale doesn’t appreciate the discipline five times less than someone who answers “5 – Strongly agree” (if that is possible at all). You might think that the variable Clutch size is a numerical one, for you can count eggs in a bird’s nest, calculate a mean clutch size for a colony of birds, etcetera. But, this is not about the number of eggs, but about the nest as a whole! A nest can belong to one of the four ordered categories, and that makes Clutch size as defined in Table 4.3 truly an ordinal, not a numerical variable. See Fig. 4.5. Figure 4.5: Great Black-backed Gull 4.2.3 Quantitative or numeric variables – measurements (Larus marinus) nest with a clutch size in the 2-to-5 eggs category. Variables that are measurable and that can be expressed in a com- ©Banangraut/CC BY-SA 3.0/Wiki- media Commons. bination of a numeric value and a unit are quantitative or numeric variables. A pulse rate of 68 bpm has a numeric value of 68, and is expressed in the unit beats per minute (bpm). Pulse rate is a numeric variable of the discrete type as it can take Chapter 4. Variable types and data organization 51 on only integer values, within natural limits (a pulse rate of zero is an obvious lowest limit). A normal pulse rate is between 60 and 100 beats per minute (bpm). You can skip a beat but not half a beat, and a pulse rate of 83.5 bpm is impossible. However, an average pulse rate is a continuous variable as it can take any value within natural limits. When your doctor takes your pulse for two minutes and records a total pulse rate of 145 beats in those two minutes, then your average pulse rate is 72.5 bpm. Numeric variables can be subdivided in interval variables and ratio variables. Ratio variables are variables that have a logical zero and of which the ratio is meaningful. Body weight, for instance, is a continuous ratio variable. A person weighing 96 kg weights exactly 96/60 = 1.6 times a person weighing 60 kg. The ratio of two ratio variables is not affected by the unit in which they are expressed. The same body weights but now in imperial pounds (lbs) still give 1 lb is defined as 0.453 592 43 kg the same ratio: 211.6437.../132.2773... = 1.6. Temperature is a typical interval variable: only the distance or interval between measurements is meaningful. It is incorrect to state that water at 40 ◦ C is twice as warm as water at 20 ◦ C. Think about what happens when you express the same temperatures in Kelvin (313 and 293 K, respectively) or degrees Fahrenheit (105 and 68 °F). The ratios (1.07 and 1.54, respectively) differ greatly with the unit used. Also, water at 0 ◦ C has a temperature of 273 K and 32 °F: no logical zeros for the interval variable Temperature. Think back, for a moment, to our independent variable Sex. You can determine whether a patient is male of female and count the number of patients in each category. Although, for instance, a count of 10 females is twice as much as a count of 5 males, this does not make Sex a numerical ratio variable! It is key to look at a single observation and determine what value it can take. In the case of our independent variable, an observation of the sex of a single patient can only take one of two values: Male or Female. Hence, Sex is a categorical nominal variable. The categories contain counts only. 4.3 Get to know your variables Correctly identifying the number and type of variables in a dataset is important when you decide to import a dataset in your favourite statistics software for analysis, and when you have to decide on a statistical procedure. The distinctions between ratio and interval, and between discrete and continuous numeric variables are statistically trivial, as they do not determine the statistical analyses that you can choose. What is important is: a) To identify the independent and dependent variable and how many there are, and 52 Tackling Data b) To identify what type they are: nominal, ordinal, or numeric: ad a) Variable type ad b) Variable type Independent Dependent Qualitative, Quantitative, categorical numeric Nominal Ordinal These taxonomies are not mutually exclusive: an independent variable can be of the numeric type, a dependent variable can be of the ordinal type, vice versa, and etcetera. 4.4 Importing observations for analysis You would probably enter the data of Table 4.1 in a similar format in an Excel spreadsheet (Fig. 4.6), save it as a.csv file (comma sep- arated values file), and attempt to import it in JASP, or some other software that you use. Figure 4.6: The data from Table 4.1 in a spreadsheet, saved as a.csv file. Fig. 4.8 shows how JASP interprets the data presented exactly in the format of the.csv file in Fig. 4.6. You can see that the process is not error free. Let’s have a closer look. An important problem lies in the fact that the data frame Figure 4.7: Symbols used in JASP to in Fig. 4.6 is not perfectly rectangular. The first column in the indicate numeric (“scale”), ordinal and imported data frame: labeled “V1” in JASP is almost completely nominal variables. empty, except for the terms “average” and “s.” You entered these terms in the spreadsheet to indicate the calculated mean values and standard deviations. This helps you and your readers to interpret the table directly; unfortunately it is useless in making data ready for import. JASP will interpret “V1” as a nominal variable with three categories: “ ”, “average”, and “s.” Yes, you noticed correctly: the value of the first category is indeed empty (“ ”), as are the cells Chapter 4. Variable types and data organization 53 Figure 4.8: The data from Table 4.1 imported as a.csv file in JASP. 1 to 11 in the first column in the imported data frame. Moreover, line 11 contains no data at all. Not what you intended. A second flaw has already been mentioned: Table 4.1 contains not only measured data, but also calculations on those data, i.e., “average” and “s” in lines 13 and 14. It is almost impossible to correctly instruct your software to distinguish between raw data and calculated data that occur in the same column. Thirdly, because the data frame is not perfectly rectangular there are quite some empty cells. JASP indicates cells without values with a dot (·). Finally, JASP interprets the pulse rate values as nominal vari- ables: compare the symbol in the column header with that in Fig. 4.7. This is rectified by clicking on the symbol in the column and change the variable type to the correct one, here: scale. 4.5 Dealing with missing and mixed-up data Missing data or missing values are variables without a value. In Fig. 4.8 the 11th row of the imported data frame does not hold values for Resting pulse rate. It could well be that you have forgot- ten to take the pulse of a subject, or that measurements failed. It could also be that you made an error in data entry by accidentally skipping a row, or that you forgot to enter the data altogether. It is therefore wise to indicate incomplete observations by labeling missing values yourselves, for instance by typing “NA” (“not avail- able”), a notation commonly used in statistics software. Do not use a numerical code such as “0” or “999” as this can interfere with calculations performed later. 54 Tackling Data Figure 4.9: JASP output of descriptive statistics of the resting pulse rates in Fig. 4.8. A mix-up of measured and calculated values in the same column and missing values can mess up the simplest of analyses. When we ask JASP to produce descriptive statistics on the resting pulse rates split by Sex we get an output as in Fig. 4.9. JASP reports one missing value each in the Male and Female cat- egories of Sex: these must be the empty cells in row 11. Although missing values are ignored, JASP still sees 12, not 10, valid (i.e., complete) observations in our dataset: the calculated means and standard deviations are imported as bona fide observations! Also, the mean values and standard deviations are included in the calcula- tion of the mean values which makes these meaningless. Lastly, JASP wrongly thinks that the standard deviations of 10 and 8 bpm for male and female subjects, respectively, are true mini- mal resting pulse rates. The fact that the import of data and calculations is erratic is not to blame on your software. You, as a researcher, are responsible for a proper format of valid data frames. 4.6 Dealing with even more variables To make matters more complicated, our observations actually in- clude more than just a record of sex and a measurement of resting pulse rate. In fact, the complete dataset includes the pulse rate dur- ing exercise (bpm) as well, patients’ body height (cm) and weight (kg), and from this a calculated body mass index (see Equation 3.1). Also a registration of whether our subjects smoke or not, and how many hours per week they engage in exercise are recorded. Displaying multidimensional data in a table that is restricted in only two dimensions is cumbersome. We can, for instance, add an extra heading to distinguish between smokers and nonsmokers, but Chapter 4. Variable types and data organization 55 this increases the number of columns from 2 to 4 (Table 4.4). You can imagine that things become too large to be convenient when we try to include even more variables in our table. Indeed, for every extra variable the number of columns will double! Table 4.4: Resting pulse rates (beats Nonsmoker Smoker per minute) in smoking or nonsmok- Male Female Male Female ing males and females. 74 78 68 80 65 88 68 83 65 59 72 79 57 70 95 75 64 66 63 75 x̄ 65 72 73 78 s 6 11 13 3 When we enter Table 4.4 in a spreadsheet (including the double headers indicating the variable values Nonsmoker/Smoker, and Male/Female), save it as a.csv file and import it in JASP, we get a garbled result (Fig. 4.10). Variables are interpreted as categorical. The column headers contain only two sensible values (Nonsmoker and Smoker), and JASP completes the headers by including V1, V3 and V5 as nondescriptive variable names for columns 1, 3 and 5, re- spectively. The second header in Table 4.4 (containing the two levels of the nominal variable Sex) now appears as a first measurement of pulse rate?! Furthermore, we have the old problems with calculated values in the same column as measurements, and the missing data. JASP can not calculate descriptive statistics for these data. The software interprets all data to be “text” (as indicated by the “a” in the lower right hand half of the symbol for nominal variables in the column headers) and cannot calculate mean values, standard deviations and other descriptives. It’s a mess. Figure 4.10: Statistics software has problems recognizing a table with multiple headers as in Table 4.4. A statistical procedure already starts with an identification of the variables that have to be included in the analysis. You will have 56 Tackling Data to construct a data frame that reflects just this. Let’s see how to do this. 4.7 Tidy data Statistician Hadley Wickham provides a standard for the structure of what he calls tidy datasets.1 Wickham formulates three impor- 1 H. Wickham. Tidy data. Journal of tant criteria for a tidy dataset (see also Fig. 4.11): Statistical Software, 59(10):1–23, 2014 1. Each variable forms a column. 2. Each observation or subject forms a row. 3. One value per cell. Figure 4.11: Illustration of the three rules that make a dataset tidy: vari- ables are