NBA 2018-19 Win Probabilities PDF
Document Details
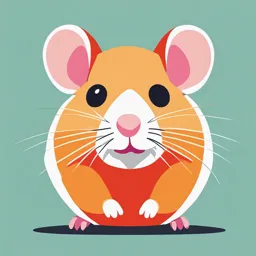
Uploaded by TruthfulRealism2101
Princess Nourah Bint Abdulrahman University
Tags
Related
- 2020 NBA Handreiking 1124 Richtsnoeren voor de interpretatie van de Wwft PDF
- Basquetebol - História e Curiosidades (PDF)
- Judge Blocks Joint Sports Streaming Service From 3 Media Giants PDF
- Michael Jordan: Một Huyền Thoại Bóng Rổ - TMAI
- Immune System Review PDF
- Bookmaker Odds Evaluation (NBA 2018/19) PDF
Summary
This document analyzes the correlation between bookmaker probabilities and actual win percentages for NBA teams in the 2018-19 season. It uses data analysis techniques to identify correlations and trends.
Full Transcript
We're going to look at the bookmaker probabilities taken from the websites that we looked at in the last video. We're going to see how closely these bookmaker's probabilities correlate with actual outcomes. We're going to take the five spots we looked at in the first course in the series, and look a...
We're going to look at the bookmaker probabilities taken from the websites that we looked at in the last video. We're going to see how closely these bookmaker's probabilities correlate with actual outcomes. We're going to take the five spots we looked at in the first course in the series, and look at the correlation between the season-long bookmaker odds and the actual outcomes in terms of win percentages of the teams in the league. We'll start by looking at the NBA for the season 2018-19. Obviously first, we import the packages that we're going to need and then we got to upload the data. We're going to call this file NBA 19. You can see here what it has is the names of the teams, the opponent, the date, and then we've got the odds of winning and the odds of losing. These are expressed as decimal or European odds, so we can obtain the probabilities by dividing one by these values. Then we got the actual points scored, whether was over time with gaming to half time, whether the first name team is the home team. We've got the identity of the game, we got the score in one go. Then here actually you already calculated the probability of winning for the home team and the outcome in terms of a win or a loss. This data is actually 2,460 rows long, which is exactly twice the number of games in the season because what we've done here is represent each team in each game in one row. We have two rows for one game, the row for the home team, and a row for the visiting team. We can then calculate the probabilities, and the results for each team, for each game across the entire season. We can re-calculate the win probability here, and so bear in mind that it's not quite as simple as just simply taking one divided by the decimal odds because we have to take account of the overround. Remember we talked about the overround of the vigorous, vig. This is the fact that the probabilities actually add up to more than 100 percent, which represent the profits of the bookmakers. What we have to do is we have to scale the outcome probabilities by the total probability associated with each outcome. That will then reduce this to our probability. In effect, scales it to 100 so that we have a genuine probability here. If we run that, we can calculate the win probabilities as follows. You can see here, in fact, we've calculated again what was already in the file, but that's not a problem. We now got these win probabilities for each game, for each season, we also know whether they won or lost the game in the dataset. What we can do is do a groupby dot groupby for each team to sum the win probabilities and the wins across the seasons. Well, we want to take the average, in fact, not taking the dot sum, but taking dot mean for these win probabilities of wins, and if we run that, we will create that as a new file, NBA team probes. If we look at that, you can see here, the first column is the win probability, in the second problem is the actual percentage of wins, win percentage for the season. Just by looking at it, you can see they're fairly closely correlated. The average ruin probability for the Atlanta Hawks during the season was 31.4 percent, let's say for each game. The actual percentage of wins during the season was 35.4. For the Celtics, the average win probability of the betting odds was 63 percent and the actual outcome for the season was just about 60 percent. As you go down the list some are closer, some are further away, but by large, these are fairly close together. Remember the win probabilities were public before the game happened. These are true predictions about what's going to happen implied by the bookmakers' odds. Just to confirm this though, we can draw a plot just to show how closely related these are. You can see here on the plot we've plotted win probabilities, each dot is one team for the season. Win probabilities on the horizontal axis, win percentage on the vertical axis and you can see here, these dots lie pretty close to a diagonal line across going from bottom left to top right of the chart, which suggests that there's almost one-to-one relationship between win probability and the actual wins for the season, suggesting that bookmakers' odds are pretty reliable. We can take this one stage further and calculate the correlation coefficient for this data and if we calculate the correlation coefficient, it turns out the correlation coefficient is plus 0.957. That is a very high correlation indeed. I got to say that's a very high figure and so that suggests that the betting odds really are, in the case of the NBA, a pretty reliable guide to the outcomes, at least when we aggregate across the season. Any one game might be closer to the truth or further away from the truth. But on average, the odds are pretty close to what the actual outcomes were. For this self-test, I suggest you try doing the same thing for the Major League Baseball data from the file supplied. But now instead let's take a look at the NHL data. NHL is slightly different because in the NHL you can think that there are three possible outcomes. It used to be that you could have tied games in the NHL, but now they got rid of that. They actually have to get a result, but the result could be in regular time or the teams could play overtime. Then if the team loses, we say that they had an overtime loss. If you win your game, you get two points for a win. But if you lose in overtime, you still get a point. Just like you used to get a point for a tie and this was long the scoring system in soccer around the world, two points for win, one point for a tie as well. Here you have that system based on but not based on ties but based on overtime losses. Let's look at the NHL data and see what we get. We load the data here and you can see the names of the teams, the date, whether the team is the home team or that's the first team name because not the opponent. Then we've got the win odds, the tie, I call them tie odds so that it's overtime loss odds, and then the losing odds. Then we've got the scores, whether it went to overtime, whether they were penalties, what the result was, win loss or overtime loss. Then as game, we've got the game details, the names of the two teams and the scores at the end of the game. You can see here, once again, the odds are represented in decimal format. To get the probabilities, we want to divide one by the decimal odds. But as with the NBA data, we have to take account of the over-round, and now that means we have to sum the probabilities of all three possible events. The win, the tie odds or overtime odds, and the losing odds. We divide the odds of each possible outcome by the sum of these three probabilities to scale the probabilities so that they add up to 100 percent. We're going again to do the same thing. We're going to compare the actual points scored in the season versus the points predicted by the probabilities. Note here that we need to know the win probability when you get two points. We need to know the tie probability when you get one point, the overtime loss. But we don't need to know the loss probability because there are no points, you get no points for loss, and so that's not going to figure in the calculation. Here we calculate the probabilities. You can see here, that the probabilities of these two outcomes if you wanted to know the loss probability, it would just be one minus these two numbers and we can go through that. Then again, we now say well, how many points did the teams actually get in the season? We know the points were equal to two times the sum of the wins plus the sum of the overtime losses. We can calculate points, and then we can calculate what we would call the expected points, for each game, what was the probability of win, and that value multiplied by two. That's your expected value of winning, plus the probability of an overtime loss or a tie times one, but the one just disappears there, and that gives us the expected points for each game. Now we have, rather like the NBA, we have a measure of performance across the season of what actually happened and we have a measure of the predictions, if you like, as implicit in the bookmaker's odds. Again, we can do a group by, and here we will just sum the actual points and the expected points. We don't need to take, we could've done it in terms of the means, but we're going to just do it in terms of the sums this time. You can see here, again as we found with the NBA, we find fairly close correlation between the expected points and the actual points. The Anaheim Ducks, based on the bookmaker odds were expected to win 73 points across the season, but in fact, they've got 80. The Coyotes we're expected to get 74, but they've got 86. The Bruins, we're expected to get 92, but they've got 107, and so on. Just again to see how closely this is related, let's draw a scatter plot. Once again, you can see the scatterplot not quite as tightly aligned on the diagonal axis here. Not quite as perfect, if you like, as we found in the NBA, but still a fairly close correlation. We can just show that by actually calculating the correlation coefficient. There you can see the correlation coefficient is what? It's point 0.88 plus 0.88. That's pretty close. Remember, the correlation coefficient must run between minus one and plus one. Plus one is completely perfectly correlated, minus one is perfectly inversely correlated and 0 is no correlation at all. You can see here, this is not quite as close to correlation as we found in the NBA, but it's still a pretty high figure in terms of the correlation between the two. Now as a self-test, you might want to go on and try this for the English Premier League data that we looked at again, English Premier League we have the same three possible outcomes as we have in the NHL. In the Premier League, we get a win, a draw, and a loss. Remember in the Premier League you get three points for a win and one point for a draw. You can do the same calculations as we've done here, for the Premier League. The correlation between total points, and expected points based on the bookmaker's odds. But again, just remembering that the scaling is different because you get three points for a win rather than two points for win. Finally, let's look at the Indian Premier League that was the 5th league we looked at and so we can look at the Indian Premier League data. This is for the 2018 season. Again, we can see the same data here, but the odds here, we've got them represented in a different format. Firstly, there are only two possible outcomes in an Indian Premier League game, win or loss. There are no ties. There are some cases of no results at all but we won't consider those. Then these are expressed as American odds and as you can see these are either how much you would win for staking a 100 or how much you have to stake in order to win a 100. Remember how you turn the moneyline odds into probabilities. If it's a positive number, it's 100 divided by 100 plus the moneyline odds. If it's a negative number, then it's the absolute value of the money line odds divided by 100 plus the absolute value of the money line odds so that should be minus the money line odds divided by 100 minus the moneyline odds, another money line value. Here I've written the code for calculating those odds which is a slightly more complicated statement, you need to use this np.where, which is like an if statement in Excel. Because it all depends on whether the moneyline odds are positive or negative, we can have a different value of the probability if it's negative or positive. But we can then calculate the win probability, the loose probability, and bearing in mind, we have to scale these win probability according to the sum of the probabilities to take account of the over-round and you can see here that if we do that, we get a win probability for each of the teams. We are interested in, again, thinking about the win probability relative to win percentage so we don't have to calculate anything for the loose probabilities since you don't get any points for loss. We could do again as we did with the previous two cases, we can do the adult groupby for the win probability and we have in the data the number of wins for each team and we can do that calculation and then remember we've only got eight teams in the Indian Premier League. Now this time, the gap seem somewhat larger between these expected values and the win probabilities. Indeed, in some cases for example, if you look at the case of the Mumbai Indians, the win probabilities are actually, based on the betting odds, these are better than 50 percent. The bookmakers thought that they would have a winning season but in reality, they actually had a quite a bad losing season, they were just below 43 percent in terms of wins. That was a rather poor season for them and if you look at for example, rural towns such as Bangalore, you see an even bigger gap. In that sense, these probabilities are not very closely related and if we draw a scatterplot again, we can see that, that's the case, there's a very poor correlation. You might think about why that is. What we found here and if you do the self tests with the Major League Baseball and the English Premier League, you see for the first four leagues we look at, we see generally this very tight correlation between Bookmaker odds and actual outcomes, the actual win percentages but we don't find this very close correlation for the Indian Premier League and we might want to ask why that is. One reason might be the fact that there are relatively few teams in the Indian Premier League so we don't have enough observations to get a reliable prediction or it might be just something about the Indian Premier League which is less predictable. It might be a fact that it's a less predictable league but it's certainly striking that it's different. But at least this has given us a way to think about the relationship between bookmaking odds, probabilities, and outcomes and give us as a sense of the reliability and how broadly speaking, the Bookmaker odds are fairly reliable predictions of outcome. In the next session, we're going to look more closely at ways of measuring this reliability and introduce the concept of the Brier Score.