Statistics and Probability - Normal Distribution PDF
Document Details
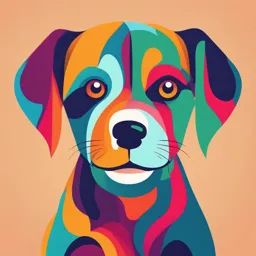
Uploaded by IntuitiveSugilite9545
Tags
Summary
This document provides a comprehensive overview of normal distributions, z-scores, and sampling distributions. It includes illustrative examples, covering topics such as normal probability distribution, the area under the normal curve, and solving for the raw score. The document also discusses different sampling techniques and their applications.
Full Transcript
Normal Distribution The study of Normal Distribution plays a significant role in the analysis and interpretation of a standard set of data or probability distribution. Normal Probability Distribution - is the data distribution where the mean,...
Normal Distribution The study of Normal Distribution plays a significant role in the analysis and interpretation of a standard set of data or probability distribution. Normal Probability Distribution - is the data distribution where the mean, median, and mode are equal and the distribution is clustered at the center. The graph of a normal distribution is a symmetrical bell-shaped curve. Properties of a Normal Curve: 1. The mean, median, and mode are equal and are represented by the central point along the horizontal axis which determines the highest point of the curve. 2. The curve is symmetrical around the mean and is symmetrical to the horizontal axis, that is, the curve extends indefinitely in both direction. 3. The total area under the normal curve is equal to 100% or 1, or 50% or 0.5 to each side from the center. Standard score or z-score - is the equivalent value of a raw score expressed in terms of the mean (µ) and standard deviation (σ) of the distribution. It measures the distance of any raw score (x) from the mean in standard deviation units. Given the raw score x, the formula for its equivalent standard score or z-score is: where: µ is the mean z= σ is the standard deviation x is the variable (sample) Illustrative Example: The DG Company has 100 branches nationwide. The annual profit is normally distributed with a mean of ₱73 million a year and a standard deviation o ₱3.25 million. Find the z-score pertaining to the number of branches having a sales of ₱80 million. Solution: Given: µ = 73; x = 80; and σ = 3.25 z= z= z= z = 2.15 Finding the Area of a Region Under the Normal Curve Illustrative Example: Using the z-table, what is the area under the normal curve from z = 0 to z = 0.25? z 0.00 0.01 0.02 0.03 0.04 0.05 0.06 0.07 0.08 0.0 0.0000 0.0040 0.0080 0.0120 0.0160 0.0239 0.0279 0.0319 0.1 0.0398 0.0438 0.0478 0.0517 0.0557 0.0636 0.0675 0.0714 0.2 0.0987 0.1026 0.1064 0.1103 0.3 0.1179 0.1217 0.1255 0.1293 0.1331 0.1368 0.1406 0.1443 0.1480 This means that the area from z=0 to z = 0.25 is 0.0987 Converting the decimal point to percentage, multiply always with 100 = 0.099 x 100 Thus, The area under the normal curve is = 9.9% Illustrative Example 2. Considering the first illustrative example that DG Company has 100 branches nationwide with a mean of ₱73 million a year and standard deviation of ₱3.25 million. What percentage of its branches have a profit of ₱73 million to ₱80 million? Z score is 2.15 Solution: z 0.00 0.01 0.02 0.03 0.04 0.05 0.06 0.06 0.07 2.0 0.4772 0.4778 0.4783 0.4788 0.4793 0.4803 0.4808 0.4812 2.1 0.4842 0.4846 0.4850 0.4854 2.2 0.4861 0.4864 0.4868 0.4871 0.4875 0.4878 0.4881 0.4884 0.4887 2.3 0.4893 0.4896 0.4898 0.4901 0.4904 0.4906 0.4909 0.4911 0.4913 From the first solution, we have obtained z = 2.15; µ = 73; x = 80 From the table: Area = 0.4842 = 48.42% How to plot the curve of the Normal Curve Every region under the normal curve corresponds to the area bounded by µ or z = 0 and the number of units of the z-score is from the center. Each area is given in percent, which also signifies the percentage of data found within the interval. Each half of the curve is equivalent to an area of 0.5 or 50%. In case of the solved problem, we can plot the curve: 48.42% µ = 73 µ = 80 z=0 z = 2.15 Illustrative Example: Consider the Illustrative example, determine the percentage of branches of DG Co. with an average profit from ₱73 million to ₱79.6 million, assuming that the standard deviation is now ₱6 million and the mean remains the same. Solution: Given: µ = 73; x = 79.6; and σ = 6 z= = z= Thus, z = 1.1 Referring to the table, the area bounded by z=0 to z=1.1 A = 0.3643 A = 36.43% of the DG Co.'s branches having a profit of ₱73 million to ₱79.6 million. To plot the curve: µ = 73; x = 79.6; σ = 6; and z = 1.1 36.43% z=0 z=1.1 Now we will discuss how to find the area under the normal curve within a given distance from the mean, To do this, we have to consider the following rules: Rule #1 1. If the two z-scores are both positive or both negative, subtract the areas. 2. If the two z-scores have different signs, add the two areas. Illustrtative Example 1: What is the area under the standard normal curve bounded by z = - 1.23 and z = 0.25? Solution: To visualize the problem, let us plot the curve according to given data: From z-table: 48.94% z = -1.23; A = 0.3907 = 39.07% z = 0.25; A = 0.0987 = 9.87% z = -1.23 z = 0.25 As per rule #1 of 2, the two-z-scores are having different signs, we have to add the two areas. Illustrative Example 2: Find the area under the standard normal curve from z1 = 1.13 to z2 = 3.06. Solution: Let us plot the curve: 12.81% From z-table: z = 1.13; A2= 0.3708 = 37.08% z = 3.06; A1 = 0.4989 = 49.89% z= 1.13 z = 3.06 z=0 From the rule: If two z-scores are both positive, subtract the areas. Thus: At = A2 - A1 = 49.89 - 37.08 At = 12.81% Activity: Find the area under the normal curve: 1. From z = - 0.15 to z = 2.18 2. From z = -2.56 to z = -3.04 How to find the area of the region to the right or left of a positive z-score. Rule # 2 If the required area is to the left of a positive z-score, then add 50% or 0.5 to Area. If the required area is to the right of a positive z-score, then subtract Area from 0.5 or 50%. Illustrative Example: What is the area under the normal curve to the right of z = 1.1; or z > 1.1? Let us plot the curve: From the z-table: z = 1.1; A = 0.3643 = 36.43% 13.57% 36.43% z =0 z= 1.1 As per the Rule (#2 of 2), if the required area is to the right of positive z-score, subtract Area from 50%: At = 50% - 36.43% Area Total = 13.57% To find the area to the right or to the left of a negative z- score: Rule # 3 If the required area is to the right of the negative z-score, then add 0.5 or 50% to the Area. If the required area is to the left of the negative z-score, then subtract Area from 0.50 or 50%. IllustrativeExample: Find the area under the normal curve to the right of z = -2.02; or z > -2.02. Solution: A = 0.4783 = 47.83% Let’s plot the curve: As to the rule above: At = A + 50% 97.83% At = 47.83 + 50 At = 97.83% z = -2.02 z=0 Activity: Find the area under the normal curve of the following in percent: 1. from z = 0 to z = 1.57 2. from z = 0 to z = -2.81 3. from z = 0 to z = 3.02 4. from z = 0 to z = 1.0 5. from z = 2.09 to z = 3.0 6. for z > 1.34 7. for z < 1.34 8. for z > -1.11 9. for z < -1.11 10. for z > -2.29 Solving for the raw score given the area under the Normal Curve. The next discussion fcuses on how to solve a raw score x given the area or percentage uncer the normal curve. From formula z = , we derive the formula for the raw score as: zσ = x - µ x = z(σ) + µ Illustrative Example: Given that: µ = 60; σ = 9; and A = 0.3944, find x if the given area under the normal curve is to the left of the mean. Solution: First we have to look for the value of z-score usiing the given area in the z-table. We look for the nearest value of 0.3944. We see in the figure below that the z-score of 1.25 has the area of 0.3944. Thus, our required value is z = -1.25 since the shaded region is to the left of the mean. z 0.00 0.01 0.02 0.03 0.04 0.05 1.2 0.3849 0.3869 0.3888 0.3907 0.3925 0.3944 Solving for x, we have: x = z(σ) + µ = -1.25(9) + 60 x = 48.75 Illustrative Example 2 Find the value of z and x when µ = 60, and σ = 9, and the area is equal to 85.5% Solution: A = 85.5% - 50% = 35.5% or 0.3550 Illustrative Example, cont'n: A = 85.5 - 50 = 35.5% From the table, the nearest possible area to 0.3550 is 0.3554 which is under z = 1.06 Since the difference is 0.0004, it is almost negligible; the z-score of 1.06 is already acceptable. Solving for x: x = z(σ) + µ = 1.06(9) + 60 x = 69.54 ACTIVITY: Fifty applicants took an IQ test and their mean score was 100 with a standard deviation of 20. Assuming that the management decided not to hire the lowest 20% of the applicants, what was the minimum score to get hired? Sample and Sampling Distributions There is a need to select a sample or subset that is of a predetermined size to represent the population and create sampling distribution for observed results. In this lesson, we will study another probability distribution which is also sampling distribution. Population - refers to the entire group under study or investigation. Sample - is a subset taken from a population either by random or nonrandom sampling techniques. Random Sampling - is a selection of n elements derived Types of Random Sampling Techniques 1. Lottery sampling - sampling technique where every member of the population, has an equal chance of being selected. 2. Systematic sampling - sampling technique in which members of the population are listed and samples are selcted in intervals called sample interval. 3. Stratified random sampling - sampling procedure wherein the members of the population are grouped based on their homogeneity. 4. Cluster sampling - sometimes called area sampling. It is applied on a geographical basis. It os generally done by first sampling at the higher levels before going down to the loer levels. For instance, Types of Nonrandom Sampling Techniques: 1. Accidental sampling - only those whom the researcher meets by chance are included in the sample when using this technique. 2. Quota Sampling - includes a specify number of persons of certain types to be taken as sample. 3. Convenience sampling - most copnvenient and fastest sampling technique taht make use of the telephone, mobile phones or internet. 4. Purposive Sampling - used in very small sample sizes. This can be used if the subjects of the study are deans of certain universitis or area managers of certain institution. How to determine the sample size: Using the formula: n = N. where: N is the population size (Sloven's Formula) 1 + Ne² e is the margin of error Illustrative Example: A researcher wants to study the academic performance in Mathematics of student from UNC. The school has a population of 12,000 students. If the researcher allows a margin of error of 5%, how many students must be included in his sample? Solution: Given: N = 12,000; e = 5% = 0.05 Using Sloven's Formula n= N. 1 + Ne² n= 12,000. = 12,000. = 12,000 1 + 12,000(0.05)² 1 + 12,000(0.0025) 1 + 30 n = 387.096 or 387 Static - is a number describes as a sample. It can be directly computed and observed. An example of a static is the sample mean. Sample of Static: sample mean sample standard deviation sample median Parameter - is a descriptive measure of a population. The value of parameter can be approximated. Sample of Parameter: population mean population standard deviation population median Illustrative Example: Construct sampling distribution of the mean and a histogram for the set of data below: 86, 89, 92, 95, 98 Solution: Step 1: Solve the population mean: µ = ΣX = 86 + 89 + 92 + 95 + 98 N 5 µ = 92 Step 2: Construct all random samples consisting of three observations ( n = 3) from the given data set. Arrange in ascending order without replacement and repetition. Then get the sample mean of each random sample. Construct Random Sample: Random Sample (n = 3) Sample Mean ( x) 86, 89, 92 89 86, 89, 95 90 86, 89, 98 91 86, 92, 95 91 86, 92, 98 92 86, 95, 98 93 89, 92, 95 92 89, 92, 98 93 89, 95, 98 94 92, 95, 98 95 Observe that 89, 90, 94, and 95 appeared only once, thus, their probability is P(x) = 1/10 or 0.1. SInce 91, 92, and 93 appeared twice, their probability is P(x) = 2/10 or 0.2 Step 3: Construct the sampling distribution of the sample means. Sample Mean (x) Probability [P(x)] 89 1/10 = 0.1 90 1/10 = 0.1 91 2/10 = 0.2 92 2/10 = 0.2 93 2/10 = 0.2 94 1/10 = 0.1 95 1/10 = 0.1 Observe that the total probability of all the sample means must be equal to 1. Step 4: Construct the histogram for the sample means: P(x) 0.2 0.1 89 90 91 92 93 94 95 ACTIVITY: 123, 126, 129, 132, 135 Using the set of data above, perform the following tasks: 1. Construct a sampling distribution of the mean. 2. Draw histogram for the sample means. Sampling Distribution of the Means Mean - is also known as average, is calculated by dividing the sum of the distribution by the total number of observations. Variance- is defined as the average of the squared deviation from the mean. The variance for sampling distribution is denoted by s² (read as s square) while the population variance is denoted as σ² (read as sigma squared) Standard Deviation - is the square root of the variance. The formula for getting the sample mean and population mean are as follows: x = Σ xi where: x is the sample mean n x1 is the value of observations in the sample n is the total number of observations in For the mean of a population for Sampling Distribution: Where is the population mean X 1 X 1 is the values of observation in the N population N is is the total number of observations in the population. FIRST FORMULAS SAMPLING DISTRIBUTION: For the Variance of a sample: 2 is the variance of a sample ( x x ) 2 Where: s s 1 2 s is the standard deviation n 1 x is the sample mean x1 For Standard Deviation: is the value of observation in 2 ( x1 x ) n s the sample n 1 is the number of observation SECOND FORMULA FOR SAMPLING DISTRIBUTION: To minimize the use of deviation, we can use the second formula instead for sample variance and standard deviation. For Variance: 2 s n x x 2 2 nn 1 Where: s 2 is the sample variance s is the sample standard For Standard Deviation x deviation is composed of values s n x 2 x 2 of observations in the nn 1 n sample, and is the total number of values in observation Illustrative Example: Find the sample, standard deviation, and variance of the following heights (in centimeters) of 10 plants: 4, 12, 14, 15, 20, 19, 18, 17, 16, 25 Solution: Construct a table. xi xi x ( xi x ) 2 4 4 - 16 = -12 (-12)² = 144 12 12 - 16 = -4 (-4)² = 16 14 14 - 16 = -2 (-2)² = 4 15 15 - 16 = -1 (-1)² = 1 16 16 - 16 = 0 (0)² = 0 17 17 - 16 = 1 (1)² = 1 18 18 - 16 = 2 (2)² = 4 19 19 - 16 = 3 (3)² = 9 20 20 - 16 = 4 (4)² = 16 25 25 - 16 = 9 (9)² = 81 x Solving for : xi 160 i ( x x ) 2 276 x 160/10 = 16 Using the First Formula: For Variance: ( xi x ) 2 = 276 = 276 s 2 n 1 10-1 9 = 30.67 For Standard Deviation: ( xi x ) 2 s = n 1 s = 5.54 Using Second Formula: For Variance: 2 n s x x 2 2 n(n 1) Using 2nd Formula: Construct a Table: x x² For Variance: 2 s n x 2 x 2 4 16 n(n 1) 12 144 14 196 s 2 10(2,836) - (160)² 15 225 10(10-1) 16 256 17 289 = 28,360 - 25,600 = 2,760 18 324 19 361 10(9) 90 20 400 25 625 = 30.67 For Standard Deviation: n x 2 x) 2 x = 160; s n(n 1) = 30.67 = 5.54 For the variance of a population: 2 2Where: is the ( Xai po- ) 2 variance of N pulation is the standard devia- Xi ( X i )2 tion of a population N N For Standard Deviation of a population: is the population mean are the values observa- tion in the population is he Central Limit Theorem - states that the sampling distribution of the sample means approaches a normal distribution as the sample size gets larger. The formulas: For the variance of a sampling distribution of the sample mean: 2 x2 n For the standard deviation oof sampling dostribution of the sample mean: x n Illustrative Example: Find the sample variace and sample standard deviation of a population. Solution: Given : σ = 5; and n = 10 For sample variance: 2 Using the formula: 2 x n 2 2 (5) 25 x 2.5 10 10 For standard deviation: Using the formula: x n 5 5 x 1.58 10 3.1623 Standard Error - is a term used to measure the accuracy with which a sample distribution represents a population by using standard deviation. If the standard deviation is used as an estimator of population parameter instead of the mean, then the Standard Error is: 2 SE x n The transformation of the z-score considering the standard error is: x z n Illustrative Example: An investigator of a case of food poisoning found that the amount of salmonella in every serving of food is normally distributed with an average of 3.7 colony forming units per gram(cfu/g) with a standard deviation of 1.19. 1. What is the possibility that a selected serving has at least 4.2 cfu/g of salmonella? 2. What is the probability that the mean of 10 randomly selected serving is at least 4.2 cfu/g? Solution: 1. From the problem: Given: x = 4.2 ; µ = 3.7; and σ = 1.19 Solving the z-value: x 4.2 3.7 0.5 z 0.42 1.19 1.19 n 1 From the table of normal curve, the area bounded by z = 0 and z = 0.42 is 0.1628 or 16.28%. 2. Then, P(X ≥ 4.2) = 50% -16.28% = 33.72%. Thus, the probability that the selected serving has at least 4.2 cfu/g of salmonella 33.72% ACTIVITY: Shinwa Company claims that the life of their battery product is approximately normally distributed with a mean length of 8hours of battery life when used in DC motors, with the standard deviation of 3.8 hours. What is the probability that a sample of 10 batteries will have a mean lifespan of less than 6 hours in DC motors? ACTIVITY: Shinwa Company claims that the life of their battery product is approximately normally distributed with a mean length of 8hours of battery life when used in DC motors, with the standard deviation of 3.8 hours. What is the probability that a sample of 10 batteries will have a mean lifespan of less than 6 hours in DC motors? Given: µ = 8; x = 6; σ = 3.8; and n = 10 Use the formula: x z From z-table, equivalent n to 45.15% Therefore there is probability that a sample of 10 batteries have life span of less than 6 hours.