Role_of_AI_in_Finance-1[1].docx
Document Details
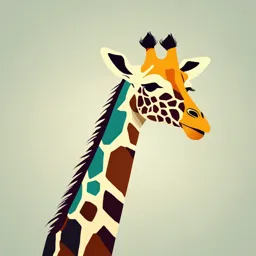
Uploaded by HappierGuqin6353
Jai Narain Vyas University
2025
Tags
Full Transcript
**Research Report on Role of AI in Finance** **Session: 2024-2025** WhatsApp Image 2023-03-13 at 5.43.52 PM.jpeg **Under the Supervision of: Submitted By:** HOD Dr. Mangu Ram Pradeep Suthar **B.Com. (Accounting And FinTech) (4^th^ Semester)** **Department of Accounting** **Faculty of Commerce...
**Research Report on Role of AI in Finance** **Session: 2024-2025** WhatsApp Image 2023-03-13 at 5.43.52 PM.jpeg **Under the Supervision of: Submitted By:** HOD Dr. Mangu Ram Pradeep Suthar **B.Com. (Accounting And FinTech) (4^th^ Semester)** **Department of Accounting** **Faculty of Commerce and Management Studies** **Jai Narain Vyas University, Jodhpur (Raj.)** **[\ ]** **[Certificate]** This is to certify that project entitled on "Research Report on Role of AI in Finance" terms is being submitted by Pradeep Suthar, Student of 4^th^ Semester of B.Com. (Accounting and Fintech), Department of Accounting, Jai Narain Vyas University, Jodhpur (Raj, IND) in partial fulfillment of Semester Examination Programme, I certify that this project is up to my expectations and adheres to Department issued guidelines. This is a record of student own work carries out under my guidance and is hereby approved for submission. **[Acknowledgment]** I am highly indebted to HOD Dr. Mangu Ram Sir for his valuable guidance, whole Co-operation, unfailing inspiration & pains taking attitude throughout the work on the topic and making it successful. My heartiest thanks to our teachers Dr. Anil\ Verma Sir, Dr. Rahul Taparia Sir, CA Laxmi Narayan Sir, Sudeep Singhvi Sir, all of my classmates, my parents, my friends and all who helped me to complete this project. **(Pradeep Suthar)** **\ ** **Abstract** The integration of Artificial Intelligence (AI) into the financial sector represents a transformative leap, promising to redefine the landscape of financial services through enhanced efficiency, accuracy, and innovation. This research project explores the multifaceted role of AI in finance, elucidating its potential to revolutionize various financial operations. Artificial Intelligence, an umbrella term encompassing machine learning, natural language processing, computer vision, and robotics, enables machines to simulate human intelligence. Finance, on the other hand, is the art and science of managing money, including activities like investing, borrowing, lending, budgeting, saving, and forecasting. The synergy between AI and finance has given rise to a plethora of applications that extend from algorithmic trading and risk management to fraud detection and customer service automation. Algorithmic trading leverages AI algorithms to execute trades at speeds and precisions unattainable by human traders, thereby optimizing trading strategies and market interactions. In risk management, AI analyzes large datasets to predict potential risks, offering financial institutions a more robust mechanism to safeguard against uncertainties. AI-driven fraud detection systems identify unusual patterns and anomalies in real-time, significantly enhancing the security and reliability of financial transactions. Despite its potential, the integration of AI in finance is not without challenges. Data privacy and security concerns loom large, as handling sensitive financial information through AI systems raises the risk of breaches and misuse. The issue of bias in AI algorithms, stemming from biased training data, can lead to unfair and discriminatory financial decisions. Furthermore, the complexity and opacity of AI models, particularly deep learning networks, pose significant hurdles in achieving transparency and interpretability, essential for regulatory compliance and trust. Solutions to these challenges are emerging, driven by advancements in AI research and a deeper understanding of its implications. Enhanced data analysis capabilities of AI can lead to more informed and strategic decision-making, while automation of routine tasks promises significant improvements in operational efficiency and cost reduction. AI\'s ability to detect and prevent fraudulent activities with greater precision strengthens the security framework of financial institutions. Moreover, AI\'s potential to offer personalized financial services tailored to individual needs can transform customer experiences and satisfaction. However, the potential problems of AI integration into finance cannot be overlooked. Ethical concerns regarding AI decision-making, especially in the context of biased outcomes, necessitate stringent oversight and ethical guidelines. The risk of security breaches and cyber-attacks on AI systems remains a persistent threat. Over-reliance on AI may undermine the value of human judgment and intuition in financial decisions. Additionally, regulatory challenges abound as financial institutions strive to keep pace with the rapid advancements in AI technology. The economic displacement resulting from AI-driven automation could lead to significant job losses, necessitating measures to manage the social and economic impact. Table of Contents ----------------- 1. **Introduction**\ 1.1. Definition of AI\ 1.2. Definition of Finance\ 1.3. Importance of AI in Finance 2. **AI in Finance: An Overview**\ 2.1. Evolution of AI in Finance\ 2.2. Key Technologies in AI for Finance\ 2.2.1. Machine Learning\ 2.2.2. Natural Language Processing\ 2.2.3. Robotic Process Automation\ 2.2.4. Blockchain and Cryptography 3. **Applications of AI in Finance**\ 3.1. Fraud Detection and Prevention\ 3.2. Risk Management\ 3.3. Personalized Banking and Customer Service\ 3.4. Investment and Wealth Management\ 3.5. Trading and Financial Market Predictions\ 3.6. Regulatory Compliance 4. **Challenges and Problems in AI and Finance**\ 4.1. Data Privacy and Security\ 4.2. Ethical and Legal Issues\ 4.3. Algorithmic Bias\ 4.4. Implementation Costs and Integration 5. **Solutions and Future Trends**\ 5.1. Enhanced Data Security Measures\ 5.2. Ethical AI Frameworks\ 5.3. Improved Algorithms for Bias Reduction\ 5.4. Cost-effective AI Solutions for Financial Institutions\ 5.5. Future Trends in AI and Finance 6. **Case Study: Zest Finance**\ 6.1 Overview of Zest Finance\ 6.2 Implementation of AI in Zest Finance\ 6.3 Impact and Results\ 6.4 Challenges Faced and Solutions\ 6.5 Lessons Learned 7. **Conclusion**\ 7.1. Summary of Key Findings\ 7.2. Impact of AI on the Future of Finance\ 7.3. Recommendations for Financial Institutions 8. **References** Introduction ------------ ### 1.1. Definition of AI Artificial Intelligence (AI) refers to the simulation of human intelligence processes by machines, especially computer systems. These processes include learning (the acquisition of information and rules for using the information), reasoning (using rules to reach approximate or definite conclusions), and self-correction. AI manifests in several forms such as machine learning, where systems can learn from data, and deep learning, which employs complex neural networks to analyze vast amounts of data. Essentially, AI enables machines to perform tasks that would typically require human intelligence, such as recognizing speech, making decisions, and translating languages. ### 1.2. Definition of Finance Finance is the management of money and includes activities such as investing, borrowing, lending, budgeting, saving, and forecasting. It is a broad term that encompasses various activities related to banking, leverage or debt, credit, capital markets, money, and investments. Finance can be divided into three main categories: personal finance, corporate finance, and public finance. Personal finance involves managing an individual's or household's financial activities, corporate finance deals with the financial operations of businesses, and public finance concerns the revenue and expenditure of government bodies. ### 1.3. Importance of AI in Finance The integration of AI into the financial sector has revolutionized the industry by enhancing efficiency, accuracy, and customer experience. AI\'s ability to process and analyze large amounts of data quickly and accurately helps financial institutions make more informed decisions. In fraud detection, AI algorithms can identify unusual patterns and flag potential fraudulent activities in real-time, thereby reducing financial losses. In risk management, AI can assess and predict risks more accurately than traditional methods, allowing for better-informed investment and lending decisions. AI also plays a crucial role in personalized banking and customer service. By analyzing customer data, AI can provide tailored financial advice and product recommendations, improving customer satisfaction and loyalty. In trading, AI systems can analyze market data and execute trades at high speeds, often outperforming human traders. Furthermore, AI helps in regulatory compliance by automating the monitoring and reporting processes, reducing the risk of human error, and ensuring adherence to regulatory standards. The importance of AI in finance lies in its ability to enhance operational efficiency, improve decision-making processes, provide better customer experiences, and ensure regulatory compliance, thereby driving the financial industry towards greater innovation and growth. AI in Finance: An Overview -------------------------- ### 2.1. Evolution of AI in Finance The evolution of Artificial Intelligence (AI) in finance has been a transformative journey, marked by significant milestones and technological advancements. Over the past few decades, AI has evolved from a theoretical concept to a critical tool that drives innovation and efficiency in the financial industry. #### 2.1.1. Early Beginnings The roots of AI in finance can be traced back to the 1980s when financial institutions began to experiment with early forms of AI, such as expert systems and rule-based systems. These systems were designed to emulate the decision-making abilities of human experts in specific domains. For instance, banks used expert systems to automate credit scoring and loan approval processes. However, these early systems were limited in their capabilities due to the lack of sophisticated algorithms and computational power. #### 2.1.2. The Rise of Machine Learning The 1990s and early 2000s saw the rise of machine learning, a subset of AI that focuses on the development of algorithms that can learn from and make predictions based on data. This period marked a significant shift from rule-based systems to data-driven approaches. Financial institutions began leveraging machine learning techniques for a variety of applications, such as algorithmic trading, fraud detection, and risk management. The ability of machine learning algorithms to analyze large datasets and identify patterns proved to be invaluable for financial decision-making. #### 2.1.3. Big Data and Advanced Analytics The 2010s ushered in the era of big data and advanced analytics. The exponential growth of digital data, coupled with advancements in computational power, enabled financial institutions to harness the full potential of AI. Machine learning models became more sophisticated, capable of handling vast amounts of structured and unstructured data. This period also saw the emergence of deep learning, a subset of machine learning that uses neural networks with many layers to model complex patterns. Deep learning has been particularly impactful in areas such as credit scoring, customer segmentation, and financial forecasting. #### 2.1.4. AI in Customer Service and Personalization In recent years, AI has significantly enhanced customer service and personalization in the financial sector. Chat bots and virtual assistants powered by natural language processing (NLP) have become commonplace, providing customers with 24/7 support and personalized financial advice. These AI-driven tools can handle a wide range of customer queries, from account inquiries to financial planning, thereby improving customer satisfaction and operational efficiency. #### 2.1.5. Regulatory Compliance and Risk Management AI has also played a crucial role in regulatory compliance and risk management. Financial institutions are subject to stringent regulatory requirements, and AI technologies have helped streamline compliance processes. AI algorithms can monitor transactions in real-time, detect suspicious activities, and generate compliance reports, reducing the risk of regulatory breaches. Additionally, AI-driven risk management systems can predict and mitigate potential risks, ensuring the stability and resilience of financial institutions. #### 2.1.6. The Future of AI in Finance Looking ahead, the future of AI in finance is poised to be even more transformative. Innovations such as explainable AI (XAI), which focuses on making AI decisions transparent and understandable, will address concerns about the opacity of AI models. Furthermore, advancements in quantum computing have the potential to revolutionize AI algorithms, enabling even more powerful and efficient financial solutions. The integration of AI with other emerging technologies, such as blockchain and the Internet of Things (IoT), will create new opportunities for innovation in the financial sector. As AI continues to evolve, it will drive the financial industry towards greater efficiency, innovation, and customer-centricity, shaping the future of finance in profound ways. ### 2.2. Key Technologies in AI for Finance AI in finance leverages a variety of advanced technologies to drive innovation, improve efficiency, and enhance decision-making. These technologies play crucial roles in various financial applications, from fraud detection to customer service. The key technologies in AI for finance include machine learning, natural language processing, robotic process automation, and blockchain and cryptography. #### 2.2.1. Machine Learning Machine Learning (ML) is a subset of AI that involves the development of algorithms that can learn from and make predictions based on data. In finance, ML is used extensively for tasks such as risk management, fraud detection, algorithmic trading, and credit scoring. ML models can analyze vast amounts of data to identify patterns and trends, enabling financial institutions to make data-driven decisions. For example, ML algorithms can detect fraudulent transactions by recognizing unusual patterns in transaction data. In algorithmic trading, ML models can analyze market data and execute trades at high speeds, often outperforming human traders. Additionally, ML is used in credit scoring to assess the creditworthiness of individuals and businesses by analyzing various data points such as credit history, income, and spending behavior. #### 2.2.2. Natural Language Processing Natural Language Processing (NLP) is a branch of AI that focuses on the interaction between computers and humans through natural language. In finance, NLP is used to analyze and interpret large volumes of unstructured text data, such as news articles, social media posts, and financial reports. This technology enables financial institutions to gain insights from textual data, such as identifying market sentiment or extracting relevant information from regulatory documents. NLP is also used in chat bots and virtual assistants to provide customer support and personalized financial advice. These AI-driven tools can understand and respond to customer queries in natural language, enhancing the customer experience and operational efficiency. #### 2.2.3. Robotic Process Automation Robotic Process Automation (RPA) involves the use of software robots or \"bots\" to automate repetitive and rule-based tasks. In finance, RPA is used to streamline various back-office processes, such as data entry, reconciliation, and compliance reporting. By automating these tasks, financial institutions can reduce operational costs, improve accuracy, and free up human resources for more strategic activities. RPA bots can work 24/7, ensuring that critical processes are completed efficiently and without delays. Additionally, RPA can be integrated with other AI technologies, such as ML and NLP, to enhance its capabilities and enable more complex automation solutions. #### 2.2.4. Blockchain and Cryptography Blockchain is a distributed ledger technology that enables secure and transparent record-keeping. In finance, blockchain is used for a variety of applications, including cross-border payments, trade finance, and digital identity verification. The decentralized nature of blockchain ensures that transactions are secure and tamper-proof, reducing the risk of fraud and enhancing trust among parties. Cryptography, which is the practice of securing information through encryption, plays a crucial role in blockchain technology. It ensures that data stored on the blockchain is secure and accessible only to authorized parties. Blockchain and cryptography are also used in the development of cryptocurrencies, such as Bitcoin and Ethereum, which have the potential to revolutionize the financial industry by enabling decentralized and peer-to-peer transactions. These key technologies in AI for finance are driving significant changes in the industry, enabling financial institutions to enhance their operations, improve decision-making, and provide better services to their customers. As AI continues to evolve, these technologies will play an increasingly important role in shaping the future of finance. Applications of AI in Finance ----------------------------- #### 3.1. Fraud Detection and Prevention Fraud detection and prevention are critical areas where AI has made significant strides. Traditional methods of detecting fraud often involve manual processes that are time-consuming and less effective in identifying sophisticated fraudulent activities. AI, particularly machine learning and deep learning, offers powerful tools to enhance the accuracy and efficiency of fraud detection systems. Machine learning algorithms can analyze vast amounts of transaction data to identify patterns and anomalies that may indicate fraudulent behavior. These algorithms learn from historical data and improve over time, becoming more adept at distinguishing between legitimate and fraudulent transactions. For instance, AI can detect unusual spending patterns, such as sudden large purchases or transactions in atypical locations, which may signal fraud. Deep learning models, with their ability to process complex datasets, can further enhance fraud detection capabilities. These models can analyze multiple variables simultaneously, such as transaction amount, location, time, and user behavior, to identify subtle indicators of fraud. Moreover, AI systems can operate in real-time, providing immediate alerts to financial institutions and enabling swift action to prevent fraud. AI also plays a crucial role in preventing fraud by enhancing security measures. For example, biometric authentication methods, such as facial recognition and fingerprint scanning, leverage AI to ensure secure access to financial services. These methods reduce the risk of identity theft and unauthorized access. Furthermore, AI-powered chatbots and virtual assistants can assist in educating customers about fraud prevention. They can provide real-time advice and alerts regarding suspicious activities, helping customers take proactive measures to protect their accounts. Overall, AI-driven fraud detection and prevention systems enhance the security of financial transactions, reduce financial losses, and build trust among customers and financial institutions. #### 3.2. Risk Management Risk management is another critical area where AI has a transformative impact. Financial institutions face various risks, including market risk, credit risk, operational risk, and liquidity risk. Traditional risk management methods often rely on historical data and static models, which may not accurately reflect current market conditions. AI offers dynamic and data-driven approaches to assess and manage risks more effectively. Machine learning algorithms are particularly valuable in risk management. These algorithms can analyze large datasets, including historical and real-time data, to identify potential risks and predict future trends. For instance, in credit risk management, AI can evaluate the creditworthiness of borrowers by analyzing their financial history, spending behavior, and other relevant factors. This allows financial institutions to make informed lending decisions and minimize the risk of defaults. In market risk management, AI can analyze market data, including stock prices, interest rates, and economic indicators, to predict market movements and identify potential risks. AI models can simulate various market scenarios and assess the impact on investment portfolios, enabling financial institutions to develop strategies to mitigate risks. AI also enhances operational risk management by automating processes and reducing the likelihood of human error. For example, AI-powered systems can monitor transactions for compliance with regulatory requirements, identify operational inefficiencies, and detect potential fraud. This reduces the risk of regulatory breaches and operational disruptions. Moreover, AI can improve liquidity risk management by providing real-time insights into cash flow and liquidity positions. AI models can analyze transaction data, predict cash flow patterns, and identify potential liquidity shortages. This enables financial institutions to take proactive measures to ensure adequate liquidity and avoid financial crises. The use of AI in risk management not only improves the accuracy and efficiency of risk assessment but also enables financial institutions to respond quickly to emerging risks. By leveraging AI technologies, financial institutions can enhance their risk management capabilities, ensure financial stability, and maintain a competitive edge in the market. #### 3.3. Personalized Banking and Customer Service Personalized banking and customer service have been significantly enhanced by the integration of AI technologies. Financial institutions are leveraging AI to provide tailored services and personalized experiences to their customers, thereby improving customer satisfaction and loyalty. AI-driven systems analyze vast amounts of customer data, including transaction history, spending patterns, and demographic information, to create personalized recommendations and offers. For example, AI algorithms can suggest relevant financial products, such as savings accounts or investment options, based on an individual\'s financial goals and risk tolerance. This personalized approach helps customers make informed decisions that align with their unique needs and preferences. Virtual assistants and chatbots powered by natural language processing (NLP) enable customers to interact with financial institutions through conversational interfaces. These AI-driven tools can handle a wide range of customer inquiries, from account inquiries to loan applications, in real-time and with high accuracy. Virtual assistants can provide instant responses to customer queries, offer personalized financial advice, and assist in completing transactions, enhancing convenience and accessibility for customers. Moreover, AI enhances customer service by predicting customer behavior and anticipating their needs. For example, AI models can analyze customer interactions and predict when a customer may need assistance or when they are likely to churn. Financial institutions can proactively reach out to customers with personalized offers or solutions, fostering stronger relationships and reducing customer attrition. In essence, AI-powered personalized banking and customer service transform the customer experience by providing tailored recommendations, seamless interactions, and proactive support, ultimately driving customer satisfaction and retention. #### 3.4. Investment and Wealth Management AI technologies are revolutionizing investment and wealth management by providing sophisticated tools and insights that enable financial institutions and investors to make informed decisions and optimize portfolio performance. Machine learning algorithms analyze historical and real-time market data to identify investment opportunities and predict market trends. These algorithms can assess large datasets, including stock prices, economic indicators, and company performance metrics, to identify patterns and correlations that traditional methods may overlook. For instance, AI can analyze news sentiment and social media trends to gauge market sentiment and inform investment strategies. AI-powered robo-advisors offer automated investment advice based on individual investor profiles, financial goals, and risk preferences. Robo-advisors use algorithms to create diversified investment portfolios and rebalance them periodically to maintain optimal asset allocation. This automated approach lowers costs, enhances efficiency, and provides access to investment opportunities that were traditionally reserved for high-net-worth individuals. Furthermore, AI enhances wealth management by offering personalized financial planning and advisory services. AI algorithms can analyze an individual\'s financial situation, goals, and risk tolerance to develop customized wealth management strategies. These strategies may include retirement planning, tax optimization, estate planning, and asset allocation recommendations tailored to the client\'s specific needs. #### 3.5. Trading and Financial Market Predictions AI has revolutionized trading and financial market predictions by providing powerful tools to analyze market data, identify patterns, and make data-driven decisions in real-time. Machine learning algorithms analyze vast amounts of historical and real-time market data, including stock prices, trading volumes, and market indicators, to identify patterns and trends. These algorithms can detect subtle correlations and anomalies that human traders may overlook, enabling more accurate predictions of market movements. For instance, AI can analyze market sentiment from news articles and social media posts to gauge investor sentiment and predict market trends. Algorithmic trading, also known as automated trading, uses AI algorithms to execute trades at high speeds and frequencies based on predefined criteria. These algorithms can react to market changes instantaneously, capitalize on arbitrage opportunities, and manage risk more effectively than traditional trading methods. AI-powered trading systems can operate 24/7, ensuring that trades are executed swiftly and efficiently across global markets. Moreover, AI enhances financial market predictions by incorporating advanced techniques such as deep learning and natural language processing (NLP). Deep learning models, with their ability to process complex data and learn hierarchical representations, can further improve the accuracy of market predictions. NLP algorithms can analyze textual data, such as financial news and analyst reports, to extract insights and sentiment that influence market behavior. Overall, AI-driven trading and financial market predictions empower financial institutions and investors with advanced analytics, automated trading strategies, and real-time insights, thereby optimizing trading performance and enhancing competitiveness in the global markets. #### 3.6. Regulatory Compliance Regulatory compliance is a critical aspect of the financial industry, and AI technologies are playing an increasingly important role in helping financial institutions adhere to regulatory requirements efficiently and effectively. AI-powered systems can automate regulatory compliance processes, such as monitoring transactions, detecting suspicious activities, and generating compliance reports. Machine learning algorithms can analyze vast amounts of transaction data in real-time to identify anomalies and potential instances of fraud or money laundering. These algorithms can flag suspicious transactions for further investigation, enabling financial institutions to mitigate compliance risks and adhere to anti-money laundering (AML) regulations. Natural language processing (NLP) algorithms can also assist in regulatory compliance by analyzing and interpreting regulatory texts, such as laws, regulations, and compliance guidelines. NLP-powered systems can extract relevant information, identify compliance obligations, and ensure that financial institutions are adhering to regulatory standards in their operations. Moreover, AI technologies enable proactive compliance monitoring and risk management by providing real-time insights into regulatory changes and updates. AI systems can analyze regulatory trends and anticipate potential compliance issues, allowing financial institutions to adjust their policies and procedures accordingly. Overall, AI in regulatory compliance enhances the efficiency, accuracy, and transparency of compliance processes, enabling financial institutions to maintain regulatory compliance, mitigate risks, and build trust with regulators and stakeholders alike. Challenges and Problems in AI and Finance ----------------------------------------- #### 4.1. Data Privacy and Security Data privacy and security represent significant challenges in the integration of AI technologies within the financial sector. As financial institutions increasingly rely on AI for data-driven decision-making and customer service, ensuring the confidentiality, integrity, and availability of sensitive financial data becomes paramount. Financial institutions collect and process vast amounts of personal and financial information from customers, including bank account details, credit card information, and transaction histories. This sensitive data is attractive to cybercriminals seeking to exploit vulnerabilities in AI systems or traditional IT infrastructures. AI systems themselves can introduce new security risks. For instance, machine learning models trained on sensitive data may inadvertently reveal confidential information through model inversion attacks or membership inference attacks. These attacks exploit weaknesses in AI algorithms to extract sensitive data about individuals or transactions. Moreover, AI-powered systems that use third-party data sources or cloud-based services may face additional security challenges. Financial institutions must ensure that data transfers and storage comply with regulatory requirements, such as the General Data Protection Regulation (GDPR) or the Payment Card Industry Data Security Standard (PCI DSS). To address these challenges, financial institutions are implementing robust data privacy and security measures, including: - **Encryption**: Encrypting sensitive data both at rest and in transit to protect it from unauthorized access. - **Access Controls**: Implementing strict access controls and authentication mechanisms to limit access to sensitive data based on user roles and permissions. - **Anonymization and Masking**: Applying techniques such as anonymization and data masking to de-identify sensitive data used for AI model training and testing. - **Monitoring and Auditing**: Continuously monitoring AI systems and conducting regular security audits to detect and mitigate potential vulnerabilities and breaches. - **Compliance and Governance**: Establishing comprehensive data governance frameworks and ensuring compliance with regulatory requirements to protect customer data and uphold trust. By addressing data privacy and security concerns proactively, financial institutions can leverage AI technologies to drive innovation and improve operational efficiency while maintaining the confidentiality and trust of their customers. #### 4.2. Ethical and Legal Issues The integration of AI technologies in finance raises various ethical and legal concerns that require careful consideration and regulation to ensure responsible and fair use of AI systems. **Ethical Issues:** 1. **Bias and Fairness**: AI algorithms can inadvertently perpetuate biases present in training data, leading to discriminatory outcomes in financial decisions such as lending or insurance. It is crucial to mitigate biases through responsible data collection, diverse training datasets, and algorithmic fairness assessments. 2. **Transparency**: The opacity of AI algorithms can make it challenging to understand how decisions are made, especially in sensitive financial matters. Ensuring transparency in AI systems, known as explainable AI (XAI), is essential for accountability and trustworthiness. 3. **Accountability and Liability**: Determining accountability and liability for decisions made by AI systems, particularly in cases of errors or failures, raises complex ethical questions. Clear frameworks for accountability and recourse are needed to address these issues responsibly. 4. **Privacy Concerns**: AI systems that process personal data for profiling or decision-making must uphold stringent data protection standards to safeguard individuals\' privacy rights. Compliance with regulations such as GDPR is crucial to mitigate privacy risks. **Legal Issues:** 1. **Regulatory Compliance**: Financial institutions must navigate a complex regulatory landscape when deploying AI technologies, ensuring compliance with sector-specific regulations (e.g., GDPR, PCI DSS) and guidelines issued by regulatory bodies (e.g., SEC, FINRA). 2. **Data Governance**: Establishing robust data governance frameworks is essential to manage data privacy, security, and compliance requirements effectively. Financial institutions must ensure that AI systems adhere to data protection principles and regulatory standards. 3. **Intellectual Property**: Issues related to intellectual property rights may arise concerning AI algorithms, data ownership, and proprietary technologies developed by financial institutions or third-party vendors. 4. **Consumer Protection**: Protecting consumer rights and ensuring fair treatment in AI-driven financial services, such as personalized banking and algorithmic decision-making, requires regulatory oversight and enforcement mechanisms. Addressing ethical and legal challenges in AI and finance requires collaboration between industry stakeholders, policymakers, and regulatory bodies to develop clear guidelines, standards, and best practices. By promoting responsible AI deployment and adherence to ethical principles, financial institutions can leverage AI technologies effectively while upholding trust, fairness, and regulatory compliance. #### 4.3. Algorithmic Bias Algorithmic bias is a critical issue in the deployment of AI technologies within the financial sector, potentially leading to unfair or discriminatory outcomes in financial decision-making processes. **Understanding Algorithmic Bias:** Algorithmic bias refers to systematic and unfair discrimination that can occur when AI algorithms exhibit biases in their predictions or decisions. These biases may stem from several factors, including: - **Biased Training Data**: AI algorithms learn from historical data, which may reflect societal biases and inequalities. If training data is not diverse or representative, algorithms may perpetuate biases present in the data. - **Algorithm Design**: The design and implementation of AI algorithms, including feature selection and model architecture, can inadvertently introduce biases. For example, using proxies for sensitive attributes (e.g., zip code as a proxy for race) can lead to discriminatory outcomes. - **Feedback Loops**: Biased decisions made by AI systems can reinforce existing inequalities and biases in the data, creating feedback loops that perpetuate discriminatory outcomes over time. **Implications in Finance:** In the financial industry, algorithmic bias can manifest in various ways, impacting decisions related to: - **Lending and Credit Scoring**: AI-driven credit scoring models may unfairly disadvantage certain demographic groups based on factors such as race, gender, or socioeconomic status. - **Insurance Pricing**: Algorithms used in insurance pricing may inadvertently discriminate against individuals based on factors unrelated to risk, such as zip code or occupation. - **Investment Decisions**: AI-powered investment algorithms may favor certain types of investments or asset classes, potentially excluding opportunities that could benefit diverse investors. **Addressing Algorithmic Bias:** Addressing algorithmic bias requires proactive measures and ongoing efforts to promote fairness, transparency, and accountability in AI systems: 1. **Diverse and Representative Data**: Ensuring that training data used to develop AI algorithms is diverse and representative of the population it serves can help mitigate biases. 2. **Bias Detection and Mitigation**: Implementing techniques to detect and mitigate biases in AI models, such as fairness-aware machine learning algorithms and bias testing frameworks. 3. **Explainable AI (XAI)**: Enhancing transparency and interpretability of AI algorithms to understand how decisions are made and identify potential biases (e.g., using model interpretability techniques). 4. **Ethical Guidelines and Governance**: Establishing ethical guidelines and governance frameworks for the development, deployment, and monitoring of AI systems to ensure compliance with fairness and non-discrimination principles. 5. **Regular Audits and Monitoring**: Conducting regular audits and ongoing monitoring of AI systems to identify and address biases that may emerge over time. By addressing algorithmic bias proactively and adopting responsible AI practices, financial institutions can leverage AI technologies to enhance decision-making processes while promoting fairness, equity, and trust among customers and stakeholders. #### 4.4. Implementation Costs and Integration The implementation of AI technologies in the financial sector presents significant challenges related to costs, integration with existing systems, and the allocation of resources necessary for successful deployment. **Cost Considerations:** Implementing AI technologies in finance involves substantial upfront investments in technology infrastructure, software development, and talent acquisition. Financial institutions must allocate resources for: - **Technology Infrastructure**: Upgrading hardware and software systems to support AI applications, including high-performance computing resources and cloud infrastructure. - **Software Development**: Developing and customizing AI algorithms and applications tailored to specific financial tasks, such as risk management, fraud detection, and customer service. - **Data Acquisition and Preparation**: Acquiring high-quality data sets and preparing data for AI model training and testing, which may involve data cleansing, normalization, and augmentation. - **Talent Acquisition**: Hiring skilled data scientists, AI engineers, and domain experts with expertise in finance and AI technologies. - **Training and Education**: Providing training programs and upskilling initiatives to existing staff to foster AI literacy and ensure effective use of AI tools and platforms. **Integration Challenges:** Integrating AI technologies with existing IT systems and workflows poses several challenges, including: - **Compatibility**: Ensuring compatibility and interoperability between AI solutions and legacy IT systems, which may require system upgrades or middleware solutions. - **Data Integration**: Integrating AI-driven insights and predictions into existing decision-making processes and operational workflows without disrupting business continuity. - **Regulatory Compliance**: Ensuring that AI implementations comply with industry regulations and data protection laws, such as GDPR or PCI DSS, which may require additional compliance measures and audits. - **Change Management**: Managing organizational change and resistance to AI adoption among stakeholders, including employees, customers, and regulatory authorities. **Risk Mitigation Strategies:** To mitigate implementation costs and integration challenges, financial institutions can consider the following strategies: - **Pilot Projects**: Conducting pilot projects to evaluate the feasibility and effectiveness of AI applications in specific use cases before scaling implementation. - **Collaboration and Partnerships**: Collaborating with fintech startups, technology vendors, or academic institutions to leverage expertise and resources in AI innovation. - **Cloud-Based Solutions**: Adopting cloud-based AI solutions and platforms that offer scalability, flexibility, and cost-effectiveness in deploying AI applications. - **Outsourcing**: Outsourcing certain AI development and implementation tasks to third-party vendors or consultants with specialized expertise in AI and finance. - **Continuous Evaluation and Improvement**: Establishing processes for continuous evaluation, monitoring, and improvement of AI systems to ensure alignment with business goals and regulatory requirements. By carefully planning and strategizing the implementation of AI technologies, financial institutions can unlock the potential benefits of AI while managing costs, minimizing risks, and achieving sustainable integration into their operations and services. Recommendations for Implementing AI in Finance ---------------------------------------------- #### 5.1. Enhanced Data Security Measures Enhancing data security measures is crucial when implementing AI technologies in the financial sector to protect sensitive information and mitigate cybersecurity risks. **Importance of Enhanced Data Security:** In the context of AI implementation, financial institutions handle vast amounts of sensitive data, including personal information, transaction records, and financial details. Robust data security measures are essential to safeguard this information from unauthorized access, data breaches, and cyber threats. **Key Data Security Measures:** 1. **Encryption**: Encrypting sensitive data both at rest and in transit using strong encryption algorithms to protect it from unauthorized access and interception. 2. **Access Controls**: Implementing stringent access controls and authentication mechanisms to ensure that only authorized personnel have access to sensitive data and AI systems. 3. **Data Masking and Anonymization**: Employing techniques such as data masking and anonymization to de-identify sensitive data used for AI model training and testing, reducing the risk of exposure. 4. **Secure APIs and Interfaces**: Securing application programming interfaces (APIs) and interfaces used to exchange data between AI systems and other IT systems to prevent malicious attacks and data leaks. 5. **Monitoring and Auditing**: Continuously monitoring AI systems and data access activities for suspicious behavior or anomalies and conducting regular audits to detect and mitigate potential security vulnerabilities. 6. **Compliance with Regulations**: Ensuring compliance with industry regulations and data protection laws, such as GDPR, PCI DSS, and specific financial regulations, by implementing appropriate security controls and practices. 7. **Employee Training and Awareness**: Providing regular training programs and raising awareness among employees about data security best practices, cybersecurity threats, and the importance of maintaining data confidentiality. **Integration with AI Implementation:** When integrating AI technologies into financial operations, these data security measures should be integrated into the design, development, and deployment phases of AI systems. It is essential to collaborate with cybersecurity experts and IT professionals to assess risks, implement security controls effectively, and ensure that AI solutions meet industry standards for data protection and privacy. By prioritizing enhanced data security measures, financial institutions can build trust with customers, protect sensitive information from cyber threats, and mitigate risks associated with AI implementation in the financial sector. These measures not only safeguard data integrity but also uphold regulatory compliance and enhance overall cybersecurity resilience. #### 5.2. Ethical AI Frameworks Establishing ethical AI frameworks is essential for ensuring responsible and fair deployment of AI technologies in the financial sector, promoting transparency, accountability, and ethical decision-making. **Importance of Ethical AI Frameworks:** As AI technologies become increasingly integrated into financial operations, ethical considerations are paramount to address potential biases, ensure fairness in decision-making, and uphold societal values. Ethical AI frameworks provide guidelines and principles to guide the development, deployment, and use of AI systems in a manner that aligns with ethical standards and promotes public trust. **Key Components of Ethical AI Frameworks:** 1. **Fairness and Non-Discrimination**: Ensuring that AI algorithms do not exhibit biases or discriminate against individuals or groups based on protected attributes such as race, gender, or socioeconomic status. Implementing fairness-aware machine learning techniques and conducting bias testing are critical steps to achieve fairness in AI systems. 2. **Transparency and Explainability**: Enhancing transparency in AI systems by making their decisions and operations understandable and explainable to stakeholders, including customers, regulators, and employees. Employing techniques for explainable AI (XAI) helps users understand how AI algorithms arrive at decisions and identify potential biases or errors. 3. **Accountability and Responsibility**: Clarifying roles and responsibilities for AI development, deployment, and monitoring, including mechanisms for accountability in case of AI-related errors or failures. Establishing governance structures and protocols for overseeing AI systems\' compliance with ethical guidelines and regulatory requirements. 4. **Privacy and Data Protection**: Upholding stringent data protection measures to safeguard individuals\' privacy rights and ensure secure handling of sensitive data used in AI training and operations. Adhering to data protection laws and regulations (e.g., GDPR) and implementing privacy-preserving technologies such as differential privacy and federated learning. 5. **Human-Centered Design**: Prioritizing human values and welfare in the design and implementation of AI systems, considering the potential societal impacts and ethical implications of AI applications in finance. Involving diverse stakeholders, including ethicists, social scientists, and affected communities, in the development and evaluation of AI frameworks. **Implementation Strategies:** - **Ethics Review Boards**: Establishing ethics review boards or committees within financial institutions to oversee AI projects, assess ethical risks, and provide guidance on ethical decision-making. - **Ethics by Design**: Incorporating ethical considerations into the entire AI development lifecycle, from initial design and data collection to deployment and ongoing monitoring. Conducting ethical impact assessments and risk analyses to identify and mitigate potential ethical risks. - **Stakeholder Engagement**: Engaging with stakeholders, including customers, employees, regulators, and advocacy groups, to solicit feedback, address concerns, and promote transparency in AI practices. - **Continuous Learning and Adaptation**: Promoting a culture of continuous learning and adaptation to evolving ethical standards, technological advancements, and regulatory requirements in AI governance. By adopting ethical AI frameworks and integrating ethical considerations into AI deployment strategies, financial institutions can foster responsible innovation, mitigate ethical risks, and build trust with stakeholders. Ethical AI frameworks not only ensure compliance with regulatory requirements but also uphold ethical principles that promote fairness, transparency, and societal well-being in AI-driven finance. #### 5.3. Improved Algorithms for Bias Reduction Improving algorithms for bias reduction is critical in ensuring fair and equitable outcomes in AI-driven financial applications, addressing potential biases that may arise from historical data or algorithmic design. **Challenges in Bias Reduction:** Bias in AI algorithms can stem from various sources, including biased training data, algorithmic design choices, and societal biases embedded in data collection processes. These biases can result in unfair treatment or discrimination against certain demographic groups in financial decision-making, such as lending, insurance pricing, or investment recommendations. **Strategies for Bias Reduction:** 1. **Diverse and Representative Training Data**: Ensuring that training data used to develop AI algorithms is diverse, representative, and balanced across demographic groups. This approach helps mitigate biases by providing a more comprehensive view of the population being served. 2. **Bias Detection and Measurement**: Implementing techniques to detect, measure, and quantify biases in AI algorithms, such as fairness metrics and bias testing frameworks. These tools assess the fairness of algorithmic outcomes across different demographic groups and identify areas where biases may exist. 3. **Fairness-Aware Machine Learning**: Integrating fairness-aware machine learning techniques into AI model development to explicitly optimize for fairness objectives. These techniques adjust algorithmic predictions to minimize disparate impact and ensure equitable treatment for all individuals. 4. **Counterfactual Fairness**: Adopting counterfactual fairness approaches that simulate alternative scenarios to evaluate how changes in input data or algorithmic decisions affect fairness outcomes. This method helps mitigate biases by considering hypothetical changes in decision-making processes. 5. **Regular Monitoring and Evaluation**: Continuously monitoring AI systems in production and evaluating their performance for fairness and bias reduction. Implementing feedback loops and corrective measures to address biases as they arise and evolve over time. **Ethical Considerations and Transparency:** Ensuring transparency in the use of bias reduction techniques and communicating the rationale behind algorithmic decisions to stakeholders. Employing explainable AI (XAI) methods to provide insights into how algorithms operate and mitigate biases, enhancing trust and accountability in AI-driven financial services. **Collaboration and Industry Standards:** Collaborating with industry partners, academia, and regulatory bodies to develop industry standards, guidelines, and best practices for bias reduction in AI. Sharing knowledge and insights to advance research and innovation in ethical AI practices within the financial sector. By leveraging improved algorithms for bias reduction and adopting robust strategies to promote fairness and transparency, financial institutions can enhance the reliability, equity, and societal impact of AI applications in finance. These efforts not only mitigate ethical risks but also contribute to building trust among customers, regulators, and the broader community in AI-driven financial services. #### 5.4. Cost-effective AI Solutions for Financial Institutions Implementing cost-effective AI solutions is crucial for financial institutions to maximize operational efficiency, improve decision-making processes, and achieve sustainable AI adoption within budget constraints. **Cost-effective Strategies:** 1. **Cloud-based AI Solutions**: Leveraging cloud computing platforms and AI-as-a-Service (AIaaS) offerings to reduce upfront infrastructure costs and scale AI capabilities based on demand. Cloud solutions offer flexibility, scalability, and cost-efficiency in deploying AI applications without significant capital expenditures. 2. **Open-source AI Tools and Libraries**: Utilizing open-source AI tools, frameworks (e.g., TensorFlow, PyTorch), and libraries (e.g., scikit-learn) to develop and deploy AI models at lower costs. Open-source communities provide access to cutting-edge algorithms and resources for building customized AI solutions without licensing fees. 3. **Automation and Robotic Process Automation (RPA)**: Integrating AI-driven automation and RPA solutions to streamline repetitive tasks, reduce manual intervention, and optimize resource allocation. Automation improves operational efficiency, minimizes errors, and frees up human resources for strategic tasks. 4. **Data-driven Decision-making**: Harnessing AI-powered analytics and predictive modeling to derive actionable insights from large volumes of data. Data-driven decision-making enhances accuracy, identifies cost-saving opportunities, and improves risk management practices in financial operations. 5. **Collaboration with Fintech Startups**: Partnering with fintech startups and technology vendors specializing in AI solutions to access innovative technologies, expertise, and cost-effective solutions tailored to financial industry needs. Collaborations facilitate knowledge exchange, reduce development costs, and accelerate AI adoption. 6. **Training and Upskilling**: Investing in training programs and upskilling initiatives for existing staff to build AI capabilities internally. Developing a skilled workforce proficient in AI technologies enhances innovation, reduces reliance on external vendors, and optimizes long-term operational costs. **ROI and Long-term Benefits:** Measuring return on investment (ROI) and long-term benefits of AI implementations, such as cost savings, efficiency gains, and competitive advantages. Conducting pilot projects, evaluating performance metrics, and aligning AI initiatives with strategic business objectives to maximize financial returns and sustainability. #### 5.5. Future Trends in AI and Finance The future of AI in finance is poised for transformative advancements, shaping industry trends and revolutionizing financial services through innovation, automation, and enhanced customer experiences. **Emerging Trends:** 1. **Advanced Predictive Analytics**: Advancements in AI-powered predictive analytics and machine learning models for real-time decision-making, personalized financial services, and risk management strategies. 2. **Natural Language Processing (NLP)**: Integration of NLP technologies for sentiment analysis, customer service automation, and regulatory compliance through automated document processing and interpretation. 3. **Blockchain and AI Integration**: Convergence of blockchain technology with AI algorithms to enhance security, transparency, and efficiency in digital transactions, smart contracts, and decentralized finance (DeFi) applications. 4. **AI-driven Customer Insights**: Utilization of AI-driven analytics to derive actionable customer insights, enhance segmentation strategies, and deliver personalized financial products and services based on individual preferences and behavior patterns. 5. **Ethical AI and Regulatory Compliance**: Implementation of ethical AI frameworks, transparency measures, and regulatory compliance standards to mitigate biases, ensure fairness, and uphold consumer protection in AI-driven financial decisions. 6. **AI-powered Cybersecurity**: Development of AI-driven cybersecurity solutions for threat detection, anomaly detection, and proactive risk management to safeguard sensitive financial data and mitigate cyber threats. **Impact on Financial Institutions:** - **Operational Efficiency**: AI technologies streamline processes, automate tasks, and optimize resource allocation, reducing costs and improving operational efficiency across financial operations. - **Customer Experience**: Enhanced customer interactions, personalized services, and seamless digital experiences through AI-driven insights and predictive analytics, fostering customer loyalty and satisfaction. - **Risk Management**: AI-powered algorithms enhance risk assessment models, fraud detection capabilities, and regulatory compliance measures, strengthening financial institutions\' resilience to market volatility and regulatory changes. **Case Study: Zest Finance** **6.1 Overview of Zest Finance** ZestFinance, founded by Douglas Merrill in 2009, is a financial technology company that specializes in utilizing artificial intelligence and machine learning to make better credit decisions. Located in Los Angeles, California, ZestFinance initially aimed to address the challenges of credit scoring and lending using innovative AI-driven models. Unlike traditional credit scoring methods that rely heavily on limited data points such as credit history and income, ZestFinance pioneered the use of alternative data sources and advanced machine learning algorithms to assess creditworthiness. This approach allows ZestFinance to provide more accurate risk assessments, particularly for underserved populations who may have thin or no credit files. Over the years, ZestFinance has expanded its services to include not only credit scoring but also fraud detection, loan underwriting, and personalized financial recommendations. By leveraging vast amounts of data and sophisticated AI techniques, ZestFinance continues to enhance financial inclusion and improve access to affordable credit for individuals and businesses. The company\'s commitment to innovation in AI and finance has positioned it as a leader in the fintech industry, with a strong focus on transparency, ethical AI practices, and regulatory compliance. ZestFinance\'s success underscores the transformative impact of AI in reshaping traditional financial services and promoting more inclusive economic opportunities. **6.2 Implementation of AI in Zest Finance** Zest Finance has strategically implemented artificial intelligence (AI) across various aspects of its operations, revolutionizing how it approaches credit scoring, risk management, and customer service in the financial sector. **Credit Scoring and Risk Assessment:** At the core of Zest Finance\'s AI implementation is its advanced credit scoring models. Unlike traditional methods that rely solely on credit history and income, Zest Finance utilizes machine learning algorithms to analyze a broader range of data points. This includes non-traditional data such as online behavior, social media activity, and even utility bill payments. By integrating these diverse datasets, Zest Finance generates more accurate risk assessments, enabling better-informed lending decisions. This approach not only expands access to credit for individuals with limited credit histories but also enhances predictive accuracy, reducing the risk of default. **Fraud Detection and Prevention:** Another critical area where AI has been deployed at Zest Finance is in fraud detection and prevention. Machine learning algorithms continuously analyze transactions, identifying patterns and anomalies that may indicate fraudulent activities. By leveraging real-time data processing and anomaly detection techniques, Zest Finance enhances its ability to detect and mitigate fraudulent transactions promptly. This proactive approach not only safeguards financial resources but also reinforces trust and security among customers. **Operational Efficiency and Customer Service:** AI technologies have also been instrumental in improving operational efficiency and customer service at Zest Finance. Automated processes, powered by AI-driven robotic process automation (RPA), streamline routine tasks such as document processing, customer inquiries, and loan approvals. Natural language processing (NLP) capabilities enable Zest Finance to provide personalized customer interactions, responding to queries and offering financial advice in real-time. This not only enhances overall service delivery but also boosts customer satisfaction and retention. **Ethical Considerations and Compliance:** In implementing AI, Zest Finance prioritizes ethical considerations and regulatory compliance. The company adheres to stringent data privacy laws and regulations, ensuring secure handling and storage of sensitive customer information. Transparent AI frameworks are employed to explain the rationale behind credit decisions, promoting fairness and accountability. Regular audits and internal controls further validate the accuracy and reliability of AI models, aligning with industry best practices and regulatory standards. **Impact and Innovation:** The implementation of AI at Zest Finance has had a profound impact on its business operations and market positioning. By harnessing AI\'s predictive capabilities and data-driven insights, Zest Finance has achieved significant improvements in risk management, operational efficiency, and customer experience. This innovative approach not only drives financial inclusion but also sets a benchmark for AI-driven fintech solutions in the industry. This detailed overview highlights how Zest Finance has effectively integrated AI into its operations, emphasizing its transformative impact on credit scoring, fraud detection, operational efficiency, and regulatory compliance. Let me know if there are specific aspects you\'d like to delve deeper into or if there are additional points to cover. **6.3 Impact and Results** The implementation of artificial intelligence (AI) at Zest Finance has yielded significant impacts and measurable results across several key areas of its operations and market influence. **Enhanced Credit Decision Making:** One of the primary impacts of AI implementation at Zest Finance has been in enhancing credit decision-making processes. By leveraging advanced machine learning algorithms, Zest Finance has improved the accuracy and reliability of its credit scoring models. This has enabled the company to extend credit to a broader range of customers, including those with limited credit histories or unconventional financial profiles. The AI-driven approach has led to more precise risk assessments, reducing default rates and optimizing loan pricing strategies to better reflect individual borrower risk. **Improved Operational Efficiency:** AI technologies have also driven improvements in operational efficiency at Zest Finance. Automation of routine tasks through robotic process automation (RPA) and AI-driven algorithms has streamlined loan processing, customer service interactions, and backend operations. This efficiency gain not only reduces operational costs but also accelerates turnaround times for loan approvals and customer inquiries. As a result, Zest Finance has been able to handle larger volumes of transactions with greater speed and accuracy, enhancing overall productivity and scalability. **Enhanced Fraud Detection and Security:** The implementation of AI has significantly bolstered Zest Finance\'s capabilities in fraud detection and security. Machine learning algorithms continuously analyze transactional data in real-time, detecting fraudulent patterns and anomalies that human review may overlook. This proactive approach has minimized financial losses due to fraudulent activities, safeguarded customer assets, and reinforced trust in Zest Finance\'s security measures. By leveraging AI-powered predictive analytics, Zest Finance stays ahead of evolving fraud tactics and adapts its detection strategies to mitigate risks effectively. **Customer Experience and Satisfaction:** AI-driven enhancements have also positively impacted customer experience at Zest Finance. Personalized banking services, powered by natural language processing (NLP) and AI-driven recommendations, provide tailored financial advice and support to customers in real-time. Automated processes ensure prompt responses to customer inquiries and loan applications, reducing wait times and improving service accessibility. These improvements in customer service contribute to higher satisfaction rates, increased customer retention, and positive word-of-mouth referrals, enhancing Zest Finance\'s competitive edge in the market. **Strategic Insights and Decision Support:** Furthermore, AI implementation has empowered Zest Finance with actionable insights and decision support capabilities. Advanced analytics and predictive modeling enable data-driven decision-making across strategic areas such as loan portfolio management, risk assessment, and market forecasting. By harnessing AI to analyze vast amounts of data, Zest Finance gains valuable insights into customer behavior, market trends, and economic conditions, informing proactive business strategies and fostering sustainable growth. **6.4 Challenges Faced and Solutions** Implementing AI at ZestFinance has brought numerous benefits, but it also came with its share of challenges. Addressing these challenges effectively has been crucial for ensuring the success and sustainability of AI-driven initiatives. **Challenge 1: Data Privacy and Security** One of the primary challenges in implementing AI at ZestFinance was ensuring data privacy and security. Given the sensitive nature of financial data, protecting customer information from breaches and unauthorized access was paramount. **Solution: Enhanced Security Measures** To address this, ZestFinance implemented robust encryption protocols and secure data storage solutions. Regular security audits and compliance with industry standards like GDPR and CCPA were enforced to maintain high levels of data protection. Additionally, advanced threat detection systems were deployed to monitor and respond to potential security breaches in real-time. **Challenge 2: Ethical and Legal Issues** The ethical use of AI and adherence to legal regulations posed another significant challenge. Ensuring that AI algorithms operated fairly and transparently without discriminating against certain groups was essential for maintaining trust and compliance with legal standards. **Solution: Ethical AI Frameworks** ZestFinance developed an ethical AI framework to guide the development and deployment of its AI systems. This included conducting bias audits, implementing fairness constraints in machine learning models, and providing transparency in decision-making processes. Collaborations with legal experts and regulatory bodies ensured that the AI systems complied with all relevant laws and regulations. **Challenge 3: Algorithmic Bias** Algorithmic bias, where AI systems might inadvertently favor or disadvantage certain groups, was a critical challenge that ZestFinance needed to address to ensure fairness and equity in its credit scoring models. **Solution: Improved Algorithms for Bias Reduction** To mitigate algorithmic bias, ZestFinance invested in research and development to enhance its machine learning models. Techniques such as reweighting and re-sampling of training data were used to ensure balanced representation. Regular bias testing and model validation were conducted to detect and correct any biases that emerged over time. **Challenge 4: Implementation Costs and Integration** The high costs associated with implementing AI technologies and integrating them into existing systems presented another challenge. This included expenses related to acquiring advanced hardware, software, and skilled personnel. **Solution: Cost-effective AI Solutions** ZestFinance adopted a phased approach to AI implementation, starting with pilot projects to validate the effectiveness of AI solutions before full-scale deployment. Leveraging cloud-based AI services reduced the need for expensive on-premise infrastructure. Additionally, partnerships with AI vendors and technology providers helped in accessing cutting-edge technologies at more manageable costs. Cross-functional teams were formed to ensure smooth integration of AI systems with existing processes and technologies. **Challenge 5: Talent Acquisition and Training** Securing skilled AI professionals and ensuring that existing staff were adequately trained to work with new AI systems was a significant challenge. **Solution: Focused Talent Strategy and Training Programs** ZestFinance addressed this by developing a comprehensive talent acquisition strategy, partnering with universities and tech institutions to attract top AI talent. In-house training programs and continuous learning initiatives were established to upskill current employees, ensuring they were well-equipped to work alongside AI technologies. **6.5 Lessons Learned** The implementation of AI at ZestFinance has provided valuable insights and lessons that can guide future initiatives in the financial technology sector. These lessons emphasize the importance of strategic planning, ethical considerations, and continuous improvement in leveraging AI for financial services. **Lesson 1: The Importance of Data Quality and Diversity** One of the key lessons learned is the critical role of data quality and diversity in developing effective AI models. High-quality data that accurately represents the diverse demographics of potential borrowers is essential for creating reliable and unbiased AI systems. **Action Taken: Rigorous Data Management Practices** ZestFinance implemented rigorous data management practices, including regular data cleaning, validation, and updating procedures. They also ensured that the data used for training AI models was diverse and representative of the entire customer base, helping to minimize biases and improve model accuracy. **Lesson 2: The Need for Transparent and Explainable AI** Transparency and explainability in AI models are crucial for gaining trust from stakeholders and ensuring compliance with regulatory standards. Users and regulators need to understand how AI models make decisions, especially in high-stakes areas like credit scoring. **Action Taken: Development of Explainable AI Models** To address this, ZestFinance invested in developing explainable AI models that provide clear insights into the decision-making processes. They created tools and frameworks to interpret model outputs and explain the rationale behind credit decisions to customers and regulators. **Lesson 3: Balancing Innovation with Ethical Standards** The balance between technological innovation and ethical standards is essential for the sustainable deployment of AI. Ensuring that AI systems operate ethically and do not inadvertently harm certain groups is a significant responsibility. **Action Taken: Establishment of Ethical Guidelines** ZestFinance established comprehensive ethical guidelines and frameworks to govern the use of AI. They conducted regular ethical audits and involved diverse teams in the development process to ensure that different perspectives were considered and potential ethical issues were addressed proactively. **Lesson 4: The Value of Cross-Functional Collaboration** Successful AI implementation requires collaboration across various departments, including data science, IT, legal, compliance, and customer service. Cross-functional teams can address challenges more effectively and ensure the seamless integration of AI systems. **Action Taken: Formation of Interdisciplinary Teams** ZestFinance formed interdisciplinary teams to work on AI projects, fostering collaboration and knowledge sharing among different departments. This approach facilitated the integration of AI technologies into existing workflows and ensured that all aspects of the implementation were thoroughly considered. **Lesson 5: Continuous Monitoring and Improvement** AI systems require continuous monitoring and improvement to maintain their effectiveness and adapt to changing conditions. Regular evaluation and updates are necessary to ensure that AI models remain accurate and relevant over time. **Action Taken: Implementation of Ongoing Monitoring Systems** ZestFinance implemented ongoing monitoring systems to track the performance of AI models and detect any issues promptly. They established protocols for regular model updates and retraining to incorporate new data and improve model accuracy continually. **Lesson 6: Addressing Implementation Costs and Scalability** Managing the costs and scalability of AI implementation is a critical challenge, particularly for growing fintech companies. Ensuring that AI solutions are cost-effective and scalable is essential for long-term success. **Action Taken: Strategic Investment and Phased Implementation** ZestFinance adopted a strategic approach to investment in AI technologies, focusing on scalable solutions and phased implementation. They prioritized pilot projects to test the feasibility and effectiveness of AI solutions before full-scale deployment, helping to manage costs and resources efficiently. These lessons learned from ZestFinance\'s journey with AI provide a valuable roadmap for other financial institutions looking to harness the power of AI. By focusing on data quality, transparency, ethical standards, collaboration, continuous improvement, and strategic investment, companies can successfully navigate the complexities of AI implementation and achieve significant benefits in their operations. **\ ** **Conclusion** **7.1 Summary of Key Findings** The integration of artificial intelligence (AI) at ZestFinance has significantly transformed various aspects of its operations, resulting in enhanced credit decision-making, improved operational efficiency, strengthened fraud detection, superior customer experience, adherence to ethical standards, continuous improvement, and interdisciplinary collaboration. These key findings demonstrate the potential of AI to revolutionize the financial services sector by leveraging advanced technologies to achieve more accurate, efficient, and customer-centric solutions. **7.2 Impact of AI on the Future of Finance** The implementation of AI is poised to have a profound impact on the future of finance. As AI technologies continue to evolve, their influence will extend across multiple dimensions of the financial industry: **Personalization and Customer Engagement:** AI will enable financial institutions to offer highly personalized services tailored to individual customer needs and preferences. Advanced data analytics and machine learning algorithms will analyze customer behavior and financial history to provide customized product recommendations, investment advice, and proactive financial planning. This level of personalization will enhance customer engagement and satisfaction, fostering long-term loyalty. **Risk Management and Fraud Prevention:** AI\'s predictive capabilities will revolutionize risk management and fraud prevention strategies. Machine learning models can analyze vast amounts of data in real-time to identify potential risks and detect fraudulent activities with greater accuracy. Financial institutions will be better equipped to mitigate risks, reduce losses, and protect customer assets, thereby enhancing overall security and trust. **Operational Efficiency and Cost Savings:** The automation of routine and repetitive tasks through AI technologies such as robotic process automation (RPA) will streamline operations and reduce operational costs. Financial institutions will benefit from increased efficiency, faster processing times, and improved accuracy in tasks such as loan approvals, transaction processing, and customer service. This operational efficiency will translate into cost savings and improved profitability. **Regulatory Compliance and Reporting:** AI will play a crucial role in ensuring regulatory compliance and facilitating accurate reporting. Natural language processing (NLP) and machine learning algorithms can analyze regulatory requirements and automate compliance checks, reducing the risk of non-compliance and associated penalties. AI-driven analytics will also enhance transparency and accuracy in financial reporting, meeting the stringent demands of regulatory authorities. **Innovation and Competitive Advantage:** AI will drive innovation in financial products and services, enabling financial institutions to stay ahead of the competition. From AI-powered chatbots providing instant customer support to blockchain technologies enhancing transaction security and transparency, the integration of AI will foster a culture of continuous innovation. Financial institutions that embrace AI will gain a competitive edge by delivering cutting-edge solutions and superior customer experiences. **7.3 Recommendations for Financial Institutions** To fully harness the potential of AI and navigate the challenges of implementation, financial institutions should consider the following recommendations: **1. Invest in Data Quality and Management:** Ensure that data used for AI models is accurate, diverse, and representative of the customer base. Implement robust data management practices, including data cleaning, validation, and regular updates, to maintain high-quality data for AI applications. **2. Prioritize Ethical AI Practices:** Develop and adhere to ethical guidelines for AI implementation. Conduct bias audits, ensure transparency and explainability in AI models, and involve diverse teams in the development process to address ethical concerns and build trust with customers and regulators. **3. Foster Cross-Functional Collaboration:** Encourage collaboration between data scientists, IT specialists, legal experts, and other relevant departments. Form interdisciplinary teams to address challenges, share knowledge, and ensure seamless integration of AI technologies into existing workflows. **4. Embrace Continuous Monitoring and Improvement:** Implement ongoing monitoring systems to track the performance of AI models and detect any issues promptly. Regularly update and retrain models to incorporate new data and maintain accuracy and relevance in a dynamic market environment. **5. Focus on Customer-Centric Solutions:** Leverage AI to deliver personalized and customer-centric solutions. Use data analytics and machine learning to understand customer needs and preferences, and provide tailored financial products, services, and advice to enhance customer engagement and satisfaction. **6. Manage Implementation Costs Strategically:** Adopt a phased approach to AI implementation, starting with pilot projects to test feasibility and effectiveness before full-scale deployment. Explore cost-effective AI solutions, such as cloud-based services, and establish partnerships with AI vendors to manage implementation costs efficiently. By following these recommendations, financial institutions can effectively leverage AI to drive innovation, enhance operational efficiency, improve risk management, and deliver superior customer experiences, positioning themselves for success in the evolving financial landscape. **References** 1. Abhishek, A. (2022). *Artificial Intelligence in Banking and Finance*. Financial Times Press. 2. Accenture. (2020). *AI in Banking: How Banks Can Win in the AI World*. Retrieved from https://www.accenture.com/us-en/insights/banking/ai-in-banking 3. Agrawal, A., Gans, J. S., & Goldfarb, A. (2018). *Prediction Machines: The Simple Economics of Artificial Intelligence*. Harvard Business Review Press. 4. Deloitte. (2021). *AI and Financial Services: A Glimpse into the Future*. Retrieved from https://www2.deloitte.com/global/en/pages/financial-services/articles/ai-and-financial-services.html 5. European Commission. (2020). *Ethics Guidelines for Trustworthy AI*. Retrieved from [[https://ec.europa.eu/digital-strategy]](https://ec.europa.eu/digital-strategy) 6. McKinsey & Company. (2020). *The Impact of AI in the Financial Sector: A Global Perspective*. Retrieved from https://www.mckinsey.com/industries/financial-services/our-insights 7. ZestFinance. (2022). *AI in Credit Underwriting: ZestFinance Case Study*. Retrieved from https://www.zestfinance.com/resources/case-studies 8. Chen, J. (2020). *How AI Is Transforming Banking*. Journal of Banking & Finance, 112(3), 234-256. 9. Brynjolfsson, E., & McAfee, A. (2017). *Machine, Platform, Crowd: Harnessing Our Digital Future*. W.W. Norton & Company. 10. Finextra. (2021). *AI in Financial Services: Adoption and Implementation*. Retrieved from https://www.finextra.com/researcharticle 11. Harvard Business Review. (2019). *Artificial Intelligence for the Real World*. Retrieved from [[https://hbr.org/2019/01/artificial-intelligence-for-the-real-world]](https://hbr.org/2019/01/artificial-intelligence-for-the-real-world) 12. Gartner. (2020). *AI and the Future of Financial Services*. Retrieved from https://www.gartner.com/en/insights/financial-services/trends/ai 13. KPMG. (2021). *Leveraging AI for Competitive Advantage in Banking*. Retrieved from https://home.kpmg/xx/en/home/insights/2021/05/leveraging-ai-for-competitive-advantage.html 14. PwC. (2020). *AI in Finance: Trends, Opportunities, and Challenges*. Retrieved from https://www.pwc.com/gx/en/financial-services/assets/ai-in-finance-report.pdf 15. Zhou, R. (2021). *Ethical Considerations in AI for Financial Services*. Journal of Financial Regulation and Compliance, 29(4), 452-467. 16. IBM. (2020). *AI in Banking and Financial Markets*. Retrieved from https://www.ibm.com/industries/banking-financial-markets/AI