Research Methods Week 2 PDF
Document Details
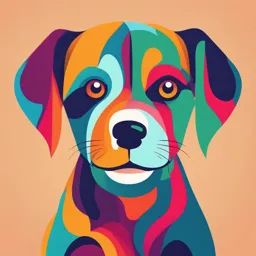
Uploaded by ExpansiveCotangent5784
Federation University Australia
Tags
Summary
This document covers research methods, including the contrast between conceptual and operational variables, different types of observations and scales of measurement, and the importance of reliability, validity, and sensitivity in measurement. It also details different types of validity and reliability, and when selecting an experimental manipulation.
Full Transcript
**Week 2: Research Methods** **Learning Outcomes** 1. Contrast conceptual and operational variables. A conceptual variable is theoretical concept or idea that is used to describe an abstract phenomenon that cannot be directly observed or measured (stress, IQ, motivation). An operational variable...
**Week 2: Research Methods** **Learning Outcomes** 1. Contrast conceptual and operational variables. A conceptual variable is theoretical concept or idea that is used to describe an abstract phenomenon that cannot be directly observed or measured (stress, IQ, motivation). An operational variable is a measurable representation of a construct in the context of a study, it defines how a construct will be observed and measured, or manipulated. 2. Summarise the different ways variables can be measured in terms of types of observations and scales of measurement. Types of observations include self-report (cheap and easy, but responses may be hindered by social desirability bias, memory errors and lack of self-awareness), behavioural observations (more objective, but costly and timely, more objective which is good however observers may have biases or make subjective interpretations that influence the accuracy of the observations, and participants may change their behaviour if they know they are being observed), and psychological measures (more costly, invasive, complex to interpret). Scales of measurement include categorical (nominal data) which is denoting information to different categories such as gender, nationality, personality type etc, ordinal data which are categories with specific order or ranking but the intervals are not known such as HD, D, P, continuous data is data that can take any value within a given range, and intervals are always the same (known); two types 1. Interval data (no zero e.g. temperature) 2. Ratio data (true zero e.g. distance) 3. Explain the importance of reliability, validity, and sensitivity in measurement. Reliability refers to the consistency of the measurement tool, reliable measurements give you more confidence that the results you\'re seeing are accurate and not due to random fluctuations in the measurement tool. Validity refers to whether a measurement tool actually measures what it is intended to measure. Sensitivity refers to the ability to detect small difference or discriminate participant responses. 4. Differentiate between types of measurement reliability and validity, and explain the relationship between validity and reliability. Reliability refers to the consistency of a measurement tool, common ways to assess reliability is test-retest ability, interrater reliability and internal consistency reliability. Validity is the degree to which the measurement tool accurately measures the construct it's supposed to. Different types of validity are construct validity (examining whether the measurement tool actually measures what it is supposed to, these include; face validity (on surface level, seeing whether it measures), content validity (assesses whether or not the measurement tool covers all the important areas), convergent validity (measures if the tool correlates with other tools measuring the same construct), discriminant validity (is the degree to which a measure *does not correlate too strongly* with measures of other constructs that are theoretically different), known-groups validity (refers to whether a measurement tool can distinguish between groups that it is theoretically expected to distinguish between). There is also criterion validity which refers to the correlating with factors known to be related to that construct, this includes concurrent validity (extent to which scores on the measurement tool correlate with scores on a criterion, when both are measured at the same time) and predictive validity (extent to which scores on the measurement tool correlate with scores on a criterion, when the criterion is measured at some point in the future). Reliability is a prerequisite to validity. 5. Explain the importance of construct validity, strength, and reliability when selecting an experimental manipulation, and how to evaluate these factors. Construct validity in experimental measures is determining whether they are actually manipulating the variable they intend to. Strength manipulation refers to manipulation needing to be strong enough to influence participants' behaviour to the desired outcome. May evaluate these manipulations by pilot testing, manipulation checks, evaluating face validity and replications of the study. 6. Define sample representativeness and explain different sampling techniques. Sample representativeness refers to the degree to which a subset of individuals, items, or data points selected for analysis accurately reflects the larger population from which it is drawn. In other words, a sample is considered representative when its characteristics closely mirror those of the population under study. Sampling is split into two groups; Probability sampling where everyone has a chance (simple random sampling, systematic sampling, stratified sampling and cluster sampling) and non-probability sampling where not everyone has a chance (convenience sampling, quota sampling, purposive sampling and snowball/referral sampling). 7. Define statistical power and the impact of power on Type II error, and summarise factors that can impact statistical power. Statical power measured the likelihood of a study to detect a significant effect (rejects null hypothesis). Type II error is when it is concluded that there is no significant effect even though one really exists. Factors that can impact statistical power include effect size, significance level, test sensitivity and study design and type of inferential analyses 8. Discuss the impact of internal, and external validity on the conclusions of research. Internal validity refers to the extent to which a study can establish a casual relationship between IV and DV. Threats to internal validity are study design, poor experimental control, use of invalid or ineffective experimental manipulations and use of invalid or unreliable measurement tools External validity refers to the extent to which measures of a study can be generalised or applied outside the study. Threats to external validity include poor ecological validity, poor psychological realism and non-representative sample. A **construct **(aka **conceptual variable**) is a theoretical concept or idea that is used to describe an abstract phenomenon that cannot be directly observed or measured, such as intelligence, motivation, or stress. Constructs are inherently abstract and can be interpreted in various ways. For instance, \"anxiety\" as a construct could encompass a range of emotions, behaviors, and physiological responses. To accurately manipulate or measure constructs, we need to **operationalise **them. An **operational variable **is a specific, measurable representation of a construct in the context of a particular study. It defines how the construct will be observed and measured, or how it will be manipulated. That is, it turns the abstract concept into a tangible, quantifiable form. In this section of the module, we will discuss how to operationalise variables in the context of choosing the right measurement tools and the right experimental manipulations **Choose the Type of Observation** **In psychological research, there are three primary ways we operationalise constructs:** **Self-Report** Self-report data involves participants providing information about themselves, typically through questionnaires, interviews, or surveys. This can include reporting on their feelings, thoughts, attitudes, behaviours, or experiences. **Example** - Ask participants to tell you how many hours they slept last night, and rate their sleep quality from 1(*very poor*) to 5(*very good*) **Advantages** - [Self-report measures provide direct access to an individual's thoughts, feelings, and perceptions, so we can measure these things even though we cannot observe them directly ourselves. ] - Self-report measures are also typically cheap and easy to administer to large groups. **Disadvantages** - Responses may be influenced by social desirability bias, memory errors, or a lack of self-awareness. **Behavioural Observations** Behavioural observations involve directly observing and recording aspects of an individual's behaviour, often within a natural or controlled setting. This can be done by researchers or through automated methods like video recording. **Example** - Measuring sleep by observing someone in a bed at recording how long their eyes are open or closed, how often they move etc. **Advantages** - Behavioural observations are more objective than self-report, as they are not impacted by self-report bias or memory errors. For example, a person may estimate they slept for 3 hours because they subjectively felt like they had a sleepless night, however, behavioural observations may indicate they actually slept for 6 hours. **Disadvantages** - Observers may have biases or make subjective interpretations that influence the accuracy of the observations. - Reactivity can occur (people might change their behaviour if they know they are being observed). - Can be time-consuming and costly. **Physiological Measures** Physiological measurements involve recording biological data from participants, such as heart rate, hormone levels, brain activity, or skin conductance. **Example** - Sleep may be measured by recording a person\'s brain waves, heart rate, respiration rate, eye movements, and muscle tension. **Advantages** - Data is objective as it does not rely on either the participant or researcher\'s subjective judgments (however interpretation of the data may still be subjective). - Offers a direct link to the physiological processes underlying psychological states. **Disadvantages** - Can be expensive and complex to do (often requires expensive equipment and technical expertise). - The relationship between physiological signals and psychological states can be complex and difficult to interpret. - Some methods can be invasive or uncomfortable for participants and may result in a change in their behaviour (e.g., placing electrodes on a participant\'s head to measure brain waves while they sleep could make them uncomfortable and disrupt their usual sleep patterns). **Choose the Scale of Measurement** - **Categorical Data** Categorical data (aka nominal data) involves assigning numbers that represent different discrete categories that are defined by specific characteristics. So each number is a label denoting the category that the participant is placed in. These categories do not have a specific order to them. Examples: whether a participant is a student or not; the participants\' nationality. - **Ordinal Data** Ordinal data consists of categories with a specific order or ranking, but the intervals between these categories are not necessarily equal or known. Examples: Grades (HD, D, C, P, F); satisfaction rating 1(*very unsatisfied*) to 5(*very satisfied*) *Ordinal data are inherently discrete, and so best characterised as a type of categorical data. However, they can sometimes be treated as continuous for analytical convenience, especially when the number of categories is large, and the order represents a progression (e.g., Likert scales).* - **Continuous Data** Continuous data refers to data that can take any value within a given range, and in which the intervals between numbers are always the same distance. For example, the difference in age between a 1-year-old and a 2-year-old is the same as the difference between a 20-year-old and a 21-year-old. Data is not restricted to specific, discrete categories. For example, a person doesn\'t have to be 20 years old or 21 years old (etc), they can be 20.3 years old, or 20.7 years old (etc). There are two forms of continuous data: 1. **Interval data**: continuous data that does not have a true, meaningful zero. A meaningful zero means that zero indicates the absence of the variable. For example, temperature does not have a true zero (0 degrees Celsius does not mean an absence of temperature). 2. **Ratio data**: continuous data with a true, meaningful zero. For example, measuring the distance a participant sits away from a confederate (0 would indicate they are 0cm away from the other person, i.e., an absence of distance); number of times a participant has engaged in exercise in a given week (0 times = absence of exercise). **validity of measurement:** This refers to the degree to which the measurement tool accurately measures the construct it's supposed to. **Construct Validity** Construct validity refers to the extent to which we are confident that a measurement tool actually measures the construct it claims to. Below are four approaches to evaluating construct validity. Ideally, we would look for evidence for each of these things to be confident a measure is construct valid. - **Face Validity:** refers to whether or not, on the surface, the tool appear to measure what it is supposed to. **How do we evaluate Face Validity?** There is no test to evaluate face validity. Rather, we must apply our own skills in logic to ask whether the characteristics of the tool subjectively appear to be related to the construct. If we think about this in the context of a self-report questionnaire, we would consider whether the items on the questionnaire seem to be asking about things relevant to the construct. For example, if the questionnaire is supposed to be measuring symptoms of depression, the items should look like they relate to symptoms of depression (e.g., "Sometimes I feel hopeless") and not unrelated symptoms (e.g., "Sometimes I hear voices when no one is in the room"). - **Content validity**: refers to the extent to which a measure represents all facets of a given construct. It assesses whether a test or tool covers the entire range of behaviours, skills, or qualities defined by the theoretical concept it is intended to measure. For example, a measure of symptoms of depression should measure all symptoms (i.e., symptoms relating to mood, motivation, cognition, behaviour etc). **How do we evaluate Content Validity?** Content validity is typically evaluated by expert and end-user judgement. Experts in the relevant field review the measurement tool to ensure that it covers the full range of the concept being measured. End-users (i.e., the population the tool is targeted at) are also often involved to evaluate whether the tool represents their real-world experiences of the construct. - **Convergent validity:** refers to whether or not the tool correlates with other measures of the same construct. **How to Evaluate Convergent Validity?** We test if scores on the measurement tool correlate with scores of a *different measure of the same construct*. For example, we would expect one self-report measure of depression symptoms to be highly correlated with other measures of depression symptoms. - **Discriminant validity** (aka divergent validity): is the degree to which a measure *does not correlate too strongly* with measures of other constructs that are theoretically different. **How to Evaluate Discriminant Validity?** We test whether scores on our measurement tool correlate with scores of an unrelated construct. If they don't correlate, or at least don't correlate too highly, this provides evidence they are measuring different things (and thus evidence for discriminant validity) - **Known-Groups Validity:** refers to whether a measurement tool can distinguish between groups that it is theoretically expected to distinguish between. **How to Evaluate Known-Groups Validity?** We administer the measurement tool with different groups of people we know should score differently on the construct, and we test if they produce significantly different scores. For example, a measure of depression symptoms should produce significantly higher scores for people with a diagnosis of Major Depressive Disorder than it would for a group of participants with no history of depression. **Criterion Validity** If your scale accurately measures the construct it claims to, then it should correlate with factors known to be related to that construct (we call these **criteria **or **criterion** variables). For example, we know that people who experience depression are at risker risk of suicidal ideation. We therefore would expect a valid measure of depression symptoms to correlate with a measure of suicidal ideation. There are two main forms of criterion validity: - **Concurrent Validity** Concurrent validity is the extent to which scores on the measurement tool correlate with scores on a criterion, when both are measured at the same time. **How to Evaluate Concurrent Validity?** Participants would complete both the measurement tool we are interested in and another measure of a criterion variable at the same time. We would then test if their scores on each are correlated. For example, participants may complete a measure of depression symptoms, and then complete a measure of suicidal ideation during the same testing session - **Predictive Validity** Predictive validity is the extent to which scores on the measurement tool correlate with scores on a criterion, when the criterion is measured at some point in the future. **How to Evaluate Predictive Validity?** Participants would complete the measurement tool we are interested at one time point, and then we would gather data about the criterion at a later time point. We would then test if their scores on each are correlated. For example, participants may complete a measure of depression symptoms in one testing session, and then complete a measure of suicidal ideation six months later. **Measurement Reliability** To evaluate the quality of a measurement tool, we also need to determine if it is reliable. **Reliability **refers to the *consistency *of a measurement tool. - **Internal consistency** assesses the extent to which all the items on a measure consistently measure the same construct. *This is only relevant for measurement tools with multiple items.* **How to Evaluate Internal Consistency** To test this, we use statistics that calculate the correlations between the items on the measure. If they all correlate highly together, this suggests they are measuring something similar. There are different statistics we can use to do this (you'll learn how to calculate these in Jamovi Week 5). - [*Cronbach's alpha (α):*** **]a single value that represents the degree to which all of the items on the scale are intercorrelated. We interpret this in a similar way to a correlation coefficient (*r*), where values close to 0 mean the items are not correlated and values close to 1 mean they are strongly correlated. This is the most common measure of internal consistency used in psychology literature. Cronbach (1951) recommended that Cronbach's αs\>.70 be regarded as acceptable, however this guideline has been criticised as being arbitrary (e.g., Taber, 2018) and the criteria for "acceptable" can vary depending on the field (e.g., in medical fields it is common to only consider alphas \>.80 to be acceptable). Tavakol and Dennick (2011) suggest that if alpha is too high (high 90s), this could suggest items are too similar and therefore redundant. - [*McDonald's omega (ω)*** **-] aka omega total. McDonald's ω is similar to Cronbach's α. It measures the degree to which items on a test are intercorrelated. However, it uses a different statistical model which is more accurate in situations where certain assumptions are not met (i.e., items are not normally distributed, have different variance etc). It is interpreted in the same way as Cronbach's α. This option is lesser known and used than Cronbach's α, but is becoming increasingly popular. - *[Item-rest correlations]*** **-- correlations between each individual item and the total of all the other items on the measure. This is a way of identifying individual items that may not be consistent so you can consider adapting or removing them. - *[Kuder-Richardson Formulas 20 and 21 (KR20 and KR21)]* -- these statistics measure the internal consistency of a scale that has binary responses (e.g., Yes/No) instead of ordinal or continuous ones. - **Test-retest reliability** refers to the consistency of a measure over time. It assesses the extent to which test scores are consistent when the same test is administered to the same group of people under the same conditions but at two different points in time. This is only relevant for constructs that are predicted to be stable over time (e.g., personality traits), and not ones that we expect to vary (e.g., mood). **How to Evaluate Test-Retest Reliability** The same measurement tool is administered to the same group of people at two different points in time. We then calculate the correlation between the two sets of scores. A high correlation indicates high test-retest reliability. **Measurement Sensitivity** Sensitivity refers to the degree to which a measurement tool can discriminate (i.e., tell the difference) between people who vary on a construct. If everyone always gets the same score, the measure is not very useful to us. If people get different scores that enable us to tell them apart, the measure is useful. **How To Evaluate Sensitivity?** - We can evaluate this in a similar way to known-groups validity -- by administering a test to people we know vary on the construct and testing whether they (a) score in the range we expect them to, and (b) if there are significant differences in scores between groups known to be different on the construct. - Another way to evaluate sensitivity is to look at the variability of scores. **Experimental Manipulation** - **Construct Validity** Experimental manipulations need to have good **construct validity** -- this means they need to actually manipulate the variable they intend to. For example, if your study aims to manipulate the emotional state of a participant, then your manipulation needs to induce the emotion you want the participant to feel. If your study aims to measure if engagement in cognitive-behavioral therapy (CBT) is effective for reducing anxiety symptoms, then you need to expose participants to CBT as you have defined it, and not other forms of therapeutic practice. - **Strength of the Manipulation** To be effective, a manipulation needs to be strong enough to influence the participants\' behavior. For example, say your study aims to manipulate the emotional state of a participant by inducing anger in one group of participants, and neutral emotion in another. If your manipulation only manages to cause mild annoyance, rather than anger, it may not be powerful enough to cause a change in behaviour. If an experimental manipulation is not strong enough, then the study may be unable to detect an effect even if one really does exist in the real world. **Evaluating Manipulations** - **Pilot Testing** Pilot studies are small studies that you run before you conduct your main study. These can be useful for testing the construct validity and effectiveness of your experimental manipulation, particularly if it's one you have devised yourself. - To test the** construct validity** of the manipulation, you might run a pilot study to test if the experimental manipulation causes changes in relevant criteria. For example, if your study aims to induce anger, you could run a pilot study that exposes participants to this manipulation, and then asks them to report how angry they feel. If the experimental manipulation has good construct validity, they should report increased anger after exposure to it. - Another way to test **construct validity** is to use a pilot study to test if participants perceive the manipulation as relevant to the construct. - Pilot testing can also be used to conduct a primary test of the **strength of the experimental manipulation**. This involves conducting a small study including both your proposed manipulation and dependent variable to see if there is preliminary evidence that it is strong enough to cause a change in behaviour. - Comparing the results of a study to the results of an initial pilot study can also provide some evidence about the **reliability **of the experimental manipulation\'s effects. *Note that not all experimental studies will conduct pilot testing, as it may not be possible to conduct these due to time and cost constraints. * - **Manipulation checks** Are measurements included in your study to verify that the manipulation is influencing the variable it was intended to manipulate. For example, if your study aims to manipulate emotional state by inducing anger, then you might ask participants to report how angry they feel directly after they are exposed to the manipulation. *Note that it may not be appropriate to use manipulation checks in all studies, as sometimes doing so can make participants suspicious of the hypotheses and bias their responses.* - **Evaluate Face Validity** You can evaluate the construct validity of an experimental manipulation by considering its face validity. That is, on the surface, how well does the manipulation align with the theoretical definition of the construct the study aims to manipulate? That is, on the surface, does it look like it should manipulate what it claims to? *If a published study does not include pilot testing or manipulation checks, you can still evaluate the face validity of an experimental manipulation using your own logic.* - **Replication** The best way to test if an experimental manipulation reliably causes a change in behaviour is to replicate the study. This means re-running the study with a new sample to verify that any effects found the first time around was not an artifact of chance. There are a few approaches to this: - **Exact replications**: involve repeating the study exactly as it was run the first time, but with a new group of people. - **Conceptual replications**: involve conducting another study to test the same hypotheses as the first, but may change some aspects of the methods (e.g., may measure the same dependent variable but in a different way). - **Replication and extension: **repeat the study using the same methodology as the original study, but add additional elements to extend on it in some way (e.g., may add additional dependent variables to see if the results generalise to other outcomes). **Sample sizes** - **Population:** The population refers to the entire group about whom the research conclusions are to be drawn. Typically, not all members of the population will participate in the study (unless the population is very small), but the aim is to learn about them from the small selection of people who do take part in the study. - **Sample:** The sample refers to the specific group of people that participate in the study. The results of the study represent their responses, and are used to make inferences about the population. **Sample representativeness** refers to the degree to which a subset of individuals, items, or data points selected for analysis accurately reflects the larger population from which it is drawn. In other words, a sample is considered representative when its characteristics closely mirror those of the population under study. If the sample is representative of the population, the results of a study are likely to generalise to the population the sample represents. If not, the results will not generalise and the research cannot make valid conclusions about the wider population based on the results from their sample. **How to Recruit a Representative Sample** **Probability sampling** These are sampling techniques where each member of the population has a known chance of being selected. - **Simple Random Sampling - **everyone in the population has an equal chance of being selected. To do this you need access to every member of that population, which is difficult unless you are working with a small group (e.g., the population of a specific town or school). - **Systematic Sampling - **The first person select from a population is random, but from then on selection follows a systematic rule (e.g., every 10th person is selected). - **Stratified Sampling - **The population is divided into subgroups (e.g., age groups) and then participants are randomly chosen from each group. - **Cluster Sampling - **The population is divided into groups, and then we randomly choose one or more of those groups and sample everyone in it. ** Non-probability Sampling** These are sampling techniques where not everyone in the population has a chance of being chosen, and selection is not random. - **Convenience sampling **- Participants are selected based on ease of access/availability - **Quota Sampling** - In this method, the researcher decides beforehand how many people of certain characteristics they need to match the population (e.g., 40% from rural locations, 60% from urban). The researcher then recruits this number of people fitting each characteristic. - **Purposive Sampling** - Participants are selected based on specific characteristics/criteria. E.g., only people working in hospitality and who have experienced food insecurity. - **Snowball or Referral Sampling** - Existing participants recruit future participants by passing on information about the study. **[How to Evaluate Sample Representativeness?]** Even if you use a sampling method that is more likely to result in a representative sample, that doesn\'t mean your sample will actually be representative. And just because you use a method that is less likely to result in a representative sample, that doesn\'t mean it won\'t be. We still need to evaluate the sample after we have collected data to determine its representativeness. Key things to look at: 1. **Sample Size**: The size of the sample can affects its representativenes, because a larger sample has a broader range of people in it, and larger samples generally provide more reliable estimates of population parameters, *however, this alone is not sufficient to ensure a representative sample.* 2. **Demographic Characteristics: **Evaluate if the** **sample demographic characteristics reflect the characteristics of the population. These may include age, gender, ethnicity, socioeconomic status, education level, geographic location, etc. A representative sample should have similar distributions of these characteristics as the population. To evaluate this you need to know what the distribution of these characteristics in the population are. **What is statistical power?** **Statistical power **refers to the probability that a statistical test will correctly reject the null hypothesis when it is false. In simpler terms, it measures the likelihood of a study to detect a significant effect, assuming the effect truly exists in the real world. Sometimes even though an effect really exists in the world, we don't find it in our data. For example, we know there is a correlation between stress and depression (e.g., Stroud et al., 2008). However, you might run a study that measures these two variables and find no correlation between them. This means you have made a Type II error (retained the null hypothesis/concluded there is no significant effect, even though one really exists). **A study with high statistical power has a better chance of detecting true effects.** **A study with high statistical power is also likely to produce more precise effect estimates, which are in turn more likely to replicate in future studies, because the study will have less error. **High statistical power increases the likelihood that a statistically significant result reflects a true effect rather than a false positive, because it is less likely that effect is an artifact of error. Therefore, studies with high power are more likely to be replicated in future studies because they are less likely to be based on spurious findings. **How much power do you need?** Cumming (2012) recommends statistical power of at least.80 - that means, 80% chance of detecting a true effect (or 20% chance of making a Type II error). In general, we aim for high-powered studies. However, there is a trade-off between power and the Type I error rate (α). A** Type I error **occurs when you find a significant effect, however it's a false positive (i.e., the effect does not exist in the real world). When power is high, the odds of a Type II error are low, but the odds of a Type I error can be higher. When power is low, the odds of a Type II error are high, but the odds of a Type I error are lower. When selecting an appropriate level of power, you need to consider this trade-off. **Image this scenario: A pharmaceutical company is testing a new medication for the treatment of insulin resistance.** - **If they make a Type I error**, they will conclude the drug works even though it actually doesn't. They may market the drug to patients with Type II diabetes, who at best will receive no benefit from it, and at worst may experience medical complications if they take this new drug instead of other drugs that really do work. - **If they make a Type II error,** they may conclude the drug does not work even though it really does. If this is the case, the drug will not be manufactured and made available to people who could benefit from it. Which outcome represents the greatest risk? When selecting the appropriate power and alpha level, researchers need to weigh up these kinds of risks. **Sample size and power** Sample size directly affects statistical power. Generally, larger sample sizes increase statistical power because they provide more information and **reduce random variability (error) in the data**. With more data points, there is less error, and it\'s easier to detect smaller effects. Conversely, smaller sample sizes often result in lower statistical power because they provide less reliable estimates of population parameters and more error in the data. **Other factors that influence power** - **Effect size:** The magnitude of the difference or relationship between variables in the population. Larger effect sizes are easier to detect, leading to higher power. - **Significance level (alpha): **The threshold set to determine statistical significance, typically denoted as *α*. A lower significance level (e.g., *α* =.01 instead of *α* =.05) decreases the chance of a Type I error but also reduces statistical power. - **Test sensitivity:** The ability of the statistical test to detect differences or relationships. More sensitive tests have higher power. - **Study design and type of inferential analyses:** Some study designs and their associated analyses are inherently more powerful than others: repeated measures designs are more powerful than between-groups designs. **How to calculate the sample size you need** An ***a priori* power analysis** is a statistical procedure conducted *before *data collection to determine the required sample size needed to achieve a certain level of statistical power for a planned hypothesis test. To conduct an a priori power analysis, you need the following information: - **Estimated effect size:** You need an idea of the size of the relationship(s) or difference(s) you are studying. You can find this by searching the literature to see the size of the effects reported in past research (if meta-analyses are available, these will provide the most accurate estimate). If there is no past research, you need to select the smallest effect size you are interested in detecting. - **Significance level (alpha)**: select the threshold for significance you will use for your inferential analyses (this should be determined *a priori*, not later when you get to the statistical analysis). - **Type of analysis:** the way you conduct a power analysis differs for each type of inferential analysis. Given the type of analysis you use is determined by your hypotheses and the type of data you have collected, you should know what it will be before you even start recruiting participants, **Internal and External Validity** **Internal validity** refers to the extent to which a study can establish a **causal relationship** between the independent and dependent variables. *Threats to internal validity mean undermine our ability to draw conclusions about causality from a study. * Threats to internal validity include: 1. **Study design: **nonexperimental studies by definition cannot test causality, and so have low internal validity. 2. **Poor experimental control**: We spoke about experimental control in Module 1. Poor experimental control means that there is a higher probability of extraneous variables confounding the results of the study. 3. **Use of invalid or ineffective experimental manipulations** or use of experimental manipulations without processes to verify their validity (they may seem to work, but are they really manipulating what they are supposed to?) 4. **Use of invalid or unreliable measurement tools**: if we cannot be sure we are measuring something accurately or consistently, we can\'t make conclusions about causation because any effects you find may not be related to the constructs you think they are. **External validity** refers to the extent to which the results of a study can be generalised or applied to settings outside the study, such as the to the general population or different environments. For example, if you conduct a study with high school students at a specific school, will their results generalise to students at other schools? If you conduct an experiemental study in a lab, will the results generalise to real world environments outside the lab? *Threats to external validity undermine our ability to generalise the results to people or environments outside of the study.* Threats to external validity include: - **Poor ecological validity**: ecological validity refers to how natural or realistic the experimental envirnonment and tasks are. When a study is conducted in artificial, highly controlled environments, they may not generalise the real world where many other uncontrolled variables are present. - **Poor psychological realism**: if the mental processes used for a task in a research study are very different to the processes a person would use in real life, the results may not generalise outside the study. For example, memorising strings of random numbers for a task in a research study may use different memory processes than recalling faces, which have systematic and predictable patterns. So a study using the former task would have poor ability to generalise results ability to recall faces in social settings. - **Non-representative samples**: if a sample differs in significant ways from the population, then their results may not generalise.