Questions for revision.docx
Document Details
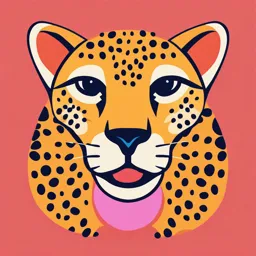
Uploaded by JudiciousNephrite2042
Full Transcript
**Questions** 1. **What is the purpose of MLM** To predict individual outcomes from other individual variables as well\ as group level variables, taking into account the grouping structure\ Lower level: micro. Upper level: macro 2. **What is an example of a macro level and m...
**Questions** 1. **What is the purpose of MLM** To predict individual outcomes from other individual variables as well\ as group level variables, taking into account the grouping structure\ Lower level: micro. Upper level: macro 2. **What is an example of a macro level and micro level** 3. **Does the grouping structure set up dependence among observations** a. Sometimes you want independent observations for statistical procedures 4. **What would be a reason you need group dependence?** You want to understand group level effects 5. **Can a model predict constructs at more than one level -- e.g. individuals nested in groups** (e.g. employees nested in organisations) 6. **What are examples of why we are interested in the dependency that a group structure creates?** How a teacher can influence a child How a work environment can influence employee productivity 7. **What is multi stage sampling**  8. What does this example suggest +-----------------------------------+-----------------------------------+ | | Two variables **below the dotted | | | line** | | | | | | X predicting y | | | | | | A dotted line indicates there is | | | a macro level not indicated by | | | the dotted line | | | | | | Could use MLM but depends | +===================================+===================================+ |  | Two variables **above the | | | dotted** line indicates Marco | | | level. | | | | | | **Z predicting Y** | | | | | | Could use MLM for this | +-----------------------------------+-----------------------------------+ | | **MULTI LEVEL MODELLING** | | | | | | **Example 1** | | | | | | Maro predicts micro level - | | | example. Z Higher resourced | | | classrooms predict higher | | | individual performance | | | | | | **Example 2 WE WILL FOCUS** | | | | | | Z predicts Y (holding constant | | | score on X) , (regression) | | | | | | **Example 3** | | | | | | X and Y dependent on Z | | | | | | Verbal iq (x) | | | | | | Individual results (y) | | | | | | School (Z) | | | | | | Verbal IQ will predict year 12 | | | results at any school | +-----------------------------------+-----------------------------------+ |  | e.g. individual student behaviour | | | predicts teacher stress | | | | | | eg. Teacher reputation predicts | | | student behaviour predicts | | | student results predicts teacher | | | stress. | +-----------------------------------+-----------------------------------+ 9. **What is an example of when you would need to change two levels into one** - Pupils within classrooms: - Variables: test result; classroom size (standardized) - Research question. Do larger classes affect test results? Pupil data = class and test Class data = class and size You would need to calculate the 10. **How would you manage an aggregation?** Calculate the means of the test and then do a regression at the macro level 11. **What is the definition of aggregation** **Aggregation** in multilevel modeling (MLM) refers to a common procedure where we take all data points at the lowest level and aggregate them into a single data point at a higher level 12. **When is it ok to use aggregation?** Aggregation is OK if you are only interested in macro-level information 13. **What are the issues with aggregation in MLM** a. *shift of meaning:* Variables aggregated to the macro level tell us about the macro level, and do not directly tell us about the micro level. b. issues related to neglect of original data structure: (e.g. reduction of power) c. prevents examination of cross-level interactions d. *ecological fallacy*: General term for mistaken attempts to interpret aggregated data at a lower level (e.g. at the micro level) 14. **What is the definition of disaggregation in MLM and why would you do it** 15. **Do we find contradictory results if we apply the class size effect test score** to the pupil data -- yes we find a difference between the aggregated and disaggregated scores 16. **What are the issue for disaggregation** - measure of macro-level variable considered as micro-level - *miraculous multiplication of the number of units* - risks of type 1 errors - Do not take into account that observations within a macro-unit could be correlated. 17. Is it recommended to combine a multilevel approach if you are looking at both a micro and macro level data to minimise the risk of erroneous conclusions? 18. **What is a random effects ANOVA** 19. Can ANOVA be represented as an ANOVA 20. What is the formula for an ANOVA 21. What is the difference between fixed effect and random effects ANOVA **For fixed effects** ANOVA we assume the groups refer to categories with its own distinct interpretation -- e.g. gender, religious denomination, treatment 22. Do all groups have the same mean for a fixed effects ANOVA 23. **Random effects ANOVA** groups are samples from a population (actual or hypothetical) of possible macro units. B0 our constant is not a fixed but random 24. Does β~0j~ = γ~00~ + u~0j~ include both a fixed and random component - Yes. - γ~00\ =\ **fixed\ effect**\ (the\ intercept).~ - *u~0j~* = (random effect) is assumed normally distributed with mean of 0 and variance *τ*^2^. (this will change depending on what class you are in). 25. Does the null hypothesis change with a random effects ANOVA? 26. What is the equation for a random effects ANOVA ***Y~ij~* = γ~00~ + u~0j~ + *ε~ij~*** 27. What is the equation for the total variance in a random effects ANOVA 28. **What is an ICC** It is also the correlation between two randomly drawn individuals in one randomly drawn group. (e.g. understand resemblance in children who study under the same teacher) 29. **Is true that the ICC is also the correlation between randomly drawn individuals in one randomly drawn group?** Yes 30. **What is the cut off from an ICC** - An ICC \>.05 is often used to justify doing MLM - In this instance the ICC is 0.147, well above that arbitrary cutoff. - A low ICC is often used to justify not doing MLM, but this is a questionable practice -- Nezlek (2008) goes into more detail about why 31. How do I interpret this table  - Random effects ANOVA: - Variance of intercept = var (u~0j~) = 6.74 - Variance due to residual = 38.99 - Total variance = 6.74 + 38.99 = 45.73 - ICC = var (u~0j~) / total variance = 6.74 / 45.73 = 0.147 - So 14.7% of variance in test score is due to the group structure 32. Does ICC fall between 0 and 1 b. Yes 33. **How would I calculate the total variance here** Add the residual plus the intercept **How would I calculate the total variance due to the group structure?** - Divide the Intercept / group variance 34. **What does the random intercept multilevel model attempt to explain** 35. **What is the random intercept (null model)** 36. **What is the random intercept with one predictor?** 37. **What is an example of a random intercept model** **Group of school** **SES (social status)** **Math Test result** --------------------- ------------------------- ---------------------- **Is SES associated with performance on a math test performance** 38. **Does the computer generate MLM in two stages?** **Yes** A two-stage strategy to investigate variables at two levels of analysis. i. Level 1: relationships among level 1 variables estimated separately for each higher level (level 2) unit. Individual level) ii. These relationships are then used as outcome variables for the variables at level 2. 39. **What is a level 1 equation.** 40. **What is a Level 2 equation** YOO (Gamma 00) becomes the overall mean. If you have equal group sizes this 41. UOJ individual schools have intercepts that vary from the ovrerall intercept or grand mean. 42. This is the null model (random intercept model. 43. What is the following showing?  1. 57.67 = overall intercept (gamma 00) 2. 66.55 = estimate over the variance of the level 1 variant 3. 10.64 = is the estimate of the between school variation intercept 4. ICC =.138 5. 13.8% is due to the variation in groups schools -- can keep going with MLM Let's run the MLM Outcome for person I in group j = fixed effect + fixed slope + (SES)ij + uoj (varies depending based on school) (four parameters above)  As SES increases by one unit, predicted math achievement rises by 3.87 units. Controlling for interclass correlation. Built a model assessing variability across schools. Intercepta are starting points at each school. We achieve the relationship between SES and math and school