Quantitative Data Analysis WorkBook_RMcC 23-24.docx
Document Details
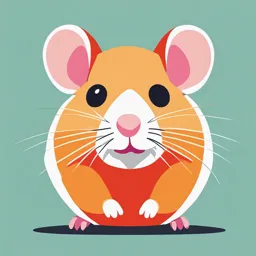
Uploaded by SteadfastHarmonica
U.C.C.
Full Transcript
CKX23 - Physiotherapy MODULE PP6005 Quantitative Data Workbook YEAR 1 Contents 1 Introduction and Overview 6 1.1 Overview 6 1.1.1 Data analysis can be divided into two: descriptive statistics and inferential statistics 7 1.1.2 The handbook will cover 7 1.1.3 Introduction to Results of a paper 8 1.1....
CKX23 - Physiotherapy MODULE PP6005 Quantitative Data Workbook YEAR 1 Contents 1 Introduction and Overview 6 1.1 Overview 6 1.1.1 Data analysis can be divided into two: descriptive statistics and inferential statistics 7 1.1.2 The handbook will cover 7 1.1.3 Introduction to Results of a paper 8 1.1.4 Galvin R, Cusack T, O'Grady E, Murphy TB, Stokes E. Family-mediated exercise intervention (FAME) evaluation of a novel form of exercise delivery after stroke. Stroke. 2011 Mar;42(3):681-6. 8 1.1.5 McCullagh R, O’Connell E, O’Meara S, Dahly D, O’Reilly E, O’Connor K, Horgan NF, Timmons S. Augmented exercise in hospital improves physical performance and reduces negative post hospitalization events: a randomized controlled trial. BMC geriatrics. 2020Dec;20(1):1-1. 8 1.2 Determine which results are descriptive and which are inferential. Where are they presented? 8 1.3 Task 1: Interpreting the results of a paper 10 1.3.1 Galvin R, Cusack T, O'Grady E, Murphy TB, Stokes E. Family-mediated exercise intervention (FAME) evaluation of a novel form of exercise delivery after stroke. Stroke. 2011 Mar;42(3):681-6. 10 1.3.2 McCullagh R, O’Connell E, O’Meara S, Dahly D, O’Reilly E, O’Connor K, Horgan NF, Timmons S. Augmented exercise in hospital improves physical performance and reduces negative post hospitalization events: a randomized controlled trial. BMC geriatrics. 2020 Dec;20(1):1-1. 13 1.3.3 List the areas that are unclear to you here. You can review these at the end of the workbook to see what remains unclear. 15 1.4 Types of data (levels of measurement) 16 2 Introduction to data analysis, how to prepare for the data analysis 18 2.1 Introduction 18 2.2 Aim 19 2.3 Sample 19 2.4 Variables 19 2.5 Task 2: From the list above, categorise as dependent and independent variables. 19 2.6 Data 20 2.7 Potential confounders 21 3 How to get to know the Data: Descriptive Statistics 22 3.1 Introduction 22 3.2 Graphs for Categorical Data 22 3.3 Graphs for continuous data. 23 3.3.1 Continuous Data 23 3.4 Supplementary Tests for Normal Distribution 26 3.5 Summarising data mathematically (in tables and text). 27 3.6 Reporting Descriptive/Summary Statistics 27 3.7 Task 3: Can you find the primary outcome in the paper? 28 4 Reporting numerical summaries. 29 4.1 Categorical/binary/dichotomous data 29 4.2 Task 4: From the SPSS output, complete the table below. 30 4.3 Interpreting statistical outputs on continuous data 30 4.4 TASK 5: Analysis of the graphical display of continuous data 31 4.5 Task 6: Do you think the data is normally distributed. Why? 32 4.6 Task 7: Analysing boxplots 32 4.7 Task 8: Let’s take another example: fesTOTAL (falls efficacy) 34 4.8 Task 9: Read the table of summaries of continuous data below 36 4.9 Task 10: Convert the findings into text 40 5 Conclusion: Reporting descriptive/summary statistics 41 6 Preparing for Inferential Statistical Analysis 42 6.1 Hypothesis testing 42 6.1.1 Step 1: Define your Hypothesis Statement 42 6.1.2 Step 2: Hypotheses - Direction of effect (1 tailed / 2 tailed) 43 6.1.3 Step 3: Setting your p-value level (probability of findings being due to chance) 44 6.2 Decision errors: Type 1 and Type 2 error 44 6.3 Confidence Intervals 46 7 Statistical tests to compare two sample means (t tests) (continuous data) 47 7.1 Parametric tests to compare means 47 7.1.1 Task 11: Interpreting the output of an independent sample t tests 47 7.2 Non-parametric tests to compare two data sets 52 7.2.1 Task 13: Interpreting a Mann Whitney U (Non-parametric equivalent to the unrelated/ independent t test’s output 52 7.2.2 Task 14: Interpreting a Wilcoxon test: (Non-parametric equivalent to the related/dependent t test) 53 8 Simple/Univariable Associations 54 8.1.1 Task 15: Define the dependent and independent variable for each of the above 55 8.1.2 Task 16: Identify potential confounder for each dependent variable. 55 8.2 Tests of association 56 8.2.1 Bi-variate distribution tables and Chi Square Test (x2) 56 8.2.2 Task 17: Cross tabulation and Chi square (x2) 58 8.3 Task 18: Complete the 2x2 table below. 59 8.4 Relative Risk (RR) & Odds Ratio (OR) 59 8.4.1 Relative risk (RR) – summarizes the strength of the association between the risk factor and the disease. 60 8.4.2 Odds ratio 61 9 Correlation 63 9.1 Graphical presentation 63 9.2 Correlation coefficient 64 9.2.1 Correlation coefficient misinterpretation 65 9.3 Task 19: How to interpret a scatterplot: Investigate the bivariate associations with continuous variables: correlation 66 9.4 Task 20: interpret the correlation co-efficient 67 9.5 Task 21: Review Table 1.2.3. (Page 8) What remains unclear? 68 Appendix A: Choosing A Statistical Test 69 Appendix B: Outcome measures 72 Quantitative Data Workbook Introduction and Overview Overview Healthcare research can be categorised as qualitative (perceptions, experiences, satisfactions; collection of language and descriptions) or quantitative (measurements, scorings, counts) in nature. Many of the phenomena of interest to health scientists, e.g. height, weight, blood pressure, haemoglobin level, radiation dose, quality of life etc., can be measured or quantified. Therefore, healthcare research can generate lots of numerical data. This workbook will help you make sense of research data. The tasks are designed to build understanding…. from simple ways of summarising and describing data to inferential statistical concepts and tests. These tasks will focus on the “how” rather than the “why”. We will try to get you through the analysis step by step, so that the emphasis is on the interpretation of the results, rather than the statistical tests. You can expect that the statistical tests will not make sense to you at times. The aim of these sessions is to learn the process (rather than understand the process) and understand how to interpret the results. The aim of the workbook is to address the following learning outcomes Articulate how to conduct and interpret quantitative analyses. Formulate hypotheses, interpret and derive conclusions from a statistical output Evaluate and summarise quantitative data using frequency tables, numerical measures, histograms and boxplots Note: Multivariable analysis will not be covered in detail in this workbook. Data analysis can be divided into two: descriptive statistics and inferential statistics These represent two phases in analysing data. Descriptive statistics describe your sample. This would include the summary statistics (mean (standard deviation), median (interquartile ranges), frequency (percentages) etc). The second form of analysis is called inferential statistics. These tests allow you to make assumptions about the population based on your sample. For this reason, your sample should be as good a representation of the population as possible. If your sample size is too small, or limited to a certain age group, or setting, differences will exist. Tests and techniques are applied to the sample’s data to allow the researcher to make inferences (infer/derive/assume something) about the population of interest (people with cancer, people with MS) on the basis of data from a sample of that population… hence the term ‘inferential’ statistics. These tasks will help to understand and interpret statistical results. Your learning will be assessed through an open-book data analysis assignment for this module. The handbook will cover 1: Introduction to data analysis, and types of data How and when to use descriptive statistics to analyse your data 2: Descriptive statistics: Summarising data graphically and mathematically Understand summary stats and graphs 3: Inferential statistics: distribution, effect size confidence intervals, and p values; hypothesis and how to interpret the output; CI; T-tests Understand the appropriateness and how to interpret t tests 4: Bivariate associations: (ii) Category data - crosstabulation; Chi Sq; relative risk; odds ratio, sensitivity and specificity (ii) Continuous data – Correlation; linear regression. Understand the appropriateness and how to interpret crosstabulation Appendix: Choosing a test: parametric and non-parametric tests Introduction to Results of a paper For this chapter, we will use three papers. Galvin R, Cusack T, O'Grady E, Murphy TB, Stokes E. Family-mediated exercise intervention (FAME) evaluation of a novel form of exercise delivery after stroke. Stroke. 2011 Mar;42(3):681-6. https://www.ahajournals.org/doi/epub/10.1161/STROKEAHA.110.594689 McCullagh R, O’Connell E, O’Meara S, Dahly D, O’Reilly E, O’Connor K, Horgan NF, Timmons S. Augmented exercise in hospital improves physical performance and reduces negative post hospitalization events: a randomized controlled trial. BMC geriatrics. 2020 Dec;20(1):1-1. https://bmcgeriatr.biomedcentral.com/articles/10.1186/s12877-020-1436-0 Determine which results are descriptive and which are inferential. Where are they presented? Authors (date) Descriptive (section text and figure/table) Inferential (section text and figure/table) Notes Galvin et al, 2011 Results: Table 1 Results: Table 2 Table 3 McCullagh et al, 2020 Results: Table 2 Results: Table 3 Fig 2 Task : Interpreting the results of a paper For this section, we will review papers we are already familiar with from the module. It is a good idea to review the lecture “Randomised Controlled Trial”, specifically the section on results precision, (p value, magnitude of the effect (also known as effect size) and 95% confidence intervals). The aim of this task is to learn how to find and interpret the results published in the paper, reflecting on the study sample and baseline characteristics. Galvin R, Cusack T, O'Grady E, Murphy TB, Stokes E. Family-mediated exercise intervention (FAME) evaluation of a novel form of exercise delivery after stroke. Stroke. 2011 Mar;42(3):681-6. https://www.ahajournals.org/doi/epub/10.1161/STROKEAHA.110.594689 Review the results for lower limb impairment and walking endurance at postintervention and at follow up to complete this table. The aim of the trial (PICO) P- People with a confirmed diagnosis of a first unilateral stroke, no cognitive impairment, participating in a physiotherapy program, and a family member willing to participate in the program (n=40) I- FAME programme. 35 min sessions with the assistance of nominated family member. C- Routine physiotherapy with no FAME intervention. O- Primary: LL-FGA. Secondary: Motor Assessment Scale, the Berg Balance Scale, the 6-Minute Walk Test, and the 100-point original Barthel Index. Baseline characteristics What potential confounders were not balanced at baseline? Were any potential confounders not considered at baseline? Was a power calculation completed? Did the researchers reach that number, even at outcome? If not, did they reasonably impute the data? How? The location where they received their physical therapy. The social element of conducting with family members. Their level of participation with physical therapy before the study. Yes Yes Results Firstly, we examine the results between groups. We identify which results did not appear to happen by chance. In other words, the results that reached a p value of less than 5% or less. Within-group differences are a finding, but between-group differences are stronger evidence of effectiveness. Note, RCTS are designed to compare between-group differences. What results were significant? There was a significant [difference / association / ly greater improvement / reduction] between [what] at [which timepoint] Significant difference between control and Fame groups for LL-FMA, MAS, BBS, SMWT metres, BI in favour of the FAME group at postintervention compared to baseline. Secondly, we examine how large the difference / association exists. What was the magnitude of the effect? The [which group] [improved / deteriorated etc] by [how much?] in [which outcome measure] The FAME group improved by an average of 164.1m in the 6MWT. Finally, we report the variability of the magnitude of the effect using the 95% CI. This means that we are 95% confident that the mean lies within these margins. The greater the sample size, the narrower the margins become, or the more precise our estimation becomes (this relates to power calculations). What else is reported instead of the 95% CI? What were the 95% CI reported? None reported Between group differences may also be related to baseline differences, as well as the intervention. It is important to check whether the researchers considered these differences in the analysis [crude /unadjusted results and adjusted/multivariable analysis]. In effect, adjusted analysis adjusts each follow-up score for their baseline score, preventing an overestimation / underestimation of effect in the presence of a baseline imbalance. (Vickers & Altman, 2001) Note any adjustments and reflect on the values. Complete this for all the outcomes of interest. Finally, reflect on the results. For those outcomes that did not reach significance, can you suggest why? [think power calculations / intervention FITT / participants adherence or suitability]. LL-FMA, MAS, BBS – Between postintervention and follow up there was no significant differences recorded. This is likely due to the participants reaching their ceiling. There comes a point where strength, balance and motor function improvements will plateau due to factors such as age. This then has a knock-on effect to the Barthel INDEX. No further improvements in functional ability will limit the improvement for ADL independence. McCullagh R, O’Connell E, O’Meara S, Dahly D, O’Reilly E, O’Connor K, Horgan NF, Timmons S. Augmented exercise in hospital improves physical performance and reduces negative post hospitalization events: a randomized controlled trial. BMC geriatrics. 2020 Dec;20(1):1-1. https://bmcgeriatr.biomedcentral.com/articles/10.1186/s12877-020-1436-0 Review the results for Short Physical Performance Battery at discharge and follow-up and length of stay at discharge to complete this table. The aim of the trial (PICO) P - Irrespective of ward allocation, medical inpatients aged 65 and over, needing an aid and/or assistance to walk on admission, and admitted from and planned for discharge home (rather than for institutional care), with an anticipated hospital stay ≥3 days were recruited (n=189). I – APEP C – Usual Care and a sham programme of breathing and stretching. O – The effects of intervention on healthcare utilisation, Physical performance (SPPB), QOL, N-EADL, Functional ambulation, Falls Rate. Baseline characteristics What potential confounders were not balanced at baseline? Were any potential confounders not considered at baseline? Was a power calculation completed? Did the researchers reach that number, even at outcome? If not, did they reasonably impute the data? How? Ward location Yes No Yes** Results Firstly, we examine the results between groups. We identify which results did not appear to happen by chance. In other words, the results that reached a p value of less than 5% or less. Within-group differences are a finding, but between-group differences are stronger evidence of effectiveness. Note, RCTS are designed to compare between-group differences. What results were significant? There was a significant [difference / association / ly greater improvement / reduction] between [what] at [which timepoint] Physical performance score at discharge showed a significant difference between groups in favour of APEP at discharge. APEP group reported significantly better QOL (6.75). Secondly, we examine how large the difference / association exists. What was the magnitude of the effect? The [which group] [improved / deteriorated etc] by [how much?] in [which outcome measure] SPPB at discharge improved by an average of 5.12 within the APEP group. Finally, we report the variability of the magnitude of the effect using the 95% CI. This means that we are 95% confident that the mean lies within these margins. The greater the sample size, the narrower the margins become, or the more precise our estimation becomes (this relates to power calculations). What else is reported instead of the 95% CI? What were the 95% CI reported? QOL at follow up - 95%CI 0.3-13.8 Physical Performance – 95%CI 0.20-1.57 at discharge (Unadjusted value). 6.75 unadjusted for QOL at follow up and 0.26 adjusted. Between group differences may also be related to baseline differences, as well as the intervention. It is important to check whether the researchers considered these differences in the analysis [crude /unadjusted results and adjusted/multivariable analysis]. In effect, adjusted analysis adjusts each follow-up score for their baseline score, preventing an overestimation / underestimation of effect in the presence of a baseline imbalance. (Vickers & Altman, 2001) Note any adjustments and reflect on the values. Adjusted based on age, gender, frailty, baseline, physical performance, and fear of falling. (Both physical performance and QOL) Complete this for all the outcomes of interest. Finally, reflect on the results. For those outcomes that did not reach significance, can you suggest why? [think power calculations / intervention FITT / participants adherence or suitability]. LOS – Did not reach power calculations, underlying conditions. Steps – Conditions, how long after operation. SBBP (follow-up) - QOL at discharge (VAS SR) - Falls Follow Up - Death Follow Up - List the areas that are unclear to you here. You can review these at the end of the workbook to see what remains unclear. Area Comments Types of data (levels of measurement) There are 4 types of quantitative data in healthcare research: Nominal and Ordinal data which are referred to as category data. Scale data can be either interval or ratio and are referred to as continuous data. Each of these has certain measurement properties. Nominal data involves only classification or category. Basically, it has a name. Examples include eye colour (blue, green, brown), gender (male or female), religion (Christian, Muslim, Jew). There is no rank to them. Ordinal data involves categories that are ranked or ordered from least to most e.g. socio-economic status (lower class, middle class, upper class) contest results, race placing (1st, 2nd 3rd). Another example could be: (eg: Do you feel you exercise (a) very frequently (b) frequently (c) sometimes (d) rarely (e) not at all). These questions can be altered depending on the researcher (eg: some may drop the (d) rarely). The results can be ranked but the intervals between the data is not always the same Note, with ordinal the categories are ranked but there are not necessarily equal intervals between the ranked categories. For instance, there may be 2 seconds between the runners who come 1st and 2nd in a race, but there may be 5 seconds between the 2nd and 3rd placed runners. For health research, there are many outcome measures that we use, such as the Borg Rate of Perceived Exertion, which is categorical/rank data, because we cannot fully say for certain that each level is equi-distant. We can say that heart rate is equi-distant, or walking speed is, but can we honestly say the RPE is equi-distant? (recognising that its correlation with HR is excellent, we still can’t say with full certainty). However, while we define RPE here as rank/ordinal data, we can treat the data as continuous. Some outcome measures used in health may be made of composite scores (eg., QOL measures), while they are ordinal, they behave as interval. Usually this happens when the subscales are added up and the total score is used. Therefore, they can be treated as interval (see below). A similar composite scores is the Short Physical Performance Battery (https://geriatrics.ucsf.edu/sites/geriatrics.ucsf.edu/files/2018-06/sppb.pdf) is made up of some rank (balance test) and some interval (walking speed and chair stands), we treat the data as interval. Scale data refers to measurement on a continuous scale. Scale level measurement has ranked categories with equal intervals between the ranked categories. If the scale has an absolute zero (in other words, if “0” means “none present”), the data can be categorised as ratio. If the zero is not absolute, but the intervals are equal, the scale can be described as interval, so all mathematical operations apply, including proportion or ratio. Examples are time i.e. time taken to perform a task, age, weight, height, score on a standardised scale. Table : Types of Data Category Rank/Order Equal Intervals Absolute zero Nominal Y Ordinal* Y Y Interval Y Y Y Ratio Y Y Y Y Nominal is always categorical Interval and ratio is always continuous Therefore, we plot the data to see its distribution, and test with the SW test Please see https://statistics.laerd.com/statistical-guides/types-of-variable.php#:~:text=In%20some%20cases%2C%20the%20measurement,variable%20is%20treated%20as%20continuous.&text=At%20the%20same%20time%2C%20some,treated%20as%20a%20continuous%20variable. For further reading (I find this website very clear). *If it is a composite score, and acts like interval data, it can be treated as such Introduction to data analysis, how to prepare for the data analysis Introduction In the first phase of analysis, the data can be examined by producing mathematical summaries and graphs. You need to get to know your data. The following questions need to be answered to prepare for inferential statistical analysis on your data. Aim Let’s take the McCullagh et al (2020) as our example throughout the workbook. The aim of the trial was to determine the effects of additional exercise on length of stay, physical performance and quality of life, and healthcare utilisation in frail medical inpatients. Sample One hundred and ninety were recruited. Power calculations suggested we needed 220 participants, but for logistical reasons, the trial had to be terminated when 190 patients had been recruited. Variables The primary outcome measure was length of stay. The secondary measures Short Physical Performance Battery (a test measuring balance, sit-to-stand, and walking speed), quality of life (EQ5D5L). For this analysis, we will focus only on the following co-variables: age, gender, fear of falling, frailty score, and adverse events. Task 2: From the list above, categorise as dependent and independent variables. Dependent variable Independent variable LOS Additional Exercise PPB Age QOL Gender Fear of falling Frailty Which type of variable (dependant / independent) informs our choice of statistical test? Data Variables Type of data (categorical OR continuous) Length of stay (nights) Continuous Short Physical Performance Battery Categorical Step-count Continuous Frailty score Categorical Gender Categorical Age Continuous No of medications Continuous Fear of falling CategoricL In order to find this out, you will need to do a number of things. Firstly, you will need to know how each variable was measured. This will tell you your type of data. For these details, see Appendix B of the paper. Secondly, you will need to describe your data, which means you will need to get to know the data. This will be covered in the next section. Potential confounders Hint: See 2.4…. How to get to know the Data: Descriptive Statistics Introduction To know what to do with the data, you will need to know what type of data you have. Categorical/dichotomous/Binary data will be presented in numbers, with (%). It is useful to plot (graphically display) your data to get to know the distribution of the data. Categorical data can simply be presented in bar charts or pie charts (see below). If you have continuous data, you will firstly need to know whether it is normally or non-normally distributed. There are two graphs that will help you to determine the distribution, and a supplementary statistical test. Decide from the graph and confirm with the supplementary test – the test will not completely determine (but rather confirm) the distribution for you, the graph will be more informative. Graphs for Categorical Data Figure 3: categorical data summaries Bar graphs and pie charts– show the number or percentage of cases in particular categories Graphs for continuous data. Continuous Data When analysing the graphics, you should look for Central tendency – central, typical or representative score Variability/Distribution/Spread – the extent to which scores are spread about the central tendency Skewness – symmetry or asymmetry (hist only) Kurtosis.- peakedness or flatness around the central tendency (hist only, and not considered as often as the others) Central tendency can be reported as Mode – most frequently occurring score in a data set (seldom used) Median – middle score in a set of ordered data Mean – average Variability Range – difference between the highest and lowest scores Inter Quartile Range (IQR) - range over the middle 50% of ordered data Standard deviation (SD) - average extent to which scores deviate from the central tendency Figure 1: Boxplot distribution (continuous data only) With box plots, the line in the middle of the box is the median (central tendency) and the box itself represents the IQR (inter-quartile range) i.e. the spread of 50% scores about the central tendency (see measures of central tendency and variability below). The whiskers extend to the lowest and highest scores in the data set. If the data is normally distributed, the median will be in the middle, and the quartiles will be evenly spread around the median. The max and min (whiskers) do not have to be as balanced to be determined as normally distributed. You also may see an asterix (*) or dots beyond the min and max; these represent outliers. You can expect the larger the number of observations in your sample, the greater the likelihood of normally distribution. Conversely, the smaller the number, the greater chance of non-normal distribution. Less than 20 observations in a group should always be considered as non-normally distributed data. Figure 2: Histogram distribution (continuous data only) The second graph that can help determine the distribution is the histogram. The “peak” is the highest number of observations at that value. If the data is normally distributed, the “peak” should be central, representing the mean. If the “peak” is to the left (left-skewed) or right (right-skewed), the data is non-normally distributed. Sometimes, the histogram can show two or more “peaks” or a gap in a certain value; also suggesting non-normal distribution. Supplementary Tests for Normal Distribution In SPSS, there are two supplementary tests for normal distribution: the Shapiro-Wilk (S-W) for samples with ≤ 2,000 observations, and the Kolmogorov-Smirnov (K-S) for numbers greater than 2000. Therefore, the S-W test is the more commonly used test. From the table above, we can interpret the results of the SW test. Note, this tests normality. In other words, you are testing a hypothesis of normal distribution. Hence, if the sig. value is greater than 0.05, we can assume the data is normally distributed. If it is below 0.05, the data significantly deviate from normality, and can be considered as non-normally distributed. Therefore, in all groups, the data is normally distributed. Note: this test is supplementary to your interpretation of the histogram. Summarising data mathematically (in tables and text). To recap Central tendency Mode – most frequently occurring score in a data set (seldom used) Median – middle score in a set of ordered data Mean – average Variability Range – difference between the highest and lowest scores Inter Quartile Range (IQR) - range over the middle 50% of ordered data Standard deviation (s) - average extent to which scores deviate from the central tendency For continuous data (scale level), the mean and standard deviation are used to indicate the central tendency and variability respectively. However, if the data is skewed, or there are a few outliers, then the median and IQR are preferred as these are not affected by extreme scores. Reporting Descriptive/Summary Statistics Report measures of central tendency and variability. Normally distributed Non normally distributed Central tendency Mean (m) Median (med) Variability Standard deviation (SD) Interquartile range (IQR) Comment on the shape of the data (approximately normal or skewed) and any outliers. The SD is displayed in brackets (SD x) using the one number that represents the variability from the mean. The correct way to report SD is to report the mean, followed by the SD (mean 77.5, SD 7.4). The correct way to report the IQR (IQR x-y) is to report the median, followed by the range (median 18, IQR 30.5 - 48). Usually, research reports will present descriptive statistics (central tendency and variability) in the findings section. Task 3: Can you find the primary outcome in the paper? Findings (report the numerical finding) Table (number) Text (page & para) The effects of the intervention on healthcare utilisation (length of stay and readmission rates), physical perform- ance (SPPB) and QoL (EuroQol 5 Domain 5 Level Scale, EQ 5D5L ) were measured. Table 1 3 Reporting numerical summaries. Categorical/binary/dichotomous data With categorical data, the frequency within each category and (%) should be reported. Therefore, report the raw number initially, and the percentage of the total dataset in brackets This is a typical SPSS output of frequency data (the McCullagh et al data is displayed in this table). Task 4: From the SPSS output, complete the table below. Each category has been presented with a numerical/ alphabetical code (see below). Use these to gather the data from the SPSS output above. N (%) Smokers Smokers (=0) Ex-smokers (=1) Non-smokers (=2) Frailty category Pre-frail (=PF) Frail (=F) DCDestF Home (=0) Convalescence (=2) Rehabilitation (=4) Died (=6) Interpreting statistical outputs on continuous data Interpretation of output from Explore in SPSS. A lot of information is generated from the Explore command in SPSS, so it is important that you know what to look for. It produces a table labelled Descriptives, providing a range of descriptive statistics. Some of this information you will recognise (mean, median, standard deviation minimum, maximum etc.) The 5% trimmed mean may be new to you. SPSS removes the top and bottom 5 % of cases and recalculates a new mean value. If you compare the original and trimmed mean you can see if any extreme scores are strongly influencing the mean (would this suggest a skewed distribution?). In a second table, labelled Tests of Normality, you are given the results of the Kolmogorov-Smirnov statistic. A non-significant result (sig value more than 0.05) indicates normality. (You test the hypothesis that the data is normally distributed. If the score is less than 0.05, you must reject the hypothesis.) The actual shape of the distribution can be seen in the Histograms. The final plot provided in the output is a boxplot of the distribution of scores. The rectangular box in the centre represents 50% of the cases. The edges of the box represent the 25% and 75% interquartile ranges. Whiskers (line protruding from the box) represent smallest and largest values (excluding outlier, outliers are shown as circles). The line inside the rectangle is the median value. TASK 5: Analysis of the graphical display of continuous data Output from Descriptive provides information on central tendency (mean), on variability (standard deviation) and also on the shape of the distribution (skewness and kurtosis). Your choice of inferential statistical test will depend on the distribution of the data. If they are normally distributed, parametric statistical tests are required (e.g. t tests, analysis of variance). Otherwise, non-parametric equivalent tests are required (Mann Whitney U test, Kruskal Wallis rank sum test). How to determine normal distribution. Is the distribution approximately normal (symmetrical) or is the data skewed (bunched at one end)? Once the histogram follows the normal curve fairly well, parametric tests can be used. Similarly, they can be used if the IQRs are fairly symmetrical. If you have numbers less than 20 – 30 observations, the chances are the data will not follow the curve. It is reasonable to assume that anything less than 20-30 should be analysed with non parametric tests, and reported in medians (IQR). Are there any outliers? With histograms, any data points sitting on their own, out on the extremes are potential outliers. With boxplots, outliers appear as little circles or asterix. If you find points like this, you need to check that the outliers are genuine and not errors. if genuine outliers, decide what to do with them. This needs to be decided on a case-by-case basis. It means something, it is a measurement, and you need to reflect on this a bit before you decide whether to keep or remove it. Let’s begin by look at frailty. Each histogram represents one group (control / intervention). Task 6: Do you think the data is normally distributed. Why? Task 7: Analysing boxplots This box plot represents both groups. Can you identify the main points of information (you should have 5 points)? Would you report these points for normal or non-normal distributed data? Why? Tests of Normality group Kolmogorov-Smirnova Shapiro-Wilk Statistic df Sig. Statistic df Sig. age 1.00.112 30.200*.968 30.482 2.00.105 31.200*.946 31.118 FRAILTY_SCORE 1.00.133 30.184.954 30.215 2.00.108 31.200*.961 31.308 fesTOTAL 1.00.171 30.026.855 30.001 2.00.184 31.009.876 31.002 COMPL_EX 1.00.224 30.001.923 30.033 2.00.205 31.002.785 31.000 *. This is a lower bound of the true significance. a. Lilliefors Significance CorrectionV Do you think that frailty is normally distributed? Why? From this, what descriptive data would you report for frailty score? Task 8: Let’s take another example: fesTOTAL (falls efficacy) Tests of Normality group Kolmogorov-Smirnova Shapiro-Wilk Statistic df Sig. Statistic df Sig. age 1.00.112 30.200*.968 30.482 2.00.105 31.200*.946 31.118 FRAILTY_SCORE 1.00.133 30.184.954 30.215 2.00.108 31.200*.961 31.308 fesTOTAL 1.00.171 30.026.855 30.001 2.00.184 31.009.876 31.002 COMPL_EX 1.00.224 30.001.923 30.033 2.00.205 31.002.785 31.000 *. This is a lower bound of the true significance. a. Lilliefors Significance Correction Do you think that fesTOTAL is normally distributed? Why? What would you report for fesTOTAL? Why? Task 9: Read the table of summaries of continuous data below This is a typical SPSS output. Note the details in the red boxes for group 1 (age) Descriptives group Statistic Std. Error age 1.00 Mean 78.80 1.409 95% Confidence Interval for Mean Lower Bound 75.92 Upper Bound 81.68 5% Trimmed Mean 78.78 Median 80.00 Variance 59.545 Std. Deviation 7.717 Minimum 65 Maximum 93 Range 28 Interquartile Range 12 Skewness.020.427 Kurtosis -.866.833 2.00 Mean 80.52 1.491 95% Confidence Interval for Mean Lower Bound 77.47 Upper Bound 83.56 5% Trimmed Mean 80.69 Median 82.00 Variance 68.925 Std. Deviation 8.302 Minimum 65 Maximum 94 Range 29 Interquartile Range 14 Skewness -.436.421 Kurtosis -.811.821 FRAILTY_SCORE 1.00 Mean 3.74.233 95% Confidence Interval for Mean Lower Bound 3.26 Upper Bound 4.22 5% Trimmed Mean 3.77 Median 4.07 Variance 1.635 Std. Deviation 1.279 Minimum 01 Maximum 06 Range 5 Interquartile Range 2 Skewness -.431.427 Kurtosis -.691.833 2.00 Mean 3.32.233 95% Confidence Interval for Mean Lower Bound 2.84 Upper Bound 3.79 5% Trimmed Mean 3.37 Median 3.53 Variance 1.677 Std. Deviation 1.295 Minimum. Maximum 06 Range 6 Interquartile Range 1 Skewness -.767.421 Kurtosis 1.454.821 fesTOTAL 1.00 Mean 49.33 2.756 95% Confidence Interval for Mean Lower Bound 43.70 Upper Bound 54.97 5% Trimmed Mean 50.33 Median 55.00 Variance 227.816 Std. Deviation 15.094 Minimum 16 Maximum 64 Range 48 Interquartile Range 23 Skewness -.988.427 Kurtosis -.128.833 2.00 Mean 48.39 2.779 95% Confidence Interval for Mean Lower Bound 42.71 Upper Bound 54.06 5% Trimmed Mean 49.26 Median 53.00 Variance 239.445 Std. Deviation 15.474 Minimum 16 Maximum 64 Range 48 Interquartile Range 28 Skewness -.651.421 Kurtosis -.805.821 COMPL_EX 1.00 Mean 6.23.701 95% Confidence Interval for Mean Lower Bound 4.80 Upper Bound 7.67 5% Trimmed Mean 6.02 Median 6.00 Variance 14.737 Std. Deviation 3.839 Minimum 0 Maximum 17 Range 17 Interquartile Range 5 Skewness 1.024.427 Kurtosis 1.187.833 2.00 Mean 7.45 1.068 95% Confidence Interval for Mean Lower Bound 5.27 Upper Bound 9.63 5% Trimmed Mean 6.80 Median 6.00 Variance 35.389 Std. Deviation 5.949 Minimum 2 Maximum 25 Range 23 Interquartile Range 6 Skewness 1.791.421 Kurtosis 2.968.821 Note: without the histograms, is there any other information in the tables above that could hint their distribution? Complete the table below Normal / Non-normal distribution Median (IQR) Mean (SD) Min, Max Age unknown Frailty Score FES Total Completed EX Task 10: Convert the findings into text Convert the summary information on Frailty Score into text. Start with something like “the groups were similar/not similar at baseline (insert mean (insert SD) in the intervention group, and (insert mean (insert SD)) in the control group. You may question whether the groups can be statistically tested for differences. In some papers, the groups are statistically compared at baseline (to be explained in the next chapter), while in other papers, the authors simply show the summary descriptive data in a table, allowing the readers to read it themselves, and notice any differences. Conclusion: Reporting descriptive/summary statistics Report measures of central tendency and variability. Comment on the shape of the data (approximately normal or skewed) and any outliers. NB. If the data is skewed (non-normal), then the descriptive statistics presented to summarise the data should be the Median and the IQR, rather than the Mean and Std deviation. Traditionally, the descriptive statistics are reported together in Table 1. This reports the data at baseline. It is important in controlled trials, where you compare the outcomes of one group (control) to another group (intervention) that the groups are comparable at baseline. Table 1 presents these summary statistics to allow the reader to draw their own conclusions. Inferential data is reported in Table 2. This reports the tests that you have completed to determine whether a difference exists or not. You usually report in the Methods section, in subheading “statistical analysis” the text, that you tested the data for normality. (see below) Preparing for Inferential Statistical Analysis At this stage, we understand why it is important to know whether the data is categorical or continuous, and for continuous data, that the data has been plotted in histograms and boxplots, and tested for normality using the Shapiro-Wilk test (for less than 2,000 observations) or Kolmogorov-Smirnov test (in cases with more than 2,000 observations). The next stage is to decide what test we need to do to determine a statistically significant result. We can compare means to measure whether groups are statistically different, or linked. For categorical data, we can determine whether there is a greater risk /odds of one variable being observed in one category more than another. These are inferential statistics. Inferential statistics are tests that allow the researcher to make inferences about a population in the basis of data gathered from a sample or sub-set of that population. By the end of this chapter, you should understand the logic and terminology of statistical inference and be able to test the statistical significance of the difference in sample means. Hypothesis testing For a hypothesis to be testable, it must enable one to predict ‘the average expected outcome from a single investigation’. It must be measureable. A hypothesis is a statement of prediction based on theory or previous research. It must come from somewhere credible. Significance testing determines the probability that findings (e.g. differences between means) are due to chance occurrence. Step 1: Define your Hypothesis Statement Usually, editors and reviewers will look for a clear statement of your hypothesis. This (your alternate hypothesis) is usually stated at the end of the introduction. However, you must first start with your null hypothesis… Null hypothesis (Ho) conditions have no effect or no association between variables What if we have to reject this null hypothesis? Before we start testing, we need to define our alternative hypothesis. Alternative hypothesis (H1) conditions have an effect or there is an association between variables Note, it just tests the chances of a difference existing or not. It does not measure the difference effect size – the “by how much”. E.g. in study examining the effect of exercise intervention on discharge physical performance, and where the control group had a sham exercise intervention, the null hypothesis would state that there would be no difference in physical performance with the exercise intervention or the sham. The alternative hypothesis would state that there would be a difference. Step 2: Hypotheses - Direction of effect (1 tailed / 2 tailed) We also talk about the direction of a test. Here is an example to illustrate direction of effect with null and alternative hypotheses Example: Do we say: The intervention will improve discharge physical performance or the intervention may either improve / negatively affect discharge physical performance. If we can predict that the intervention will enhance performance (on the basis of theory or previous research) then we can use a one tailed test. However, if we are unsure about the effects of the intervention, whether it will improve or negatively affect physical performance, then we have to use a two tailed test – the effect can go in either direction Two-tailed hypotheses: Ho – The intervention will not affect physical performance H1 – The intervention will affect physical performance One-tailed hypotheses: Ho – The intervention will not affect physical performance H1 – The intervention will improve physical performance As a rule of thumb, you should use two-tailed unless you are completely sure that it can only go one way, or that if it goes the other way, it is not relevant to the study. We should measure the chances that the intervention can have negative effects as well as positive. The only problem is that you will require a larger sample size. Step 3: Setting your p-value level (probability of findings being due to chance) A hypothesis is tested by calculating a test statistic (e.g. t-test). This calculation takes into account the difference in means for two sample groups and also sampling error (standard error) which is determined by (i) the extent of the variability in the data sets and (ii) sample size (SE = s/√n). (Andy Scally will go through this again in the first lecture in January) Traditionally, if that probability is less than 5% (p<0.05), then the difference is statistically significant. This is not set in stone (it was chosen by a highly influential statistician in 1925’s, who selected this number as it was “convenient”, 2 SD away from the mean…). You will come across papers using p<0.1 (1 SD away from the mean) as they do not want to “miss” any possible links, and others using p<0.01 (3 SD away from the mean) as they want to be very sure. And so on… Decision errors: Type 1 and Type 2 error Always exercise caution with inferential statistics. We estimate probability of the findings being due to chance or sampling error (remember, the sample should represent the population). If the probability is less than 5%, then we can conclude our results are statistically significant. However, there is still a 5% chance that we are wrong – there is a 5% chance that we have selected an oddball sample. This is a type 1 error – finding an effect when there is not one in reality (the population). This can be referred to as a false positive (a false difference found, that really is not there; we just found it by chance). Conversely, a type 2 error is when our sample produces a result which falls in the accept region i.e. we do not find an effect although there is one in reality (the population). This is referred to as a miss (we missed the significant finding). Always bear in mind when you come across results that are statistically significant and an effect is claimed, that there is still a chance (albeit a small chance) that the result is wrong (type 1 error). Figure 4: Normal distribution and standard deviation Figure 5: Hypothesis rejection / acceptance Confidence Intervals So far, we have discussed how p-values can be used in hypothesis testing but there is another approach to statistical inference – confidence intervals and are usually reported as 95% confidence intervals; you are 95% confident that the mean lies within this range. As with p-values, the larger the sample size (n), the more precise your results, leading to narrower CI’s. The narrower the CI, the greater our certainty of where the true mean lies. When CI do not cross 0, (in other words, they are both either positive or negative), there is a positive or negative effect. For ratio scores (Relative Risk/ Odds Ratio, later in the workbook), when the CI does not cross 1, there is no effect. The information from each of these approaches to statistical inference (probability testing and confidence intervals) is complementary. Both should be reported. Bone density in women with depression Depressed M(SD) not depressed M(SD) Mean diff (95% CI) p value Lumbar Spine 1.0 ± 0.15 1.07 ± 0.09 0.08 (0.02 to 0.14) 0.02* Trochanter 0.66 ± 0.11 0.74 ± 0.08 0.08 (0.04 to 0.13) 0.01* Radius 0.68 ± 0.04 0.70 ± 0.04 0.01 (- 0.1 to 0.04) 0.25 (NS) (Michelson et al, Bone density in women with depression NEJM, 1996 1176-1180) The lumbar spine 95% CI does not contain zero, and p= 0.02 => difference is significant The radius 95% CI contains zero, and p>0.05 therefore difference is not significant Statistical tests to compare two sample means (t tests) (continuous data) Often we want to compare the mean scores for two sets of data to find out if a difference between them is statistically significant e.g. males vs females on a maths test or physical functioning scores for treatment and control groups following the clinical trial of an exercise intervention for hospitalized patients. A test commonly used for this is the t test. This is a parametric test because it uses the mathematical properties and parameters of a symmetrical distribution similar to the normal distribution (the t distribution). If your data reaches the criteria, you then have to choose the correct test (see Choosing Test). For normally distributed data, there are two types of t tests: Dependent (related) t test for related (repeated measures or matched pairs) Independent (unrelated) t test – for two separate (unmatched) samples. Parametric tests to compare means Task 11: Interpreting the output of an independent sample t tests Parametric tests are the t tests (independent or matched/paired). Non-parametric equivalent tests are the Mann-Whitney (independent) or Wilcoxon (matched/paired). Independent samples t-test Let’s assume the authors want to compare the BMI between groups at baseline. Was there a significant difference? Note the null (H0) and alternative hypotheses Note whether the test is one or two tailed Note the significance level (usually 0.05) Recap the steps – having explored the data, the authors have used the histograms and the SW tests to determine distribution. They have concluded that the data is normally distributed. Choose/ justify test: The authors have chosen the independent t test Two tables are displayed Group descriptive statistics Independent samples Test Group Descriptive statistics table Group Statistics group N Mean Std. Deviation Std. Error Mean BMI 1 30 25.92 6.115 1.116 2 31 26.07 7.142 1.283 Note the numbers in each group. Independent samples Test Table Independent Samples Test Levene's Test for Equality of Variances t-test for Equality of Means F Sig. t df Sig. (2-tailed) Mean Difference Std. Error Difference 95% Confidence Interval of the Difference Lower Upper BMI Equal variances assumed.684.411 -.085 59.933 -.145 1.705 -3.556 3.267 Equal variances not assumed -.085 58.148.932 -.145 1.701 -3.549 3.259 There are two lines of values, the correct one to use is indicated by the result of Levene’s test for equality of variances. This examines the two sets of data for equality of variance. The “F” is a measurement of the amount of variability between scores. To interpret the outcome, read the “Sig” score. If the F statistic (in the “Sig.” column) is significant then the two sets of data are not equal in variance and the bottom set of data must be used. If the F statistic (in the “Sig.” column) is not significant i.e. > 0.05 (95% level) then the two sets of data have acceptably equal variances and the top set of values may be used In the output table, SPSS reports the p value (sig 2 tailed), and the 95% CI for the difference between the means of the two samples. So in this example we have a p value (NS) of (p= 0.93) which is greater than 0.05 and therefore non-significant. The 95% CI cross the value of zero (95% CI, -3.556 to 3.267), further suggesting that there is no significant difference between the groups. Interpretation of independent t-test in text format. Therefore, we can conclude that in this sample there is no significant difference in BMI. Writing these results in text would be as follows: There was no significant difference in BMI between the groups at baseline (intervention group, 25.92 (SD 6.1), control group 26.07 (SD 7.1), (p= 0.93, 95% CI, -3.556 to 3.267). NB Levene’s test for equality of variance indicates that there is no statistically significant difference in the variances of the two data sets, one of the criteria for this parametric test (independent t test). Task 12: Interpreting a related/paired samples t-test and the output Let’s measure the difference in physical performance between baseline scores (SPPB_A) and outcome (SPPB_F). Once again, note the the null (H0) and alternative (H1) hypotheses, one or two tailed, and significance level (usually 0.05) Output is comprised of three tables: Paired sample statistics Paired samples correlations Paired samples test The Paired Sample Statistics table details descriptive statistics Paired Samples Statistics Mean N Std. Deviation Std. Error Mean Pair 1 SPPB-A_TOTAL 2.93 56 2.271.304 SPPB-F_TOTAL 3.70 56 2.296.307 The Paired Samples Correlations shows Pearson’s correlation coefficient with its significance. It thereby tests the correlation between our paired set of samples Paired Samples Correlations N Correlation Sig. Pair 1 SPPB-A_TOTAL & SPPB-F_TOTAL 56.728.000 The Paired Samples Test table contains the test statistic ‘t’, standard deviation, standard error, 95% CI, and the p value (2 tailed). If p < 0.05, the difference in physical performance pre and post intervention is significant. Paired Samples Test Paired Differences t df Sig. (2-tailed) Mean Std. Deviation Std. Error Mean 95% Confidence Interval of the Difference Lower Upper Pair 1 SPPB-A_TOTAL - SPPB-F_TOTAL -.768 1.684.225 -1.219 -.317 -3.412 55.001 Interpretation of paired t-test in text format. How to interpret the results in text format? Therefore, we can conclude that in this sample there is a significant difference in physical performance between the baseline and outcome scores (mean difference -7.68 (±1.68), p= 0.01; 95% CI, -1.22 to -.317). Non-parametric tests to compare two data sets If the following conditions for using t tests are not met, then non-parametric tests (based on the ranks of values in the data sets rather than actual values) should be used to test hypotheses: i) interval/ratio measurement ii) normal distribution iii) equal variance (approx) DESIGN NUMBER OF COMPARISONS PARAMETRIC NON-PARAMETRIC Unrelated 2 means/data sets Unrelated t test Mann Whitney. (un-paired) Related 2 means/data sets Related t test Wilcoxon (Paired) NB ……If the data is skewed (non-normal) and therefore does not meet the criteria for a parametric test and a non-parametric test is applied, then the descriptive statistics should include the Median and the IQR, rather than the Mean and SD. Task 13: Interpreting a Mann Whitney U (Non-parametric equivalent to the unrelated/ independent t test’s output In order to determine whether physical performance was different at the end of the intervention, we could run an independent t-test to compare means between the outcome scores (SPPB_FINAL). However, suppose the baseline (SPPB_A) scores were not comparable, any difference at baseline could affect the outcome. One simple way to deal with this, is to measure the difference in physical performance (SPPB_DIFF). Having “Explored” the data, we know that SPPB_DIFF is not normally distributed. Therefore, we must use tests for non-parametric data. The groups are independent, and therefore, we use the non-parametric equivalent of the independent t-test (Mann Whitney U test). The table presents the following results Test Statisticsa SPPB_DIFF Mann-Whitney U 378.000 Wilcoxon W 843.000 Z -1.268 Asymp. Sig. (2-tailed).205 a. Grouping Variable: group Interpretation of MWU test in text format. How to interpret the results in text format? Therefore, we can conclude that in this sample there is no significant difference in the changes in physical performance after the intervention (p=0.2). (The results do not report median (IQR) here, however, these should also be reported in the sentence). Task 14: Interpreting a Wilcoxon test: (Non-parametric equivalent to the related/dependent t test) The table presents the following results when we compare the SPPB scores between baseline and outcome. Test Statisticsa SPPB-F_TOTAL - SPPB-A_TOTAL Z -3.154b Asymp. Sig. (2-tailed).002 a. Wilcoxon Signed Ranks Test b. Based on negative ranks. Interpretation of MWU test in text format. How to interpret the results in text format? Therefore, we can conclude that in this sample there is a significant difference in physical performance between the baseline and outcome scores (p=0.002). (Once again, the median (IQR) should be reported in this sentence). Simple/Univariable Associations Often researchers are interested in the relationship between variables, otherwise known as bivariable relationships. Simple associations are concerned with exploring how the change in one variable is associated with change in another variable. For example, Is studying for longer (continuous variable) linked to better grades (continuous variable)? Does owning a dog (dichotomous) lead to better fitness levels (continuous)? Is there a link between Irish office workers (categorical) and the presence of heart disease (dichotomous) It can also be used to predict. This can be very useful. For example, can we predict Are more physiotherapy interventions associated with an earlier return to play? Is higher intensity training linked with better fitness levels in cardiac patients? Are more recently qualified physiotherapists more likely to use exercise prescription in their interventions? Task 15: Define the dependent and independent variable for each of the above Task 16: Identify potential confounder for each dependent variable. Independent Variable Dependent Variable Potential Confounder (3rd variable associated with ind/dep variable) 1 Studying Grades 2 Owning a dog Fitness levels 3 Office workers Heart Disease 4 5 6 It’s important to note at this point that the link/ prediction does not mean that one causes another to happen. There might be a link, but because the “independent variable” is not the only influence, we cannot say for certain that one causes another (more physiotherapy leads to earlier return to play, HIIT leads to better fitness, recent qualification leads to more exercise prescription). These other potentially influencing factors are known as confounding variables. Reporting a cause-effect is a common mistake. The only time that we can say that the independent variable causes a change in the dependent variable is in experimental studies (RCT). Even in quasi-experimental studies (when the participants are not randomly assigned), we cannot be sure of the cause and effect. Tests of association The table shows the presentation techniques and statistics that are used for examining the relationship between (i) two category variables (ii) two continuous variables. Level of measurement Presentation Statistic Both Nominal BD* Table Chi Square X 2 1 Nom & 1 Ord BD Table Chi Square X 2 Both Ord (few cat) BD Table Chi Square X 2 Both Ord (many cats) Scatterplot Spearmans Rho correlation coefficient Interval/Ratio Scatterplot Pearson’s r correlation coefficient BD* bivariate distribution Bi-variate distribution tables and Chi Square Test (x2) Bivariate table Cancer (nominal) by smoking habit (nominal) Smoking habit Smoker Non smoker Total Cancer 230 78 308 No cancer 465 652 1117 Total 695 730 1425 The above table shows data from a study investigating the relationship (link) between smoking and cancer. Of the 1425 people who participated in the study 695 (almost half) were smokers and of the smokers about 1/3 contracted cancer whereas of the 780 non-smokers only 78(about 1/10) contracted cancer. The question is ‘does this data shown a relationship between smoking and cancer and is it statistically significant ‘(is it unlikely to be due to chance- p<0.05)? We use an inferential test called Chi Square to determine the probability of our findings being due to chance. Pearson's chi-squared test (χ2) is a statistical test applied to sets of categorical data to evaluate how likely it is that any observed difference between the sets arose by chance. This involves comparing the frequency in each cell of the table (observed frequency, (o) with the frequency we would expect to find for that cell if there is NO association (expected frequency, e). Again, we begin with the concept that there is no association. Note: Chi square does not provide information on the strength of an association. We cannot say smoking causes cancer. In our study, we would like to see if the intervention was associated with less/more adverse events. Therefore, we will see if gender was associated with adverse outcomes from the study. Task 17: Cross tabulation and Chi square (x2) Output The SPSS Output window shows descriptive statistics, cross tabulation, chi squared test to compare adverse outcomes in women/men. (females are represented as “1”; males as “0”). The crosstabulation shows the number of adverse events in each group (female=1, male=0), with the total counts and percentages. female * AED Crosstabulation AED Total 0 1 female 0 Count 23 2 25 % within female 92.0% 8.0% 100.0% 1 Count 35 1 36 % within female 97.2% 2.8% 100.0% Total Count 58 3 61 % within female 95.1% 4.9% 100.0% Chi-Square Tests Value df Asymptotic Significance (2-sided) Exact Sig. (2-sided) Exact Sig. (1-sided) Pearson Chi-Square.860a 1.354 Continuity Correctionb.106 1.745 Likelihood Ratio.846 1.358 Fisher's Exact Test.562.364 Linear-by-Linear Association.846 1.358 N of Valid Cases 61 a. 2 cells (50.0%) have expected count less than 5. The minimum expected count is 1.23. b. Computed only for a 2x2 table The Chi-squared test reports the association between females and adverse events. The results indicate that there is no significant association between females and adverse events (Chi-square, p=0.35; Fisher’s Exact test p=0.56). Fishers exact test can be used when the sample size is >1,000. Some report it has better accuracy for small numbers.(http://www.biostathandbook.com/fishers.html). These tests will not tell us the level of association. However, they will give us the information required to complete a 2x2 table for reporting results. Task 18: Complete the 2x2 table below. Adverse Outcome No Adverse Outcome Total Female Male Report the significance and test used underneath in text format. Relative Risk (RR) & Odds Ratio (OR)1 The strength of an association between category data can be summarised and interpreted using relative risk (RR) and odds ratio (OR) statistics. Epidemiological studies often explore risk factors (eg. smoking) for diseases (eg lung cancer). The dependent variable is usually categorical (lung cancer: disease present / absent). These studies are often cohort and case-controlled studies and would usually report their findings using RR and OR. Cohort studies are prospective in nature and involve follow up. Normally the sample (the cohort) will be measured at baseline, at the beginning of the intervention / exposure, and are followed up for a period of time (eg. For 10 years / until disease onset). They involve a fixed cohort e.g. birth cohort: all those born in 1984, industrial cohort: all those working in the nuclear power industry. The number of participants is considerable. It can be in the 1,000s frequently. The main reason is because the outcome variable is binary categorical (the disease / no disease). Therefore, power calculations would indicate greater numbers. If the studies are case controlled, it means that the group are compared to a control group (who have not had the intervention /exposure). They are also not randomized. Case control studies are retrospective in nature, looking back from disease to identify exposure to risk factors. Example: Cohort study: smoking and lung cancer Here is an example of a cohort study to investigate the link between smoking and lung cancer. The common ways (statistics) for assessing the relationship between the two variables are relative risk and odds ratio. Cohort Study: Smoking and Lung Cancer Lung cancer No Lung cancer Total Incidence rate Smokers 39 (a) 29 961 (b) 30 000 (e) 1.3/1000/yr Non-smokers 6 (c) 59 994 (d) 60 000 (f) 0.10/1000/yr 45 89 995 90 000 Relative risk (RR) – summarizes the strength of the association between the risk factor and the disease. RR = incidence among exposed Incidence among non-exposed In other words RR = (smokers) lung cancer / (lung cancer + no lung cancer) (Non-smokers) lung cancer / (lung cancer + no lung cancer) Using the notation in the table above the RR among the exposed is a/e and among the non-exposed is c/f. RR = 39/ 30,000 6/ 60,000 This ratio is called the relative risk. It equals: ae/bf, which is the cross-product of the table. a x f / b x e i.e. (39 x 60,000) / (6 x 30,000) Because these are ratios, differences are detected when they are above or below 1 (not zero). RR>1 - risk of disease greater among those exposed RR<1 – risk is lower among those exposed (factor protective) RR = 1.3/0.10 = 13 This result suggests that smokers are thirteen times more likely to develop cancer than non-smokers. Odds ratio An alternative measure of incidence is the odds of disease to non-disease. This equals the OR = total number of events Total number of non-events (at the end of the study). In other words OR = Events / non-events (smokers) Events / non-events (non-smokers) Using the notation in the table above the odds among the exposed is a/b and among the non-exposed is c/d. OR = 39/ 29,961 6/ 59,994 This ratio is called the odds ratio. It equals: ad/bc, which is the cross-product of the table. a x d / b x c i.e. (39 x 59994) / (6 x 29961) The odds ratio estimated from the lung cancer study table equals 13 (OR = 13). This means smokers are 13 times more likely to develop cancer than non-smokers. NOTE: the less frequent the observation, the more likely the RR and OR will produce the same result. Let’s take another example. Case control study of cancer of the pancreas and coffee consumption Coffee drinking Cases Controls Total Yes 140 (a) 280 (b) 420 No 11 (c) 56 (d) 67 Total 151 336 487 OR - The odds ratio is the cross product ratio of the table i.e. ad/bc So the odds ratio is 2.5 This means that coffee drinkers are 2.5 times more likely to develop pancreatic cancer than those who don’t drink coffee. Does this mean that it should be illegal to drink coffee? There may be other factors/variables that may affect or account for the relationship between coffee drinking and cancer of the pancreas. Remember, we referred to these as potential confounders. In order to control for potential confounders in case control studies, the researcher may use a matched pairs design. In this type of study, subjects are matched in terms of key characteristics that may affect the findings from the study. This tries to mirror RCTS; that the groups are more comparable at baseline; any potential confounders can cancel each other out. In many studies, when patient selection or the intervention cannot be manipulated, this is a good way to strengthen your results. Correlation Two techniques are used to study the relationship between two continuous variables: Correlation analysis – concerned with nature direction and strength of the relationship Regression analysis which is concerned with prediction of one variable based on knowledge of a value of the other variable These are two techniques that although related, serve different purposes. Graphical presentation Scatterplots are used to explore the relationship between two continuous variables (e.g. age and self-esteem). You should always generate a scatterplot before calculating correlations. This will show whether or not the variables are related in a linear fashion. Only linear relationships are suitable for correlational analyses. * * * * * * * Y Y * * * * * * * * * * * X variable X variable The idea of correlation can be seen more clearly if we plot the data. Each axis represents a variable. The dots represent a pair of scores e.g. weight and height, blood pressure and age. These graphical representations are called scatterplots (e.g. height and weight). The first graph shows a positive correlation i.e. the values on one variable increase as the values of the other variable increase. The second graph shows a negative correlation i.e. the values on one variable increase as the values of the other variable decrease, hence the pattern indicates a line of plots falling from left to right Correlation coefficient We can describe both the strength and the direction of a relationship between two variables by calculating a correlation co-efficient. The size of the correlation coefficient tells us about the degree of closeness of the relationship. It has a value ranging from 0 (no correlation) to 1 indicating a perfect correlation. A value of 0.8 indicates a strong relationship whereas 0.2 indicates a weak relationship Correlation coefficient -1 0 +1 Perfect No Relationship Perfect Negative Positive A plus (+) or minus (-) sign indicates the direction of the relationship, positive or negative Pearson’s r is a parametric statistic used with scale (continuous) data Spearman’s Rho is used when the data is ordinal (with many categories) or when scale data do not meet the parametric criteria required for the Pearson’s r. There are a few points to note about the correlation technique: Correlations are not causal designs; therefore, we cannot talk about cause and effect. Correlation depends on other variables being kept constant e.g. you would find a correlation between body weight and spelling but not when age is included (in other words, age is a potential confounder) Correlation is specific to the range of x studied - what happens beyond this range is not necessarily the same It is never safe to interpret a correlation coefficient without a scatterplot – as a coefficient is vulnerable to misinterpretation Correlation coefficient misinterpretation * * * * * * * * * * * * * * * * * * Correlation within clusters Outlier(s) responsible swamped by relationship for all or most of between clusters correlation Correlation coefficient misinterpretation * * * * * * * * * * * * * * * Outliers grossly Floor and Ceiling effects depress correlation depress the correlation Always plot data before calculating and interpreting a correlation co-efficient Task 19: How to interpret a scatterplot: Investigate the bivariate associations with continuous variables: correlation Here we will be examining the strength of association between two continuous variables in a linear form. We would like to see whether physical performance or frailty scores at baseline are associated with age From this, we can see that as participants get older, their physical performance deteriorates. (Negative correlation) Here, as participants get older, their frailty increases (positive correlation). Task 20: interpret the correlation co-efficient Correlations age SPPB-A_TOTAL age Pearson Correlation 1 -.318* Sig. (2-tailed).012 N 61 61 SPPB-A_TOTAL Pearson Correlation -.318* 1 Sig. (2-tailed).012 N 61 61 *. Correlation is significant at the 0.05 level (2-tailed). The table reports that there is a negative association between age and SPPBA_TOTAL. The co-efficient is 0.318 (a small to moderate association). This relationship is significant at p=0.012. The Spearman’s Rho Correlation uses the same form of SPPS computation and can be used for data that is ordinal or for scale data that is not normally distributed. REMEMBER: Always plot data before calculating and interpreting a correlation co-efficient Task 21: Review Table 1.2.3. (Page 8) What remains unclear? Appendix A: Choosing A Statistical Test By now you should be aware that there are different types of data with different levels of measurement precision – Nominal, Ordinal, Interval and Ratio We have looked at the various ways you can describe and summarise your data (this should be the first step in analyzing or making sense of data – summarizing and exploring it, - looking for patterns and looking at the shape of the data – to see if it’s symmetrical or skewed and if there are any outliers The next step involves applying an inferential statistical test to test a hypothesis. Remember - tests of statistical significance determine the probability of findings being due to chance (sampling error). If p<0.05 then the results are statistically significant and we can then reject the Null hypothesis and accept H1. (Is there is a difference between groups/ conditions or there is an association between two variables). So far we have looked at statistical tests for difference between two groups. e.g. if you wanted to test the difference between two means, you would use an independent t test if the design was unrelated i.e. two separate groups and dependent t test if the design was related (i.e. repeated measures or matched pairs.) If the same two groups/data sets are to be compared but they did not meet the criteria for a parametric test, then you would use the non-parametric Mann Whitney test for an unrelated design (two separate groups and the non-parametric Wilcoxon test for a related design (repeated measures or matched pairs). We have also looked at statistics for investigating an association between two variables - bivariate associations (cross-tabulation and Chi square for category data and scatterplots and Persons r for continuous variables) Specifying a statistical test There are lots of statistical tests and stats packages expects the user to specify the correct test for a specific question and type of data. This session explains how to choose the correct statistic using the flow chart. A statistic should be chosen for one hypothesis or research question at a time. The flow chart illustrates the factors that you need to consider: type of question you wish to address (association or difference), nature of the data that you have for each of the variables (nominal. ordinal or scale) assumptions that must be met for parametric statistics design of the study (unrelated or related (repeated measures or matched pairs). number of data sets (groups/variables) DATA ANALYSIS - CHOOSING A TEST Type of test Level of measurement Assumptions Design 2 groups Appendix B: Outcome measures To measure frailty, the SHARE-FI tool has been chosen as it is a valid and simple measurement of frailty. Five SHARE variables approximating Fried’s frailty definition are used: fatigue, loss of appetite, grip strength, functional difficulties and physical activity. Scores range between 2.7 to 13.4 and the SHARE-FI calculators (gender-specific) are freely available on the web to interpret the level of frailty. Using this calculator, patients can be categorised as frail, pre-frail, not frail. Physical Performance is measured using the Short Physical Performance Battery (SPPB), which includes walking speed. The SPPB has been chosen as it is quick, practical and safe to use with this population. The scores range from 0 (unable to stand independently,) to 12 (independent tandem balance for 10 seconds, able to walk 4 metres within 4.82 seconds and sit to stand 5 times in 11 seconds). Walking speed is known to be a strong indicator of patients’ physical performance and is an independent predictor of survival and institutionalisation. All patients scoring less than 1 on the SPPB will be eliminated from the study to allow us to detect functional decline while in hospital. At baseline only, fear of falling is measured using the Falls Efficacy Scale-International (FES-I). This tool consists of 14 activity-related questions. The questions aim to determine how concerned older adults are about falling while performing these activities on a scale of 0 (not concerned at all) to 4 (very concerned). 1. Romero-Ortuno R, Walsh CD, Lawlor BA, Kenny RA: A frailty instrument for primary care: findings from the Survey of Health, Ageing and Retirement in Europe (SHARE). BMC geriatrics 2010, 10:57.2. Guralnik JM, Simonsick EM, Ferrucci L, Glynn RJ, Berkman LF, Blazer DG, Scherr PA, Wallace RB: A short physical performance battery assessing lower extremity function: association with self-reported disability and prediction of mortality and nursing home admission. Journal of gerontology 1994, 49:M85-94.3. Delbaere K, Close JC, Mikolaizak AS, Sachdev PS, Brodaty H, Lord SR: The Falls Efficacy Scale International (FES-I). A comprehensive longitudinal validation study. Age and ageing 2010, 39(2):210-216. For a clear explanation of the difference between RR and OR https://stats.seandolinar.com/statist\ics-probability-vs-odds/ https://www.theanalysisfactor.com/the-difference-between-relative-risk-and-odds-ratios/↩