Psyc 200 Study Guide PDF
Document Details
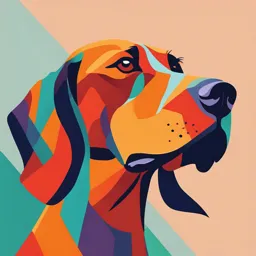
Uploaded by RestoredKeytar
University of Richmond
Tags
Related
- Psychological Statistics Prelims Reviewer PDF (A.Y. 2024-2025)
- PSYC 204 Introduction to Psychological Statistics - Week 1 Lecture Notes (2024-09-03) PDF
- Research Methods and Statistics PDF
- Psychology Past Paper PDF
- استخدام الاحصاء في علم النفس PDF
- PSY 201 Introduction to Statistics for Psychology I Lecture Slides PDF
Summary
This document is a study guide for a Psyc 200 course, covering topics in statistics and research methods. It includes definitions of variables, experimental designs, and statistical concepts, aiding in understanding and applying psychological research methodologies .
Full Transcript
Why is disseminating information integral to science? How do Statistics help with this? To reduce cognitive load What are the two main regulatory boards who monitor and approve ethical science? IRB General definitions of statistics? What is the general purpose of statistics? What makes a good sta...
Why is disseminating information integral to science? How do Statistics help with this? To reduce cognitive load What are the two main regulatory boards who monitor and approve ethical science? IRB General definitions of statistics? What is the general purpose of statistics? What makes a good statistic? Statistic definition– its a numerical value, graphical figure or output analysis that’s meant to represent a larger subset of data Statistics are also a language, we use to interpret this data A good statistic is succinct and representative (each stat included is equally weighed against the other observations, single value explains behavior of larger group) Be able to identify data, variables, data sets, independent and dependent variables and levels of variables. A collection of variables = data set Collect data= collecting # of variables Independent variable– something we are changing. dependent variable– something that we hope to see the change in. The change is dependent on the change we make (independent variable) Qualitative variables Quantatative variables Discrete variables – each level of the variable is ‘whole’-- does not allow for any intermediates. Ex. hair color, eye color, movie, gender Continuous variables– each level can have a theoretical infinite number of levels between. Ex. time to respond to a question (no discrete steps) 1.642371 Levels of IV– different presentations of IV (common levels of IV: control vs experiment; placebo vs not) What is an extraneous variable? What kinds of extraneous variables exist? What makes an extraneous variable a confounding variable? Extraneous variables– other variables not directly accounted for by the experimenter which may impact the results if not controlled for. – environmental variables: easy to control for should be done before the start — Personal Variables– difficult to control for, usually not considered unless important A confounding variable– those that change systematically with the variables of interest (IV and DV) Extraneous variables that are related to our relationship of interest are confounding. (make it harder for us to understand the true nature of the relationship. Sources that could impact results) What is a control group? Why is it called a negative or null control? A control can be maintaining consistency between experimental sessions, or a control group An experimental group with a known / predictable outcome to compare against the experimental group which is known as a null or negative control group. Study this one Define and be able to identify: qualitative and quantitative variables, discrete and continuous variables. Qualitative variables Quantatative variables Discrete variables – each level of the variable is ‘whole’-- does not allow for any intermediates. Ex. hair color, eye color, movie, gender Continuous variables– each level can have a theoretical infinite number of levels between. Ex. time to respond to a question (no discrete steps) 1.642371 Qualitative variables= discrete Quantitative variables= continuous Butttt there are some excpetions (what are those?) Be able to define and identify the scales of measurement (nominal, ordinal, interval, ratio) Least complex to most complex: Nominal, ordinal, Interval, Ratio Nominal– names or categories. Levels of a variables are different only by title, they don't have a specific order to them (eye color, restaurant style) Ordinal scale– levels of a variable are unique from one another, and HAVE AN INHERENT ORDER to them. (income brackets for tax, satisfaction scales) allows for comparisons Interval Scale– levels of a variable are ordered, and have a SET DISTANCE OR MAGNITUDE BETWEEN EACH LEVEL. (Temperature in C or F, Likert scales) Ratio Scale– name categories for each object (#’s serve as labels), same difference at two places on the scale (interval) AND 0 is real and meaningful. (height, weight, salary, most quantifiable variables) Remember NOIR (black in french) for order least to greatest Side Note: Categories– different numbers or names express different things (green is different from blue, 1 is different from 10) Rank order– items follow an inherent logic of sequentiality, the order items are presented in make a meaningful difference. Why doesn't it make sense to represent class standing as “Sophomore, Senior, Junior, Freshman? Equal spacing– the magnitude between values is consistent A 1 degree difference is the same distance weather 0-1 or 99-100 True 0– a measurement of 0 represents a true lack of observation (STUDY THIS ONE) What scale of measurement are likert scales? How are they treated in the field? What is the rationale for this discrepancy? Likert Scale– ordinal although commonly abused and treated as interval It's a rating scale used to measure attitudes, opinions or perceptions by asking participants to rank their level of agreement or disagreement with a series of arguments. Rationale: Psychologists don't know whether it serves as true numerical differences or just ranked categories. With more scales 7 - 11 plus tend to behave more like intervals STUDY MORE What is the relation between populations and samples? Why do we collect sample (purpose and rationale) We collect samples to make inferences about a population Population– as wide or narrow as we define it, as long as trait is shared Sample– any subset of a population Sampling bias– conclusions only apply to our sample Types of Sampling Exist? Which is best and why? Simple random sample– every member of the population has an equal chance of being selected into a sample; choses a sample by pure chance small sample= not representative Large sample= more representative Stratified sampling– conducts random sampling along specific guidelines Making sure both groups are equally represented to ensure good statistics Convenience sampling– using a sample that’s convenient to collect All my data collection this semester will be convenience sampling Convience sampling is the best because it’s quicker and efficient (check answer) WILL WE NEED TO KNOW THE DIFFERNT RESEARCH DESIGNS? IE. QUASSI-EXPERIMENTAL DESIGN NON-EXPERIMENTAL DESIGN What forms can distribution take? Be able to identify them in a bar chart/histogram as well as by using measures of central tendency. Central tendency= average (where the center of a distribution tend to fall) Average= Mode, Median, Mean Mode– the most commonly observed level of the variable. Which level has the highest f (highest peak or bar) ○ Unimodal– one peak ○ Bimodal– two peaks ○ Multimodal– many peaks ○ Major mode= ? ○ Minor mode= ? (if two values are not exactly tied, but obvious peaks exist) Mode is resistant to change of values or number of observations, only cares about most popular level. Shown in nominal data Median– where 50% of the scores are above it, and 50% of the scores are below it. ○ Median is sensitive to the number of observations in a data set, less sensitive to values ○ Ordinal data Mean– average of values in a data set, representing the balancing point. ○ 𝜇, 𝑀, 𝑜𝑟 𝑥ҧ = sum x/ N ○ Best measure of central tendency for continuous data ○ Mean is sensitive to both the number of observations in a data set, and to the values of those observations. ○ Sum of distance between mean and the scores of data set will always be 0 (WHAT DOES THIS MEAN) Uniform distributions– do not change as levels change (probability of rolling a single number of 6 sided die) Normal distributions– have a peak in the middle and slowly taper off equally on either side Skewed distributions– have a much longer tail to one side than the other ○ Positive skew– have the long tail going towards the positive (right) side ○ Negative Skew– have the long tail going to the negative (left) side Central tendency to identify Skew Outliers cause skewed distributions If Mean= Median= Mode – normal distribution If meanmode= positive skew STUDY THIS When is each measure of central tendency used and why are those most appropriate? Mode= nominal data only, while not the middle showcasing the most prevalent levels is the mose succinct (what does this mean) Median= used for ordinal data only, since there’s a middle and the value is not relevant to ordinal logic Mean– used in continuous data only, rarely falls on real number continuous allows for intermediates (STUDY THIS ONE) When people mention an average, which measure of central tendency are they referring to? What type of mean do we use in this course? They are referring to the mean We are discussing the arithmetic mean Why is central tendency considered a good statistic? Why is a measure of central tendency often paired with a measure of variability? Because it expresses where most people score. It gives us a central point of the distribution (for mean=unbiased statistic) Central tendency is often paired with measures of variability to give one an idea of what the distribution might look like, by telling us where it’s centred and how spread out it is Why is understanding variance important especially in psychology? LOOK OVER THIS Humans are inherently diverse organisms; humans are too variable (individual variations) to use as a model organism when trying to find systematic differences It's a characteristic of a sound mental state Variability= how spread out a data set is (a group of scores are) It measures how much the values in a dataset differ from each other and from the central tendency. What is the systematic difference? Why is it important to establish this difference in the sample group? Systematic Differences– A difference that follows a clear pattern instead of happening by chance Ex. students who do homework get good grades. Students who sleep more than 7 hours every night perform better on memory tests than those who sleep less. Its important to identify this in a sample group because if seen in a sample it most likely persists in the larger population. What are the main measures of variability? When is it best to use each? Range– The distance between the highest and lowest score ○ Interquartile range- the 50 percentile points centered around the mean ○ For discrete variables count # of levels ○ For continuous variables Formula = Xmax - Xmin ○ And describing variability of ordinal data Variance– The mean of squared deviance scores in a distribution ○ The average (squared) distance between an observation and mean. Standard deviation– the square root of variance ○ A corrected/adjusted average distance between an observation and the mean How would you use the interquartile rule to identify outliers? Interquartile range= range between 25th and 75th percentiles of observations in a distribution ○ Most commonly used in continuous data ○ And when describing variability of ordinal data ○ Divide into 4 equally portioned sections Steps are: 1. First, organize ordinally (1,2,3,4) in order 2. Count the number of variables and divide into 4 equally portioned sections 3. Find the rand of the inner 2 (highest - lowest) Example : First, organize ordinally... N = 20, 20/4 = 5 – each quartile should have 5 values in it. Find the range of the inner 2 (highest – lowest). 30, 30, 30, 37, 43, 43, 43, 44, 48, 52, 54, 55, 57, 59, 60, 61, 62, 62, 65, 66 Range = 66 – 30 = 36, IQR = (60-43) = 17 ****How would you use interquartile range to determine outliers? HOW DO YOU DETERMINE OUTLIERS USING INTERQUARTILE RULE SLIDE 18 IN 1ST VARIABILITY SLIDE SHOW In calculating variance, why do we square residuals? 1. Helps to accentuate extreme scores far from mean (easily identify outliers) 2. Helps remove negative values from the equation 3. Remember that the mean is a fulcrum and will always equal 0 Variance measures how spread out the values in a data set are. The average squared distance from the mean. However because its squared its not statistic is not the same as the original data. (no longer gives an accurate representation of distance between scores) So we inverse it and take the square root of the variance to give us the standard deviation to get back closer to the original score. Equations for standard deviation: What is the relationship between variability and predictability/reliability? Why? High variability reduces predictability and reliability, leading to uncertainty and inconsistency. Low variability increases predictability and reliability, making it easier to forecast outcomes and trust measurements. Reliability— the ability for the same assay/test/assessment to get the same results in similar situations. Validity– the ability for the test to correctly measure what it is intended to measure (accuracy) Measurements of reliability and validity help us to ensure that we are accurately measuring a contrstruct using a particular operational definition. ○ Constructs– intangible or difficult to measure phenomena to direct observations. ( we use operational definitions to define how a measurement will support or refute an internal construct) Ex. self reports, external behavior, physiological processes Why is a sample standard deviation a biased estimate of the population standard deviation? What direction is it biased in/how is it biased? Why do we not have these concerns for arithmetic mean? (writing question) COME BACK TO THIS QUESTION Using N, instead of n-1, produces a biased statistic What are degrees of freedom? How does the calculation of DF differ for measures of variability and central tendency differ? Why? Degrees of freedom (DF)-- the number of pieces of information we have to assess or estimate our statistic ○ DF= N-1 for measures of Central tendency; Since all n data points contribute freely to the mean ○ DF= N of variance; The degrees of freedom are reduced by one because the mean (Xˉ) is already estimated from the data, imposing a constraint on the variability calculation. What is causality? How can we increase our likelihood of finding causal links or results? Causality– the relationship between two variables where a change in one variable (cause) directly leads to a change in another variable (effect) ○ Temporal sequencing– our IV happens before our DV We give our treatment, then measure the change in DV ○ Non-spurious relationship– IV is not related to DV by chance Purpose of hypothesis testing “there’s a low probability these samples are from the same population ○ Eliminating alternative causes– There is no other variable to explain the relationship This is why well designed experiments are the only types of research that explore causality Randomly assign people to treatment and control groups to eliminate bias then record the results Define reliability and validity. Why is psychology especially sensitive to these concerns? Why is it difficult to identify valid but not reliable results, especially in psychology? Measurements of reliability and validity help us to ensure that we are accurately measuring a construct using a particular operational definition. ○ Constructs– intangible or difficult to measure phenomena to direct observations. ( we use operational definitions to define how a measurement will support or refute an internal construct) Ex. self reports, external behavior, physiological processes A process could be valid (accurate in what they are measuring) but not reliable (different results each trial). Reliability is the foundation for validity as without consistency in a measurement it becomes unclear whether an instrument truly captures the intended construct or if observed validity is just random. Reliability— the ability for the same assay/test/assessment to get the same results in similar situations. Validity– the ability for the test to correctly measure what it is intended to measure (accuracy) What are constructs? What is an operational definition? Constructs– intangible or difficult to measure phenomena that are resistant to direct observation. ○ Personality traits, emotional states (fear), attitudes, and abilities (athleticism) Operational Definitions– definition of a variable in terms of precisely how it is to be measured. ○ Self reports ○ Behavioral measures– aspects of a participants behavior is recorded or observed How do we measure reliability? Be able to define the three types of reliability discussed in class. Test- Retest Reliability– examines consistency over time in the same people ○ Assessed through correlation of multiple testing times Internal Consistency– consistency between questions addressing the same construct ○ Assessed through split-half correlation and Cronbach (WHAT'S cronbach?) Inter-rater reliability– consistency between researchers/scorers ○ Assessed through correlation of multiple scorers What types of validity exist? Why is validity more difficult to measure compared to reliability? Face validity– the amount to which something appears to measure the correct construct ○ Easy to assess, but generally a weak informal measure ○ What this measures at face value Content validity– do the measurements of the construct match all aspects of the construct. ○ If we believe something has a bhavior reaction and psychological component, our measurements should cover both these characteristics Criterion validity– how well do the measurements for this construct match other measurements for the construct? ○ Inverse of discriminant validity (ow well can a test differentiate different constructs) ○ If you develop a new measure of depression, does it correlate with existing measures (hamilton depression rating scale) Validity is harder to prove because: — Validity requires theoretical justification and external evidence. — There are multiple types of validity, making assessment more complex. — A measure can be reliable but still invalid, requiring additional steps to confirm validity. When deciding what type of test/assay/treatment to use, what types of validity should NOT drive our decision making? Face validity as its generally a weak informal measure of what we see at face value. And Content validity as it checks weather a test covers all aspects of a concept it is supposed to measure. It’s unreliable on its own as even if the test includes all relevant components it doesnt prove it actually measures the right construct and leads to accurate predictions. ○ Example: A cancer diagnostic panel might test for multiple genetic markers (good content validity), but if those markers don’t actually predict cancer, the test is useless. What characteristics are required for a good graph? When should you use a histogram vs a bar chart? Characteristics of a good graph: Graph title– often the effect of IV on DV Legend– useful to identify different shapes or line types Trend Line– especially in big data sets, shows line of best fit Clear data- individual data points, averages, and error bars should be easily identifiable X- axis IV Y axis DV Histograms– uses connected bars to represent different levels of a variable, used in continuous data Bar Charts– uses disconnected bars to represent different levels of a variable, used with discrete data What are the characteristics of a frequency table? What differentiates a grouped and ungrouped frequency table? What cost/benefit trade off for grouping data? Characteristics: Summarizes data presented ○ Helps reduce cognitive load F denotes frequency (the count, how many) Ungrouped frequency table: Every level of the variable is represented with it’s count Even levels with f=0 are included Continuous, discrete data often ungrouped Ex. 1=3, 2=4, 3=7 Grouped frequency table: Equal magnitude, start at the lowest observation, have a number of groups= range/magnitude ○ Or magnitude = range/ # of groups Make it easier to understand while sacrificing some nuance ○ Generally if more than 10 levels of continuous variable you should group Ex. A Variable: 0-3 = f: 4, 4-7 = 11 Pros: reduces cognitive load Cons: oversimplification may lose crucial information What are the characteristics of a normal distribution? Why does psychology use normal distributions so much? Normal distribution— a classification we can apply to datasets that have particular characteristics ○ Symmetric around the mean ○ median=mean=mode ○ Distribution has a mean and standard deviation ○ Area underneath curve= 1 ○ Distribution is dense in the center and less dense at the tails Psychology uses normal distribution so much because most naturally occurring dta, given a large enough sample size, will usually form a normal distribution What is a Z-score? What are some benefits of using z/standardized scores? Zscore– a collection of z-scores drawn from a known population ○ Z score is a standard normal distribution (b/c its population) ○ Z score can be positive or negative (relative to the mean) ○ Z score = # of standard deviations between X and mean ○ Centered around mean of Z=0 ○ Asymptotic theoretical frequency distribution ○ Larger z score implies further from mean ○ Sign tells us direction, value tells magnitude Benefits Standarizing variables: ○ Comparisons of variables across different scales. ○ Allows us to see how spread differs between variables ○ Allows for more direct comparisons because all the values are on the same plane now ○ Identifying outliers Measure significance (commonly used in hypothesized testing) Be comfortable computing z-scores from raw scores, and vice versa (skills packet). What is probability? Be able to calculate the probability of simple events. Probability– likelihood of an event occurring ○ Calculate– dividing the number of hits or successes by the number of attempts or outcomes Be comfortable finding the probability of events in a normal distribution (using a normal probability table). Develop practice problems similar to in class ones. Found in lecture 02/05 What is the central limit theorem? How does it integrate with our work in inferential testing? What is the distribution of sample means? How does it relate to CLT and why is it important for hypothesis testing in psychology? What characteristics of the population or the sample need to be present for the sampling distribution to be normally distributed?