PS 295 Week 4 2024 Post (1) PDF
Document Details
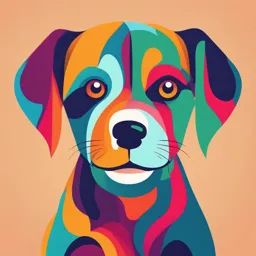
Uploaded by NimbleMoldavite7730
WLU
2024
Tags
Summary
This document provides an outline for Week 4 of PS 295, covering research ethics, including ethics in human and animal research, research misconduct, data analysis practices, open science reforms, and common types of measures. The document also includes an assignment description and guidelines for completing an academic integrity quiz.
Full Transcript
Week 4 Outline Research Ethics Ethics in research with humans Core principles Tri-Council policy – TCPS2 Review process at WLU Ethics in research with animals Research misconduct Blatant dishonesty or fraud Data an...
Week 4 Outline Research Ethics Ethics in research with humans Core principles Tri-Council policy – TCPS2 Review process at WLU Ethics in research with animals Research misconduct Blatant dishonesty or fraud Data analysis practices Open science reforms Common Types of Measures Scales of Measurement Reliability of Measures Test-retest reliability Internal reliability Interrater reliability Validity of Measures Face validity Content validity Criterion validity Convergent and discriminant validity Assignment 1 Assignment 1 is available on MyLS You need to complete an Academic Integrity Quiz to access Dropbox Need 100% (10 out of 10) Can complete repeatedly Instructions on materials to review are in the Newsfeed post Research Ethics; Measurement What do we mean by Ethics? Morals in human conduct Moral principles Rules of conduct Morals and Morality: -Distinctions between right and wrong -Accepted rules and standards of behaviour Research ethics Research ethics with humans Research ethics with animals Research misconduct HUMAN RESEARCH ETHICS Moral Foundations of Ethical Research: Core Principles 1. Respect for Persons 2. Concern for Welfare 3. Justice Core Principles: Respect for Persons Respect the autonomy of participants People make own decisions about participating Free from coercion or interference Applied primarily through informed consent Information that would influence decisions Purpose of study, risks, benefits, right to refuse Usually in an informed consent form Avoid coercion Less autonomous groups get special protection Children, cognitive limitations, prisoners Core Principles: Concern for Welfare Minimize risks and maximize benefits of research Applied by performing a “risk-benefit analysis” For participants For science and society Benefits must outweigh the risks Comparisons can be difficult Risks are primarily to participants Benefits primarily for science and society Risk-Benefit Analysis Benefits for Participants: Monetary payments, gifts, or the possibility of winning a prize Points toward a course grade Learning about psychology and the scientific process Receiving a helpful treatment or acquiring a new skill or way of thinking Satisfaction from contributing to a scientific investigation Benefits for Science and Society: Basic knowledge: enhanced understanding of human behavior Improvement of research or assessment techniques Practical outcomes that directly improve the welfare of people or other animals (e.g., therapies, teaching practices) Risks for Participants: Physical harm, threats to health Loss of time and productivity Psychological stress or discomfort Aversive states such as pain, anxiety, boredom, mental fatigue Feelings of inadequacy if unable to complete a task Loss of privacy and confidentiality Risks for Science and Society (of unethical or poor quality research): Monetary costs in terms of salaries, equipment, supplies Loss of time, effort, and productivity Diminished reputation of the field of psychology Societal misunderstanding of research results with harmful consequences Core Principles: Justice Treat people fairly and equitably Give participants adequate compensation Make sure benefits are distributed fairly At societal level Ensure particular groups are not overburdened Ensure particular groups are not excluded from opportunities without scientific justification Applied primarily through fair recruitment methods Offer participation to diverse social groups Exercise: Identify the core principle 1. Ensuring that certain groups in society (e.g., historically marginalized groups) do not face more than their fair share of the risks or burdens of research. 2. Considering the risk of physical or psychological harm to participants. 3. Respecting the autonomy of research participants by ensuring free and informed consent. 4. Ensuring that participants are not exposed to unnecessary risks. 5. An obligation to treat people fairly and equitably. 6. Considering participants' privacy and maintaining their confidentiality. 7. At a societal level, ensuring that certain groups are not unjustly excluded from research opportunities. 8. Protecting those incapable of exercising autonomy (e.g., due to youth, cognitive impairment, or mental health issues). 9. Weighing the potential risks to research participants against the potential benefits for science and society 10. Providing adequate compensation to participants and making sure that benefits and risks are distributed across all participants. Tri-Council Policy Statement (TCPS-2) The TCPS-2 is a joint policy of Canada’s three research agencies: SSHRC – Social Sciences and Humanities Research Council NSERC – Natural Sciences and Engineering Research Council CIHR – Canadian Institute of Health Research Tri-Council Policy Statement (TCPS-2) The TCPS-2 is policy that promotes the ethical treatment of humans in research. TheTCPS-2 provides guidelines that researchers in Canada must follow when conducting research with human participants. Labs will use the TCPS-2 certificate tutorial Tri-Council Policy Statement (TCPS-2): The Dynamic Nature of Ethics Policy “Norms for the ethics of research involving human subjects are developed and refined within an ever- evolving societal context, elements of which include the need for research and the research community, moral imperatives and ethical principles, and the law.” Ethics Review at WLU WLU human ethics application submission and approval process 1.Researcher completes ethics application. Purpose of the research Detailed description of participants and procedures Copy of all study materials 2.Application submitted to research ethics board (REB) for review. The panel can decide to: Approve Require changes Reject 3.Applicationapproved by REB chair. 4.Researcher conducts the approved study. 5.Researcher submits an annual or final report to REB. 1. Whether study is ongoing or complete 2. Number of participants in the study 3. Any unexpected or adverse events Ethics Review at WLU The Research Ethics Board (REB) An REB is an institutional panel of individuals (staff, faculty, community member) that ensure research abides by the TCPS-2 guidelines. An REB reviews and approves institutional research involving human participants. Mandate: To review the ethical acceptability of research on behalf of the institution, including approving, rejecting, proposing modifications to, or terminating any proposed or ongoing research involving humans. *in the U.S. this is an IRB – Institutional Review Board Ethics Review at WLU Research requiring REB approval Faculty research Graduate student research Undergraduate student research for thesis Undergraduate student research for courses in which people outside the course participate (e.g., some research experience courses) Research NOT requiring REB approval Research done with publicly accessible information Quality assurance studies, program evaluations, and employee performance reviews Research done with students in a course for educational purposes only (e.g., classroom demonstrations) ANIMAL RESEARCH ETHICS Animal Research Ethics: Animal Welfare The “animal welfare” perspective Use of animals in research has important benefits for science and society Concern is with the animal’s quality of life Three main types of welfare concerns Basic health and functioning - animals should be well fed and housed, free from injury and disease, and relatively free from the adverse consequences of stress. Affective states of animals - animals should be relatively free from negative states, including pain, fear, discomfort and distress, and capable of experiencing normal pleasures and comforts. Ability to perform important types of natural behaviour - animals should be able to carry out normal patterns of behaviour, including normal affiliation with other animals and those behaviours that they are highly motivated to undertake, in an environment that is well suited to the species. Animal Research Ethics: The Three Rs The Three Rs tenet guides scientists on the ethical use of animals in research: Replacement - methods which avoid or replace the use of animals in an area where animals would otherwise have been used Reduction - any strategy that will result in fewer animals being used Refinement - the modification of husbandry or experimental procedures to minimize pain and distress Animal Research Ethics: Governing Body Canadian Council of Animal Care (CCAC) The CCAC oversees the ethical use of animals in science in Canada Actsas a quasi-regulatory body and sets standards on animal use Funded primarily by Canadian Institutes of Health Research (CIHR) and Natural Sciences and Engineering Council (NSERC) Animal Research Ethics Animal Care Committee (ACC) An ACC is an institutional panel of individuals (researchers, veterinarian, community member, technical staff, student) that ensures the ethical treatment of animals in research. An ACC oversees all aspects of animal care and use, including animal facilities. The WLU animal care committee includes Faculty in biology, kinesiology, psychology Veterinarian Non-research observers Research Misconduct Behaviours that violate the integrity of the scientific enterprise Research Misconduct Blatant dishonesty or fraud 1. Falsification or fabrication of data e.g., Diederik Stapel 2.Plagiarism Data analysis practices 1. Cleaning and deleting data selectively 2. Overanalyzing data (p-hacking) 3. Selective reporting (cherry picking) 4. Post-hoc theorizing (HARKing: ) Data Fabrication: The Stapel Case Diederik Stapel was a prominent social psychologist at a Dutch University Data fabrication uncovered by students and lab assistants Admitted to faked data when confronted Extensive investigations; paper retractions See NY Times interview: http://www.nytimes.com/2013/04/28/magazine/diederik-stapels-audacious-academic- fraud.html?pagewanted=all Ethical Data Analysis Data analysis practices 1.Cleaning and deleting data selectively 2. Overanalyzing data (p-hacking) 3. Selective reporting (cherry picking) 4. Post-hoc theorizing (Hypothesizing After Results are Known - HARKing) Reforms to Improve Scientific Integrity The “Open Science” Movement see Open Science Framework https://osf.io/ Encourages specific practices to make the research process more public and transparent: 1.Full disclosure of information 2.Pre-registration of studies 3.Open data and materials Measurement Three Common Types of Measures Self-Report Involves having people tell you about themselves; the replies people give to questionnaires and interviews Cognitive self-reports Affective self-reports Behavioral self-reports Observational (or Behavioral) Involves the direct observation of behavior May measure anything an animal or person does that researchers can observe Physiological Involves measuring a bodily process Often used to assess processes within the nervous system Three Common Types of Measures: (example: Speaking Anxiety) Self-report Rating scale: How anxious were you while giving the speech (1=not at all, 7=extremely)? Open-ended: How did you feel while giving the speech? Observational (behavioral) Observers watch speaker and look for markers of anxiety such as sweating, trembling, speech disfluencies Need multiple observers Physiological Record physiological responses that reflect the internal state of anxiety EMG –Muscle tension GSR – Sweating Heart rate Scales of Measurement Categorical variables Quantitative variables – Ordinal scale – Interval scale – Ratio scale Properties of the real number system Identity: each number has a unique meaning Magnitude: numbers have an inherent order Equal intervals: difference between units is the same anywhere on the scale True zero: zero on the scale is a true zero; complete absence of the variable Note: These properties of the number system allow us to add, subtract, multiple, divide, etc. Scales of measurement and their numerical properties 1. Categorical (Nominal, Qualitative) Identity 2. Ordinal Identity, Magnitude 3. Interval Identity, Magnitude, Equal intervals 4. Ratio Identity, Magnitude, Equal intervals, True Zero Scales of measurement 1. Categorical (Nominal, Qualitative) Levels are categories 2. Ordinal Numbers represent rank order 3. Interval Numbers represent rank order Numbers represent equal distances 4. Ratio Numbers represent rank order Numbers represent equal distances Zero represents “none” – true zero Categorical (Nominal, Qualitative) Numbers (or just names) indicate different categories that differ from one another E.g., gender, culture, major Can’t be ordered (2 is not “more than” 1) Ordinal Numbers indicate rank ordering Measurements by ranking (e.g., taste preference) Ranked categories (low, medium, high) Higher numbers indicate more of the quality being measured “greater than,” “equal to,” “less than” But differences between consecutive values are not necessarily equal E.g., finishers in a race E.g., taste preferences: coke, pepsi, vinegar Interval Intervals between adjacent scale values are equal Difference between 1 and 2 is equal to the difference between 3 and 4, etc. E.g., thermometers, IQ, rating scales No absolute zero point Zero point is arbitrary Ratio Do have an absolute zero point E.g.,length, weight, time, numbers of responses, numbers of people Ratios using the scale scores are meaningful “A person who weighs 220 lbs is twice as heavy as one who weighs 110 lbs” “… responded twice as fast…” Are rating scales really interval? Strictly speaking, maybe not Cannot be sure intervals are equal distance But by convention they are treated as interval Seen as more like “interval” than “ordinal” Sometimes say “interval or near interval” We will follow this convention in the course -treat scale ratings and test scores as “interval” Exercise: Which Scale of Measurement? The birth weights of babies born at Grand River Hospital last week. Today's temperature (in Celsius) in the eight biggest cities across Canada. The number of hours you spent studying each day during the past week. The make of phone used by each student in the class. In a sportswriter opinion poll, the most promising Canadian hockey teams for next year were listed as Toronto first, Montreal second, and Vancouver third. Yellow walls in your office and white walls in your boss's office. A rank ordered list of the top 10 finishers in a talent contest. Your rating of your current happiness on a scale from 1 (not at all happy) to 10 (extremely happy) Ethnic groups of people in a neighborhood. The amount of time (in hours) it took to complete a task. Ranking of 10 movies from least to most favorite. Students' height in cm. Preferred political party. Why Scale of Measurement Matters Information conveyed Statistical analyses Reliability of a measure Reliability Consistency or dependability of a measure How consistently a measure assigns the same number to the same observation e.g., measuring height Frequency of Scores: True Height is 6 ft 100 90 Reliable 80 Unreliable 70 60 Frequency 50 40 30 20 10 0 5.6 5.7 5.8 5.9 5.1 5.11 6 6.1 6.2 6.3 6.4 6.5 6.6 Height Using Scatterplots and Correlation Coefficients to Evaluate Reliability We look for evidence of consistency between two measurements (two administrations of the same measure) Scatterplots Correlation coefficient (r) Using a Scatterplot to Evaluate Reliability Name Head Head Circumference in circumference in centimeters: 1 centimeters: 2 Taylor 50 52 Kendra 61 59 Mateo 65 64 Kurt 75 80 Using the Correlation Coefficient r to Evaluate Reliability Correlation coefficient (r) Direction Strength The Correlation Coefficient A statistic that indicates the degree of relation between two measurements Most common type is the Pearson correlation coefficient (r) Varies from -1.0 to +1.0 Indicates two things: 1. Direction of correlation 2. Strength of correlation Absolute numerical value For reliability want r ~.70 or greater Assessing the reliability of measures 1. Test-retest reliability 2. Interal reliability (inter-item) 3. Inter-rater reliability 1. Test-retest reliability Degree of consistency over time Measure the same people at two (or more) points in time Are scores at different times highly correlated? Useful for stable characteristics Abilities, personality traits, values Example Measure: Rosenberg Self-Esteem Scale Indicate your degree of agreement or disagreement with each item using the following scale: 1--------------------2---------------------3---------------------4 Strongly Disagree Agree Strongly Disagree Agree 1. On the whole, I am satisfied with myself. 2. At times I think I am no good at all. [R] 3. I feel that I have a number of good qualities. 4. I feel that I do not have much to be proud of. [R] 5. I take a positive attitude toward myself. 6. I certainly feel useless at times. [R] 7. I feel that I’m a person of worth, at least on an equal plane with others. 8. I wish I could have more respect for myself. [R] 9. All in all, I am inclined to feel that I am a failure. [R] 10. I am able to things as well as most other people. Test-retest Correlation Total Score Total Score Time 1 Time 2 Person 1 27 29 Person 2 21 20 Person 3 12 9 Person 4 19 20 Person 5 23 24 Test-retest Correlation Total Score Total Score Time 1 Time 2 Person 3 12 9 Person 4 19 20 Person 2 21 20 Person 5 23 24 Person 1 27 29 Limitations of test-retest reliability Familiarity of items Person may remember questions and answers – could inflate correlation Responses may be influenced by previous administration Only meaningful for stable constructs Conceptual variable may fluctuate E.g.: mood, well-being, hunger, fatigue 2. Internal reliability Degree of consistency among items on a scale Are all items on a multi-item scale measuring the same construct? Assessed by: Item-total correlations (not in text) Cronbach’s alpha coefficient (correlation-based statistic) Low reliability may indicate measurement error, or may indicate the construct has different components Example Measure: Rosenberg Self-Esteem Scale Indicate your degree of agreement or disagreement with each item using the following scale: 1--------------------2---------------------3---------------------4 Strongly Disagree Agree Strongly Disagree Agree 1. On the whole, I am satisfied with myself. 2. At times I think I am no good at all. [R] 3. I feel that I have a number of good qualities. 4. I feel that I do not have much to be proud of. [R] 5. I take a positive attitude toward myself. 6. I certainly feel useless at times. [R] 7. I feel that I’m a person of worth, at least on an equal plane with others. 8. I wish I could have more respect for myself. [R] 9. All in all, I am inclined to feel that I am a failure. [R] 10. I am able to things as well as most other people. Item-Total Correlation Item 1 Total Score Person 1 2 20 Person 2 4 36 Person 3 3 29 Person 4 3 27 Person 5 1 12 Item-Total Correlation Item 1 Total Score Person 5 1 12 Person 1 2 20 Person 3 3 29 Person 4 3 27 Person 2 4 36 Extraversion 1 2 3 4 5 Not at Very all true true 1.I am outgoing. ____ 2.I am friendly. ____ 3.I am talkative. ____ 4.I am gregarious. ____ 3. Inter-rater reliability Consistency among two (or more) researchers who observe and record scores Applicable to observational measures where two (or more) observers provide scores Want consistency to conclude that scores are reasonably independent of who did the rating Quantitative rating scales: Scatterplots and correlations between raters Coding categories: Percent agreement Kappa statistic– adjusts for expected agreement Scatterplots Can Show Interrater Agreement or Disagreement Percentage agreement RATER 1 RATER 2 AGREE? DYAD 1 Segment 1 C C YES Segment 2 C C YES Segment 3 A A YES Segment 4 A A YES DYAD 2 Segment 1 C A NO Segment 2 A A YES Segment 3 C C YES Segment 4 C C YES DYAD 3 Segment 1 A A YES Segment 2 A C NO Segment 3 A A YES Segment 4 C C YES Percentage agreement: Controlling chance agreement RATER 1 RATER 2 AGREE? DYAD 1 Segment 1 C C YES Segment 2 C C YES Segment 3 A A YES Segment 4 A A YES DYAD 2 Segment 1 C A NO Segment 2 A A YES Segment 3 C C YES Segment 4 C C YES DYAD 3 Segment 1 A A YES Segment 2 A C NO Segment 3 A A YES Segment 4 C C YES Percentage agreement: Base-rate problem RATER 1 RATER 2 AGREE? DYAD 1 Segment 1 C A NO Segment 2 C C YES Segment 3 C C YES Segment 4 C C YES DYAD 2 Segment 1 C C YES Segment 2 A C NO Segment 3 C C YES Segment 4 C C YES DYAD 3 Segment 1 C C YES Segment 2 C C YES Segment 3 C C YES Segment 4 C C YES Reliability as low measurement error Every score on a measure consists of two components Observed score = True score + Measurement Error True score: Score if measure were perfect Measurement error: Result of factors that distort the observed score, so that it differs from the true score Sources of measurement error Transient states of participants Tired, bad mood, anxious Stable attributes of participants Motivation level, social anxiety Situational factors in the research setting Researcher’s behavior, room temperature Characteristics of the measure itself Ambiguous questions, length of test Mistakes in recording Data entry errors, misperception Increasing Reliability Standardize administration Clarify instructions and questions Train observers Minimize coding errors The “more is better” rule Reliability is likely to increase as we increase the number of… Observers (or raters) Observations (or items) Occasions Random measurement error will average out Validity of a measure Refers to the extent to which a measure actually measures what it is supposed to measure Are we measuring what we think we are measuring? Relation between reliability and validity A measure can be reliable but not valid E.g., head size as a measure of intelligence A measure cannot be valid without being reliable Reliability is necessary (but not sufficient) for validity A measure can be valid only to the extent that it measures something reliably Reliability and validity Reliability: Is the measure consistent? Validity: Does the measure adequately reflect the construct of interest? Reliable and Valid Reliable, not Valid Not Reliable, not Valid Validity of Measurement: Does It Measure What It’s Supposed to Measure? Face validity and content validity: Does it look like a good measure? Criterion validity: Does it correlate with key behaviors? Convergent validity and discriminant validity: Does the pattern make sense? Measurement Validity of Abstract Constructs 1. Face validity & Content validity Both are subjective ways to assess validity Face validity: The measure looks like what you want to measure It appears “on the face of it” to measure what it is supposed to measure Content validity: The measure contains all the parts that your theory says it should contain Problem: Relies on subjective judgment Prefer to see empirical evidence 2. Criterion-related validity Is the measure related to a key behavioural criterion? Need to identify a specific behaviour or outcome that represents the variable Two ways to show criterion validity Correlational evidence Known groups evidence Criterion Validity: Does It Correlate with Key Behaviors? Correlational evidence for criterion validity Criterion Validity: Known-Groups Paradigm Another way to gather evidence for criterion validity is to use a known-groups paradigm. 3. Convergent validity & Discriminant validity Does the measure relate to other measures in ways it should? Convergent validity Does it correlate with other measures it should correlate with? Other measures of the same construct Theoretically-related constructs Can you see similarity? Discriminant validity Does it not correlate with other measures it should not correlate with? Can you see differences? Convergent Validity and Discriminant Validity: Does the Pattern Make Sense? Convergent Discriminant validity validity Convergent validity & Divergent validity example Single-item Self-esteem Scale (SISE) Convergent: Existing measures of SE Domain-specific self-evaluations Neuroticism Discriminant Academic performance Note difference between negative relation vs. no relation Discriminant = no relation Convergent = positive OR negative relation Relation between reliability and validity Correlation is used to assess reliability and validity Reliability Correlation using just your measure E.g., correlation of the measure with itself across time E.g., correlation among parts of your measure Validity Correlation of your measure with other different measures E.g., other measures of same variable E.g., other measures of different variables When do researchers evaluate reliability and validity? Sometimes you need to create a new measure for your study Some assessments can be done within the main study (e.g., internal reliability, inter-rater reliability) Some may need to be done before conducting the main study (in a pilot study for scale development and validation) Sometimes you can use an existing measure Tests of reliability and validity may have already been performed Sources of existing measures Journal articles Books and online databases (e.g., Mental measurements yearbook; Tests in print; Registry of scales and measures) Commercially published scales Exercise: Reliability and Validity The next slides present short scenarios in which researchers have measured variables. Each one most directly illustrates one of the following qualities of a measure: Good test-retest reliability Poor test-retest reliability Good internal reliability Poor internal reliability Good interrater reliability Poor interrater reliability Good face validity Poor face validity Good content validity Poor content validity Good criterion validity Poor criterion validity Good convergent validity Poor convergent validity Good discriminant validity Poor discriminant validity Exercise Scenarios A 1. Dr. Jones administers a job-skills test to 100 job applicants. The company then hires the 50 applicants who had the highest scores on the test. Among these 50 applicants, after they have worked at the company for six months, those who scored higher on the job-skills test are performing substantially better on the job. 2. When Dr. Jones asks a group of business professionals and scientists to examine the job-skills test, they generally agree that the items in the test appear to be highly relevant and appropriate questions to use for assessing people's job skills. 3. Data analyses reveal that several of the items included in the job skills test are not correlated with the total score on the test. Exercise Scenarios B 1. Dr. Suzuki has developed a self-report measure, containing 10 items, to measure people's general level of optimism. Research consistently shows that scores on Dr. Suzuki's optimism test are not highly correlated with other psychological tests of optimism commonly used by researchers 2. Dr. Suzuki's measure of optimism was given to a large sample of students at the beginning of the semester, and the researchers reported that Cronbach's alpha was.88. 3. The same sample of students completed Dr. Suzuki's measure at the end of the school term. Data analysis indicated that their optimism at the end of term was correlated r =.12 with their optimism at the start of the term. Exercise Scenarios C 1. A researcher creates a 5-item measure of conscientiousness (I get chores done right away, I follow a schedule, I not make a mess of things, I follow instructions carefully, I do my best at all tasks). Employee scores on the test are found to be correlated (r =.86) with how many times the employee has been late to work each month. 2. The item-total correlation is high for each item. 3. The researcher sends the measure to 10 experts in personality psychology who all confirm that the items all capture the construct of conscientiousness. 4. The experts also agree that the measure is not missing any important aspects of conscientiousness.