Inferential Statistics (Correlation, Regression & Statistical Tests) PDF
Document Details
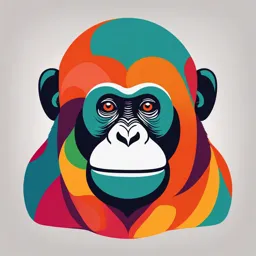
Uploaded by RestfulIntellect
Elizabeth City State University
Tags
Summary
These lecture notes cover inferential statistics, including correlation, regression and statistical tests. The document outlines the concepts of correlation and regression, along with various types of correlation and regression.
Full Transcript
Inferential Statistics (Correlation, Regression & Statistical Tests) Portion Ten Contents of the Session Introduction to Inferential Statistics Correlation Regression Statistical Tests Learning Outcomes By the end of the session, participant will be able t...
Inferential Statistics (Correlation, Regression & Statistical Tests) Portion Ten Contents of the Session Introduction to Inferential Statistics Correlation Regression Statistical Tests Learning Outcomes By the end of the session, participant will be able to: Identify bases and major assumptions in inferential statistics Be familiar with basic concept of correlation in research Recognize interpretation of coefficient of determination in correlation analysis Understand concept and essential types of regression Become conscious on application of correlation and regression in conducting research on urban issues Introduction Inferential Statistics provide a means for drawing conclusions about a population given the data actually obtained from the sample. They allow a researcher to make generalizations to a larger number of individuals, based on information from a limited number of subjects. Inferential statics are based on: Probability theory Statistical inference Sampling distributions Correlation Correlation is a statistical method that determines the degree of relationship between two different variables. Correlation does not imply causation because two variables are correlated, it does not mean that one variable caused the other But, If there was no relationship between the variables, or the correlation coefficient is close to or equal to zero, then no predictions can be made with any reliability. Correlation … Cont’d It is a statistical method-enables the researcher to find whether two variables are related and to what extent they are related. Considered as the sympathetic movement of two or more variables When a change in one particular variable is accompanied by changes in other variables as well, & this happens either in the same or opposite direction, then the resultant variables are said to be correlated. Correlation … Cont’d Correlation Coefficient (r) A single summary number that tells you whether a relationship exists between two variables, how strong that relationship is and whether the relationship is positive or negative It measures the nature and strength between two variables of the quantitative type. The sign of r denotes the nature of association While the value of r denotes the strength of association. Correlation … Cont’d If the sign is +ve this means the relation is direct (an increase in one variable is associated with an increase in the other variable and a decrease in one variable is associated with a decrease in the other variable). While if the sign is -ve this means an inverse or indirect relationship (which means an increase in one variable is associated with a decrease in the other). Correlation … Cont’d The value of r ranges between ( -1) and ( +1) The value of r denotes the strength of the association as illustrated by the following diagram. -1 -0.75 -0.25 0 0.25 0.75 1 Strong Strong Intermediate Weak Weak Intermediate Indirect/Negative Direct/Posetive Perfect Correlation No Relation Perfect Correlation Correlation … Cont’d If r = Zero this means no association or correlation between the two variables. If 0 < r < 0.25 = weak correlation. If 0.25 ≤ r < 0.75 = intermediate correlation. If 0.75 ≤ r < 1 = strong correlation. If r = l = perfect correlation. Three Major Measures of Correlation 1. A scatter diagram A scatter diagram is a diagram that shows the values of two variables X and Y, along with the way in which these two variables relate to each other. Positive relationship: is one where more of one variable is related to more of another, or less of one is related to less of another. Negative relationship: A negative relationship is one where more of one variable is related to less of another or the other way round. No relationship: A scatter diagram … Cont’d I. Positive Relationship Strong Positive Correlation Weak Positive Correlation A scatter diagram … Cont’d II. Negative relationship Strong Negative Correlation Weak Negative Correlation A scatter diagram … Cont’d III. No relation 2. Karl Pearson’s Coefficient of correlation The single most common type of correlation to measure the strength of a relationship between two continuous variables It has widely used application in Statistics. It is denoted by r. 2. Karl Pearson’s Coefficient of correlation … Cont’d Where X and Y are the variables and X = First score; Y = Second score N = Number of values or elements ∑XY = Sum of the product of the first and second scores ∑X = Sum of the first scores ∑Y = Sum of the second scores ∑X2 = Sum of square of first scores ∑Y2 = Sum of square of second scores 2. Karl Pearson’s Coefficient of correlation … Cont’d Example Question: If data set under x is area reserved for garden in the compound of the households (in m2) and the data set under y is distance of the houses from the main road in km, calculate the Pearson’s Coefficient of correlation. 2. Karl Pearson’s Coefficient of correlation … Cont’d Solution: N=6 Determine the values for XY, X2, Y2 ∑X , ∑Y , ∑XY , ∑X2 , ∑y2. ∑X=157 ∑Y=30.2 ∑XY=816.4 ∑X2=4365 ∑Y2=176.38 157 r= √(1541)(146.24) = 0.33 3. Spearman's-Rank correlation Coefficient Spearman's rank correlation coefficient allows us to identify easily the strength of correlation within a data set of two variables, and whether the correlation is positive or negative. The Spearman coefficient is denoted with the Greek letter rho (ρρ). 6 (di) 2 rs 1 n(n 1) 2 3. Spearman's-Rank Correlation Coefficient … Cont’d Example In study of the relationship sample level education Income b/n income of those who numbers (X) (Y) were registered for A Two bed rooms 25 condominium houses in B One bed room 10 Koye Feche area around AA and number of bed rooms of C Four Bed rooms 8 the houses. Find the D Three Bed rooms 10 relationship between them E Three Bed rooms 15 and comment. F Studio 50 G Four bed rooms 60 3. Spearman's-Rank correlation Coefficient … Cont’d Answer (X) (Y) Rank Rank di di2 X Y Two bed rooms 25 5 3 2 4 One bed room 10 6 5.5 0.5 0.25 Four Bed rooms 8 1.5 7 -5.5 30.25 Three Bed rooms 10 3.5 5.5 -2 4 Three Bed rooms 15 3.5 4 -0.5 0.25 Studio 50 7 2 5 25 Four bed rooms 60 1.5 1 0.5 0.25 ∑ di2=64 3. Spearman's-Rank correlation Coefficient … Cont’d 6 (di) 2 rs 1 n(n 1) 2 6 64 rs 1 0.1 7(48) This shows that there is an indirect weak correlation between number of rooms in the area and income. Multiple Correlation When one variable is related to a number of other variables, the correlation is multiple. Example: Relationship between effectiveness of urban housing provision with both access to land and access to housing loan together is multiple correlations Multiple Correlation Effectiveness of urban housing provision Access to land Access to housing loan % a urb ge of rel an ho effec a n at e t b d to using ivene of u r acc prov ss of ne ss t o ess i e tiv elate d t o l s i on e ffe c on r and g e of ovisi loan % a ing pr using o us ho h t o c e ss ac Partial Correlation Partial correlation is the relationship between two variables while controlling for a third variable. The purpose is to find the unique variance between two variables while eliminating the variance from a third variables. You typically only conduct partial correlation when the 3rd variable has shown a relationship to one or both of the primary variables. You can conduct partial correlation with more than just 1 third variable. You can include as many third-variables as you wish. Options of Partial Correlation Coefficient r We study the partial impacts of the 2 nd variable on the 1 st 12.3 variable keeping the 3rd independent variable constant r We study the partial impacts of the 3 rd variable on the 2 nd 23.1 variable keeping the 1st independent variable constant r We study the partial impacts of the 3 rd variable on the 1 st 13.2 variable keeping the 2nd independent variable constant r12.3 = r12-r13xr23 2 2 √1-r √1-r The Coefficient of Determination The squared value of r2 is called the coefficient of determination and it has two important interpretations. a)It explains the proportion of variance in one variable accounted for by the other variable. For example, if r =.25, then r2 = (.25)(.25) =.0625. Therefore, the variable explains approximately 6% of the variation in the other variable which means 94% of the total variance between the variables remains unexplained. The former variance is called shared variance and the latter variance is called uncommon, unshared, or unexplained variance. The Coefficient of Determination … Cont’d b). The second interpretation of r2 is its use as a measure of strength between two or more r values. For example, if given r =.25 and r =.50, it is clear that the second r-value is twice as great as the first r value. However, the r2 values are 6.25% and 25% respectively. Thus, r =.50 actually explains four times as much variance as does an r =.25. Regress ion Regression is the process of determining the association and forecasting the relationship between two or more variables. Regression can be: 1. Simple regression or simple linear regression or 2. Multiple regression analysis Assumptions of Linear Regression Linear regression analysis makes several key assumptions: 1.There must be a linear relationship between the outcome variable and the independent variables. 2.Multivariate Normality– the residuals are normally distributed. 3.No Multicollinearity—the independent variables should not be highly correlated with each other. This assumption is tested using Variance Inflation Factor (VIF) values. 4.Homoscedasticity– means ‘equal variances. The size of the error term is the same for all values of the independent variable. If the error term, or the variance, is smaller for a particular range of values of independent variable and larger for another range of values, then there is a violation of homoscedascity. Simple Regression or Simple Linear Regression In simple linear regression, a regression equation is used to plot a straight line through the middle of the scatter plot. The formula is: Y = a + bX +ε Where, Y is the dependent variable or the value to be predicted a is the Y intercept or the point at which the straight line crosses the ordinate or Y axis when X is 0 and b defines the slope or the angle of the straight line Simple Regression … Cont’d Simple regression -statistical measure that attempts to determine the strength of the relationship between one dependent variable and one independent variable. A statistical technique used to explain the behavior of a dependent variable. Linear regression used to find the straight line is called the least squares regression line. Simple Regression … Cont’d Ordinary Least Square (OLS) Method: One of the aim of the regression is to find the line that fits the points best of all OLS is a technique for fitting the ‘best’ straight line to the sample XY observation It involves minimizing the sum of the squared (vertical) deviation of point from the line: Simple Regression … Cont’d Where, x and y are the variables. b = the slope of the regression line is also called as regression coefficient a = intercept point of the regression line which is in the y-axis. N = Number of values or elements X = First Score Y = Second Score ∑XY = Sum of the product of the first and Second Scores ∑X= Sum of First Scores ∑Y = Sum of Second Scores ∑X2 = Sum of square First Scores. Example Question Data set under Y is the satisfaction of the respective respondents by the housing dvt and provision by the related sector (in %) and data set under X is the age of the respondents (in years). So, determine the regression equation by computing the regression slope coefficient and intercept value. Solution First determine the values for XY, X2 Simple Regression … Cont’d Determine the following values ∑X , ∑Y , ∑XY , ∑X 2. ∑X=330 ∑Y=282 ∑XY=18760 ∑X 2=22150 Slope (b) = N∑XY – (∑X)(∑Y) N∑X2 – (∑X)2 Slope (b) = (5X18760) – (330)(282) = 0.4 (5X22150) – (330)2 Intercept (a) = ∑Y – b(∑X) N a = 282– 0.4 (330) = 30 5 Therefore, the regression equation Y = a +bx Y = 30 + 0.4x Multiple Regression Multiple Regression is a statistical tool that allows you to examine how multiple independent variables are related to a dependent variable. Once you have identified how these multiple variables relate to your dependent variable, you can take information about all of the independent variables and use it to make much more powerful and accurate predictions about why things are the way they are. Multiple Regression … Cont’d The equation of the probabilistic multiple regression is Y’ = a + b1X1 + b2X2 … + bnXn + ε where, Y’ is the predicted value of Y (the dependent variable) a is the regression constant (the Y intercept) b1, b2,........., bn is the partial regression coefficients for the independent variables, 1, 2,...., i respectively. ‘n' is the number of independent variables. ε is error Multiple Regression … Cont’d How to Calculate b1, b2, … bn ? b1 = ry,x1 – ry,x2rx1,x2 SDY SDX1 1- (rx1,x2)2 b2 = ry,x2 –ry,x1rx1,x2 SDY SDX2 Where, 1- (rx1,x2)2 ry,x1 = Correlation coefficient b/n y and x1 ry,x2 = Correlation coefficient b/n y and x2 rx1,x2 = Correlation coefficient b/n x1 and x2 (rx1,x2)2 = The coefficient of determination (r squared) for x1 and x2 SDY = Standard Deviation for your Y (dependent variable) SDX1 = Standard Deviation for X1 Multiple Regression … Cont’d How to Calculate “a”? a = Y –b1X1 – b2X2 Y = Mean of Y (dependent variable) b1X1 = The value of b1 multiplied by mean of X1 b2X2 = The value of b2 multiplied by mean of X2 Parametric and Non-parametric statistics (tests) Parametric Test Is a branch of inferential statistics which assumes that: The observations must be drawn from normally distributed populations (the normality assumption) The normal distributions have the same variances or SD (the assumption of homogeneity of variance) The data is taken from an interval or ratio scale (since it is impossible to have normal distributions on any other kind of scale) Parametric Test … Cont’d If those assumptions are correct, parametric methods can produce more accurate and precise estimates (they are said to have more statistical power). However, if those assumptions are incorrect, parametric methods can be very misleading. Example Income in the population and The incidence rates of rare diseases are not normally distributed With a sample of small size at hand, analyzing such variables Parametric Test … Cont’d Still, parametric tests can also be applied even if we are not sure that the distribution of population is normal as long as our sample is large enough. If our sample is very small, however, then those tests can be used only if we are sure that the variable is normally distributed. Nonparametric Tests Do not make assumptions about the population distribution Treat samples made up of observations from several different populations. Data that are ordinal, ranked and data subject to outliers are analyzed by nonparametric tests They are available to treat data which are classificatory Easier to learn and apply than parametric tests Low precision when compared to parametric test Low power Testing distributions only Higher-ordered interactions not dealt with nonparametric tests Power of a Test Statistical power is the probability of rejecting the null hypothesis when it is in fact false and should be rejected Power of parametric tests – calculated from formula, tables, and graphs based on their underlying distribution Power of nonparametric tests – less straightforward; calculated using simulation methods Selecting a Statistical Test There is at least one nonparametric test equivalent to a parametric test Type of Data Goal Measurement Rank, Score, or Binomial/dichotomous (From normal measurement (From non- in nature distribution) normal distribution) (2 possible outcomes) Describe one group Mean, SD Median, Inter-quartile range Proportion Compare one group One-sample t Wilcoxon test Chi-square or Binomial to a hypothetical test test value Compare two Unpaired t test Mann-Whitney Fisher’s test (Chi-square unpaired groups for large samples) Compare two Paired t test Wilcoxon test McNemar’s test paired groups Compare three or One-way Kruskal-Walls test Chi-square test more unmatched ANOVA groups Selecting a Statistical Test … Cont’d Type of Data Measurement Rank, Score, or Binomial/ Goal (From normal measurement (From non- dichotomous in distribution) normal distribution) nature (2 possible outcomes) Compare three or Repeated Friedman test Cochrane Q more matched measures groups ANOVA Quantify association Pearson Spearman Correlation Contingency b/n two variables correlation coefficients Predict value from Simple linear Non parametric regression Simple logistic another measured Regression or regression variable Non-linear regression Predict value from Multiple linear Multiple logistic several measured or Regression or regression binomial variables multiple non- linear regression t- test Assumption: The samples come from two normally distributed populations. and are assumed equal but not known Under these assumptions, we can use the t-distribution ( n1 n 2 2) ( t / 2 (n1 n 2with 2)degrees ) for a given level oftosignificance of freedom (). The find the critical test values statistic is given by: X1 X 2 t cal 1 1 Sp n1 n2 t- test... Cont’d Where Sp is the pooled standard deviation defined as: (n1 1)S12 (n 2 1)S22 Sp n1 n 2 2 Here S12 and S22 are the variances computed from the samples. Decision rule: Reject H0 if | t cal | t / 2 (n1 n 2 2) t- test... Cont’d Example 1: The following summary statistics are on the annual household income (in thousands of birr) of individuals who are not educated (group 1) and educated (group 2) on their bank loans. Not educated (group 1) Educated (group 2) Mean 41.2131 47.1547 Variance 1858.949 1171.019 Sample size 183 517 Test if there is a significant difference in the mean income of educated and not educated at the 5% level of significance. t- test... Cont’d. The hypothesis of interest is: H 0 : 1 2 H A : 1 2 (n1 1)S12 (n 2 1)S22 (183 1)(1858.949) (517 1)(1171.019) = 36.7477 Sp n1 n 2 2 183 517 2 X1 X 2 41.2131 47.1547 t cal = - 1.686 1 1 1 1 Sp 36.7477 n1 n2 183 517 = 0.05 t / 2 (n1 n 2 2) t 0.025 (183 517 2) t 0.025 (698) 1.960 Decision: Since the absolute value of the test statistic tcal = 1.686 does not exceed the critical value, we do not reject H. t- test... Cont’d -1.960 1.960 Conclusion: There is no significant difference in the mean income of Educated and not educated at the 5% level of significance. Statistical Test … Cont’d Difference between Parametric and Non Parametric Parametric Non Parametric Information about population is No information about the completely known population is available Specific assumptions are made No assumptions are made regarding the population regarding the population Null hypothesis is made on The null hypothesis is free from parameters of the population parameters distribution Statistical Test … Cont’d Difference between … Cont’d Test statistic is based on the Test statistic is arbritary distribution Parametric tests are applicable It is applied both variable and only for variable artributes No parametric test exist for Non parametric test do exist for Norminal scale data norminal and ordinal scale data Parametric test is powerful, if it It is not so powerful like exist parametric test Thank You 55