OR Chapter One - Introduction to Operations Research PDF
Document Details
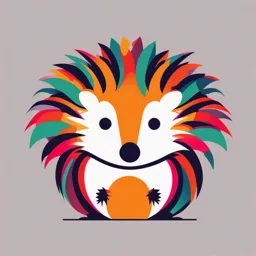
Uploaded by UnabashedBluebell1015
Werabe University
Tags
Summary
This document introduces the concept of Operations Research (OR) as a quantitative approach to decision-making in today's complex business environment. It explains how OR techniques can be used to evaluate alternative courses of action and potential outcomes.
Full Transcript
CHAPTER ONE: INTRODUCTION TO OPERATIONS RESEARCH 1.1. OR – Quantitative approach to decision making Decision-making in today’s social and business environment has become a complex task. The uncertainty of the future and the nature of competition and social interaction greatly i...
CHAPTER ONE: INTRODUCTION TO OPERATIONS RESEARCH 1.1. OR – Quantitative approach to decision making Decision-making in today’s social and business environment has become a complex task. The uncertainty of the future and the nature of competition and social interaction greatly increase the difficulty of managerial decision-making. Knowledge and technology are changing rapidly, the new problems with little or no precedents these problems and provide leadership in the advancing global age, decision-makers cannot afford to make decisions by simply applying their personal experiences, guesswork or intuition, because the consequences of the wrong markets, producing the wrong products, providing inappropriate services, etc., will have major, often disastrous consequences for organizations. Operations Research as one of the quantitative aid to decision-making, offers the decision maker a method of evaluating every possible alternative (act or course of action) by using various techniques to know the potential outcomes. This is not to say, however, that management decision-making is simply about the application of operations research techniques. In general, while solving a real-life problem, the decision-maker must examine in both from quantitative as well as qualitative perspective. Information about the problem from both these perspectives needs to be brought together and assessed in the context of the problem. Based on some mixes of the two sources of information, a decision should be taken by the decision-maker. The evaluation of each alternative is extremely difficult or time consuming for two reasons: First, the amount and complexity of information that must be processed; second the number of alternative solutions could be so large that a decision maker simply can not evaluate all of them to select an appropriate one. For these reasons when there is lack of qualitative information, decision makers increasingly turn to quantitative methods and use computers to arrive at their optimal solution to problems involving large number of alternatives. The study of these methods and how decision makers use them in the decision process is the essence of operations research approach. 1|Page 1.2. History of Operations Research It is generally agreed that operations Research came is to existence as a discipline during World War II when there was a critical need to manage scarce resources. The term “Operations research” was coined as a result of research on military operations during this war. Since the war involved strategic and tactical problems which were greatly complicated, to expect adequate solutions from individual or specialists in a single discipline was unrealistic. Therefore, group of individuals who collectively were considered specialists in mathematics, Economics, statistics and probability theory, engineering, behavioral, and physical science were formed as special unit within the armed forces to deal with strategic and tactical problems of various military operations. The objective was the most effective utilization of most limited military resources by the use of quantitative techniques. After the war ended, scientists who had been active in the military OR groups made efforts to apply the operations research approach to civilian problems, related to business, industry, research and development, and even won Nobel prizes when they returned to their peacetime disciplines. There are three important factors behind the rapid development in the use of operations research approach. (i) The economic and industrial boom after World War II resulted in continuous mechanization, automation, decentralization of operations and division of management factors. This industrialization also resulted in complex managerial problems, and therefore application of operations research to managerial decision-making become popular. (ii) Many operation researchers continued their research after war. Consequently, some important advancement were made in various operations research techniques: linear programming and its solution by a method known as simplex method, statistical quality control, dynamic programming, queuing theory and inventory theory were well developed during this time. 2|Page (iii) Analytic power was made available by high-speed computers. The use of computers made it possible to apply many OR techniques for practical decision analysis. 1.3. Nature and Significance Operations Research The Operations research approach is particularly useful in balancing conflicting objectives (goals or interests), where there are many alternative courses of action available to the decision-makers. In a theoretical sense, the optimum decision must be one that is best for the organization as a whole. It is often called global optimum. A decision that is best for one or more sections of the organization is usually called suboptimum decision. The OR approach attempts to find global optimum by analyzing inter-relationships among the system components involved in the problem. In other words, operations research attempts to resolve the conflicts of interest among various sections of the organization and seeks the optimal solution which may not be acceptable to one department but is in the interest of the organization as a whole. 1.4. Operation Research: Some definitions The British/Europeans refer to "operational research", the Americans to "Operations research" - but both are often shortened to just "OR" (which is the term we will use). Another term which is used for this field is "management science" ("MS"). The Americans sometimes combine the terms OR and MS together and say "OR/MS" or "ORMS". Yet other terms sometimes used are "industrial engineering" ("IE"), "Decision Science" ("DS”) and “problem solving”. In recent years there has been a move towards a standardization upon a single term for the field, namely the term "OR". Because of the wide scope of application of operations research, giving a precise definition is difficult. However, a few definitions of OR are given below. Operations research is the application of the methods of science to complex problems in the direction and management of large systems of men, machines, materials and money in industry, business, government and defense. The distinctive approach is to develop a scientific model of the system incorporating measurements of factors such as chance and risk with which to predict and compare the out comes 3|Page of alternative decisions, strategies, or controls. The purpose is to help management in analyze its policy and actions scientifically. Operations Research Society, UK Operations research is concerned with scientifically defining how to best design and operate man-machine systems usually requiring the allocation of scarce resources. Operations Research Society, America Operations research is a systematic application of quantitative methods, techniques and tools to the analysis of problems involving the operation of systems. Operations research is essentially a collection of mathematical techniques and tools which in conjunction with systems approach, is applied to solve practical decision problems of an economic or engineering nature. Operations Research, in the most general sense, can be characterized as the application of scientific methods, techniques and tools, to problems involving operations of a system so as to provide those in control of the operations with optimum solutions to the problems. Operation research seeks the determination of the optimum course of action of a decision problem under the restriction of limited resources. It is quite often associated almost exclusively with the use of mathematical techniques to model and analyze decision problems. 1.1. Features of Operations Research Approach From the previous discussions and various definitions of OR, important features or characteristics can be drawn. These features of OR approach to any decision and control problems can be summarized as: 1.4.1. Inter-disciplinary approach Interdisciplinary teamwork is essential because while attempting to solve a complex management problem, one person may not have complete knowledge of all its aspects such as economic, social, political, psychological, engineering, etc. This means we should not expect a desirable solution to managerial problems from a single individual or discipline. Therefore, a team of individuals specializing in mathematics, statistics, computer science, 4|Page psychology, etc, can be organized so that each aspect of the problem could be analyzed by a particular specialists in that field. But we shouldn’t forget that certain problem situations may be analyzed even by one individual. 1.4.2. Methodological Approach Operation research is the application of scientific methods, techniques and tools to problems involving the operations of systems so as to provide those in control of operations with optimum solutions to the problems. Note: A system is defined as an arrangement of components designed to achieve a particularobjective(s) according to plan. The components may be either physical or conceptual or both but they share a unique relationship with each other and with the overall objective of the system. 1.4.3. Holistic Approach or Systems Orientation While arriving at a decision, an operation research team examines the relative importance of all conflicting and multiple objectives and the validity of claims of various departments of the organization from the perspective of the whole organization. 1.4.4. Objectivistic Approach The OR approach seeks to obtain an optimal solution to the problem under analysis. For this, a measure of desirability (or effectiveness) is defined, based on objective(s) of the organization. A measure of desirability so defined is then used to compare alternative courses of action with respect to their outcomes. 1.4.5. Decision Making – OR increases the effectiveness of management decisions. It is the decision science which helps management to make better decisions. So the major premise of OR is decision making, irrespective of the situation involved. 1.4.6. Use of Computers: OR often requires a computer to solve the complex mathematical model or to perform a large number of computations that are involved. 1.4.7. Human factors: In deriving quantitative solution, we do not consider human factors which doubtlessly play a great role in the problems. So study of the OR is incomplete without a study of human factors. 1.5. Models and Modeling in Operations Research 5|Page Both simple and complex systems can easily be studied by concentrating on some portion or key features instead of concentrating on every detail of it. This approximation or abstraction, maintaining only the essential elements of the system, which may be constructed in various forms by establishing relationships among specified variables and parameters of the system, is called a model. In general, models attempt to describe the essence of a situation or activity by abstracting from reality so that the decision- maker can study the relationship among relevant variables in isolation. Models do not, and cannot, represent every aspect of reality because of the innumerable and changing characteristics of the real life problems to be represented. Instead, they are limited approximation of reality. For example, to study the flow of materials through a factory, a scaled diagram on paper showing the factory floor, position of equipment, tools, and workers can be constructed. It would not be necessary to give such details as the color of machines, the height of the workers, or the temperature of the building. For a model to be effective, it must be representative of those aspects of reality that are being investigated and have a major impact on the decision situation. A model is constructed to analyze and understand the given system for the purpose of improving its performance. The reliability of the solution obtained from a model depends on the validity of the model in representing the system under study. A model, allows the opportunity to examine the behavioral changes of a system without disturbing the on-going operations. Note: The key to model building lies in abstracting only the relevant variables that affect the criteria of the measures of performance of the given system and expressing the relationship in a suitable form. But oversimplification of problem can lead to a poor decision. Model enrichment is accomplished through the process of changing constants in to variables, adding variables, relaxing linear and other assumptions, and including randomness. 1.5.1. Classification of OR Model As we discussed earlier, OR model is an abstract representation of an existing problem situation. It can be in the form of a graph or chart, but most frequently an OR model consist of a set of mathematical relationships. These mathematical relationships are made up of numbers and symbols. 6|Page There are many ways to classify models: i) Classification based on structure a) Physical Models These models provide a physical appearance of the real object under study either reduced in size or scaled up. Physical models are useful only in design problems because they are easy to observe, build, and describe. Since these models cannot manipulated and are not very useful for prediction, problems such as portfolio analysis selection, media selection, production scheduling, etc cannot be analyzed by physical models. b) Symbolic models These models use symbols (letters, numbers) and functions to represent variables and their relationships to describe the properties of the system. ii) Classification based on function or purpose Models based on the purpose of their utility include: a) Descriptive models Descriptive models simply describe some aspects of a situation, based on observation, survey, questionnaire results or other available data of a situation and do not recommend anything. Example: Organizational chart, plant layout diagram, etc. b) Predictive Models These models indicate “If this occurs, then that follow”. They relate dependent and independent variables and permit trying out, “what if” questions. In other words, these models are used to predict outcomes due to a given set of alternatives for the problem. These models do not have an objective function as a part of the model to evaluate decision alternatives. For example, S = a + bA +cI is a model that describes how the sales (S) of a product changes in advertising expenditures (A) and disposal personal income (I). Here, a, b, and c are parameters whose values must be estimated. 7|Page c) Normative (Optimization) models These models provide the “best” or “Optimal” solution to problems subject to certain limitations on the use of resources. These models provide recommended courses of action. For example, in mathematical programming, models are formulated for optimizing the given objective function, subject to restrictions on resources in the context of the problem under consideration and non negativity of variables. These models are also called prescriptive models, because they prescribe what the decision maker ought to do. iii) Classification Based on Time Reference a) Static Models Static models represent a system at some specified time and do not account for changes over time. For example, an inventory model can be developed and solved to determine an economic order quantity for the next period assuming that the demand in planning period would remain the same as that for today. b) Dynamic models In dynamic models, time is considered as one of the variables and allows the impact of changes due to change in time. Thus, sequences of interrelated decisions over a period of time are made to select the optimal course of action to optimize the given objective. Dynamic programming is an example of a dynamic model. iv) Classification based on Degree of certainty a) Deterministic Models If all the parameters, constants and functional relationships are assumed to be known with certainty when the decision is made, the model is said to be deterministic. Thus, in such a case, the outcome associated with a particular course of action is known. That is, for a specific set of input values, there is a uniquely, determined output which represents the solution of the model under consideration of certainty. The results of the models assume single value. Linear programming models are examples of deterministic models. b) Probabilistic (Stochastic) models Models in which at least one parameter or decision variable is a random variable are called 8|Page probabilistic (or stochastic). Since at least one decision variable is random, a dependant variable which is the function of independent variable(s) will also be random. This means consequences or pay off due to certain changes in the independent variable cannot be produced with certainty. However, it is possible to predict a pattern of values of both the variables by their probability distribution. Insurance against risk of fire, accidents, sickness, etc are examples where the pattern of events is studied in the form of a probability distribution. v) Classification Based on Method of solution or Quantification a) Heuristic Model These models employ some sets of rules which, though perhaps not optimal, do facilitate solutions of problems when applied in a consistent manner. b) Analytical Models These models have a specific mathematical structure and thus can be solved by known analytical or mathematical techniques. Any optimization model (which requires maximization or minimization of an objective function) is an analytical model. c) Simulation Models These models have a mathematical structure but are not solved by applying mathematical techniques to get a solution. Instead, a simulation model is essentially a computer-assisted experimentation on a mathematical structure of a real-life problem in order to describe and evaluate its behavior under certain assumptions over a period of time. Simulation models are more flexible than mathematical ones and therefore, can be used to represent a complex system which otherwise cannot be represented mathematically. These models do not provide general solution like those of mathematical Models. 1.5.2. Advantage of Models Models in general are used as an aid for analyzing complex problems. However, a model can also serve other purposes as: i) A model provides economy in representation of the realities of the system. That is, 9|Page models help decision makers to visualize a system so that he/she can understand the system’s structure or operation in a better any. For example, it easier to represent a factory lay out on paper than to construct it. It is cheaper to try out modifications of such systems by rearrangement on paper. ii) The problem can be viewed in its entirety, with all the components being considered simultaneously. iii) Models serve as aids to transmit ideas and visualization among people in the organization. For example, process chart can help the management to communicate about better work methods to workers. iv) A model allows us to analyze and experiment in a complex situation to a degree that would be impossible in the actual system and its environment. For example, the experimental firing of satellite may be costly and require years of preparation. v) Models simplify he investigation considerably and provide a powerful and flexible for predicting the future state of the process or system. 10 | P a g e