The Growing Influence of Industry in AI Research PDF
Document Details
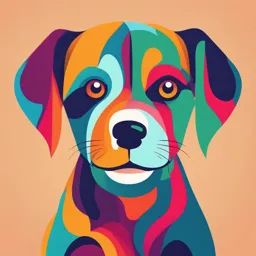
Uploaded by WorldFamousLogic8685
Politecnico di Milano
Nur Ahmed, Muntasir Wahed, Neil C. Thompson
Tags
Related
- LdpvfFcBXkT39AFuF-Role of Artificial Intelligence AI in Media Industry.pdf
- GEIT112 Artificial Intelligence Chapter 4 PDF
- 2024 Fraunhofer IAO PDF: Künstliche Intelligenz aus Sicht von Unternehmen
- Artificial Intelligence and Machine Learning Applications in Smart Production (PDF)
- Quantum Computing: A Beginner's Guide PDF
- We Need to Think Differently About Artificial Intelligence PDF
Summary
This policy forum article examines how industry is increasingly dominating AI research by controlling key inputs like computing power, large datasets, and skilled researchers. The article also discusses the impact of this dominance, including its influence on academic publications and benchmarks. Concerns about public interest alternatives for AI tools are raised.
Full Transcript
INSIGHTS 2013 (see SM), but supply has only been able P OLICY FORUM to meet 20% of this demand in recen...
INSIGHTS 2013 (see SM), but supply has only been able P OLICY FORUM to meet 20% of this demand in recent years. Industry’s ability to hire talent and harness ARTIFICIAL INTELLIGENCE greater computing power likely arises be- cause of differences in spending. Although in- The growing influence vestments in AI have gone up substantially in both the public and private sectors, industry’s investments are larger and growing faster (see of industry in AI research SM). We compare industry with the major source of public-interest AI research: govern- ments, which both fund their own research Industry is gaining control over the technology’s future and are a key source of academic funding. In 2021, nondefense US government agencies allocated US$1.5 billion on AI. In that same By Nur Ahmed1,2, Muntasir Wahed3, of talent, we see that industry is winning this year, the European Commission planned to Neil C. Thompson1,2 contest. Data on North American universi- spend €1 billion (US$1.2 billion). By contrast, ties (where we are able to get the best data) globally, industry spent more than US$340 F or decades, artificial intelligence (AI) show that computer science PhD graduates billion on AI in 2021, vastly outpacing public Downloaded from https://www.science.org at Politecnico Di Milano on January 22, 2025 research has coexisted in academia specializing in AI are going to industry in un- investment. As one example, in 2019 Google’s and industry, but the balance is tilt- precedented numbers (see the first figure). In parent company Alphabet spent US$1.5 bil- ing toward industry as deep learning, 2004, only 21% of AI PhDs went to industry, lion on its subsidiary DeepMind, which is a data-and-compute-driven subfield of but by 2020, almost 70% were. For compari- just one piece of its AI investment. In Europe, AI, has become the leading technology son, this share of PhDs entering industry is the disparity is smaller but is still present; AI in the field. Industry’s AI successes are easy already higher than in many areas of science Watch estimates that “the private and public to see on the news, but those headlines are and will likely soon pass the average across sector account for 67% and 33% of the EU the heralds of a much larger, more system- all areas of engineering (see SM). Computer AI investments respectively” (4) (see SM). For atic shift as industry increasingly dominates science research faculty who specialize in comparison, in recent decades, research the three key ingredients of modern AI re- AI have also been hired away from univer- funding in the pharmaceutical industry has search: computing power, large datasets, and sities to work in industry. This hiring has been split roughly evenly between the pri- highly skilled researchers. This domination risen eightfold since 2006, far faster than vate sector and governments or nonprofits of inputs is translating into AI research out- the overall increase in computer science re- (see SM). An example of the scale of funding comes: Industry is becoming more influential search faculty (see the first figure). Between needed to pursue AI research comes from in academic publications, cutting-edge mod- the PhD students and faculty leaving for in- OpenAI, which began as a not-for-profit with els, and key benchmarks. And although these dustry, academic institutions are struggling the claim to be “unconstrained by a need industry investments will benefit consum- to keep talent (2). This concern is not lim- to generate financial return” and aiming ers, the accompanying research dominance ited to US universities. In the UK, Abhinay to “benefit humanity as a whole” (5). Four should be a worry for policy-makers around Muthoo, Dean of Warwick University’s King’s years later, OpenAI changed its status to the world because it means that public inter- Cross campus, said, “The top tech firms are a “capped for-profit organization” and an- est alternatives for important AI tools may sucking the juice from the universities” (3). nounced that the change would allow them become increasingly scarce. The computing power being used by aca- “to rapidly increase our investments in demia and industry also shows a growing compute and talent” (6). INDUSTRY’S INPUT DOMINANCE divide. In image classification, the comput- Industry has long had better access to large, ing power being used by industry is larger THE INCREASING DOMINANCE OF economically valuable datasets (1) because and has grown more rapidly than that used INDUSTRY IN AI RESEARCH their operations naturally produce data as by academia or by industry-academia col- Industry’s dominance of AI inputs is now they interact with large numbers of users and laborations (see the first figure). Here, we manifesting in an increasing prominence in devices. For example, in 2020, WhatsApp us- proxy for the computing power used in a AI outcomes as well—in particular, in pub- ers sent roughly 100 billion messages per day. model with the number of parameters—both lishing, in creating the largest models, and Thus, it is unsurprising that most large data because the number of parameters is one in beating key benchmarks. Research papers centers are owned and operated by industry of the key determinants of the computing with one or more industry co-authors grew [see supplementary materials (SM)]. In this power needed and because the deep learn- from 22% of the presentations at leading AI article, we show that industry’s dominance ing scaling law literature has shown strong conferences in 2000 to 38% in 2020 (see the extends beyond data to the other key inputs relationships between them. In 2021, indus- second figure). Alternate definitions of what of modern AI: talent and computing power. try models were 29 times bigger, on average, constitutes an industry paper yield substan- Demand for AI talent has grown much than academic models, highlighting the vast tially similar results (see SM). Industry’s more quickly than supply over the past decade difference in computing power available to dominance is even more apparent in the (see SM), generating increased competition the two groups. This is not just a difference largest AI models (7) and in benchmark per- for AI talent. Across two different measures in approach but a shortfall in computing formance. Industry’s share of the biggest AI available to academics. For example, data models has gone from 11% in 2010 to 96% in 1 Sloan School of Management, Massachusetts Institute from Canada’s National Advanced Research 2021 [see the second figure; data are from of Technology (MIT), Cambridge, MA, USA. 2Computer Computing Platform reveals that academic (8)]. We use model size as a proxy for the ca- Science and Artificial Intelligence Laboratory, MIT, demand for graphics processing units pabilities of large AI models, as is common in Cambridge, MA, USA. 3Department of Computer Science, Virginia Tech, VA, USA. Email: [email protected]; (GPUs; the most common chips used in AI) the literature. Model size is also often used as [email protected] on their platform has increased 25-fold since a proxy for computing power (see the first fig- 884 3 MONTH 2023 VOL 379 ISSUE 6635 science.org SCIENCE ure). This dual usage reflects how important machine translation benefits international about job replacement and AI-induced in- compute is for predicting the performance of trade (10)] and can streamline processes equality. Some researchers are concerned deep learning systems (9). that drive down a firm’s costs. Industry’s in- that we may be on a socially suboptimal We investigate when academia, indus- vestment in AI also produces tools that are trajectory (13) that focuses more on substi- try, or academia-industry collaborations led valuable to the whole community (such as tuting human labor rather than augmenting performance on AI benchmarks (see the PyTorch and TensorFlow, which are widely human capabilities. second figure). When looking across these used in academia), hardware that facilitates Even with a growing divide between in- six benchmarks in image recognition, senti- efficient training of deep-learning models dustry and academia, one might imagine that ment analysis, language modeling, semantic [such as tensor processing units (TPUs)], and the field could settle into a division of labor segmentation, object detection, and machine publicly accessible pretrained models (such similar to that of other disciplines, in which translation—as well as 14 more that cover as the Open Pretrained Transformer model basic research is primarily done in universi- areas such as robotics and common sense by Meta). ties, and applied research and development is reasoning (see SM)—industry alone or in At the same time, the concentration of primarily done by industry. But in AI, such a collaboration with universities had the lead- AI in industry is also worrisome. Industry’s clear divide does not exist; the same applied ing model 62% of the time before 2017. Since commercial motives push them to focus on models used by industry are often those push- 2020, that share has risen to 91% of the time. topics that are profit oriented. Often such in- ing the boundaries of basic research [a situa- For example, sentiment analysis can be used centives yield outcomes in line with the pub- tion akin to what Donald E. Stokes referred to to understand the emotional tone of written lic interest, but not always. Were all cutting- as “Pasteur’s Quadrant” because of a similar Downloaded from https://www.science.org at Politecnico Di Milano on January 22, 2025 overlap between applied and basic research in pasteurization (14)]. For example, transform- AI research inputs ers, a type of deep-learning architecture, were (Top left) Percentage of US artificial intelligence (AI) PhDs hired by industry. (Top right) Growth of US university developed in 2017 by Google Brain research- AI research faculty hired by industry, with a reference line for the total size of computer science research ers. Not only was this an important step for- faculty. (Bottom) The total number of model parameters (a rough proxy for compute) for image recognition on ward in basic research, it was also applied ImageNet (see supplementary materials). almost immediately in models being used by industry. One benefit of this overlap is that it Percent of AI PhDs hired by industry AI research faculty hired by industry means that academic work can benefit indus- 75% 12 AI research faculty hired by industry* try directly (and industry has been supportive Computer science research faculty* of efforts to increase public investment in AI). 50 8 But this overlap also has a drawback: It means that industry domination of applied work also 25 4 gives it power to shape the direction of basic research. Given how broadly AI tools could be 0 0 applied across society, such a situation would 2004 2006 2008 2010 2012 2014 2016 2018 2020 2006 2008 2010 2012 2014 2016 2018 hand a small number of technology firms an Computing power usage in image classification models enormous amount of power over the direction of society. For many around the world, this Academia Academia-industry Industry 1010 collaboration concern is further heightened because these organizations are “foreign firms” to them. For model parameters 109 example, the Future of Life Institute argues Number of that “European companies are not developing 108 general-purpose AI systems and are unlikely to start doing so anytime soon due to their 107 relative competitive disadvantage vis-a-vis 106 American and Chinese players” (15). 2012 2014 2016 2018 2020 2012 2014 2016 2018 2020 2012 2014 2016 2018 2020 Even absent public alternatives to indus- * Data normalized to 2006. try research, one might imagine that regula- tion, through auditing or external monitoring work. Until 2017, academia led this bench- edge models from industry, situations would of industry AI, could be the solution. For ex- mark 77% of the time. But since 2020, indus- arise when no public-minded alternatives ample, in 2018 Joy Buolamwini, an academic, GRAPHIC: ADAPTED FROM AHMED ET. AL BY K. FRANKLIN/SCIENCE try alone or in collaboration has led 100% of would exist. This possibility raises concerns and Timnit Gebru, then a Microsoft em- the time. So whether measured by building akin to those about the pharmaceutical in- ployee, documented gender and racial biases state-of-the-art AI models (as measured by dustry, where investment disproportionately in commercial face recognition systems (16). either size or benchmark performance) or by neglects the needs of lower-income countries Establishing monitoring or auditing require- publishing in leading research outlets, our (11). Recent empirical work finds that “pri- ments (such as those in the Liability Rules for analysis shows industry’s increasing promi- vate sector AI researchers tend to specialise AI in Europe) can help mitigate these types of nence in AI outputs. in data-hungry and computationally inten- harms. However, if academics do not have ac- sive deep learning methods” and that this is cess to industry AI systems, or the resources POLICY IMPLICATIONS at the expense of “research involving other to develop their own competing models, their Industry’s increasing investment in AI has AI methods, research that considers the so- ability to interpret industry models or offer the potential to provide substantial benefits cietal and ethical implications of AI, and ap- public-interest alternatives will be limited. to society through the commercializing of plications in sectors like health” (12). These This is both because academics would be technology. Firms can create better prod- questions about the trajectory of AI and who unable to build the large models that seem ucts that benefit consumers [for example, controls it are also important for debates to be needed for cutting-edge performance, SCIENCE science.org 3 MONTH 2023 VOL 379 ISSUE 6635 885 INSIGHTS | P O L I C Y F O RU M but also because some useful capabilities of scribed since its launch almost a decade ago. must also be taken for the other key inputs AI systems seem to be “emergent,” meaning Chinese authorities have recently approved a to AI. Building public datasets will be im- that systems only gain these capabilities once “national computing power network system” portant but also a challenge because mod- they are particularly large (17). Some nega- (19) that will enable academics and others to ern AI training datasets can be billions of tive characteristics of models also seem to access data and computing power. In Europe, documents. Of particular interest should be scale with size [for example, toxicity in AI- similar initiatives have yet to emerge, al- creating important datasets for which there generated language, and stereotyping (7)]. In though there is a clear recognition of the risk. are no immediate commercial interests. It is either case, academics without access to suf- As French president Emmanuel Macron said, also important to provide the resources to ficient resources would be unable to mean- “if you want to manage your own choice of keep top AI researchers in academia. For ex- ingfully contribute to these important areas. society, your choice of civilization, you have ample, the Canada Research Chairs Program Around the world, this concern about aca- to be able to be an acting part of this AI revo- (CRCP), which provides salaries and research demia’s resource disadvantage in AI research lution” (20). For many countries, the scale funds, has proven to be a successful means of is being recognized, and policy responses are needed for these types of investments may be attracting and retaining top talent in Canada. beginning to emerge. In the United States, daunting. In such cases, the key question for For policy-makers working on this prob- the National AI Research Resource (NAIRR) policy-makers will be whether they can pool lem, the goal should not be that academia task force (18) has proposed the creation of sufficient resources with like-minded collab- does a particular share of research. Instead, a public research cloud and public datasets. orators to reach the scale needed to create AI the goal should be to ensure the presence In Canada, the national Advanced Research systems that reflect their own priorities. of sufficient capabilities to help audit or Downloaded from https://www.science.org at Politecnico Di Milano on January 22, 2025 Computing platform has been serving the Computing power is not the only area in monitor industry models or to produce country’s academics and has been oversub- which remedies should be offered. Steps alternative models designed with the pub- lic interest in mind. With these capabili- ties, academics can continue to shape the AI research outputs frontier of modern AI research and bench- (Top) The proportion of papers at leading AI conferences that have at least one industry co-author. (Middle) mark what responsible AI should look like. The fraction of the largest AI models that are from industry (3-year rolling average). (Bottom) Periods Without these capabilities, important pub- when the state-of-the-art model for leading AI benchmarks were from academia, industry, or collaborations lic interest AI work will be left behind. j (see supplementary materials). REF ERENCES AND NOTES 1. R. Shokri, V. Shmatikov, in Proceedings of the 22nd ACM Publications by industry at leading AI conferences SIGSAC Conference on Computer and Communications Security (ACM, 2015), pp. 1310–1321. 40% affiliated with industry 2. R. Jurowetzki, D. Hain, J. Mateos-Garcia, K. Stathoulopoulos, arXiv:2102.01648 [cs.CY] (2021). Percent of papers 30 3. “UK universities alarmed by poaching of top computer science brains,” Financial Times, 9 May 2018. 20 4. T. Evas et al.,“AI Watch: Estimating AI investments in the European Union” (Publications Office of the European Union, 2022). 10 5. G. Brockman, I. Sutskever, OpenAI,“Introducing OpenAI,” OpenAI, 11 December 2015. 0 6. G. Brockman, I. Sutskever, OpenAI,“OpenAI LP,” OpenAI, 11 March 2019. 2000 2002 2004 2006 2008 2010 2012 2014 2016 2018 2020 7. D. Ganguli et al., in 2022 ACM Conference on Fairness, Accountability, and Transparency (ACM, 2022), Percent of the 10 biggest AI models that are from industry pp. 1747–1764. 8. J. Sevilla, L. Heim, M. Hobbhahn, T. Besiroglu, A. Ho, 100% arXiv:2202.05924 [cs.LG] (2022). AI models from industry 9. N. C. Thompson, K. Greenewald, K. Lee, G. F. Manso, Percent of largest arXiv:2007.05558 [cs.LG] (2020). 75 10. E. Brynjolfsson, X. Hui, M. Liu, Manage. Sci. 65, 5449 (2019). 50 11. P. Trouiller et al., Global Health 267 (2017). 12. J. Klinger, J. Mateos-Garcia, K. A. Stathoulopoulos, SSRN 10.2139/ssrn.3698698 (2020). 25 13. E. Brynjolfsson, Daedalus 151, 272 (2022). 14. D. E. Stokes, Pasteur’s Quadrant: Basic Science and 0 Technological Innovation (Brookings, 1997). 15. Future of Life Institute,“Emerging non-European monopo- 2002 2004 2006 2008 2010 2012 2014 2016 2018 2020 lies in the global AI market” (Future of Life Institute, 2022); GRAPHIC: ADAPTED FROM AHMED ET. AL BY K. FRANKLIN/SCIENCE https://bit.ly/3k2ckD9. Increasing domination of industry in AI benchmarks 16. J. Buolamwini, T. Gebru, in Conference on Fairness, Accountability and Transparency (PMLR, 2018), Academia Academia-industry collaboration Industry pp. 77–91. 17. J. Wei et al., arXiv:2206.07682 [cs.CL] (2022). Image classification1 18. D. E. Ho, J. King, R. C. Wald, C. Wan,“Building a national AI research resource,” white paper (Stanford University Sentiment analysis2 Human-Centered Artificial Intelligence, 2021) 19. CAICT,“White paper on China’s computing power develop- Language modeling3 ment index” (CAICT, 2021); https://cset.georgetown.edu/ wp-content/uploads/t0402_compute_white_paper_ Semantic segmentation4 EN-2.pdf. 20. N. Berggruen, N. Gardels, The Washington Post, 27 Object detection5 September 2018. Machine translation6 SUPPLEMENTARY MATERIALS science.org/doi/10.1126/science.ade2420 2013 2014 2015 2016 2017 2018 2019 2020 2021 2022 2023 Benchmarks: 1ImageNet. 2SST-2. 3One Billion Word. 4ADE20K. 5COCO test-dev. 6WMT2014. 10.1126/science.ade2420 886 3 MONTH 2023 VOL 379 ISSUE 6635 science.org SCIENCE