Cramer's Rule and Matrix Inverse PDF
Document Details
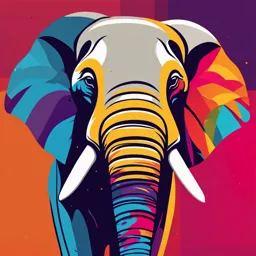
Uploaded by ResourcefulPanFlute
The University of Arizona
Tags
Summary
This document provides an explanation of Cramer's rule, properties of determinants, and how to construct the inverse matrix based on a given matrix. It also covers eigenvalues, eigenvectors, and eigenspaces in linear algebra. The document appears to be lecture notes or a study document.
Full Transcript
## 3.3 Cramer's Rule and Formula for the Inverse Suppose we want to solve the system Ax = b, where A is an (n x n) matrix, with columns a<sub>1</sub>,... a<sub>n</sub>, A = (a<sub>1</sub>|a<sub>2</sub>|...|a<sub>n</sub>). Make new matrices A<sub>i</sub> by replacing column # i with b: A<sub>1</s...
## 3.3 Cramer's Rule and Formula for the Inverse Suppose we want to solve the system Ax = b, where A is an (n x n) matrix, with columns a<sub>1</sub>,... a<sub>n</sub>, A = (a<sub>1</sub>|a<sub>2</sub>|...|a<sub>n</sub>). Make new matrices A<sub>i</sub> by replacing column # i with b: A<sub>1</sub> = (b|a<sub>2</sub>|...|a<sub>n</sub>), A<sub>2</sub> = (a<sub>1</sub>|b|a<sub>3</sub>|...|a<sub>n</sub>), A<sub>3</sub> = (a<sub>1</sub>|a<sub>2</sub>|...|b). and so on. **Theorem 7 Cramer's Rule** (All these matrices are (n x n)) For any b ∈ R<sup>n</sup> Solution of Ax = b can be Computed by formulas x<sub>1</sub> = det(A<sub>1</sub>)/det(A), x<sub>2</sub> = det(A<sub>2</sub>)/det(A), ... x<sub>n</sub> = det(A<sub>n</sub>)/det(A) I am skipping the proof. **Example** A=( <sup>1</sup>/<sub>3</sub> <sup>2</sup>/<sub>3</sub> ), b = ( <sup>3</sup>/<sub>-5</sub> ) Solve Ax = b. **Cramer's rule yields:** det(A) = -1 - 6 = -7 x<sub>1</sub> = det( <sup>3</sup>/<sub>-5</sub> <sup>2</sup>/<sub>3</sub> )/det (A) = -3 + 10 = 7 = -1 x<sub>2</sub> = det( <sup>1</sup>/<sub>3</sub> <sup>3</sup>/<sub>-5</sub> )/det (A) = -5 - 9 = -14 = 2 So, x = (<sup>-1</sup>/<sub>2</sub>). Check that this works, i.e. Ax = (<sup>3</sup>/<sub>-5</sub>) ## A Couple More Properties of Determinants This is from Section 2 **Theorem 5, Section 2** If A is an (n x n) matrix, then det(A<sup>T</sup>) = det(A). **Theorem 6, Section 2** If A and B are (n x n) matrices, then det(AB) = det(A)det(B) **Theorem 8, Section 3, A formula for the inverse of a matrix** Let A bean (n x n) matrix, and C<sub>ij</sub> be cofactors, i.e. C<sub>ij</sub> = (-1)<sup>i+j</sup> det(A<sub>ij</sub>), where A<sub>ij</sub> is A without column i and row j. Then A<sup>-1</sup> = 1/det(A) [ C<sub>11</sub> C<sub>12</sub> ... C<sub>1n</sub> ]<sup>T </sup> [ C<sub>n1</sub> C<sub>n2</sub> ... C<sub>nn</sub> ]<sup>T</sup> Note the transposition, This matrix is called the adjugate of A. (Not to be confused with the adjoint matrix that is something entirely different.) ## Chapter 5, Section 5.1 Eigenvectors and Eigenvalues **Def** Suppose A is an (n x n) matrix. If X is a non-zero vector such that Ax = λx, where λ is some scalar, then λ is called an eigenvalue of A, and x is an eigenvector of A corresponding to this λ. Note that x = 0 is not an eigenvector. λ is allowed to take any value, including 0. If x is an eigenvector of A, this means that Ax is parallel to x (pointing in the same direction). In other words, A preserves directions of its eigenvectors (but not the length). **Example** Suppose A = ( <sup>5</sup>/<sub>6</sub><sup>2</sup>/<sub>6</sub> ) and x = ( <sup>-5</sup>/<sub>6</sub> ) Compute Ax = ( <sup>5</sup>/<sub>6</sub> <sup>2</sup>/<sub>6</sub> )( <sup>-5</sup>/<sub>6</sub> ) = ( <sup>-25</sup>/<sub>36</sub> <sup>-10</sup>/<sub>36</sub> ) = - <sup>5</sup>/<sub>9</sub> (-5) = - λx. This means λ = -4 is an eigenvalue, and x is the corresponding eigenvector (together, they are an eigen pair). Suppose y = ( <sup>1</sup>/<sub>1</sub> ). Is y an eigenvector? Compute Ay = ( <sup>5</sup>/<sub>6</sub> <sup>2</sup>/<sub>6</sub> )( <sup>1</sup>/<sub>1</sub> ) = ( <sup>5</sup>/<sub>6</sub> <sup>2</sup>/<sub>6</sub> ) = λ( <sup>1</sup>/<sub>1</sub> ) = 4y. So y is an eigenvalue, and y is the eigenvector corresponding to λ = 4. Suppose z = ( <sup>-1</sup>/<sub>1</sub> ). Az = ( <sup>5</sup>/<sub>6</sub> <sup>2</sup>/<sub>6</sub> )( <sup>-1</sup>/<sub>1</sub> ) = ( -<sup>5</sup>/<sub>6</sub> <sup>-2</sup>/<sub>6</sub> ) ≠ λ (<sup>-1</sup>/<sub>1</sub>) Since vectors ( <sup>1</sup>/<sub>1</sub> ) and ( -<sup>5</sup>/<sub>6</sub> <sup>-2</sup>/<sub>6</sub> ) are not parallel, So, z is not an eigenvector. Note that the problem of finding both λ and x, just solving from the equation Ax = λx is more difficult than a linear system ## Suppose we are interested in a particular value of λ. Then, in order to find x, we need to solve the linear system Ax = λx. This can be re-written using identity matrix I: Ax = λx Ax = λIx Ax - λIx = 0 (A - λI)x = 0 This means we are solving a homogeneous system. There are two possibilities: Either there is only a trivial solution (recall x = 0 cannot be an eigenvector) then λ is not an eigenvalue! Or, there is a non-trivial solution. Then, x is an eigenvector. But, when a non-trivial solution exists, it is not unique. There is a whole null space of the matrix A - λI, i.e. there are infinitely many eigenvectors corresponding to eigenvalue λ). These eigenvectors form "an eigenspace" corresponding to the eigenvalue λ. **Example 4** A = ( <sup>4</sup>/<sub>2</sub> <sup>-1</sup>/<sub>-1</sub> <sup>6</sup>/<sub>6</sub> ) Somebody has informed us that the eigenvalue λ = 2. Find the eigenvectors and the eigenspace. The eigenvectors are all the solutions of (A - λI)x = 0 Take λ = 2. Then (A - 2I) = ( <sup>4</sup>/<sub>2</sub> <sup>-1</sup>/<sub>-1</sub> <sup>6</sup>/<sub>6</sub> ) - 2( <sup>1</sup>/<sub>0</sub> <sup>0</sup>/<sub>1</sub> ) = ( <sup>2</sup>/<sub>2</sub> <sup>-1</sup>/<sub>-1</sub> <sup>6</sup>/<sub>6</sub> ) - ( <sup>2</sup>/<sub>0</sub> <sup>0</sup>/<sub>2</sub> ) = ( <sup>2</sup>/<sub>2</sub> <sup>-1</sup>/<sub>-1</sub> <sup>6</sup>/<sub>6</sub>) - ( <sup>2</sup>/<sub>0</sub> <sup>0</sup>/<sub>2</sub> ) = ( <sup>2</sup>/<sub>2</sub> <sup>-1</sup>/<sub>-1</sub> <sup>6</sup>/<sub>6</sub> ) The augmented matrix for (A - 2I)x = 0 is ( <sup>2</sup>/<sub>2</sub> <sup>-1</sup>/<sub>-1</sub> <sup>6</sup>/<sub>6</sub> ) ~ ( <sup>2</sup>/<sub>0</sub> <sup>0</sup>/<sub>0</sub> <sup>6</sup>/<sub>0</sub> ) ~ ( <sup>2</sup>/<sub>0</sub> <sup>0</sup>/<sub>0</sub> <sup>0</sup>/<sub>0</sub> ) This is equivalent to the solution 2x<sub>1</sub> - x<sub>2</sub> + 6x<sub>3</sub> = 0 with X2, X3 being free variables, or x<sub>1</sub> = <sup>1</sup>/<sub>2</sub> x<sub>2</sub> - <sup>3</sup>/<sub>2</sub> x<sub>3</sub>. So, x = ( <sup>1</sup>/<sub>2</sub> x<sub>2</sub> - <sup>3</sup>/<sub>2</sub> x<sub>3</sub> ) = ( <sup>1</sup>/<sub>2</sub> ) x<sub>2</sub> + ( <sup>-3</sup>/<sub>2</sub> ) x<sub>3</sub> So, vectors ( <sup>1</sup>/<sub>2</sub> ) and ( <sup>-3</sup>/<sub>2</sub> ) are eigenvectors corresponding to λ = 2. But most importantly, any linear combination of these two vectors is also an eigenvector corresponding to λ = 2. So, the eigenspace is equal to span{( <sup>1</sup>/<sub>2</sub>), ( <sup>-3</sup>/<sub>2</sub>)}. **Observation** For a given λ, system (A - λI)x = 0 has a non-trivial solution iff matrix A - λI is singular, But a matrix is singular iff its determinant is equal to 0. Therefore, A - λI has eigenvectors iff det(A - λI) = 0. **Theorem 1** If A is a triangular matrix (upper- or lower), the eigenvalues of A are the diagonal entries. **Proof** (For a (3 x 3) matrix) Suppose A = ( <sup>a<sub>11</sub></sup>/<sub>0</sub> <sup>a<sub>12</sub></sup>/<sub>a<sub>22</sub></sub> <sup>a<sub>13</sub></sup>/<sub>a<sub>23</sub></sub>), Then det(A - λI) = det ( <sup>a<sub>11</sub>-λ</sup>/<sub>0</sub> <sup>a<sub>12</sub></sup>/<sub>a<sub>22</sub>-λ</sub> <sup>a<sub>13</sub></sup>/<sub>a<sub>23</sub>-λ</sub> ) = (a<sub>11</sub> - λ)(a<sub>22</sub> - λ)(a<sub>33</sub>- λ) (because det of a triangular matrix is the product of diagonal entries) Solve (a<sub>11</sub> - λ)(a<sub>22</sub> - λ)(a<sub>33</sub>- λ) = 0. There are three possible solutions: λ = a<sub>11</sub>, λ = a<sub>22</sub>, λ = a<sub>33</sub>. Proven.