Non-Parametric Tests PDF
Document Details
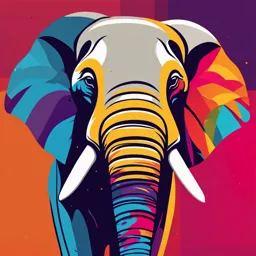
Uploaded by BriskPiccoloTrumpet
Taibah University
2024
Tags
Summary
This document is a lecture or course material on nonparametric tests, specifically for pharmaceutical sciences, part of the biostatistics curriculum. It outlines the types of nonparametric tests, their application scenarios, and provides clear explanations of use.
Full Transcript
Non-Parametric tests Biostatistics for Pharmaceutical Sciences - PHRM 103 – Theory Department of Pharmacy Practice, College of Pharmacy, Taibah University 2024-1446...
Non-Parametric tests Biostatistics for Pharmaceutical Sciences - PHRM 103 – Theory Department of Pharmacy Practice, College of Pharmacy, Taibah University 2024-1446 1 Learning Objectives Learning Objectives: 1. Understand the fundamental principles and applications of nonparametric tests. 2. Identify when to use nonparametric tests versus parametric tests. 3. Apply basic nonparametric tests such as Wilcoxon Signed-Rank, Mann-Whitney U, Kruskal-Wallis, and Spearman’s Rank Correlation. 4. Interpret the results of nonparametric tests and understand their implications in real-world pharmacy research. 5. Develop the ability to report nonparametric test outcomes in a clear and structured manner. 2 3 Introduction to Nonparametric Tests Definition: Nonparametric tests are statistical tests that do not assume a specific distribution for the data. Purpose: Used when data do not meet the assumptions required for parametric tests (e.g., normal distribution). Examples in Pharmacy: Comparing patient satisfaction scores, analyzing treatment effects where data are not normally distributed. 4 Parametric vs. Nonparametric Tests Parametric Tests: Require assumptions like normal distribution and homogeneity of variance (e.g., t-test, ANOVA). Nonparametric Tests: Do not require these assumptions and can be used with ordinal data or small sample sizes. 5 Advantages of Nonparametric Tests Flexibility: Suitable for non-normal data and ordinal data. Robustness: Not affected by outliers as much as parametric tests. Ease of Use: Often simpler to apply and interpret. 6 Common Nonparametric Tests Overview Wilcoxon Signed-Rank Test: For paired or related samples. Mann-Whitney U Test: For comparing two independent groups. Kruskal-Wallis Test: For comparing more than two independent groups. Ch-square test: Used to test relationships between categorical variables. Spearman’s Rank Correlation: Measures the strength of association between two variables. 7 Wilcoxon Signed-Rank Test Purpose: Compares the median differences between paired observations. Example: Comparing blood pressure readings before and after treatment in the same patients. How It Works: Calculate the differences between paired observations. Rank the absolute differences. Sum the ranks of positive and negative differences separately. Determine significance using the test statistic. 8 Mann-Whitney U Test Purpose: Compares two independent groups to determine if their distributions are different. Example: Comparing test scores between two groups of pharmacy students. Steps: Rank all data points across both groups together. Calculate the sum of ranks for each group. Use the U statistic to determine significance. Interpretation: If the p-value < 0.05, conclude that there is a significant difference between the two groups. 9 Kruskal-Wallis Test Purpose: Extension of the Mann-Whitney U Test for comparing more than two independent groups. Example: Comparing satisfaction scores of patients across three different pharmacies. Steps: Rank all data points together. Calculate the sum of ranks for each group. Compute the Kruskal-Wallis H statistic. Interpretation: If p-value < 0.05, at least one group differs significantly. 10 Spearman’s Rank Correlation Purpose: Measures the strength and direction of association between two variables. Example: Relationship between patient age and adherence to medication. How to Calculate: Rank the data points for both variables. Compute the differences between the ranks. Calculate Spearman’s rho using the formula. Interpretation: Rho value close to +1 or -1 indicates strong correlation, while 0 indicates no correlation. 11 Ch-square test Purpose: The Chi-Square Test is primarily used to: Test for independence: Determine if there is an association between two categorical variables. Test for goodness of fit: Assess whether observed data matches an expected distribution. Example: A hospital wants to find out if patient satisfaction (categorized as “Satisfied,” “Neutral,” “Dissatisfied”) is associated with the type of service received (e.g., “In-person” or “Online”). 12 Ch-square test Interpretation: If the p-value is less than 0.05, it indicates that the difference in patient satisfaction levels between in-person and online services is statistically significant, suggesting that the type of service impacts patient satisfaction. If the p-value is greater than 0.05, there is not enough evidence to say that patient satisfaction is related to service type. 13 Real-Life Applications in Pharmacy Scenario: A pharmacy wants to assess whether a new service (e.g., a medication counseling program) has improved patient satisfaction. Appropriate Test: Use the Wilcoxon Signed-Rank Test since it is designed for paired samples where the distribution of the differences between pre- and post-service satisfaction scores may not be normal. Interpretation: If the p-value < 0.05, conclude that the new service significantly impacted adherence rates. 14 Real-Life Applications in Pharmacy Scenario: A study aims to analyze whether adherence rates to a chronic medication differ between two age groups (e.g., patients under 50 and those 50+). Appropriate Test: Use the Mann-Whitney U Test to compare the two independent groups because adherence rates may not follow a normal distribution. 15 Real-Life Applications in Pharmacy Scenario: A study investigates whether patient satisfaction scores differ across three pharmacy branches (A, B, and C) offering varying levels of service. Appropriate Test: Use the Kruskal-Wallis Test to compare more than two independent groups where data distribution might not be normal. Interpretation: If the p-value < 0.05, there is a significant difference in satisfaction scores between the branches, suggesting that at least one branch has a different satisfaction level from the others. 16 Real-Life Applications in Pharmacy Scenario: A study aims to determine the relationship between the number of pharmacist consultations a patient receives and their medication adherence level. Appropriate Test: Use Spearman’s Rank Correlation to measure the strength and direction of the association between these two variables, especially when the relationship is not linear. Interpretation: A positive rho close to +1 indicates a strong positive correlation, meaning that more consultations are associated with better adherence. 17 Purpose Parametric Test Nonparametric Test Comments Comparing two t-test (independent Mann-Whitney U Test Use the Mann-Whitney U independent groups samples) Test when data is not normally distributed or for ordinal data. Comparing two Paired t-test Wilcoxon Signed-Rank Test Wilcoxon is suitable for related/paired samples paired observations with non-normal data. Comparing more than two One-way ANOVA Kruskal-Wallis Test Use Kruskal-Wallis when independent groups data is not normally distributed across groups. Measuring the association Pearson’s Correlation Spearman’s Rank Spearman’s is used for between two variables Correlation ordinal data or non-linear relationships. 18