Introduction to Statistics PDF
Document Details
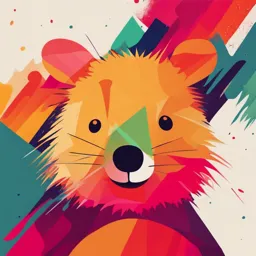
Uploaded by FriendlyTrust
University of KwaZulu-Natal
2019
Dr B. Tlou
Tags
Summary
This document is a presentation on the introductory concepts of statistics, specifically focusing on data exploration, summarization, and different types of statistical analyses. It features various types of data distributions like categorical and numerical data, with visual presentations like histograms, bar graphs, and frequency polygons, ultimately discussing summary statistics, including measures of central tendency (mean, median, mode) and measures of variability (range, standard deviation, IQR).
Full Transcript
Exploring and summarising data (26 AUG 2019) Dr B. Tlou Discipline of Public Health Medicine School of Nursing and Public Health University of KwaZulu-Natal UKZN INSPIRING GREATNESS Introduction to Statistics “Statisticsare like bikinis. What they reveal is suggesti...
Exploring and summarising data (26 AUG 2019) Dr B. Tlou Discipline of Public Health Medicine School of Nursing and Public Health University of KwaZulu-Natal UKZN INSPIRING GREATNESS Introduction to Statistics “Statisticsare like bikinis. What they reveal is suggestive, but what they conceal is vital.” Aaron Levenstein + Learning outcomes Describe the statistical method and its application Differentiate between different types of variables Use frequency tables and graphs to present data Calculate summary measures for numerical data Use Excel for data presentation and calculation of summary statistics + Some definitions “Statistics is the science of collecting, summarizing, presenting and interpreting data, and of using data to estimate the magnitude of associations and test hypotheses.” Betty Kirkwood "Statistics is a curious amalgam of mathematics, logic and judgment" Douglas Altman + Scope of statistics Statistics divided into: Descriptive statistics Methods to summarise and present data Analytic statistics Methods to test associations and draw inferences from the sample to a population + Statistics in epidemiology Statistics is a way of handling variability. It allows us to separate out the real effect from that which could have happened due to chance variability (random error). It allows us to make inferences about a larger population from a smaller sample. + What do we measure? Variables = characteristics about exposure or health event that vary among people enrolled in the study Types of variables Categorical Numerical The type of variable influences the type of statistical analysis applied + Types of variables TYPES OF VARIABLES Numerical Categorical Continuous Discrete Binary Nominal Ordinal Parity Hypertensive (Y/N) Social Class Heart beats per minute Weight Employed (Y/N) + Categorical variables (Qualitative variables) Nominal: Categories in no order, are identified by name e.g. gender, marital status, ethnic group Ordinal There is some order and can be recorded in categories e.g. socio-economic status, severity of a disease Binary (or dichotomous) Variables that have only two possible categories e.g. alive or dead, smoking status + Examples What is your religion? Taking ARVs helps you live Christian, Muslim, Hindu, Shembe, longer. African Traditional, Other, None Strongly disagree to strongly Does your household have a agree (code 1-5) TV, fridge, cell phone? Yes or No At what age did you start having sex Does everyone who needs 18years. retroviral drugs? Yes, No, Don’t know Highest education? No school, up to grade 4, grade 5-8, grade9-12, tertiary + Numerical variables (Quantitative variables) Discrete Numbers that can only take on certain values e.g. count of events, count of people (55, 314, 21, etc) Continuous A measure that can take on any value e.g. height, blood pressure, weight (24.265, 1.925, etc) + Overview of steps in data analysis Check your data – cleaning (most time consuming) Exploratory data analysis Through graphical display Summarise the data Categorical variables summarised by number and percentage in a certain category Numerical variables summarised by measures of central location and variability (spread) Estimate population parameters (what does it say about the population being studied) By applying statistical analyses Types of analyses + Data checking/cleaning Identify errors Can occur when data is coded, transcribed, entered Double entry and comparing of discrepancies advisable Categorical variables Check plausibility Sex=G Check missing values Numerical values Check plausibility – are extremes possible E.g. Height=250cm Height=5cm What does ‘0’ mean What do missing values mean Check missing values – go back to raw data Use of computer software that prevents wrong entries + …data checking/cleaning Cross checking variables If demographic information is asked more than once, do the answers agree E.g. sex1=M sex2=F In longitudinal studies are the changes in values between assessments plausible E.g. age at week 1= 25yrs; at week 52= 35yrs Do related questions give plausible results E.g. sex= male; use of oral contraceptive= yes Do you smoke = No; age at which started smoking = 11 + Check the data on this table: Subject Age Sex Current Ever Age Smoker smoked Started smoking 1 45 M Y Y 15 2 32 F Y N - 3 35 F N Y 31 4 46 M N Y 40 5 25 M Y Y 28 6 20 M N N - 7 18 F Y Y 16 8 20 M Y Y - 9 21 F Y Y 18 10 30 N N 20 + Summarising data Summarizing data is the first step of statistical analysis In descriptive studies Use frequency distributions for categorical variables Use summary statistics for quantitative variables + Summarising & Presenting Categorical Data + Summarising Categorical Data Frequency distribution Count the number of observations in each category Calculate the percentage (relative frequency) for each category For e.g. Sex of 40 respondents SEX Number % Males 18 45% Females 22 55% TOTAL 40 100% + Presenting categorical data Bar graph Used to display data from one variable table Each value or category is represented by a bar The length of the bar is proportional to the number of events in that category Makes it easy to compare the relative size of the different categories Bars can be presented either horizontally or vertically + Bar Graph What is wrong with the way these data are presented? 24 22 20 No 18 16 14 Males Females Sex + Bar Graph Sex distribution of people living in Camp A – June 2001 24 22 20 No 18 16 14 Males Females Sex + Bar graph vs. Histogram Bars are separated Bars are joined Shows the frequency distribution Shows the frequency of a variable with discrete, non- distribution of a continuous continuous categories variable E.g. gender or race E.g. age categories in the population pyramid + Pie Chart Useful for showing the component parts of one single group or variable i.e. Can only be used where the categories add up to 100% Each category is represented by a slice of the pie The size of the slice of the pie represents the number of observations (or percentage) in each group + Summarising & Presenting Numerical Data + Tables When the variable takes on a limited number of values (8-10) list all the individual values + Tables: Class intervals When the variable can take on more than 10 values – group into class intervals (4-8) + Developing Class Intervals Mutually exclusive Include all the data Fractional data – round off > 0.5 round up (6.5 becomes 7) < 0.5 round down (6.4 becomes 6) Grouped Frequency Distribution Birth weight (Kg) No of births 1.76 - 2.0 4 2.01 - 2.25 3 2.26 - 2.5 12 2.51 - 2.75 34 2.76 - 3.0 115 3.01 - 3.25 175 3.26 - 3.5 281 3.51 - 3.75 261 3.76 - 4.0 212 4.01 - 4.25 94 4.26 - 4.5 47 4.51 - 4.75 14 4.76 - 5.0 6 5.01 - 5.25 2 Total Births 1260 + Graphical display of numerical data Also known as exploratory data analysis Shows the distribution of numerical data Detects: Strange values Patterns Relationships Whether intended statistical analyses are appropriate + Symmetrical versus asymmetrical distributions Symmetry of data detected in: Histograms Box plots Relationships detected through: Bivariate (or scatter plots) Histogram 300 250 200 Frequency 150 100 50 0 2 2.25 2.5 2.75 3 3.25 3.5 3.75 4 4.25 4.5 4.75 5 5.25 Birth weight (Kg) Histogram & Frequency Polygon 300 250 200 Frequency 150 100 50 0 2 2.25 2.5 2.75 3 3.25 3.5 3.75 4 4.25 4.5 4.75 5 5.25 Birth weight (Kg) Frequency Polygon 300 250 200 Frequency 150 100 50 0 2 2.25 2.5 2.75 3 3.25 3.5 3.75 4 4.25 4.5 4.75 5 5.25 Birth weight (Kg) Frequency Polygon 300 250 200 Frequency 150 100 50 0 2 2.25 2.5 2.75 3 3.25 3.5 3.75 4 4.25 4.5 4.75 5 5.25 Birth weight (Kg) Normal & Skewed Distributions tail tail Positively Skewed Negatively skewed Normal & Skewed Distributions Bimodal + Box and whisker plot 180 160 140 120 100 80 60 + Box (and whisker) plot Shows the distribution of data Not all observations are plotted Only selected summary values Median (or 50th percentile) 25th percentile 75th percentile Maximum and minimum values + Obtaining the Centile k × (n+1) 100 Where k represents the centile (25th, 50th or 75th) and n represents the sample size + Box (and whisker)plot E.g. Hypertension study: Diastolic blood pressure was measured on a sample of 16 subjects The values are: 75, 84, 80, 97, 105, 188, 64, 78, 68, 86, 79, 105, 89, 88, 93, 92 Draw a box and whisker plot to summarise this data + Box and whisker plot Median = 87 180 25th percentile = 78.25 160 75th percentile = 96 140 Maximum value = 188 120 Minimum value = 64 100 80 60 Different KAP distributions by staff categories S 8 6 0 p m g a P 0 e d tr e t t a 0 o ad c t c e u e i t f c d i c a f a e e s te l d a c t/ s g g s c a ce e o o t s er e c e go p e o e c r e y t Knowledge Attitudes Behaviours Administration General staff Health professionals + Bivariate (or scatter plot) Used to plot two continuous numerical variables against one another (e.g. height and weight) Used to explore the relationship between variables Compact pattern indicates high correlation Drawing the scatter plot should precede a statistical analysis Plot one variable on the X-axis and plot the other on the Y-axis + Bivariate plot of height and weight + Bivariate plot of the relationship between lung function and age 20 18 16 Laser Doppler VAR (au) 14 12 10 8 6 4 2 0 0 0.2 0.4 0.6 0.8 1 1.2 1.4 TcpO2 Index Failed Healed + Summarising numerical data Measures of central tendency In symmetrical distributions Mean In asymmetrical distributions Median Geometric mean Mode Measures of variability Range Standard deviation Interquartile range + Measures of central location In symmetrical distributions Mean In asymmetrical distributions Median Geometric mean Mode + Mean Arithmetic mean or average Obtained by adding all the numbers together and dividing the total by the number of numbers Useful in symmetrical distributions of data because sensitive to extreme values In mathematical shorthand/formula ∑x x= n Where: x = the mean ∑x = the sum of all the numbers n = sample size + Median The median represents the middle of the set (i.e. half the observations above and half the observations below) Not sensitive to extreme values - Useful in asymmetrical distributions of data Arrange the numbers in order Then find the middle number In data sets with an odd number of numbers, the median is the number which divides the set in half In data sets with an even number of numbers, the median is the average between the two middle numbers + Geometric mean Used less commonly Used for data that is not symmetrically distributed, but follows an exponential pattern (1, 2, 4, 8, 16, etc.) or a logarithmic pattern (1/2; ¼; 1/8; 1/16; etc.) It is the mean or average of a set of data measured on a logarithmic scale Calculated with the assistance of a scientific calculator + Mode The number that occurs most frequently Useful if we are interested in knowing which values are most popular or to assess whether a measuring instrument has a preference for a certain value Every set of data has one mean and one median, but could have one mode, no mode, or multiple modes Ages of 40 respondents living in Area A in 2000 13 32 28 27 35 22 65 28 47 15 36 16 25 39 19 12 22 45 72 44 53 13 18 15 52 39 31 57 15 59 55 37 43 29 14 61 14 43 83 44 + Measures of variability (or dispersion) Range Standard deviation Interquartile range + Range The largest minus the smallest value or denoted by indicating the smallest and largest value separately 12, 13, 13, 14, 14, 15, 15, 15, 16, 18, 19, 22, 22, 25, 27, 28, 28, 29, 31, 32, 35, 36, 37, 39, 39, 43, 43, 44, 44, 45, 47, 52, 53, 55, 57, 59, 61, 65, 72, 83 What is the range? + Range 12 to 83 or 71? + Standard deviation Gives the average distance from the mean Used with symmetrical data Calculated as follows: First calculate the mean Then find the difference (deviation) of each number from the mean Square these differences (multiply each difference by itself) Add up all the squared differences Divide by the sample size (n) minus 1 Take the square root of the answer + Standard deviation Data value Difference from Squared mean (x = 7) difference 3 -4 16 4 -3 9 4 -3 9 6 -1 1 7 0 0 12 5 25 13 6 36 SUM: 49 0 96 + Standard deviation n=7 and x=7 96 96 σ= = = 16 = 4 7 -1 6 + Variance The variance is the square of the standard deviation Or put in another way – the standard deviation is the square root of the variance std deviation =s= ∑ xi - x( ) 2 2 variance = s = ∑ x-x ( ) 2 n-1 n -1 + Gaussian Curve + Interquartile range Used to summarise data that is asymmetrical (i.e. where there are outliers) Therefore used with the median Calculation – 75th percentile minus the 25th percentile Or can give the 25th and the 75th percentile Gives the range of values between which 50% of the data in the sample lie + Interquartile range In the following data set of 10 children with the following ages: 2, 4, 6, 7, 8, 10, 11, 12, 14, 16 What is the median? What is the 25th percentile? What is the 75th percentile? What is the interquartile range? + Interquartile range Median = 9 25th percentile = 5.5 75th percentile = 12.5 Interquartile range = 7 or 5.5 to 12.5 + Graphic representation of IQR + Introduction to Inference The ultimate goal of statistics is to say something about the population from which the sample was selected. One way to start is to derive a range of values between which we are relatively sure the true population value will lie based on the sample estimate. Every time we take a sample we introduce error – sampling error Derive imprecise estimates – must therefore calculate the precision of a sample. + Precision – Confidence Intervals The precision of an estimate is dependant on the variability of the data and the sample size Confidence intervals give a range of values considered plausible for the population, based on the sample data Calculated using the sample estimate, the standard error, the degree of certainty we want (95% or 99%), and the cut off value for the probability distribution + Example 10 clinic attenders, 4 HIV+ % HIV+ is 40% (95CI 12%-74%) Wide confidence interval because the sample is small Cannot say what the HIV prevalence is 100 clinic attenders, 40 HIV+ %HIV+ is 40% (95CI 30% - 50%) Narrower confidence interval because of the larger sample Can say with more certainty what is the HIV prevalence rate amongst clinic attenders KEALEBOGA NGIYABONGA THANK YOU