Module 3 Lesson 2 - Correlation PDF
Document Details
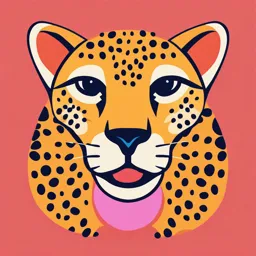
Uploaded by EventfulLorentz
WorldQuant University
Tags
Related
- Financial Markets And Institutions PDF
- Introduction To Financial Markets PDF
- The Capital Structure of Chaebols PDF
- Mestrado em Matemática Financeira - Econometria dos Mercados Financeiros (FCUL e ISCTE) PDF
- Empirical Banking Finance and Growth Levine and Zervos PDF
- Introducción al Sector Inmobiliario PDF
Summary
This is a lesson discussing correlation and diversification in the financial markets. The document explains various types of correlation and their effects on portfolio volatility. It demonstrates how a diversified portfolio can outperform concentrated ones.
Full Transcript
1/23/25, 9:02 AM My Path Task | WorldQuant University Home My Courses Financial Markets M3: Correlation Lesson Notes Lesson Notes MODULE 3 | LESSON 2 CORRELATION Reading Time...
1/23/25, 9:02 AM My Path Task | WorldQuant University Home My Courses Financial Markets M3: Correlation Lesson Notes Lesson Notes MODULE 3 | LESSON 2 CORRELATION Reading Time 45 minutes Prior Return, Variance, Standard Deviation Knowledge Diversification, Correlation, Hedging, Pearson Correlation, Spearman Keywords Correlation In the previous lesson, we saw the role that return and volatility played at the portfolio level. The standard deviation was very much dependent on the value of correlation between the two securities in the portfolio. In this lesson, we examine the correlation value up close and assess its impact upon the portfolio's volatility. We'll also examine a correlation measure that works when the association between variables is not linear. 1. Diversification by Lowering Correlation Diversification is the absence of perfect correlation. You've often heard that people want to have more diversification in their portfolio. In finance, diversification is an investing strategy used to manage risk. Instead of concentrating money in a single company, industry, sector, or asset class, investors diversify their investments across a range of different companies, industries, and asset classes. Let's be clear about it: diversification is not a strategy aimed at maximizing portfolio returns. In fact, investors who concentrate capital in a limited number of investments might, over a specific time, outperform a diversified investor. However, over time, a diversified portfolio generally outperforms the majority of more focused ones. One key element of diversification is owning investments that perform differently in similar markets. For example, when stock prices rise, bond yields tend to fall in general. We describe this by saying that stocks and bonds are negatively correlated. While not each and every investment in a well-diversified portfolio will be negatively correlated, the goal of diversification is to buy assets that do not move in lockstep with one another. https://learn.wqu.edu/my-path/courses/financial-markets/modules/m-3-correlation/tasks/lesson-2-correlation-lesson-notes 1/11 1/23/25, 9:02 AM My Path Task | WorldQuant University Imagine you had a team of 10 movie critics. They agree with each other so much that their opinions are perfectly correlated. Do you need all 10 to get an opinion? In other words, if you had one of the reviewers rate a movie and that person presented their view, then would you need the other nine reviewers? No. A perfect correlation would indicate that the reviews of the remaining nine people would be the same. Perfect correlation might sound harmonious and friendly, but it provides no difference of opinion. Stated in the opposite way, perfect correlation lacks diversification. Indeed, a diverse-thinking group would offer diverse opinions and therefore diversification of thought. Correlation is a statistical measure that can range from -1 to 1, and if correlation equals 1, then there is no diversification. Whenever the correlation is less than 1--by any amount--you have diversification. Although it may not seem like it, there is a diversification benefit even at a correlation of.99. The diversification benefit is not much, but it exists. Now if the correlation decreases, the diversification benefit increases. In fact, the lower correlation goes, the greater this benefit becomes. If correlation goes down to as far as -1, then the diversification benefit is maximized. In the next section, let's compute the covariance with different values of correlation. Then, we'll quantify the diversification benefit. 2. Negative Correlations Let us emphasize some of these correlations. In this section, we’ll consider two different values of negative correlation. We'll be using the same example we've considered several times already, except with a change to the value of correlation. Example. Suppose we have an equally weighted portfolio between A and B and that 𝑟𝐴=5%, 𝑟𝐵 =11%, 𝜎𝐴=3%, 𝜎𝐵 =8%, and 𝜌𝐴, 𝐵 =1.0, 0.5, 0, -0.5, -1. What is the portfolio standard deviation in each case? Answer. Using the formula for standard deviation given in the previous lesson, we can compute all these standard deviations. If then: If $\rho_{A,B} = 0.5, then When we find Moving to we get instead https://learn.wqu.edu/my-path/courses/financial-markets/modules/m-3-correlation/tasks/lesson-2-correlation-lesson-notes 2/11 1/23/25, 9:02 AM My Path Task | WorldQuant University Finally, when we achieve the lowest possible standard deviation for this portfolio, which is A correlation of -1 means that you have perfect negative correlation. In financial terms, this is known as a perfect hedge. In the real world, it would be extremely difficult to find an example where two securities have a correlation that is -1. As you can see from historical correlations, it is hard to get close to either -1 or +1. In the real world, it's extremely difficult to find an example where two securities have a correlation of -1 because it would mean that any time one security gains, the other is always losing, thereby reducing the volatility of the combined portfolio. A perfect hedge is a trader's dream whereby they can hedge the risk of a particular security, such as a derivative, with a perfect negatively correlated underlying that would effectively guarantee that there's a risk-free component. Indeed, in the theoretical world, such a case does exist. When we derive a formula for option pricing, we will find exactly that: a correlation of -1 that gives us a perfect hedge. In our last example, we can see that when the correlation is minus 1, we obtain the minimum variance portfolio (for our two assets). Notice that this result is true as long as we require that all assets have nonnegative weights. The result might seem unintuitive, but to emphasize, a portfolio can have a smaller standard deviation (or variance) than either of the individual standard deviations (or variances). If we were to allow for short positions (negative weights), the result could fail to hold. If, in the same example, we were to choose we would end up with the same standard deviation as the asset with the lower standard deviation: Generally, it's not possible to match the higher standard deviation. As we found with we have the same variance as Stock A. Should we expect a better return with Stock A alone or with a 50% A and 50% B portfolio, given that there is -77% correlation? Having some amount of Stock B will only increase the return, so clearly, the portfolio with A and B should have a higher expected return. This illustrates the benefit of diversification: a higher expected return for the same amount of risk. Therefore, the portfolio’s Sharpe ratio exceeds that of Stock A. The takeaway is this: portfolios can perform better if we simply add another security to which there's a small amount of correlation but a higher expected return. Constructing portfolios is not our goal here. That will be the goal of a course devoted to Portfolio Management. But for now, it's important to understand that correlation determines diversification. Let’s see what happens when you set correlation to 0. In the case of zero correlation, we should notice that we end up with This number is less than the straight average of of the standard deviation of assets A and B. This confirms that https://learn.wqu.edu/my-path/courses/financial-markets/modules/m-3-correlation/tasks/lesson-2-correlation-lesson-notes 3/11 1/23/25, 9:02 AM My Path Task | WorldQuant University standard deviation is not a linear entity. Finally, in the case where we observe that zero correlation means that if one security has a positive return, the other security can have a positive or negative return with equal probability. Knowing the direction of one return tells us nothing about the direction of the other one. We end up with a value in between and but not equal to the midpoint of and As our final example, when we end up with , which is exactly the midpoint between and In other words, when there is perfect positive correlation, then the variances combine with each other. 8% + 3% = 11%. The average of these is effectively half that number: 5.5%. Despite our final example, do not lose sight of the fact that the effects of standard deviation on correlation are not linear. Between correlations of -1 and 1, we do NOT expect to see a straight line but rather a curve. Students can utilize this Shiny application to observe how optimal portfolios are constructed along a curve called the Efficient Frontier. 3. A More Elegant Look at the Diversification Benefit Let's consider the diversification benefit quantitatively. When our correlation was 1, the standard deviation of our portfolio invested 50-50 in assets A and B was 5.500%. When our correlation was 0, our standard deviation was 4.272%. We diversify our portfolio by using securities that have less than perfect correlation (less than 1). In doing so, we lower the portfolio's variance from 5.5% to 4.272%, By diversifying the portfolio even further (negative correlations), we can lower the standard deviation of the portfolio down to By diversifying our portfolio, we benefit from lower portfolio volatility. Here's a formula for how much the variance is reduced when compared to the situation of perfect correlation ( ). If the portfolio has a correlation of 1, there is no diversification benefit. Just plug the value of 1 into the variance reduction formula, and you see the first term goes to 0. (The same is true for the standard deviation reduction formula.) Any correlation less than 1 will cause some reduction in the standard deviation. Clearly, this will make Sharpe ratios more attractive, earning a relatively larger amount of return per unit of risk. Correlations that are 0 make the reduction large, but if correlations were negative, that would maximize the reduction. To complete what we learned about diversification, consider a portfolio, P, containing a single asset, A. Then, the variance of this portfolio is clearly https://learn.wqu.edu/my-path/courses/financial-markets/modules/m-3-correlation/tasks/lesson-2-correlation-lesson-notes 4/11 1/23/25, 9:02 AM My Path Task | WorldQuant University Now, consider a new portfolio, Q, obtained by adding a second asset, B, to portfolio P. This means that now we will have to characterize the allocations in the portfolio via weights, and Since the weights have to sum to 1, we must have The weights can be positive or negative, but they must sum to 1; therefore, it is not possible for both to be negative. The variance of the new portfolio is then given by Now we ask ourselves: under what circumstances does portfolio Q allow for a diversification benefit? Well, for this, we need to have Comparing the two expressions for and with a little algebra, we find that we need to have Using the fact that we finally get: However, it should be clear that these simple comparisons fail when we consider portfolios consisting of 3 or more assets, and overall, we cannot rely on such easily concocted formulae. Nevertheless, we can still compute the volatility of different portfolios and check if adding certain assets or changing the weights of the individual assets in the portfolios provides diversification benefits or not. This will be covered in depth in the Portfolio Management course. Figure 1: Portfolio Standard Deviation Surface https://learn.wqu.edu/my-path/courses/financial-markets/modules/m-3-correlation/tasks/lesson-2-correlation-lesson-notes 5/11 1/23/25, 9:02 AM My Path Task | WorldQuant University The Standard Deviation of a portfolio that consists of assets and , with. 4. Correlation as Linear Association There are three ways to measure correlation: Pearson correlation Kendall correlation Spearman correlation https://learn.wqu.edu/my-path/courses/financial-markets/modules/m-3-correlation/tasks/lesson-2-correlation-lesson-notes 6/11 1/23/25, 9:02 AM My Path Task | WorldQuant University 4.1 Pearson Correlation The first formula, Pearson correlation, is the simplest, and it measures the strength of the linear association between two random variables. Its formula is: Where is the correlation coefficient between A and B is the covariance between A and B; is the standard deviation of A; and is the standard deviation of B. Pearson’s coefficient measures the degree of linear association. One way to think of it is to assume you have two variables, X & Y. You graph one versus the other. Then, you fit the best regression line to them. Correlation asks the following question: how tightly wrapped around that line are the data points? Suppose all the points were along the line. Then, you have perfect correlation. If that line is sloping upwards, then you'd have a perfect positive correlation. If the line were downward sloping, then you'd have perfect negative correlation. In both cases, you don't see a cloud; you would just see points on the line. The more the points resemble a cloud that lacks a slope, the closer to 0 that correlation is. In all these cases, correlation is using a line as a reference and measuring the tightness around it. In finance, however, we may not be merely interested in linear relationships. Sometimes, we may be more interested in co-movements, even if they are not linear. There are other ways to think of correlation, the most important being as a measure of the degree of monotonic association. Note that a linear association is just a simple case of a monotonic association; therefore, the next two measures are more general and more powerful than Pearson's correlation. To be able to use Pearson's correlation, we need the observations, i.e., the pairs , to be independent and normally distributed as well. Furthermore, there must be no outliers in the data. The two methods that are presented below do not require normality. As such, we sometimes read that Pearson's correlation is a parametric measure while Spearman's and Kendall's correlations are non- parametric. NOTE (Optional, but worth reading). Why is normality required? This is a question that has been debated in many forums on the internet. It is technical and thus beyond the purpose of this lesson and course. However, it is good to have at least a qualitative idea of why normality is important. We have said that Pearson's correlation coefficient can range from to Well, if the data is bivariate normal, the bounds and are achievable. However, if we assume that the data are bivariate log=normal instead of normal, then the possible range of Pearson's correlation can be shown to be. In another instance where one variable is normal and the other is log-normal, it is possible to show that the range for Pearson's correlation would be about The author of this lesson learned these insights about the achievable range for Pearson's correlation coefficient from a discussion with Karl Ove Hufthammer. https://learn.wqu.edu/my-path/courses/financial-markets/modules/m-3-correlation/tasks/lesson-2-correlation-lesson-notes 7/11 1/23/25, 9:02 AM My Path Task | WorldQuant University 4.2 Spearman Correlation Let's consider a functional relation between X and Y such that, for example, Specifically, let In this case, we compute Pearson's correlation, which turns out to be This is high, but since we have a perfect relationship between and ideally, the correlation should be 1. The Pearson correlation fails to capture that perfect relationship because it focuses on linear association and penalizes the cubic association for being nonlinear. Now, to alleviate this issue, we can employ the Spearman correlation, also known as Spearman's This correlation is more work than Pearson's correlation. Spearman's formula replaces the data points with the ranks of the data points. In other words, imagine if you had 10 data points in each set. You're going to replace the value of with its rank: 1 refers to the largest data point, 2 to the second largest data point, and so on. Similarly, you do the same for replace the largest value of with 1, replace the second largest value of with 2, and so on. If there are ties, then all those ties are assigned the same number. In our case, suppose there are two numbers tied for the largest. Ordinarily, these would have ranks 1 and 2. We average the ranks (1.5) and then assign each of these numbers to 1.5. Now, we have a set of ranks for the values and a set of ranks for the values. Now we apply the Pearson correlation to the ranks of the data. This method is exactly what the Spearman correlation does. For each data point, Spearman computes a value, d, between the 2 ranks. Then, we square and sum this value The Spearman correlation is then ʻ Where is the number of points. Since it is also typical to use the symbol for Pearson's coefficient, we can use for Pearson's correlation coefficient and for Spearman's correlation coefficient. Think of Spearman as the correlation of ranked data. Ranking doesn’t care about linear or non-linear; it simply cares about maintaining the order of the data points. In our previous example, we would find that https://learn.wqu.edu/my-path/courses/financial-markets/modules/m-3-correlation/tasks/lesson-2-correlation-lesson-notes 8/11 1/23/25, 9:02 AM My Path Task | WorldQuant University Then hence, and This is what we were hoping for. In our example, the ranks of all line up with the ranks of. There are no discrepancies in ranks. When we have the nth largest value, we have the nth largest value as well. The advantage of Spearman’s correlation over Pearson’s correlation is that we can simply see if things move together instead of adding linearity to the requirement. Imagine that you are a trader and you want to determine whether one security is likely to go up if another security goes up. Which correlation would be more useful? Well, Pearson’s correlation would help; however, Spearman's correlation focuses entirely on ranks, so it would be a more useful way to measure co-movements. This method of replacing data with ranks is a technique from non-parametric statistics and can be helpful if the data contains outliers or skewness, problems that we discussed in the previous module. What we've learned so far should not make us think that Spearman is the solution to all of our problems. In fact, consider the example immediately below. Example. Consider the following simple dataset: and There is clearly a perfect relationship between and i.e., However, we could show that both Pearson’s and Spearman’s correlations are zero. Why is that? Well, the relationship between and is quadratic and thus not linear, which explains why we get no correlation with Pearson’s formula. It is not in Pearson’s formula’s job description to detect quadratic relationships in general. Why is Spearman failing then? Well, the relationship between X and Y in the range of the values is not monotonic either, and that explains why we get zero using Spearman’s formula as well. Again, it is not Spearman’s formula’s job to detect non-monotonic relationships in general. We just encountered an example where and exhibit a perfect relationship, but according to either correlation formula, no correlation is actually detected. https://learn.wqu.edu/my-path/courses/financial-markets/modules/m-3-correlation/tasks/lesson-2-correlation-lesson-notes 9/11 1/23/25, 9:02 AM My Path Task | WorldQuant University Students can experiment with several functional forms of the relationships between and and check which formula provides the best answer. 4.3 Kendall Correlation This is even more complicated to compute. Kendall's correlation coefficient is usually indicated with the Greek letter We can describe it as follows: Here, the points and are considered as a pair for Compute the ranks of and so that now we have the pairs Organize the points into two columns, the first column for and the second for The data is organized based on the ranks of so that the first column will contain the ranks but the second column will contain the ranks of the point associated with Hence, the second column will not show the ranks as in general. We now calculate the concordant pairs and discordant pairs as follows: for the columns of the ranks, start from the first value and count how many ranks are above the rank of Then, do the same for and so on. This creates a new column of the number of concordant pairs. Once the concordant pairs have been computed for all ranks of we create another column where we count the number of discordant pairs instead, i.e., we start by looking for the number of ranks that are below the rank of then we look for the number of ranks that are below the rank of etc.; At this point, we have two columns and Now let and Finally, Kendall's is given by ʻ One important advantage of the Spearman and Kendall correlations is that they can be used with ordinal data as they rely on ranks. On the other hand, Pearson's correlation requires that the random variables are quantitative (continuous). Example. Assume that we have a universe consisting of 15 stocks, which we indicate simply with A, B, C, D, E, F, G, H, I, J, K, L, M, N, and O. We assume further that we have two analysts who are asked to rank these assets in order of how well they will do from worst to best. Their picks are as follows: while Compute Kendall's for the picks of these two analysts. https://learn.wqu.edu/my-path/courses/financial-markets/modules/m-3-correlation/tasks/lesson-2-correlation-lesson-notes 10/11 1/23/25, 9:02 AM My Path Task | WorldQuant University Answer. Kendall's is computed using the following spreadsheet. The result is 0.8476, which tells us that the opinions of the two analysts have a relatively strong correlation. Given that the values of and are not numbers but letters, we cannot compute Pearson's correlation coefficient in this case. Kendall's is usually preferred to Spearman's because it is more efficient and more robust (in the presence of outliers). It also has better statistical properties. Kendall's and Spearman's do not always give the same value, and Spearman's is usually larger than Kendall's. While both Sperman and Kendall’s correlations are suitable to be used with ordinal data, Kendall’s is more robust in the presence of tied ranks in the sense that it can provide a more accurate measure of correlation in those situations. Since tied ranks tend to occur often with ordinal datasets, Kendall’s tau is preferred. One last word of caution: we have learned to compute 3 different coefficients of correlations and have looked at which one is most suitable in certain instances vs. others. This is all fine if we are working with the entire population of data for and On the other hand, if we are using samples from populations, and like any statistics, are actually random variables and the value we compute alone is not enough. In order to determine if the value computed is significant, we would need to employ formal statistical tests. However, this is beyond the level of this introductory course in Financial Markets. 5. Conclusion In this lesson, we examined the role of correlation, its effect on diversification, and how different values affect overall portfolio standard deviation. We also saw an alternative to this correlation, Spearman correlation, that uses ranks instead of the data to perform correlation. In the next lesson, we’ll turn to a security that has lots of correlation in it: the exchange-traded fund. References Brzenk, Phillip. "Asset Class Correlations Affect Portfolio Volatility and Return." Seeking Alpha, Aug. 2018, https://seekingalpha.com/article/4198887-asset-class-correlations-affect-portfolio-volatility- and-return. https://learn.wqu.edu/my-path/courses/financial-markets/modules/m-3-correlation/tasks/lesson-2-correlation-lesson-notes 11/11