Module 2 - Inference and Hypothesis Testing PDF
Document Details
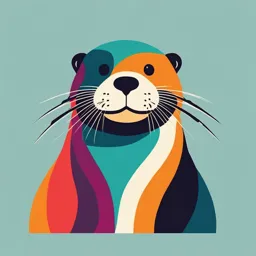
Uploaded by AppreciativeSasquatch
Tags
Summary
This document provides an overview of inference and hypothesis testing, including probability distributions. It covers the application of statistical techniques to data analysis.
Full Transcript
MODULE 2 – INFERENCE AND HYPOTHESIS TESTING Statistical inference: process of drawing conclusions from data. Testing for significant differences, associations or trends in data. o Important to understand the concepts of hypothesis testing, probability, variabili...
MODULE 2 – INFERENCE AND HYPOTHESIS TESTING Statistical inference: process of drawing conclusions from data. Testing for significant differences, associations or trends in data. o Important to understand the concepts of hypothesis testing, probability, variability, significance and confidence intervals. This module: o Define scientific hypotheses o Identify steps associated with testing a scientific hypothesis o Understand the reasoning behind the interpretation of a hypothesis test o Understand the concept of Type I errors and significance. Context o Clean data manage & clean data describe and summarise data (descriptive statistics) inferential statistics and hypothesis testing. Descriptive statistics: graphs, tables and information. Inferential statistics: what can we say about the population based on the data. PROBABILITY Brief history o Probability theory developed with gambling 4-sided die of animal bone dated to >6000 years old. o Two French mathematicians wrote the rules of probability for a gambler who was trying to beat the casino Probability of an event o E.g. rolling a six on a die All probabilities are between 0 and 1. o Probability of 1 = event is certain to occur. (e.g. guaranteed that sometime in the next 100 years I will die) o Probability of 0 = event is certain not to occur (e.g. I will not win the lottery this weekend because I have not bought a ticket) Probabilities are sometimes expressed as: o A percentage (0 to 100%) o As odds (e.g. 10:1) Probabilities can change overtime as more information becomes available. o E.g. the probability of death increases as you get older and you engage in risky behaviour. Known probability o In some circumstances, the probability is not known, but may be estimated based on observed data. Smoking data from age 18-65, what is my probability of dying of lung cancer? What is the probability of a family suffering the death of 2 infants from SIDs? Probability & Perspective o Put yourself in the shoes of someone with high BP. You visit the doctor and they recommend a new drug that will: Lower BP by 10mmHg with 60% probability Have no effect on BP with 30% probability Increase BP by 20mmHg with 10% probability o 3 possible events – BP decreases, increases and is unchanged. o Probability of all events must add to 1 (or 100%) because one of them will occur. Doctor’s viewpoint – population level perspectives o If the doctor prescribes this drug for all their patients with high BP, then the average change in BP will be: o Good choice for doctor on average Average loss of -4mmHg. Patient’s viewpoint o As an individual you are not interested in the doctor’s statistics but rather the likely impact on you as an individual. There is a better than average chance (0.60) that the drug will work for you. But you won’t get 0.60 of the benefit. You will either get all of the benefit (if it works) or none of the benefits (if it doesn’t work) o Some people take more risks than others, o Likely to be a complex personal decision-based on many factors Trust of doctor Previous experience with medication Alternative and impacts – what if you were told you were likely to die in a month if you didn’t lower your BP? PROBABILITY DISTRIBUTIONS Probability distributions assign a probability to each possible event/outcome in a random experiment Applies to categorical & continuous data Can be displayed numerically (table) or graphically. Graphically similar to histogram except vertical axis is probability rather than count/frequency. Normal distributions o Height of adult men in UK Mean = 171.5cm SD = 6.5cm o Area under the normal curve = 1 (if expressed as a proportion) 100% if expressed as a percentage - Should cover all possible values between the minimum and maximum Symmetric Probability Distributions - Can calculate probability of certain events by addition/subtraction - If we know the probability distribution of BMI in a population, then we can calculate: o Proportion of population, with BMI>30 o Proportion of population with BMI is between 20 and 40. o BMI corresponds to the 5th percentiles. Normal distribution - Also called Gaussian distribution o Normal distribution was defined by mean and SD. Minimum value ~ mean – 3SD Maximum value ~ mean + 3SD - Standard normal distribution is a normal distribution with mean=0 and SD=1. - Can convert any normally distributed data into a normal distribution by rescaling. - Increase mean = increased x-axis - Increase standard deviation = flatter (wider range in sample) - Decrease standard deviation = smaller range of samples Normal Distribution Equation - 68% of values are within 1 sd of mean (blue) - 95% of values are within 2 sd of mean (blue+pink) - 99.6% of values are within 3sd of mean (blue+pink+green) - 68% of men are between 165-178cm - 95% of men are between 158.5-184.5cm in height. SAMPLING AND POPULATIONS It is difficult & expensive to collect information on the whole information Samples can be used to gain information about the population o E.g. how type 2 diabetic patients respond to a treatment o Advantages – cheaper, quicker, logistically easier, ethically better (may not always be representative of the population) Statistical Inference: make inferences about populations based on data from the sample. Different samples from the same population will differ (e.g. individuals in the sample differ) Causes summary statistics from different samples to differ o Mean of sample 1 does not equal sample 2 If the samples use the same sampling method, then the differences in statistics should be random. Sampling Variability o Differences in results between samples is called sampling variability or sample error. o If you use a random sampling technique to collect samples Results from each sample are equally valid Results are unbiased, which means they are a good measure of the average. o Still possible to get an odd sample by bad luck Chances decrease with larger sample sizes o Sampling variability is the variation in results we get from different (random) samples of the same population. o What we are interested in its trend or pattern in data. Differences between groups. Differences over time. o Challenge is to identify the trends on a background of sampling variability. Inference o To make inferences about the population you need your sample results to be representative. o Would it be fair to generalize the results for ‘clinical health knowledge’ in this class to: Other students Other students in Australia Other Australian adults o What about if the question related to the amount of rent paid? o Generalisibility depends on the question. SAMPLING VARIABILITY Example – interested in infant birth weight o Record of every birth in a hospital in a year, which is our population (mean weight = 3450g, sd=460g). o Take a sample of the births that occurred during the year and assume they are representative 3 samples of 10 people o Sample of 1 – mean birth weights recorded o Means are different – sampling variability. Means indicated by purple arrows – sample means different but still close to true population mean. o If samples were biased, mean may move left/right. o Underestimated true population mean o Can be higher than true population mean – overestimated. Suppose we collected 100 samples and calculated the mean for each sample o Could draw a histogram of the means o It would look fairly normal, but the shape (& width) would depend on the size of the samples. Sample of size 10 or sample size of 100. - Narrower = more confidence in mean as sample size increases in mean - Less variability in the samples What happens if we keep taking samples of larger size? o For larger sample sizes, the variability decreases o Sample size of 64 (100 samples) – standard deviation = 59 o 100 samples of sample size 8, standard deviation = 152, much larger. Sample Means Sample means fluctuate around the population mean, regardless of sample size. Unbiased because on average, they are close to population mean If each additional sample was random & representative, we expect the fluctuation around population mean to be random & normal But we don't normally take multiple samples so how do we know the size of the sampling variability?? If only 1 sample of population Standard Error Standard error of the mean (se) = standard deviation of the sample means Standard error decreases as sample size increases Precision (or reliability) of sample mean increases as sample size increases o Larger sample size, more confidence in sample mean and how close it is to the population POPULATION + SAMPLE DISTRIBUTIONS Mathematical properties As the sample size increases o Sample mean approaches population mean Sample of 1 million would be close to population mean o Same error of mean decreases Precision/reliability gets better o Histogram of sample means approaches a normal distribution. Central limit theorem o Implication: even if raw data are not normally distributed, means of samples taken from the distribution will be normally distributed. Histogram does not look normal o Heavily skewed If we take several samples and calculate the mean, the distribution of the means will look normally distributed o Even if the original data is not normal. Notation Generalising Results Population characteristics are inferred from a representative sample Can be hard to get representative sample Report your results with caveat (based on a study of 1000 mothers from Brisbane) Describe the characteristics of your sample Let others decide if the results are generalisable to their population (e.g. Mothers in California?? Mothers in Vietnam?) A better-quality study should give more generalisable results Simple random samples are the gold standards Randomness is a great destroyer of bias Convenience sample can be fraught as it may not be representative of the population. HYPOTHESIS TESTING Recap: Normal Distributions Normal distribution is a particular type of ‘bell-shaped’ curve Plays a central role in statistical inference, particularly parametric techniques o If you know the mean and variance you can understand the probability distribution. Central limit theorem implies a very important result: even if the data aren’t normal, if we take lots of samples, the means will be normally distributed. o E.g. missing teeth example from module. Even if the data aren’t normal, a bunch of means form the data will be… - Looks heavily skewed. - But mean will be normally distributed. Normal Distributions More useful to consider sample distribution of means If I took 1000 samples & calculated their means, I would expect 95% of them to fall between 6.2 and 8.4. - There is a 95% chance that the population mean falls between 6.2 & 8.4 SD = 3.1 Sample size = 27 Note: SE is used = 3.7. What if we want to compare groups? - Can you say that on average, healthy individuals and those with heart disease have significant different WBC counts? Subject of hypothesis testing. Hypothesis Testing Robust and scientific approach to uncovering truths Example truths: - A new drug does lower BP - A new drug doesn’t lower BP - The more teenagers have, the more likely they are to experiment with drugs Alternative – less likely to experiment. Disproving hypothesis - Hypothesis: all swans are white - We could: Prove the hypothesis by finding every single swan in the world and checking that they are all white Disprove the hypothesis by finding just one black swan It is easier to find evidence against a hypothesis than to provide to be correct. Scientific Hypotheses - Hypotheses always come as a pair (must cover every possible outcome) - Null Hypothesis (H0): nothing is happening E.g. H0: the new drug doesn’t change blood pressure - Alternative hypothesis (H1/HA): something is happening E.g. H1: the new drug does change blood pressure. One-tailed vs Two-tailed Hypothesis One-tailed hypothesis has a direction o New drug lowers blood pressure o Money increases teenager’s experimentation with drugs Two-tailed hypotheses do not have a direction, just a change (could be positive or negative) o New drug changes blood pressure o Most common because they are the most conservative. One-tailed example o H0: the new drug does not decrease blood pressure. o H1: the new drug decreases blood pressure. Two-tailed example o H0: the new drug does not change blood pressure. o H1: the new drug changes blood pressure. Research Hypotesis & Alternative Hypothesis Generally, alternative hypothesis coincides with what we are trying to demonstate & for this reason is often associated with our research hypothesis Research hypothesis – usually the statement form of our research question. Example: o Does a new drug lower BP? H1: the new drugs lower BP H0: the new drug does not lower BP (increases BP or doesn’t change) HYPOTHESIS TESTING STEPS 1. State null and alternative hypothesis (comes from RQ). 2. Collect data. 3. Summarise data appropriately (summary statistics and graphs) 4. What would data look like if the null hypothesis (H0) was true expected data 5. Calculate discrepancy between groups (or magnitude of association) – test statistic 6. Make decision (reject H0 or don’t reject H0) & make conclusion. Example: Consider 2 suburbs, one with lots of roads and one with lots of parks RQ: are air pollution levels different between the two suburbs We know that air pollutions cause: - Cardio & respiratory deaths & hospitalisations - Exacerbation of asthma - Low birth weight. Step 1: Null Hypothesis Two-tailed hypothesis - H0: there is no difference in the pollution level between the two suburbs But there is a lot of different types of air pollution, so make more specific hypothesis H0: there is no difference in particulate levels between the two suburbs OR H0: there is no difference in sulphur dioxide levels between the two suburbs. Step 2: Collect Data Fixed site particulate monitors for similar times & days? o Similar times – air pollution varys throughout the day and week Randomly placed sites? o Sample from whole suburb – number of instruments across the suburb o Minimise bias – if instruments are close to a road for one suburb and close to the park for another suburb = introducing bias Same techniques? o Same measurement techniques used for the two suburbs. No bias from one particular reading instrument than the other instrument. Step 3: Present Data - Variability is similar - Can present these results numerically and graphically Mean and standard deviation seem similar. Step 4: What would the data look like under H0: If the null hypothesis is true, we would expect no difference in average particulate levels. - Shown by blue dotted line Rare – random variation Suburbs could be no different from each other, but sampling results will see a small difference Typically see something around zero. - Null hypothesis based on how likely the values are. Normal Difference Using the central limit theorem, assume the difference in means for the suburbs is normally distributed – if the null hypothesis is true. o If the sample means are normally distributed – follows logic Difference between means will also be normally distributed around the true population means ‘called the null distribution’ o Normal distribution red line at zero What are the typical values we see about the null hypothesis Indicated by green shaded area (68%). -10 and 10 typical. Less typical indicated by blue shaded area (95%) -20 and 20. Surprising (99.6%). Greater than 20/-20. How wide should the curve be? What's our range of typical differences? Green = skinnier Red = flatter Both could be the null hypothesis and if observed results -17 or -16 would be surprising for the green distribution but less surprising for the red distribution. o Red allows for more variability for -16/-17 compared to green. Step 5: Test Statistic Test statistic (T) is the discrepancy between the data & what is expected under the null hypothesis. How would you calculate the expected & precision part of this equation – depends on the type of data Null hypothesis was no difference in particulate level expected difference = 0. - Observed difference = 297-230=67 (difference between means). - We use standard error as a measure of precision. Calculate an overall estimate of standard error using both samples. STATISTICAL SIGNIFICANCE Example: air pollution - Value of the test statistic determines the p-value and ultimately whether the difference is statistically significant. Given the distribution, how likely is the difference we will expect if the null hypothesis is true? P-values P-values are used to express our belief in the null hypothesis (also known as ‘alpha-level’ P-value: probability of observing the data if the null hypothesis is true - Probability of observing the difference if the null hypothesis is true. Example: - Test statistic = 5.33. P-value