Module 1.pdf
Document Details
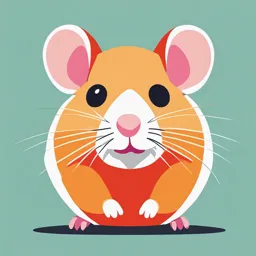
Uploaded by AffordableLimit
Tags
Related
- Virtual TechPathways: Industry-Led Project Placements PDF
- Data Analytics for Decision Making PDF
- Data Analytics (Digital) for Decision Making PDF
- Data Analytics (Digital) for Decision Making - Les Roches PDF
- Topic1_Part3_M9118_Data_Analytics.pdf
- IBPO - Module 8 Future Trends in BPO & Career Opportunities PDF
Full Transcript
Course Introduction: Friday, August 9, 2024 3:15 PM Course Introduction: Welcome to this introductory course on Data Analytics, the first in a series aimed at preparing you for a career as a junior data analyst. The Importance of Data Analytics: According to a Forrester consulting report, da...
Course Introduction: Friday, August 9, 2024 3:15 PM Course Introduction: Welcome to this introductory course on Data Analytics, the first in a series aimed at preparing you for a career as a junior data analyst. The Importance of Data Analytics: According to a Forrester consulting report, data analytics is increasingly recognized as a crucial factor for business competitiveness. Companies are hiring and upskilling employees, expanding teams, and creating centers of excellence to enhance their data and analytics initiatives. This has led to a high demand for skilled data analysts, making it a well-paid and sought-after profession. Career Opportunities: You can choose to master data analytics as a career or use it as a stepping stone to other data-related fields like data science, data engineering, business analytics, and business intelligence analytics. Who This Course is For: This course is suitable for fresh graduates, working professionals considering a mid-career change, data-driven decision-makers, or anyone in an analytics- enabled role. What You Will Learn: The course introduces the core concepts, processes, and tools needed to enter the field of data analytics or strengthen your current role. You will learn about the data ecosystem, data gathering, wrangling, mining, analysis, and data visualization. The course also provides insights into a day in the life of a data analyst, career options, learning paths, and what employers look for in a data analyst. What Lies Ahead: The future is bright for both the field and your career as a data analyst. Congratulations on starting this journey, and good luck! Career in Data Analysis - Mastering data analytics as a career path - Associate Data Analyst - Data Analyst - Senior Data Analyst - Lead Analyst - Principal Analyst - Or branching into other data professions - Data Science - Data Engineering - Business Analytics - Business Intelligence Analytics Module 1 Page 1 Modern Data Ecosystem: Friday, August 9, 2024 3:34 PM Modern Data Ecosystem: The modern data ecosystem is a constantly growing network of data sources, tools, and technologies. Data is created and shared at increasing speeds and comes from a wide range of sources like text, images, videos, social media, IoT devices, and more. The first step is gathering data from these sources into a central storage (data repository), ensuring it's reliable, secure, and in the right format. After collection, data needs to be organized, cleaned, and made accessible while adhering to regulations (like privacy laws) and organizational standards. Business stakeholders, including analysts and applications, access this data for various needs, like creating reports or running data science models. Emerging technologies like cloud computing, machine learning, and big data are essential in this ecosystem, offering powerful tools, limitless storage, and new ways to analyze massive datasets. Module 1 Page 2 Key Players in the Data Ecosystem: Friday, August 9, 2024 9:22 PM Key Players in the Data Ecosystem: Data Engineers: They build and maintain the systems that collect, store, and organize data, making it accessible for analysis. They focus on ensuring data is clean, well-structured, and ready to be used. Data Analysts: They turn data into understandable insights. By cleaning and analyzing data, they find patterns, trends, and correlations that help organizations make informed decisions. They also create charts and dashboards to present their findings. Data Scientists: They build predictive models using past data. These models help forecast future outcomes, like predicting customer behavior or identifying potential risks. They need strong skills in math, statistics, and programming. Business Analysts: They use the insights provided by data analysts and data scientists to make business decisions. They focus on how these insights can impact the business and suggest actions to take. Business Intelligence (BI) Analysts: Similar to business analysts, but with a focus on external factors like market trends. They use data to improve business performance by understanding how external forces affect the business. Summary: Data Engineers prepare and organize data. Data Analysts generate insights from data. Data Scientists predict future outcomes using data. Business Analysts and BI Analysts use these insights to make decisions that help grow the business. These roles work together to help organizations use data effectively. Module 1 Page 3 Defining Data Analysis: Friday, August 9, 2024 9:38 PM Defining Data Analysis: Data Analysis: The process of collecting, cleaning, examining, and interpreting data to find patterns and relationships. This helps businesses understand their past performance and make informed decisions for the future. Four Primary Types of Data Analysis: 1. Descriptive Analytics: Answers "What happened?" by summarizing past data. Example: Tracking past sales or performance. 2. Diagnostic Analytics: Answers "Why did it happen?" by digging deeper into data to find the cause of a particular outcome. Example: Analyzing a sudden increase in sales in a specific region. 3. Predictive Analytics: Answers "What will happen next?" by using historical data to forecast future trends. Example: Predicting future sales based on past data. 4. Prescriptive Analytics: Answers "What should be done?" by analyzing past decisions and outcomes to suggest the best course of action. Example: Self- driving cars making real-time decisions based on environmental data. Key Steps in Data Analysis: 5. Understanding the Problem: Clearly define the problem and the desired outcome before starting the analysis. 6. Setting a Clear Metric: Decide what will be measured and how (e.g., sales in a specific region over a certain period). 7. Gathering Data: Identify the required data, sources, and tools needed for analysis. 8. Cleaning Data: Fix data quality issues like missing values or outliers to ensure accuracy. 9. Analyzing and Mining Data: Examine the cleaned data to find patterns, trends, and correlations. 10. Interpreting Results: Evaluate and understand the results, considering any limitations or potential objections. 11. Presenting Findings: Effectively communicate the analysis through reports, dashboards, charts, etc., to aid decision-making. Module 1 Page 4 Viewpoints: What is Data Analytics?: Friday, August 9, 2024 9:46 PM Viewpoints: What is Data Analytics?: In this video, various data professionals share their perspectives on what data analytics means to them: 1. Storytelling with Data: Data analytics involves collecting and analyzing information to test hypotheses and tell a story. It’s about conveying the state of the world clearly and concisely to others. 2. Everyday Decision-Making: Data analysis is not just a technical term; it’s something we naturally do every day, like deciding what to wear based on the weather. The difference is that now, data analytics is done on a larger scale for more complex decisions. 3. Problem-Solving: Data analytics begins with identifying a problem, forming a hypothesis, and then using data to test it. The process includes collecting, cleaning, analyzing, and presenting data to z 4. Business Insights: For a CPA, data analytics means using financial data to understand a company’s past, present, and future, helping to predict outcomes and make informed decisions. 5. Communication and Decision-Making: Ultimately, data analytics is about sharing your analysis to help others understand what’s happening in the business or data, leading to better decision-making. 6. Targeted Decision-Making: Data analytics can be defined as taking information from a specific population (like customers or social media audiences), breaking it down into subsets, and using it to make decisions about products, services, or content that appeals to the target audience. Module 1 Page 5 Data Analytics vs. Data Analysis: Saturday, August 10, 2024 12:01 AM Data Analytics vs. Data Analysis: Analysis: This involves a detailed examination of elements or structures. It doesn’t always involve numbers or data, and can be applied to various fields like business or psychology. Analytics: This is the systematic computational analysis of data or statistics. It typically involves numerical manipulation and is focused on deriving insights from data. Usage Difference: o Data Analysis: Often refers to examining historical data to understand past performance and generate insights. o Data Analytics: Sometimes used to predict future performance and involves more systematic data processing. Course Approach: In this course, "Data Analysis" and "Data Analytics" are used interchangeably. Both terms are considered to mean the same thing, despite their subtle differences in other contexts. Module 1 Page 6 Summary and Highlights: Saturday, August 10, 2024 12:01 AM Summary and Highlights: Modern Data Ecosystem: A complex network of interconnected elements, including diverse data formats and sources, an enterprise data environment for organizing and cleaning data, and end-users like business stakeholders and analysts who use this data. o Includes a network of interconnected entities. o Involves various data formats, structures, and sources. o Raw data is organized, cleaned, and optimized in an enterprise data environment. o End-users like business stakeholders and analysts utilize this data. Cloud Computing, Machine Learning, and Big Data are reshaping how data is used and understood. Data Engineers, Data Analysts, Data Scientists, Business Analysts, and Business Intelligence Analysts all contribute to deriving insights and business results from data. Types of Data Analysis: o Descriptive Analytics: Answers “What happened?” o Diagnostic Analytics: Explains “Why it happened?” o Predictive Analytics: Forecasts “What will happen next?” o Prescriptive Analytics: Suggests “What should be done next?” Data Analysis Process: o Understand the problem and desired outcome. o Set clear metrics for evaluation. o Gather, clean, analyze, and mine data to interpret results. o Communicate findings to impact decision-making. Module 1 Page 7