MAC210 Note 3-1.docx
Document Details
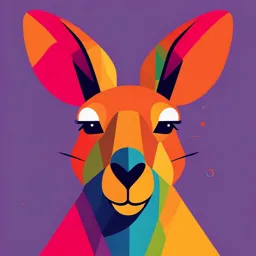
Uploaded by EliteSurrealism
Kwara State University
Tags
Full Transcript
**Population, Sample and Sampling Method** ========================================== **1.1 Population** ------------------ One of the primary objectives in scientific research is to describe the characteristics of a population, which can consist of subjects, variables, concepts, or phenomena with...
**Population, Sample and Sampling Method** ========================================== **1.1 Population** ------------------ One of the primary objectives in scientific research is to describe the characteristics of a population, which can consist of subjects, variables, concepts, or phenomena within a specific category. In some cases, researchers investigate the entirety of this category, which is known as conducting a census. *A population under study refers to the complete set of elements within a particular environment that a researcher intends to examine*. These elements could encompass individuals, objects, plants, artifacts, or events that a researcher consciously chooses to investigate with the goal of gathering the necessary data to address a particular research problem. **1.2 Sample** -------------- In many instances, it is impractical to examine an entire population due to limitations in time and resources. In such cases, researchers typically opt to select a sample from the population. *A sample is a subgroup of the population that accurately represents the entire population*. The key term here is \"representative.\" If a sample fails to represent the population accurately, regardless of its size, it becomes unsuitable for testing purposes because the findings cannot be extended to the population as a whole. When a sample is chosen following appropriate criteria and is representative of the population, the results obtained from a study using that sample can be applied to the broader population. Nevertheless, it\'s essential to exercise caution when generalizing research findings, as all research carries some inherent degree of error. When a sample is drawn from a population, researchers require a method to gauge the extent to which the sample differs from the population. Since a sample does not yield precisely the same data as the entire population, researchers must account for potential errors when interpreting research outcomes. Consequently, research error signifies the extent to which measurements of the sample deviate from those of the entire population under investigation. Probability sampling, a mathematical guideline employed in sample selection, offers researchers the ability to compute sampling error by providing insight into the likelihood of selecting each unit. **1.3 Sampling Techniques** --------------------------- Sampling methods pertain to the approach or system used to select respondents or samples with the aim of gathering information for a specific study. There exist two main categories of sampling: Probability and Nonprobability Sampling. Probability sampling adheres to mathematical principles, whereby the likelihood of selecting each unit is precisely determined. Conversely, nonprobability sampling does not follow the rigorous guidelines of mathematical probability. However, the primary distinguishing feature between these two types of sampling lies in the fact that probability sampling enables researchers to quantify the degree of sampling error inherent in a research study, whereas nonprobability sampling does not provide this advantage. When deciding whether to employ probability or nonprobability sampling, four key factors should be considered: Study Objective: Certain research studies are not devised to generalize findings to the entire population. Instead, they aim to explore relationships between variables or collect preliminary data for designing questionnaires or measurement instruments. In such cases, nonprobability sampling is appropriate. Cost-Benefit Analysis: The chosen sampling method should offer the highest value relative to the investment made. If the cost associated with probability sampling outweighs the quality or type of information collected, or is misaligned with the study\'s purpose, then nonprobability sampling is often a satisfactory alternative. Time Constraints: Researchers engaged in preliminary data collection may often work under time limitations imposed by funding agencies, management directives, or publication requirements. Since probability sampling can be time-consuming, nonprobability sampling may serve as a suitable interim solution. Tolerance for Error: In initial or pilot studies where strict error control is not a primary concern, a nonprobability sample usually suffices. Top of Form Although a nonprobability sample has merit in some cases, it is always best to use a probability sample when a study is conducted to support or refute a significant research question or a hypothesis and the results will be generalized to the population. Probability sampling generally uses some type of systematic selection procedure, such as a table of random numbers, to ensure that each unit has an equal chance of being selected. However, it does not always guarantee a representative sample from the population, even when systematic selection is followed. It is possible to randomly select 50 members of the student body at a university to determine the average number of hours the students spend watching television during a typical week and, by extraordinary coincidence, end up with 50 students who do not own a TV set. Such an event is unlikely, but possible, and this underscores the need to replicate (repeat) any study. ### **1.3.1 Types of Non-Probability Sampling** **Convenience or accidental sampling:** Within mass media research, non-probability sampling methods are frequently employed, particularly in the form of convenience samples. A convenience sample, also known as an available sample, consists of readily accessible subjects, elements, or events for examination. For instance, this could involve a group of students enrolled in an introductory mass media course or shoppers in a mall. While convenience samples can be valuable for gathering preliminary information and occasionally yield useful data, they come with inherent uncertainties. Researchers must carefully weigh the advantages and disadvantages of using convenience samples in their research. In most cases, it is advisable to steer clear of convenience samples due to the potential bias introduced by the proximity of respondents to the research context. However, convenience samples can serve a useful purpose in pretesting questionnaires or conducting preliminary pilot studies. They often assist in identifying and resolving potential issues related to research procedures, testing, and methodology before embarking on the final research study, which should involve a properly selected sample.\" **Unqualified volunteer sample:** This represents another form of nonprobability sampling, where participants willingly choose to take part in a research endeavor without being chosen based on any mathematical principles. While unqualified volunteer samples are unsuitable for scientific research, the media and numerous websites erroneously endorse volunteers by conducting various polls or so-called \'studies\' for radio and television stations, TV networks, the Internet, newspapers, and magazines Purposive Sample -- This is another nonprobability sample which includes respondents, subjects, or elements selected for specific characteristics or qualities and eliminates those who fail to meet these criteria. In other words, the sample is deliberately selected nonrandomly. Purposive samples are used frequently in mass media studies when researchers select respondents who use a specific medium and are asked specific questions about that medium. A purposive sample is chosen with the knowledge that it is not representative of the general population. **The quota sample** -- In this method, subjects are selected to meet a predetermined or known percentage. For example, a researcher interested in finding out how DVD owners differ from non-DVD owners in their use of television may know that 40% of a population owns a DVD. The sample the researcher selects, therefore, would be composed of 40% DVD owners and 60% non-DVD owners (to reflect the population characteristics). Quota sample guarantees the inclusion in the sample of diverse elements of the population and ensures that the diverse elements are taken note of in the proportions in which they occur in the population. **Snow-ball Sampling** - The term snowball sampling is used most often in academic research. In private sector research, this approach is known as referrals.) In either case, the method is the same. A researcher (or research company or field service) randomly contacts a few qualified respondents and then asks these people for the names of friends, relatives, or acquaintances they know who may also qualify for the research study. These referrals are then contacted to determine if they qualify for the research. While this sampling procedure sounds legitimate, researchers don't use it for legitimate research because the sample may be completely biased. A researcher may find that the sample consists only of respondents from a club, organization, or group. ### **1.3.2 Types of Probability Sample** **Simple random sample**: The most basic type of probability sampling is the simple random sample, where each subject, element, event, or unit in the population has an equal chance of being selected. If a subject or unit is drawn from the population and removed from subsequent selections, the procedure is known as *random sampling without replacement*---the most widely used random sampling method. *Simple random sampling with replacement* involves returning the subject, element, or unit to the population so that it has a chance of being chosen another time. Sampling with replacement is often used in more complicated research studies such as nationwide surveys. Researchers often use a table of random numbers to generate a simple random sample. For example, a researcher who wants to analyse 10 primetime television programs out of a population of 100 programs to determine how the medium portrays elderly people can take a random sample from the 100 programs by numbering each show from 00 to 99 and then selecting 10 numbers from a table of random numbers. First, a starting point in the table is selected at random. There is no specific way to choose a starting point; it is an arbitrary decision. The researcher then selects the remaining 9 numbers by going up, down, left, or right on the table---or even randomly throughout the table. Simple random samples for use in tele-phone surveys are often obtained by a process called random digit dialing, or RDD. One RDD method involves randomly selecting four-digit numbers (usually generated by a computer or through the use of a random-numbers table) and adding them to the three-digit exchange prefixes in the city in which the survey is conducted. A single four-digit series may be used once, or it may be added to all the prefixes. A problem with this digit dialing is that some line may have been disconnected but a way to curb this is to select triple of the sample size to cover for that problem One can also add one to three random digits to the phone numbers selected or conduct random selection using household addresses. There are several methods to develop random numbers or households, but irrespective of the methods adopted, two rules always apply: (1) each unit or subject in the population must have an equal chance of being selected, and (2) the selection procedure must be free from subjective intervention by the researcher. The purpose of random sampling is to reduce sampling error; violating random sampling rules only increases the chance of introducing such error into a study. **Advantages of Simple Random Sampling** - Detailed knowledge of the population is not required. - External validity may be statistically inferred. - A representative group is easily obtainable. - The possibility of classification error is eliminated. **Disadvantages** - A list of the population must be compiled. - A representative sample may not result in all cases. - The procedure can be more expensive than other methods. **Systematic random sampling:** This is similar to simple random sampling in which every nth subject, unit, or element is selected from a population. For example, to obtain a sample of 20 from a population of 100, or a sampling rate of 1/5, a researcher randomly selects a starting point and a sampling interval. Thus, if the number 11 is chosen as the starting point, the sample will include the 20 subjects or items numbered 11, 16, 21, 26, and so on. To add further randomness to the process, the researcher may randomly select both the starting point and the sampling interval. For example, an interval of 11 with a starting point of 29 generates the numbers 40, 51, 62, 73, and so on. Systematic samples are used frequently in mass media research. They often save time, re-sources, and effort when compared to simple random samples. In fact, since the procedure so closely resembles a simple random sample, many researchers consider systematic sampling as effective as the simple random procedure. The method is widely used to select subjects from lists such as telephone directories or directories of organizations or groups. The researcher must have a comprehensive list that includes all the elements in the population (sampling frame) and where such list is unavailable, it would not be advisable to use a systematic sampling technique. Assuming you want a sample size of 20 out of a population of 100 elements, this technique involves the following steps: Step 1 -- Number the subjects serially up to 100 Step 2 -- divide the population by the sample size, that is N/n = 100/20 = 5 Step 3 -- randomly select a starting point say 10 Step 4 -- select every 10^th^ subject after the first. **Advantages of Systematic Random Sample** - Selection is easy. - Selection can be more accurate than in a simple random sample. - The procedure is generally inexpensive. **Disadvantages** - A complete list of the population must be obtained. - Periodicity (arrangement or order of list) may bias the process. **Stratified Sample** -- A stratified sample is the approach used to get adequate representation of a subsample. The characteristics of the sub-sample (strata or segment) may include almost any variable: age, gender, religion, income level, or even individuals who listen to specific radio stations or read certain magazines. Stratified sampling ensures that a sample is drawn from a homogeneous subset of the population---that is, from a population that has similar characteristics. Homogeneity helps researchers to reduce sampling error. For example, consider a research study on subjects' attitudes toward two-way, inter-active cable or satellite television. The investigator, knowing that cable and satellite subscribers tend to have higher achievement levels, may wish to stratify the population according to education. Before randomly selecting subjects, the researcher divides the population into three education levels: grade school, high school, and college. Then, if it is determined that 10% of the population completed college, a random sample proportional to the population should contain 10% of the population who meet this standard. As Babbie (2010) notes: Stratified sampling ensures the proper representation of the stratification variables to enhance representation of other variables related to them. Taken as a whole, then, a stratified sample is likely to be more representative on a number of variables than a simple random sample. Stratified sampling can be applied in two different ways. *Proportionate stratified sampling* includes strata with sizes based on their proportions in the population. If 30% of the population is adults ages 18--24, then 30% of the total sample will be subjects in this age group. This procedure is designed to give each person in the population an equal chance of being selected. *Disproportionate stratified* sampling is used to oversample or over represent a particular stratum. The approach is used because that stratum is considered important for marketing, advertising, or other similar reason. For example, a radio station that targets 25 to 54-year olds may have ratings problems with the 25- to 34-year-old group. In a telephone study of 400 respondents, the station management may wish to have the sample represented as follows: 70% in the 25--34 group, 20% in the 35--49 group, and 10% in the 50--54 group. This distribution would allow researchers to break the 25-34 group into smaller subgroups such as males, females, fans of specific stations, and other subcategories, and still have reasonable sample sizes. **Advantages of Stratified Sampling** - Representativeness of relevant variables is ensured. - Comparisons can be made to other populations. - Selection is made from a homogeneous group. - Sampling error is reduced. **Disadvantages** - Knowledge of the population prior to selection is required. - The procedure can be costly and time-consuming. - It can be difficult to find a sample if incidence is low. - Variables that define strata may not be relevant. **Cluster Sampling**: This involves selecting the sample in groups or categories. For example, analysing magazine readership habits of people in Kwara State would be time-consuming and complicated if individual subjects were randomly selected. With cluster sampling, the state can be divided into zones/local governments, communities/ wards districts, areas/streets and groups of people can be selected from each area. Cluster sampling creates two types of errors: errors in defining the initial clusters and errors in selecting from the clusters. For ex-ample, a zip code area may contain mostly residents of a low socioeconomic status who are unrepresentative of the rest of the state; if selected for analysis, such a group may create problems with the results. To help control such error, most researchers suggest using small areas or clusters, both to decrease the number of elements in each cluster and to maximize the number of clusters selected. **Advantages of Cluster Sampling** - Only part of the population need be enumerated. - Costs are reduced if clusters are well defined. - Estimates of cluster parameters are made and compared to the population. **Disadvantages** - Sampling errors are likely. - Clusters may not be representative of the population. - Each subject or unit must be assigned to a specific cluster. An important sampling technique that is closely associated with the cluster sampling is the **[multi stage sampling]**. It involves selecting samples in stages by narrowing cluster through random selection of districts/zones and areas within the principal cluster. The researcher can adopt any of the selection techniques in the stages to select the actual sample. **Resources:** -------------- Onwubere, C. H., Wilson, D & Esiri, M. (2018). Communication Research. Abuja: NOUN; Wimmer, R. D. and Dominick (2011) Mass Media Research: An Introduction Boston: Wadsworth