Test Norms and Related Concepts PDF
Document Details
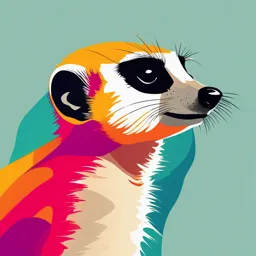
Uploaded by SimplifiedNewton
Tags
Summary
This document covers test norms and standardization procedures, emphasizing the importance of representative samples and cultural sensitivity in interpreting test results. It explores the concept of a normative sample and describes various types of norms like national and specific norms. The lesson also discusses issues related to applying norms to different populations and the limitations of using previously established norms with new populations.
Full Transcript
Psychological Testing and Measurement (PSY-P631) VU Lesson 08 Test Norms and Related Concepts Standardizatio...
Psychological Testing and Measurement (PSY-P631) VU Lesson 08 Test Norms and Related Concepts Standardization: Norms are established for the sake of standardization of any test. Standardization is the process whereby a test is administered to a representative sample of population whom the test is meant for, for the sake of establishing norms. A standardized test is the one that has normative data, as well as clearly specified administration and scoring procedures. The Normative Sample: When we are using test scores for any purposes, or interpreting them and making judgment about the test taker, we should keep in mind, that norms that are being referred to be representative of a particular population from which the standardization or normative sample was selected. The mean scores of that sample are assumed to represent the parent population. Therefore if the sample comprised women alone, than their mean performance score should be used as a norm for women’s raw scores alone. And if women from any specific cultural/regional background alone were used for norm development, then one should be very careful in interpreting the scores of women belonging to completely different cultures. It is therefore advisable that the standardization sample should include maximum characteristics of the population. Also the sample should be of a large enough size to ensure stable values. However the standardization sample can be as small as one person (Cohen, & Swerdlik, 19) depending upon the population of interest. Nevertheless the significance of the size of sample cannot be ignored. As the size of sample increases, the chance of making error reduces. This is because when the sample is small and does not include all characteristics of the population of interest, then many possible sources of error may become intervening variables. Therefore the size of the sample should be good enough to generate a meaningful distribution of raw scores. Also it should not be ignored that over inclusion or under inclusion may take place. Therefore great care is required in sample selection. In order to obtain a true representative sample, careful sampling procedures need to be followed. Most populations comprise sub groups or strata. The sample therefore should include members from all strata. Proportionate stratified random sampling is the best approach for selecting a representative sample. In this type of sampling members from each subgroup/stratum are included in the sample in the same proportion in which they are found in population. The characteristics of the sample will indicate the type of population to which the results can be generalized. Ideally speaking, we should decide and define our population in first place. Then as a second step we can select the representative sample. But practically speaking this can be somewhat difficult. Identifying all possible characteristics from the population and then selecting a sample with all those qualities can be difficult. The second, more practical, option can be that we take a purposive sample that we believe contains all characteristics that we are interested in catering for. Norms may be developed for that sample, and the population can be defined accordingly. For example if the standardization sample included children aged 12 to 16 years, with six years of schooling, then the norms will be meant for a population of children within the same age range and similar educational background. There is no test that provides norms for all sorts of population altogether. “No test provides norms for the human species”! (Anastasi, & Swerdlik). We have a common tendency to use tests developed for, and standardized in, western countries with our local population. We should be cautious while interpreting the results of such tests and making judgments about personality or ability of local tests takers because the available norms were not established for either population of their origin, or even for a very similar population. Another point that needs to be considered while interpreting scores with reference to the norms is that whether any such specific conditions prevailed at the time of norm development that could have affected the performance or scores of the members of normative sample. These could be any special societal conditions or any special selective variables (Anastasi, 1985). National Norms: If a test is standardized using a nationally representative sample of the population, than it would be called a “national sample”. The sample containing all characteristics of interest is chosen from different geographical region/location, communities, socioeconomic status, institutions etc. For example, if we were to establish norms for a test meant for measuring achievement of university students in Pakistan, then we will have to select a normative sample that represents university students from all region of the country. National Anchor Norms: ©copyright Virtual University of Pakistan Psychological Testing and Measurement (PSY-P631) VU We have a variety of tests that measure the same ability or human trait. People who are tested on the same ability through different tests, many obtain different scores on all of these tests. The psychologist, or professional who is going to interpret the scores will need information regarding the equivalence of these scores i.e., how do we compare and interpret a score of 25 on test ABC, and a score of 34 on test XYZ of verbal ability? National anchor norms provide solution to this problem. These norms provide equivalency table for scores on the various tests of the same ability. Equivalency of scores on various tests is calculated using the ‘equipercentile method’. Test scores are compared and their equivalence is determined with reference to their corresponding percentile scores. A score on two tests will be considered to be equal only when they have equal percentiles in the group being studied. Therefore a score of 34 on test ABC carries 85th percentile, and so does the same score in test XYZ then they are equivalent. But if a score of 35 on ABC had 85th percentile, and a score of 29 on XYZ had the same percentile, then 35 on ABC will be equivalent to 29 on XYZ. National anchor norms are very helpful is assessing the equivalence of scores, but they should not be used as a single and fully dependable source of judgment. The difficulty level, detailed contents, and the sample from which scores have been obtained is very important. It is a prerequisite that every member of the sample should have taken all the tests whose equivalence is being determined. Tests should be interchangeable in the true sense before they are described as equated or fully equivalent. Specific Norms: As discussed earlier, national anchor norms do provide information regarding equivalence of test scores, but relying completely on these may be problematic. An alternate solution to the problem of non- equivalence of tests and their comparability is to use specific norms. This solution requires that tests should be standardized on more narrowly defined population. It should be chosen in a manner that it suits to the specific purposes of each test. Rather than using broadly defined samples from broadly defined populations, tests can be standardized on narrowly defined samples. Normative samples may be selected on the basis of purposive sampling, including precisely that type of subjects that fit into the purpose for which the test is making measurements. Such samples are chosen on the basic of specific purpose of a test or subtests. In case of using specific samples, the chances of controlling nonequivalence are reduced. However when the norms for such tests are reported, a clear report of the limits of the normative sample has also to be made. Additionally the use of such tests should be avoided with samples chosen from populations that are beyond the limits of a specific normative sample. Highly specific norms are considered to be useful for most testing purposes. Even when representative norms from broadly defined populations are available, availability of separately reported ‘sub group’ norms is considered very helpful (Anastasi & Swerdlik). If a large group, population of interest, includes distinguishable subgroups then it is better to have overall groups’ norms as well as specific norms. For example, medical profession comprises a large community of doctors. Within this broadly defined population, it is believed that doctors working in different wards or areas of specialization undergo different types and levels of stress and public dealing. It is believed that the experiences of doctors working with dying patients, burn victims, and newborns in an obstetrics nursery are entirely different. Therefore whereas we can have one single inventory to measure occupational and personality variables in doctors as one community, we may also develop separate subscales or other measures to assess variables of interest in doctors working under different levels of stress and in different working conditions. At times norms may be even more narrowly defined than specific norms. There are occasions when institutions or organization prefer to develop their own norms. These norms, developed by test takers themselves are called local norms. For example a university may decide to develop norms on its students; norms may be accumulated for students entering the first year and then these may be used to predict achievement in following years. An organization may establish norms for selectees or new recruits and on the basis of it their future performance may be predicted. For this purpose data regarding performance and progress will also be gathered. Fixed Reference Group: Although conventionally developed norms, whichever type, give a good reference for interpretation and comparability of test scores, there are other approaches to interpretation as well. At times non normative scales are used. In one such type a fixed reference group is used. This is called the ‘fixed reference group ©copyright Virtual University of Pakistan Psychological Testing and Measurement (PSY-P631) VU scoring system’. In this system the distribution of scores obtained on the test from one group of people who took the test is used as the basis for the calculation of test for future administration of the test. The group from which the scores were obtained is called the ‘fixed reference group’. This system does not provide normative evaluation of performance, but ensures comparability and continuity of scores. The College Board Scholastic Aptitude Test or SAT is an example of this system. The test was later on renamed as Scholastic Assessment Test (SAT). The first administration of SAT took place in 1926. At that time its norms were based on the mean and standard deviation of people who took the test. Till 1941 SAT scores were expressed on a normative scale in terms of mean and standard deviation of test takers at every administration. With the passage of time more and more colleges became members of the College Board. The variety of colleges also expanded. It was felt that there was a need to bring changes into the normative scale because of two reasons: a) The element of scale continuity needed to be maintained. Failing this the test taker’s scores would depend on the characteristics of the group tested during a particular year. b) It was observed that there was variation in students’ scores in tests taken at different times during the year. Students performed less well at certain times of the year than those who took SAT at other times. It was concluded that this was a function of the time of year when test was administered. It was speculated that different factors operated at different times when the test was administered. The system was therefore changed in 1941. In the same year, approximately 11,000 candidates had taken the test. The distribution of scores of this sample was taken as a standard, and all SAT scores were expressed in terms of mean and standard deviation of these candidates. This standard was used for future conversion of raw scores. For subsequent forms of the test, these 11,000 candidates constituted the fixed reference group. A score of 500 corresponded to the mean of this group; 600 meant one SD above mean, and 400 was one SD below. In each form of the SAT, a short anchor test (set of common items) was included in order to allow translation of raw scores on any form of the SAT into these fixed reference scores. A chain of items extending back to the 1941 form was developed. This happened as each new form was linked to one or two earlier forms which in turn were linked with other forms, thus ending into a chain. These non- normative scores could then be interpreted through comparison with any appropriate distribution of scores e.g., a particular college, a type of college, region etc. In 1995, a new fixed reference group began to be used. This one comprises those more than a million (2 million,according to Cohen) who took the SAT in 1990. After April 1, 1995, the scores of SAT takers are reported on the “recentered” scale derived from the 1990 reference group. In order to assist test users in converting individual and aggregate scores from the former scale and vice versa, interpretive aids and materials have been developed. Item Response Theory: Item response theory can be understood in terms of the ‘latent trait models’. Beginning from the 1970’s, psychologists have been increasingly interested in a class of mathematically sophisticated procedures for scaling the difficulty of test items. The availability of high speed computers made such procedures possible. The general title of ‘latent trait models’ was used for these approaches. The basic measure used by these is the probability that a test taker with a talent trait (or a specific ability) succeeds on an item of specified difficulty. There is no implication regarding the existence of the trait as such. The latent traits are mathematically derived statistical constructs. These are derived from empirically observed relations among test responses. The total score that a test taker obtains on the test is a rough, initial estimate of their latent trait. The term latent trait model was later replaced by Item Response Theory (IRT) because ‘latent trait’ created a false impression of a specific trait. The purpose of IRT models is to establish a “sample-free” scale of measurement that is uniform, is applicable to individuals and groups having widely varying ability levels, and to test contents that vary widely in terms of difficulty levels. Rather than using the mean and standard deviation of a specific reference group to define the origin and the unit size of the scale, IRT models set origin and unit size in terms of data representing a wide range of ability and item difficulty. This may be obtained from many samples rather than a single sample. ©copyright Virtual University of Pakistan