Quantitative Research Lecture 8 (1) PDF
Document Details
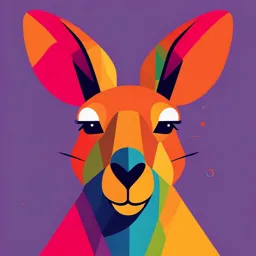
Uploaded by TalentedRealism
Tags
Summary
This document is a lecture on quantitative research methods, specifically focusing on T-tests and ANOVA. It explains their uses and different types, such as One Sample T-Test, Paired Samples T-Test, and Independent Samples T-Test. The lecture also discusses the assumptions and procedures for using these tests. It also touches on visualizations such as box plots.
Full Transcript
Quantitative Research T-Tests and ANOVA: - Significance testing of 2 sample means - Significance testing of 2 or more sample means What does the T-test do? - The t-test is like a “truth checker” that helps us see if the difference in test scores is big enough to believe that working ou...
Quantitative Research T-Tests and ANOVA: - Significance testing of 2 sample means - Significance testing of 2 or more sample means What does the T-test do? - The t-test is like a “truth checker” that helps us see if the difference in test scores is big enough to believe that working out practical exercises actually works better than just reading - If the difference is big enough, the t-test lets us say; Yes, working out practical examples really makes a difference - If the difference is small, we might conclude that it is probably just a coincidence - T-tests help us figure out if something like a new way of learning, or anything else we’re testing actually works or if the difference we see might just be random T-Tests: - A t test is used to compare the means of two groups - It is a hypothesis-testing procedure in which the population variance is unknown; It compares t scores from a sample to a comparison distribution called a t distribution - It determines whether a treatment, process or intervention has an effect on the population of interest, or whether two groups differ from one another Your 5-a-day: - T-tests helps you figure out if the differences between two groups are real or if they could have happened by chance - Everyone should have at least 5 portions of a variety of fruit and vegetables every day Examples for the Different Types of T-Tests: - One Sample T-Test; Determines whether the sample mean is statistically different from a known or hypothesized population mean E.g. sample mean = 4 portions vs. population mean = 3.5 portions - Paired Samples T-Test; Compares the means between two related groups on the same continuous, dependent variable E.g. number of portions before and after a 5-a-day health awareness training - Independent Samples T-Test; Compares the means between two unrelated groups on the same continuous, dependent variable E.g. Who is more likely to have at least 5 portions of fruit or veg per day? Men or women? Older or younger? B. Psy (Hons) or B.A. students? Those who use a reminder app, or those who are told the benefits of the 5 a day? What does the T-Test do? - The t-test helps you figure out if the difference in portions of fruit and veg per day between the two groups is big enough to be meaningful or if it could have happened just by random luck - It is a tool that measures how “spread out” the fruit and veg eating numbers are in each group If the numbers are all close together, the tool will show a small number If the numbers are all over the place, the tool will show a bigger number - The t-test looks at these numbers from both groups and compares them. If the numbers are very different, it tells you there's a good chance the two groups really do eat different amounts of fruit and veg But if the numbers are pretty similar, it suggests that the difference might just be due to random chance Types of T-Test: Examples for the Different Types of T-Tests: - One Sample T-Test; Determines whether the sample mean is statistically different from a known or hypothesized population mean E.g. Hours spent daily on TikTok by teenagers – sample mean = 2.5 hrs vs. population mean =2.9 hrs - Paired Samples T-Test; Compares the means between two related groups on the same continuous, dependent variable E.g. mood before and after using social media in the same group of people - Independent Samples T-Test; Compares the means between two unrelated groups on the same continuous, dependent variable E.g. Who is more likely to report symptoms of anxiety? Men or women? Independent Samples T-Test: Who is More Likely to Report Symptoms of Anxiety? Men or Women? - Dependent Variable; Score on a scale measuring anxiety symptoms (e.g. Hamilton Anxiety Rating Scale - HAM-A) Continuous Variable; 14 items, each scored on a scale of 0 (not present) to 4 (severe), with a total score range of 0–56, where Explore - Click Plots > Deselect Stem and Leaf > Select Normality plots with tests > Click Continue. In the main window Click OK Learning How to Read the Output: The Box Plot: - A boxplot is a standardized way of displaying the distribution of data based on a five number summary: “minimum”, first quartile (Q1), median, third quartile (Q3), and “maximum” It can show you outliers. It can also tell you if your data is symmetrical, how tightly your data is grouped, and if and how your data is skewed - Boxplots are drawn as a box with a vertical line down the middle, and has horizontal lines attached to each side (known as “whiskers”) The box is used to represent the interquartile range (IQR) — or the 50 percent of data points lying above the first quartile and below the third quartile — in the given data set The whiskers are used to represent the variability of the minimum, maximum and any outlier data points in comparison to the IQR (the longer the whisker, the wider the variability of the attached data points to the IQR) Box Plot on a Normal Distribution: Outliers: - Any data points that are more than 1.5 box lengths from the edge of their box are classified by SPSS as outliers and are illustrated as circular dots - Any data points that are more than 3 box lengths away from the edge of their box are classified as extreme points (extreme outliers) and are illustrated with an asterisk (*) Is the Data Normally Distributed? - Use the Shapiro Wilks test It should be NOT statistically significant for both groups - This is only recommended for small sample sizes (n < 50), while for larger groups it is best to use the Q-Q Plots - If two groups are approximately the same size, we can get away with violation of normality - Alternatively, we would need to use the Mann Whitney U (non- parametric) B. Run the Independent Samples T-Test Procedure: 1. Analyse > Compare Means and Proportions > Independent Samples T Test 2. In the dialogue box, move the dependent variable to ‘Test Variables’ field and the independent variable to ‘Grouping Variable’ field 3. Click ‘Define Groups’ button. Enter values as labelled in your data 4. Click ‘Continue’ > return to the main dialogue box 5. Click ‘Options’ > leave everything as is. Return to main dialogue box 6. Click OK > generate output Group Statistics Table: - There were 30 men and 30 women in the sample Higher levels of anxiety were reported by female participants (M = 30.70, SD = 17.7) in comparison to male participants (M = 28.90, SD = 18.37) Independent Samples Test Table: - Look at the Levene’s Test for Equality of Variances’ columns It must NOT be statistically significant - If the Levene’s p-value > 0.05, the two standard deviations are similar and we quote the first p-value of the t-test (equal variances assumed) - If the Levene’s p-value < 0.05, the standard deviations differ considerably and we quote the p-value from the second row (equal variances are not assumed) Reporting the T-Test: - To know if the T Test is statistically significant, check Sig (2 tailed) column - Because our groups are equal, go with upper row p > 0.05 - NB. If you see SPSS Statistics state that the " Sig. (2 tailed) value is ".000", this means that p <.0005; it does not mean that the significance level is actually zero - Report t coefficient, degrees of freedom, and statistical significance of the test - There was no statistically significant difference in mean Anxiety score between males and females, t (58) = -0.386, p = 0.701 Effect Size: - How important is your result? - Cohen’s d = divides the mean difference between the groups by the pooled standard deviation For Cohen’s d 0.2 = small 0.5 = medium 0.8 = large Mann Whitney U Test: When to Use? - The Mann Whitney U test is a rank based nonparametric test that can be used to determine if there are differences between two groups on a continuous or ordinal dependent variable - Assumptions; 1. One dependent variable (continuous or ordinal) 2. One independent variable (categorical, dichotomous) 3. Independence of observations 4. Distribution of scores for both groups of independent variable either have the same OR different shapes How does it Work? - Mann-Whitney U test is used to interpret whether there are differences in the “distributions” of two groups OR differences in the “medians” of two groups - Based on whether the distribution of scores for both groups of the independent variable have the same shape or a different shape - If the two distributions have a different shape, the Mann-Whitney U test is used to determine whether there are differences in the distributions of the two groups - If the two distributions are the same shape, the Mann-Whitney U test is used to determine whether there are differences in the medians of the two groups Mann Whitney U Procedure in SPSS: - Analyse > Nonparametric Test > Independent Samples - Keep ‘Objectives’ tab as is - In ‘Fields’ > place your dependent variable in ‘Test Fields’; place your - independent variable in ‘’Groups’ Click ‘Settings’ > Choose ‘Test Options’ - In ‘Test Options’, if you’re running one test only, leave everything as is - ‘Click ‘Run’ to generate the output Calculate the Medians: - Analyse > Compare Means > Means - Place dependent and independent variables into their respective fields - Click Options - Move ‘Median’ to ‘Cell Statistics’ - Continue > OK Interpreting the Output: - Depends on the (dis)similarity of the shapes of distributions - If they are similar, you can compare medians - If not, you can only say whether one group's values were higher or lower than the others Comparing mean ranks - Determine by visual inspection Interpret and Report the Mann-Whitney U: - Report U coefficient and p value and if possible, medians; - “A Mann-Whitney U test was run to determine if there were differences in engagement score between males and females. Distributions of the engagement scores for males and females were similar, as assessed by visual inspection. Engagement score was not statistically significantly different between males (Mdn = 5.58) and females Mdn = 5.38), U = 145, z = 1.488, p =.142.” - “A Mann-Whitney U test was run to determine if there were differences in engagement score between males and females. Distributions of the engagement scores for males and females were not similar, as assessed by visual inspection. Engagement scores for males (mean rank = 23.25) and females (mean rank = 17.75) were not statistically significantly different, U = 145, z = -1.488, p =.137” Two Independent Groups: - T-Test; Independent Samples T Test; it compares the means between two unrelated groups on the same continuous, dependent variable Independent variable = dichotomous Dependent variable = continuous Approximately normally distributed - Mann-Whitney U; This is a rank-based nonparametric test that can be used to determine if there are differences between two groups Independent variable = dichotomous Dependent variable = continuous or ordinal Paired Samples T-Test: Dependent Samples T-Test: - Used when conducting a study with one group of participants who are measured twice at two points in time - To identify whether the mean values of the two dependent samples differ significantly from each other - Measurement is in pairs; e.g., measuring the same person twice - Null hypothesis; there is no significant different between the means of the two dependent groups - Alternative hypothesis; there is a significant difference between the means of the two dependent groups Assumptions of the Dependent Samples T-Test: - Samples must be dependent - Metric scales: to calculate the mean value of the samples - Differences of the paired variables must be normally distributed * - *If the variables are not metric and the data is not normally distributed, the Wilcoxon test should be used. It is the non-parametric counterpart of the dependent samples t-test Single Samples T-Test: - For related samples e.g. before and after, we use the single-samples t- test and use as subject scores the computed differences between the two measures - We compute a new Difference (D) variable and compute the t-test on the sample of different scores - It is the differences that are sampled, not the individual scores before and after ANOVA: Introduction to ANOVA: - As with other experiments, the purpose is to demonstrate a relationship between an independent variable and a dependent variable - The only difference is that we now have 3 (or more) samples = 3 different distributions = 3 different means - We face the usual problem; the differences in means may be due to sampling error - This needs to be eliminated before we conclude that differences are statistically significant Assumptions of ANOVA: 1. One dependent variable. Measured at the continuous level 2. One independent variable that consists of two or more categorical, independent groups. N.B. You won’t be able to tell which groups differ without follow up tests 3. Independence of observations 4. There should be no significant outliers 5. Your dependent variable should be approximately normally distributed for each group of the independent variable 6. Homogeneity of variance - ANOVA is robust and works well even if certain assumptions are effectively violated Analysis of Variance: - A collection of statistical models and their associated estimation procedures used to analyse the differences among group means - Developed by Ronald Fisher - Uses an F statistic (a ratio of two variances) The F-test statistic is compared (or use a computer) with the F- distribution, using the Ftable - The F statistic simultaneously compares all sample means to determine whether 2 or more differ significantly - It uses variance (SD2) to represent mean differences The F-Test: - When the F statistic is not significant (i.e. p-values), our different sample means do not represent statistically significant differences in the population - When the F statistic is significant, it indicates that somewhere amongst our groups is a statistically significant difference such that at least two groups in the population are likely to have different means - Further post-hoc tests (e.g. t-tests) may be required to determine the precise location of differences - The F statistic takes into account 2 potential sources of variance Scores may differ from each other within the same condition (e.g. not all Group A will have the same happiness) Scores may differ from each other between conditions (e.g. Group A are more likely to be happier - Variance; within groups & between groups - Estimates for each are computed in computing the F statistic; termed mean square (MS) - The F statistic is computed by dividing the MSbn / MSwn One-Way ANOVA: - Is there a difference in exam performance based on test anxiety levels amongst students? - Independent Variable; Test Anxiety Level - Dependent Variable; Exam Performance The Null and Alternative Hypotheses: - H0; all group population means are equal (i.e., µ1= µ2= µ3=... = µk) - (µ = population mean and k= number of groups) - HA; at least one group population mean is different (i.e., they are not all equal) - It is important to note that you are not prescribing a particular way in which the groups mean differ in the population, only that they do differ in some way Procedure: 1. Run the Explore procedure (testing assumptions #4 and #5) 2. Analyse boxplots and make decisions about outliers (if any) 3. Check Shapiro-Wilks test results (normality) and make decisions. If normality is violated, you can either do data transformation or use Kruskal-Wallis H test 4. Run the One-Way ANOVA procedure (with Levene’s test to check assumption #6) 5. Follow-up the One-Way ANOVA with post hoc tests 6. Calculate effect size ANOVA in SPSS: - Two procedures can be used; ONEWAY or GLM - ONEWAY includes Levene’s test in the output but doesn’t allow to calculate effect size - GLM is used to be able to calculate the effect size a. Explore b. One-Way c. GLM a. Explore: - Analyse > Descriptive Statistics > Explore - Click Plots > Deselect Stem and Leaf > Select Normality plots with tests > Click Continue. In the main window Click OK b. One-Way: - Analyse > Compare Means > One-Way ANOVA - In Options, choose ‘Descriptive’, ‘Homogeneity of Variance test’ and ‘Welch’ + check the “Means plot” box - Click the “Post Hoc” button. Choose ‘Tukey’ in the “Equal Variances Assumed” part and ‘Games-Howell’ in “Equal Variances Not Assumed” part c. GLM: - Analyse > General Linear Model > Univariate - Move dependent and independent variables to relevant fields - In ‘Options’ check “Estimate of effect sizes” box - Continue > OK Interpreting the Output: - First, look at the ‘Descriptives’ table and consult the ‘Means Plot’ - Look at the ‘Test of Homogeneity of Variances’ table - NB: if you do not have homogeneity pf variances, interpret the results of the Welch ANOVA and use Games Howell post hoc test - Check ‘Sig.’ level in ANOVA table - IF ANOVA IS STATISTICALLY SIGNIFICANT, move on to interpreting the post hoc test (Tukey) - To find the effect size (partial eta squared (η 2)), see ‘Tests of Between Subjects Effects’ table Writing Up the Results: - Give Background - Mention variables and groups - Explain tests of normality, outliers and homogeneity of variance - Report ANOVA results - Post-hoc tests Sample: - Analysis of Variance (ANOVA) was conducted to examine the impact of different teaching methods on students' test scores. Participants were classified into three groups: Group A (n = ?), Group B (n =?), and Group C (n=?). There were no outliers as assessed by boxplot; data was normally distributed for each group as assessed by Shapiro-Wilk test (p > 0.05); and there was homogeneity of variance as assessed by Levene's test of homogeneity of variance (p = ?) - Descriptive statistics revealed that Group A (M = X, SD = Y) had a mean test score of X with a standard deviation of Y, Group B (M = X, SD = Y) had a mean test score of X with a standard deviation of Y, and Group C (M = X, SD = Y) had a mean test score of X with a standard deviation of Y - The ANOVA results indicated a significant difference between the teaching methods, F (df between, df within) = F-value, p < 0.05. Post hoc tests using [appropriate post hoc test, e.g., Tukey's HSD] revealed that [specific group differences] were statistically significant (p < 0.05) - These findings suggest that the teaching method used significantly influenced students' test scores, with specific differences identified between certain teaching groups Good to Know: - One-way ANOVA using one independent variable - Two-and Three-way ANOVAs with two or three independent variables - ANCOVAs; these incorporate a covariate - MANOVAs; these include more than one dependent variable ANOVA: - ANOVA is used to compare more than two groups - Is there a different in the population between the different groups of the independent variable with respect to the dependent variable - One-factor (or one-way) ANOVA - Two-factors (or two-way) ANOVA - One-factor ANOVA with repeated measurements - Two-factors ANOVA with repeated measurements One-Factor (or One-Way) ANOVA: - Is there a difference in the population between the different groups of the independent variable with respect to the dependent variable? - E.g. Investigating whether three different therapies result in different therapeutic successes for treating a shoulder injury Two-Factor (or Two-Way) ANOVA: - Does factor 1 have an effect on the dependent variable? - Does factor 2 have an effect on the dependent variable? - Is there an interaction between factor 1 and factor 2? One-Factor ANOVA with Repeated Measurements: - An extension of the t-test for dependent samples for more than two groups Two-Factors ANOVA with Repeated Measurements: - Does the first factor with measurement repetition have an effect on the dependent variable? - Does the second factor have an effect on the dependent variable? - Is there an interaction between factor 1 and factor 2?