Quantitative Research SoHP503 Project Studies PDF
Document Details
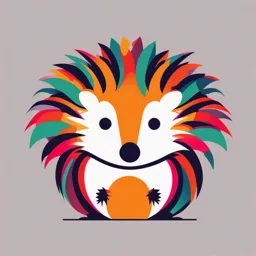
Uploaded by ManeuverableHarpsichord
University of Plymouth
2023
SoHP503
Dr Krithika Anil
Tags
Related
- Characteristics, Strengths, & Weaknesses of Quantitative Research PDF
- Lecture 1: Quantitative Analysis in Social Sciences - PDF
- Research Methods 1 Lecture 1 2023-24 PDF
- Quantitative Research vs Qualitative Research PDF
- PSCI 2702 Textbook: Statistics for Social Research - PDF
- Quantitative Data Analysis PDF
Summary
This is a set of lecture notes on quantitative research, covering topics such as descriptive statistics, distribution, and inferential statistics. The notes are presented in a slide format with examples.
Full Transcript
Quantitative Research SoHP503 Project studies Dr Krithika Anil Research Fellow Virtual Meeting Etiquette SoHP503 Turn off your phone Leave the keyboard alone (unless you are typing something in the chat, or you are doing an activity) Dress appropriately (Don’t wear your pyjamas, have a show...
Quantitative Research SoHP503 Project studies Dr Krithika Anil Research Fellow Virtual Meeting Etiquette SoHP503 Turn off your phone Leave the keyboard alone (unless you are typing something in the chat, or you are doing an activity) Dress appropriately (Don’t wear your pyjamas, have a shower before the session starts and something to eat and drink) Be aware of your surroundings (No beds, No messy rooms, No inappropriate posters/ backgrounds) Mute your microphone when you’re not talking Speak up Stay seated and stay present (Position your camera so that your whole head is visible and not just your forehead, chest or the room) 02/01/2023 SoHP503 Quantitative Research | Dr Krithika Anil 2 What is this lecture about? You have collected quantitative data. Now what? Learning Objectives: Ø Understand and apply appropriate descriptive statistical techniques to your data Ø Understand and present data appropriately Ø Understand and apply appropriate inferential statistical techniques Ø Critically appraise the techniques available in relation to your research aim SoHP503 Quantitative Research | Dr Krithika Anil 3 What makes numbers important? Score = 5 A 5/5 All students got this score B 5/10 50% of students got this score Highest score was 7/10 C 5/100 Top 50% of students got this score or more Highest score was 15/100 Example illustrates: Numerical context SoHP503 Quantitative Research | Dr Krithika Anil 4 What makes numbers important? Image taken from: https://www.physio-pedia.com/Numeric_Pain_Rating_Scale Example illustrates: Topic context SoHP503 Quantitative Research | Dr Krithika Anil 5 What makes numbers important? Your research question and objectives Your research question Report data based on research Analyse data question and objectives and objectives Exploratory Hypothesis Example illustrates: Analysis focus SoHP503 Quantitative Research | Dr Krithika Anil 6 Data type Categorical What type of data have you collected? Numerical Ordinal Nominal Numerical data is in the Ordinal represents Nominal represents non- form of numbers ordered narrative data ordered narrative data Examples: height, number Examples: rankings, Examples: preferences, of patients, walking satisfaction rating, spice nationality, hair colour speed, assessment score tolerance SoHP503 Quantitative Research | Dr Krithika Anil 7 Descriptive statistics Descriptive statistics means describing the data you have collected Common descriptive analysis techniques: Frequency Central tendency Distribution Mean difference SoHP503 Quantitative Research | Dr Krithika Anil 8 Descriptive statistics – Frequency Likeliness to Eye Colour Watch Horror Not likely Brown Likely Brown Frequency table Definitely Green Likeliness to Not likely Blue Count how many Watch Count Eye Colour Count Definitely Blue times each value Horror Likely Green appears Not likely 5 Blue 5 Not likely Blue Likely 4 Brown 4 Likely Green Definitely 4 Green 4 Not likely Blue Definitely Blue Definitely Green Not likely Brown Likely Brown SoHP503 Quantitative Research | Dr Krithika Anil 9 Descriptive statistics - Frequency Two categorical variables (Cross-tab) Frequency table Likeliness to Likeliness to Watch Horror (%) Which data display is better? Eye Colour Watch Horror Eye Colour (%) Definitely Likely Not likely Total Blue 2 (15%) - 3 (23%) 5 (38%) Both are fine as they provide easy-to- Not likely Brown read information Brown - 2 (15%) 2 (15%) 4 (31 %) Likely Brown Green 2 (15%) 2 (15%) - 4 (31 %) Definitely Green Tables provide more specific Total 4 (31 %) 4 (31 %) 5 (38 %) 13 (100%) Not likely Blue information Definitely Blue Bar chart Graphs provide “at-a-glance” Likely Green 4 information that is easy to grasp Likeliness to Watch Horror Not likely Blue Likely Green 3 Too many variables? A table will be Not likely Blue better (Count) 2 Defi nitely Definitely Blue Likely Use a graph to highlight your main or Definitely Green 1 Not l ikely interesting results Not likely Brown Likely Brown 0 (Note: You can do the same here with 1 Blue Brown Green Eye Colour categorical variable and 1 numerical variable) SoHP503 Quantitative Research | Dr Krithika Anil 10 Descriptive statistics - Frequency Two numerical variables Frequency table Scatter plot with trend line Age 162 Age Height (cm) Height (cm) 40 41 43 45 47 49 Total 161 153 - 2 (15%) - - - - 2 (15%) 43 154 160 154 - - 1 (8%) - - - 1 (8%) 159 41 153 Height (cm) 155 1 (8%) - 1 (8%) - - - 2 (15%) 158 45 156 156 - - 1 (8%) 2 (15%) - - 3 (23%) 157 157 - - - 2 (15%) - - 2 (15%) 156 47 159 159 - - - - 1 (8%) - 1 (8%) 155 49 161 160 - - - - 1 (8%) - 1 (8%) 154 161 - - - - - 1 (8%) 1 (8%) 153 45 157 Total 1 (8%) 2 (15%) 3 (23%) 4 (31%) 2 (15%) 1 (8%) 13 (100%) 152 47 160 40 41 42 43 44 45 46 47 48 49 50 43 156 Age (years) 45 157 41 153 Which data display is better? 45 156 40 155 The frequency table is too crowded and hard to read 43 155 The scatter plot is much easier to read and we can immediately see what’s going on Use a scatter plot when showing two numerical variables SoHP503 Quantitative Research | Dr Krithika Anil 11 Descriptive statistics - Frequency Two numerical and one categorical Scatter plot with trend lines coloured by category Age Height (cm) Eye Colour 162 161 43 154 Brown 160 Blue 41 153 Brown 159 Brown Height (cm) 158 45 156 Green Green 157 47 159 Blue 156 49 161 Blue 155 154 45 157 Green 153 47 160 Blue 152 43 156 Green 40 41 42 43 44 45 46 47 48 49 50 Age (years) 45 157 Blue 41 153 Blue 45 156 Green 40 155 Brown You can choose to plot 2 numerical variables and 1 categorical variable as above 43 155 Brown If it’s too messy/hard to grasp, use a table instead! SoHP503 Quantitative Research | Dr Krithika Anil 12 Descriptive statistics - Central tendency Central tendency represents a centre point of a dataset Mean – The sum of all values divided by the total number of values Median – The middle value when data is arranged in ascending order Mode – The most frequent value Example Data 18, 19, 45, 21, 41, 21, 37, 35, 44, 21, 46 Median Mean Ascending order Mode Sum of all values/total = number of values 18, 19, 21, 21, 21, 35, 37, 41, 44, 45, 46 Most frequent value = Middle value = 35 = 348/11 21 = Two middles values? Median is the 31.64 mean of those two values SoHP503 Quantitative Research | Dr Krithika Anil 13 Descriptive statistics – Distribution Likeliness to Watch Horror Frequency table Count how many 6 Likeliness times each value 5 Height to Watch Count Count appears 4 (cm) Count Horror AND 3 Not likely 5 153 2 Put the count on the 2 1 Likely 4 154 1 y-axis and the value 0 Definitely 4 155 2 names on the x-axis Not likely Like ly Definitely 156 3 157 2 Height (cm) (note: if value names 4 159 1 are numerical or 160 1 ordinal, make sure 3 161 1 Count they are in ascending 2 order on the x-axis) 1 0 153 154 155 156 157 159 160 161 SoHP503 Quantitative Research | Dr Krithika Anil 14 Descriptive statistics - Distribution Normal Distribution Skewed Distribution 5 9 8 4 7 6 3 Count Count 5 4 2 3 1 2 1 0 0 155 156 157 158 159 160 161 162 163 155 156 157 158 159 160 161 162 163 Height (cm) Height (cm) Mean = 159cm O Mean = 157cm O Median = 159cm O Median = 156cm O Report mean Report median (note: height data here has more values than the previous height data example) SoHP503 Quantitative Research | Dr Krithika Anil 15 Descriptive statistics - Distribution Standard deviation (SD) indicates the spread of data The further the points are away from the mean (i.e. more spread) = higher SD 1 standard deviation has 68% of the data points 2 standard deviations has 95% of the data points GIF taken from: https://commons.wikimedia.org/wiki/File:Dp-sim%C3%A9trica.gif σ = Standard deviation μ = Mean 3 standard deviations 99.7% of the data points SoHP503 Quantitative Research | Dr Krithika Anil 16 Descriptive statistics - Distribution Small spread = small SD Large spread = large SD Mean Mean Product review example Product has a mean of 4.5 stars Most of the reviews will be 4-5 stars Still mostly 4-5 stars, but more 3 stars Medicine example Group A and B took X nausea medicine X medicine decreased nausea by a mean of 10 units Group A has a small SD Group B has a large SD Most of Group A had a decrease of 10 units Most of Group B had a decrease of 10 units, but more participants experienced less of a decrease compared to Group A SoHP503 Quantitative Research | Dr Krithika Anil 17 Descriptive statistics - Distribution SD is not useful for a skewed distribution. Instead, Example Data report the median and the interquartile range (IQR). 18, 19, 45, 21, 41, 21, 37, 35, 44, 21 Median The IQR tells you the spread of the middle half of your data, i.e. the value at Q1 and the value at Q3. Ascending order (Q2 would be your median.) = 18, 19, 21, 21, 21, 35, 37, 41, 44, 45 Split your data in half so you have your “1st” half and Median (Q2) = 28 your “2nd” half. The median of the 1st half is your Q1 and the median of the 2nd half is your Q3. 1st half = 18, 19, 21, 21, 21 Median (Q1) = 21 2nd half = 35, 37, 41, 44, 45 Median (Q3) = 41 SoHP503 Quantitative Research | Dr Krithika Anil 18 Descriptive statistics - Distribution t b ox p lo le da How do I report it? ca l T h is is Report the median with Q1 and Q3 in brackets From the example on previous slide Q1 = 21 Median (Q2) = 28 Q3 = 41 Report it as: “The median was 28 (IQR = 21-41)” Image taken from: https://commons.wikimedia.org/wiki/File:Boxplot_vs_PDF.svg SoHP503 Quantitative Research | Dr Krithika Anil 19 Descriptive statistics – Mean Difference Mean difference is the difference in mean between Standardised mean difference (SMD) is the mean two groups difference divided by the SD It measures the size of an effect, i.e. an effect size Use SMD if comparing outcomes measured with Example different tools, such as in systematic reviews Group A and B took X medicine, which reduces nausea Ø E.g. weight was measured using kg, ounce, and Group A had a mean reduction of 10 units lbs Group B had a mean reduction of 6 units Ø E.g. depression outcome was measured using Becks Depression Inventory and the PHQ-9 Mean Difference Ø Also known as Cohen’s d MeanA – MeanB = Example 10-6 Group A and B had an average SD of 2 = SMD 4 Mean difference/SD = 4/2 = 2 SoHP503 Quantitative Research | Dr Krithika Anil 20 Inferential statistics Inferential statistics uses the data you have collected to make assumptions, i.e. make “inferences” Common inferential analysis techniques: Correlation T-test ANOVA SoHP503 Quantitative Research | Dr Krithika Anil 21 Inferential statistics – Sample Size Sample The group of individuals your recruit from your target population Sample Size The number of individuals you recruited Important! Before conducting inferential statistics, make sure you have a large enough sample size Otherwise, just use descriptive statistics Why is sample size important? Inferential statistics makes assumptions based on the data you have collected To be confident in these assumptions, you need an appropriately large sample size If you have multiple groups, ensure the groups are equal in size for accurate comparisons SoHP503 Quantitative Research | Dr Krithika Anil 22 Inferential statistics – Sample Size Product Review Example Which one will you more likely buy? 12 reviews 232 reviews Kettle A Kettle B SoHP503 Quantitative Research | Dr Krithika Anil 23 Inferential statistics – Sample Size But how do I calculate sample size? Random samples A sample size calculation is based on how likely your results will fall within a certain range 320 Population size Dieticians Mean = 46.5 You need three things: Population size Confidence level 9508 460 Margin of error (i.e. confidence interval) Dieticians in Dieticians Mean = 55.7 The UK 130 Dieticians Mean = 39.78 Mean will always fall within a certain range BUT you don’t know this range, so you have to guess SoHP503 Quantitative Research | Dr Krithika Anil 24 Inferential statistics – Sample Size But how do I guess the results range? Use the confidence level and margin of error (i.e. confidence interval) Confidence Level Margin of error How confident do you want to be in your guess? The size of your range If you have a 100% confidence level, you have 0% E.g. Your mean is 42.3 chance of being wrong 10 confidence interval = 42.3 ± 10 Unrealistic 2 confidence interval = 42.3 ± 2 Impossible to do any research The margin of error you choose depends on your field If you have a 70% confidence level, you have 30% chance of being wrong The smaller your margin of error, the smaller your Not good enough results range Unreliable results The smaller your results range, the more accurate your results are likely to be A 95% confidence level is the norm BUT the smaller margin of error, the more participants you need SoHP503 Quantitative Research | Dr Krithika Anil 25 Inferential statistics – p-value The p-value The likelihood that an outcome was by chance The p-value is used to decide whether or not to reject the null hypothesis Null hypothesis: There is no difference between the research groups Alternative hypothesis: There is a difference between the research groups If the p-value is significant, you reject the null hypothesis! It does not mean that your research hypothesis is true! A significant p-value only points out that there is a difference between research groups, and nothing more. SoHP503 Quantitative Research | Dr Krithika Anil 26 Inferential statistics – p-value A significant difference A meaningful difference A significant p-value A significant p-value VERSUS The difference is practically useful Example Example Group A had usual care Group A had usual care Group B took part in a weight Group B took part in a weight gain intervention lasting 3 gain intervention lasting 3 months months Group B gained an average of 200g Group B gained an average of 8kg more than Group A, where the p- more than Group A, where the p- value was significant value was significant SoHP503 Quantitative Research | Dr Krithika Anil 27 Inferential statistics – Parametric and Non- Parametric Parametric Statistics Non-Parametric Statistics The statistical test are based on distribution The statistical test are not based on assumptions about your data distribution assumptions about your data Example Assumptions Your data is normally distributed x Your data is linear Your data has no outliers x Linear: Non-Linear: Trend is a straight line Trend is a not straight line SoHP503 Quantitative Research | Dr Krithika Anil 28 Inferential statistics - Correlation A correlation is the extent to which one variable moves in relation to another A correlation coefficient is the correlation value This value can be between -1 and 1 E.g. 0.9 or -0.9 is a strong correlation E.g. 0.2 or -0.2 is a weak correlation Correlation IS NOT causation Positive correlation: Negative correlation: No correlation: When one variable When one variable The variables do not increases, the other increases, the other relate to each other increases decreases SoHP503 Quantitative Research | Dr Krithika Anil 29 Inferential statistics - Correlation Scatter plot with trend line Pearson’s r (parametric) assumptions: 162 161 Your data type is numerical 160 Your data is normally distributed Height (cm) 159 158 157 156 Your data is linear 155 154 You have no outliers 153 152 40 41 42 43 44 45 46 47 48 49 50 Age (years) Note: If your data’s relationship has more than one direction, don’t use a correlation (whether it is parametric or non- Positive correlation: parametric) As height increases, age also increases Data goes up and then down again SoHP503 Quantitative Research | Dr Krithika Anil 30 Inferential statistics – T-test A t-test compares the means of two groups Example BUT, is this a significant difference? Group A and B took X nausea medicine (i.e. did this just happen by chance?) Group A had a mean reduction of 10 units Group B had a mean reduction of 6 units A t-test will produce a t-value Mean Difference The bigger the t-value, the more likely the MeanA – MeanB difference is significant = It can be negative or positive, which indicates 10-6 direction = o E.g. A-B = 4 4 o E.g. B-A = -4 SoHP503 Quantitative Research | Dr Krithika Anil 31 Inferential statistics – T-test General t-test (parametric) assumptions: Your data type is numerical Your data is normally distributed Your data is independent (i.e. data points in one group are only counted once) You have no outliers There needs to be homogeneity of variances (i.e. the distribution of the two groups are similar) Types of t-tests: 1. Independent t-test – Group A and Group B are two different populations (e.g. diabetic v non-diabetic) 2. Paired t-test – Group A and Group B are the same population (e.g. pre v post intervention) 3. One sample t-test – Comparing one group to a specific value (e.g. comparing height to the national average) SoHP503 Quantitative Research | Dr Krithika Anil 32 Inferential statistics – Variable Types Independent Variable Dependent Variable The variable the researcher changes The variable that is changed by the type of (i.e. the cause) independent variable (i.e. the effect) Examples Examples Type of medicine: paracetamol vs aspirin Pain level Type of care: usual care vs intervention Patient self-management Type of exercise: strength vs balance vs stamina Functional mobility SoHP503 Quantitative Research | Dr Krithika Anil 33 Inferential statistics – ANOVA An ANOVA (analysis of variance) compares the means of more than two groups Example BUT, is this a significant difference? Group A, B, and C took X medicine, which reduces nausea An ANOVA will produce an F-value Group A had a mean reduction of 10 units The bigger the f-value, the more likely the Group B had a mean reduction of 6 units difference is significant Group C had a mean reduction of 1 unit Conduct a post-hoc test, which tells you how the Mean Difference Mean Difference Mean Difference groups differ from each other MeanA – MeanB MeanA – MeanC MeanB – MeanC = = = 10-6 10-1 6-1 = = = 4 9 5 SoHP503 Quantitative Research | Dr Krithika Anil 34 Inferential statistics – ANOVA General ANOVA (parametric) assumptions: Your data type is numerical Your data is normally distributed Your data is independent (i.e. data points in one group are not related to data points in another group) You have no outliers There needs to be homogeneity of variances (i.e. the distribution of the two groups are similar) Types of ANOVAs: 1. One-way ANOVA – 1 independent variable and 1 dependent variable (e.g. medicine type and pain level) 2. Two-way ANOVA – 2 independent variables and 1 dependent variable (e.g. medicine type, severity type, and pain level) 3. MANOVA – More than 1 dependent variable (e.g. medicine type, pain level, and side effects severity) 4. Repeated measures – Participants are the same in all groups (e.g. pre v post intervention) SoHP503 Quantitative Research | Dr Krithika Anil 35 Activity – Practical Example Type 2 Diabetes Intervention Using a Mobile App1 Objective: This study aims to evaluate the effectiveness of the My Diabetes Coach (MDC) app designed to support diabetes self-management in the home setting over 12 months. Methods: Adults with type 2 diabetes were randomized to the intervention arm (MDC) or the control arm (usual care). Program use was tracked over 12 months. Outcomes included changes in glycated hemoglobin (HbA1c) and health- related quality of life (HRQoL). Results: A total of 187 adults with type 2 diabetes were randomly allocated to the intervention (n=93) and control (n=94) arms. The mean HbA1c decreased in both arms (intervention: 0.33% and control: 0.20%), but the net differences between the two arms in change of HbA1c (−0.04%; p=.83) was not statistically significant. HRQoL scores improved in the intervention arm, compared with the control arm (between-arm difference: 0.04%; p=.04). SoHP503 Quantitative Research | Dr Krithika Anil 36 Activity – Practical Example Type 2 Diabetes Intervention Using a Mobile App1 Methods: Adults with type 2 diabetes were randomized to the intervention arm (MDC) or the control arm (usual care). Program use was tracked over 12 months. Outcomes included changes in glycated hemoglobin (HbA1c) and health-related quality of life (HRQoL). Information Gathered Two groups: the intervention group and the usual care group (i.e. the independent variable: type of care) Multiple times points (potentially): study was 12 months long Outcome measures: glycated haemoglobin and health-related quality of life (i.e. dependent variables) SoHP503 Quantitative Research | Dr Krithika Anil 37 Activity – Practical Example Type 2 Diabetes Intervention Using a Mobile App1 Results: A total of 187 adults with type 2 diabetes were randomly allocated to the intervention (n=93) and control (n=94) arms. The mean HbA1c decreased in both arms (intervention: 0.33% and control: 0.20%), but the net differences between the two arms in change of HbA1c (−0.04%; p=.83) was not statistically significant. HRQoL scores improved in the intervention arm, compared with the control arm (between-arm difference: 0.04%; p=.04). Information Gathered For now, just look at the numbers and ignore the statements Sample size was equal in both groups (but we don’t know if this sample size was large enough) Glycated hemoglobin differed between the groups but it was not significant Health-related quality of life differed between the groups and it was significant Questions to Ask Was the significant difference a meaningful difference? No Make sure to read the Do the numbers match the statements? Technically, but not completely truthful rest of the paper for more context, don’t just read the abstract! SoHP503 Quantitative Research | Dr Krithika Anil 38 References 1. Gong, E., Baptista, S., Russell, A., Scuffham, P., Riddell, M., Speight, J.,... & Oldenburg, B. (2020). My Diabetes Coach, a mobile app–based interactive conversational agent to support type 2 diabetes self-management: randomized effectiveness-implementation trial. Journal of medical Internet research, 22(11), e20322. SoHP503 Quantitative Research | Dr Krithika Anil 39 Please use the discussion board in case of questions. Thank you! Thank you! J