Lecture 1 PDF
Document Details
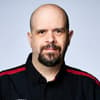
Uploaded by SmarterZircon
Tags
Related
- Medical Terminology in Epidemiology and Public Health PDF
- Chapter 2 Principles of Epidemiology in Public Health Practice PDF
- Epidemiology of Diseases of Public Health Significance Group 2 PDF
- Epidemiology BS Public Health 2024 PDF
- Public Health Lecture Notes PDF
- Epidemiology in Community Health Care PDF
Summary
This lecture introduces the concepts of epidemiology, focusing on the distribution and determinants of health-related events in a population. It covers key measures such as prevalence (point and period prevalence) and incidence (cumulative incidence, incidence rate), as well as mortality rate, morbidity rate, and attack rate.
Full Transcript
SPEAKER 0 So study about incidence prevalence. So if you look at the formal definition. Epidemiology is the study of the distribution and determinants of health related states or events in a specified population. And then you just don't stop at describing these other variables. But you also apply this...
SPEAKER 0 So study about incidence prevalence. So if you look at the formal definition. Epidemiology is the study of the distribution and determinants of health related states or events in a specified population. And then you just don't stop at describing these other variables. But you also apply this to the study of to control health problems as well. So this sort of breaks down the world based on its origin. So epi means upon the most people and logos means study. So you're essentially looking at what is happening among the population that you're interested in looking at, and the study of what is upon the people. I've highlighted the words that are most important. If somebody asks you what is epidemiology, you should quickly be able to say it's looking at distribution and determinants of disease. Okay. So one of the objectives of the field or the discipline. So epidemiology seeks to identify factors that are causes of diseases. We count the cases of disease. And you know look at how much disease is present in in a population. You can study the natural history and prognosis of a disease, and that that information is going to help you and guide you through designing and evaluating interventions that help us improve our health. So that is sort of the broad objectives of the discipline itself. And from this, you can easily derive the need for knowing how to measure what is going on. Right. And that's why we come to this idea of looking at diJerent measures of disease frequency. So the main measures that we are going to talk about in class today is prevalence. Two types of prevalence measures point prevalence and period prevalence. And then incidence which can be measured using two metrics cumulative incidence incidence rate. And then we look at some special type of incidence measures uh mortality rate morbidity rate and attack rate. Okay. So I'll review each of these measures in details. How to calculate that and what each of it means. So the first one is prevalence. So prevalence is quite straightforward. You're looking at the number of people with a certain disease in a population. And you divide it by the total number of people in your population. The important part here is you have to specify the time period that you're counting these existing cases for. Okay. So you have the number of people with the disease divided by total number of people that are in your in your population at a specified period in time or specified time. Two ways to do that. And then if you, you know, if somebody asks you what is prevalence, essentially it is people who are aJected by the disease at a given time. It can range from 0 to 1. It's useful for assessing the burden of a disease in the population because you're accounting for who already has the disease, right. So it's telling you the burden of the disease. It's valuable for planning health services. And why is that? Because if you know that a particular disease has a high burden in a population, you're able to take action to address it, right? So we've seen that with Covid, where every day you would get the number of new cases. Then over time it went on to the number of cases over weeks. And the reason for that is that we are accounting for prevalence rate. Is this an issue that needs our urgent attention? What is the burden of this disease in the population? Right. And it's not obviously useful for determining what causes the disease. It just tells you how much, but not how and why a disease has, you know, happened to a certain group of people. Okay. So what you need to remember here is prevalence is a measure of disease frequency. We are counting the number of existing cases, the burden of disease. And we calculated by dividing the number of people who have the disease by the total number of people in the population. SPEAKER 2 Okay. SPEAKER 0 Two ways to account for prevalence point prevalence and period prevalence. So let's see what the diJerence is. For point prevalence. You're looking at the proportion of a population that has the disease at a specific point in time. So it could be a calendar day or it could be a life event like birth death entry into the military for example. So you're looking at a very narrow, specific point in time to account for how many people already have the disease, whereas period prevalence accounts for the existing cases. But now you're looking at this period of time. Typically it would be 12 months depending on what disease you're looking at. But let's say if you're looking at flu or something like that, then maybe uh, period prevalence can be accounted on a weekly basis as well. So the time will define whether you are calculating point prevalence or period prevalence. But the big diJerence is for point prevalence. It is it is a specific point in time period. Prevalence usually is over a time period. So let's look at an example. So think of a scenario where you have 20 students in the class. The cross represents those who got flu. And let's assume that those who caught the flu recovered in seven days. So let's think of this whole. X mark as the time period that somebody has has the flu. Okay. And then you have these months represented by these colored boxes. So we have these three months here okay. And let's see. I ask you the question, what is the point prevalence for this population on 28th of Feb. Okay. Now remember for prevalence how do we calculate it. Number of cases divided by the total population. Right. So let's say this is Feb 28th. That's where the month ends. How many cases? Two. And what is the total population? 20. Right. So point prevalence on 28th Feb. Will be two over 20, which is 10%. This tells you the existing cases. Everybody's following. Now let's look at period prevalence. So the same situation. But I ask you the question diJerently. So I ask you to calculate the period prevalence of flu during the month of January. So the numerator is the number of cases. So we have Jan how many cases three. So that's going to be your numerator right. Number of existing cases divided by the total population 20. To three over 20. That is 15%. Is everybody following? SPEAKER 2 Yes. SPEAKER 5 There is 1%. That is the. Yeah, those two are between February and March. These two are in the same position in January. Really, he would be like, fine. In that case, yes. SPEAKER 0 Is everybody following. So you're basically looking at existing cases over total population specified by whatever time period you're looking at. And based on the time period, it could either be point prevalence or it could be period prevalence. Everyone's following. Now let's look at incidents. So incidents is diJerent from prevalence because for incidents we are accounting for the frequency of new cases during a span of time in the people that are at risk. And we'll come to this at risk in in on the next slide. So we are focusing on measuring the probability of developing the disease during a span of time. And the formula or how you calculate that is you divide the number of new cases divided by the total population at risk. Okay. And I'll explain in a bit what this at risk means. Is everybody following prevalence existing cases incidence new cases prevalence numerator is existing cases by total population incidence is new cases by the total population at risk okay. So now let's see what this at risk is. So we are basically considering the denominator to include only those who are at risk of developing the outcome. So that means that they either don't already have the disease, or they are essentially going to exclude people who cannot get the disease for whatever reason. You know, either they are immune to it through vaccination or they don't have an organ or, you know, let's say for an example, you're looking at, um, ovarian cancer, right? So from your denominator, males are not at risk because they don't have the organ itself. So that is this idea of at risk where from the denominator you exclude people because either they already have the disease or they are not going to get the disease for whatever reason. Is everybody following this idea of at risk? And then incidence can be measured using two metrics. One is called cumulative risk. It's also known as incidence proportion. Sometimes people just refer to it as plain risk. And then the other measure is incidence rate. Okay. Now let's see how we calculate that. The cumulative risk or incidence proportion. You have the numerator as the number of new cases and you divide. Divide that by the number of persons at risk of disease at the beginning of the study period. Okay. And then again, just as a note, I should have mentioned that in the intro for your final exam or any of the exams, our expectation is not that you memorize all the formulas. You will be given sort of a formula sheet that you can refer to. Uh, because this is this is not a math math course. Uh, so but again you have to know how to apply, uh, some of these ideas. Right. It's not like you can just have the formula sheet and not, not review the material, but you don't have to memorize the formulas, okay. Does that make most of y'all feel better now? SPEAKER 2 Okay. SPEAKER 0 So so you will have, uh, like a, like a sheet that has the formulas on it. SPEAKER 2 Okay. SPEAKER 0 So let's look at an example. So let's say a study was conducted in 2020. Uh looking at asthma among persons with dementia. Uh the study recruited 200 adults with dementia. Of the 260 had a prior diagnosis of asthma. And over the first year of this study, seven adults developed asthma. Okay, so is the situation clear what's happening here? There's a study looking at a population of people with dementia. And we are interested in the incidence, uh, or occurrence of asthma in this population. Okay. And we have 200 adults. 60, were diagnosed with asthma at the start of the study. And then all your went by and then you checked in again with them, and you found that there were seven additional people who have been diagnosed with asthma. Okay. Oh, so now if I have to calculate the incidence, the formula tells you it's the number of new cases during a given time period. So you have seven adults who have developed asthma. And now how would you calculate the total population at risk. 121 140 and can can you how how did you get that? 146. SPEAKER 2 Yeah. Yeah. Right. It's new to people. SPEAKER 0 So. So one person raise your hand and. SPEAKER 2 So, um, we have 200 adults and six of them already had asthma. SPEAKER 0 Exactly. So they are not at risk? SPEAKER 2 Correct. I already have this disease. Correct. So basically 200 -60 is going to be one quality. SPEAKER 0 Correct? Exactly. You're absolutely right. Is everybody following how we got that 140. We have 200 adults but 60 of them already have asthma at the start of the study. And that's why they cannot get estimates again in that one year period. Right. So that's why you subtract them because they are no longer at risk of experiencing this bad health outcome. Okay. So then you can say the one year incidence. And it's important to mention the time period. Because if you don't mention that it can be confusing. Okay. So the one year incidence of asthma among adults with dementia is 5%. You can also express it as 50 cases per 1000 persons with dementia. Again there's there's really no right or wrong between the two. Depends on what you prefer to write out as your answer. Is everybody following how to calculate incidence. And this idea of who you should not include in the denominator. So the idea of cumulative risk is not valid when large number of people leave your study, right. Because in your denominator, you're excluding people who already are not at risk. But imagine out of those 140. If I tell you that 100 people left the study, they just didn't show up. When you were assessing estimate status at the end of year one, right? So you really don't know what happened to those 100 people who left your study. And in that situation, you're not going to get an accurate estimate of the incidence using cumulative risk as as a measure. And why do you think people will not stay in your study? What are some reasons? Did I? Yes. They might move somewhere. SPEAKER 2 Other reasons they don't want to go. SPEAKER 3 They don't want to be a part of the stadium. SPEAKER 0 Yeah, yeah, it's just too far. I committed to something, and now I don't think it's worth my while to travel. I just decide not to show up. SPEAKER 3 I don't trust the investigators. SPEAKER 0 They don't trust the investigators. Think of like a drug trial, right? Like I decide to participate and I experience horrible nausea after taking the drug. I might decide this is, like, horrible. And I'm not just going to show up, just the side eJects that I'm experiencing because of the drug. So various reasons as to why people will not, you know, continue to be a part of the study. Right. And that's the situation where we don't want to use cumulative risk because it's not going to be an accurate estimation of, uh, incidence. So the epidemiological term for people leaving your study is called loss to follow up. Okay. So the idea that people drop out of your study for whatever reason. In epidemiology is referred to as loss to follow up. And there's this special type of loss to follow up where people leave your study because they die is referred in epidemiology as competing risk. That means people no longer are a part of your study because they die. Obviously, if death was your outcome of interest, then there is no competing risk, right? That they died and they experienced the bad outcome that you were interested in looking at. Okay, so the epidemiological term for people not continuing to be a part of your study is called loss to follow up. And why is loss to follow up a problem? I already told you one reason you're not going to get an accurate estimate. Think of the situation that we had out of the one 4000 people. Just don't show up. Only 40 people show up. You're not going to accurately estimate the true incidents, right? What could be the other reason why this is problematic? And we should be concerned about it? SPEAKER 4 You can skew the. SPEAKER 6 Building up the results you're expecting? Yeah. SPEAKER 2 Fires and it's like. SPEAKER 0 Yeah, you you just don't know what's going on in the population. Right? Of the 100 people that left maybe out of them instead of 740 already had asked the mayor or something like that. So you just don't know. So it's not going to be an accurate measure to quantify incidents. I also give you examples where you see that the dropout rate is diJerent between study groups. In a situation like I said, your your part taking part in a drug trial, you experienced such bad side eJects from the drug itself that you decide to no longer continue your participation and you just just don't show up. That means if your study goal was to see the eJectiveness of some drug, you're missing on the side eJects that people are experiencing just because they didn't show up to or don't want to continue in your study. Right? So it's problematic because then you're not going to accurately get an estimate of what's going on in in the population that you're interested in looking at. SPEAKER 6 Also, follow up something to consider before you start a study. SPEAKER 0 So there is going to be some loss to follow up in any study. Just just for practical reasons. Uh, ideally you want to maximize participation. And one way of doing that is either providing some type of incentive, having more like, you know, regular check ins with your study participants, uh, that can minimize loss to follow up. Uh, but there is going to be some amount of loss to follow up. When you're analyzing your data, you want to ensure that that loss to follow up is not because of reasons that are maybe associated with your intervention itself. So, uh, so I think your question is like, how do we how do I know how much loss of follow up I'm going to have at the start of the study? Right? Yeah. So we won't be able to estimate that based on, uh, prior research, like it's it's like 10%, 5%. Usually the researchers will say that we are going to at least recruit like, 400 participants. And out of which if we have like 2%, 10%, depending on what the research question is, uh, lost to follow up, we are going to ignore that in the analysis if we, you know, don't meet whatever pre-decided threshold we have, we are going to be concerned in the analysis stage. But but in reality, for practical reasons, there's always going to be participants that don't continue to be a part of your study. Right? For for whatever reason. Yeah. Okay. SPEAKER 3 Really? Two bites? Yes. SPEAKER 0 And we'll be talking about that in, in a, in a couple of maybe like 3 or 4 weeks. So the take home over here is cumulative. Risk is a measure of incidence looking at new cases of disease. It has limitations because it does not account for this idea of loss to follow up because the denominator does not account for that okay. So is it then valid to use this as a risk measure? And the answer is yes. And when would that be. So risk is more valid in studies where the follow up period is short and lost to follow up, and competing risk is low. So eJectiveness of flu vaccine in children really short period of time. You could run a study for a year and see how eJective the vaccine was. Very unlikely that children are going to die. So there the the issue of competing risk is not going to be a problem. So you can think of scenarios in which using risk is is valid because you're you're sort of meeting or accounting for that denominator accurately. Everyone's fallen. Now let's look at the other measure to calculate incidence is called incidence rate. And incidence rate addresses that limitation of not being able to account for loss to follow up in the denominator that risk has right. So incidence rate the numerator is the same number of new cases. But now we change the denominator to account for this idea of loss to follow up. So we instead of you know having a number where we say the denominator is total population at risk. Now we are going to count for the total time at risk. And we'll quickly get to that. How to do that. But from this slide what you need to remember is now we have another measure incidence rate, that addresses the limitation of our risk measure by accounting for the time that each participant is at risk of whenever they are, you know, part of your study. Now, how do you calculate that denominator? We will quickly review that. Is everybody following the diJerence between incidence and or cumulative incidence and incidence rate. Numerator is the same number of new cases. The denominator is diJerent because your risk measure does not account for loss to follow up, whereas incidence rate will do that by looking at the total time at risk. No, not necessarily. This just accounts for the problem that we have with loss to follow up, because the denominator takes into account the time that each participant is at risk. So your your accurately counting how much time they are actually at risk. Okay, we will come to your question because when I show you how to calculate that, that might clarify the diJerence. Okay. So people refer to it as incidence density rate, percent time rate, hazard rate. It's essentially incidence rate. I know it can be a little annoying to have like five names for the same thing. Uh, but for for this class, we will stick to, uh, calling the first one cumulative incidence or incidence proportion. And then we'll refer to this as incidence rate okay. So this is just the formula. Number of new cases divided by the total time at risk of the people that you're following or the amount of person time at risk. Conceptually, if you think this denominator is person time, which is people number of people multiplied the amount of time each one of them is contributing to your study. Obviously, many people are going to contribute diJerent times, and we'll see how that, uh, you know, plays in how we calculate the denominator. Okay. So these are some of the assumptions that we're making. So people are at risk for a disease until they have the disease obviously they are at risk or they die or their loss to follow up or the study ends. So these are the assumptions that we are going to make when we are calculating the time at risk. Okay. So now think of this grid as sort of a simple way to understand how we calculate percentile. Okay. So over here you have a study with ten participants. I have put them as their IDs P1 to P10. So ten participants the vertical or the horizontal or the or the row shows you the time period this T one to t 20. This T could be anything. It could be days. It could be weeks. It could be years. Just think of this as time okay. So we have 20 units of time. Is everybody following ten participants. And time. So this study ran for 20 units of time. Okay. Everyone's following. Okay. And then that red lightning bolt that you see there denotes that a person basically experienced whatever disease you are interested in looking. So for simplicity's sake, let's think we have ten participants followed over a period of 20 weeks. And that red lightning bolt is when they were diagnosed with flu. Okay. Everybody's understood what this chart is showing. So now for personal time. What we essentially do is we count the amount of time each participant is contributing to your study, and we add it up to be the denominator for incidence rate. Now let's look at what's happening here. So for participant one. If you see this. Time. That is the time that participant one was at risk, right? Until they actually experienced that event, the red lightning bolt. So for participant one, what you are going to do is you're going to calculate the amount of time that they were at risk. So we add this. So for participant one how much time and assume that you know you're diagnosed at the end of that time period. So at the end of the 14th week. So how much time did participant one contribute to your study. What was the time that they were at risk? 14 units right. So this is the time that we're interested in once they have the flu, which is our disease of interest, they still continue to be a part of your study, denoted by that green highlighting. But we are not interested in that time because they're no longer at risk of developing the flu. Okay. I mean, someone could get the flu again, but very unlikely that they're going to get it in the remainder of six weeks. Is everybody following? SPEAKER 2 But this person is. SPEAKER 0 So this person would be included in the cumulative risk as well, but not the time. They are just going to be counted as one, like one person. We'll go through an example and that will help you with that clarification. So for participant one, this participant has contributed 14 units of time to your study. Okay. So now what is the time at risk for participant four and participant five. So participant four. The blue is the time that they are at risk. There is no red lightning bolt, so they didn't experience their disease, so they are lost to follow up. We don't know what happened to them, right? So how much time are they at risk? 11 units. Right. And how much time is person five at risk? Play 20 units at the end of the 20th week. Poor fellow did get the flu, right? Is everybody following how you count accounting for each participant's time at risk? Basically counting the time that they are a part of your study. The retirement risk ends. Either they get the disease or they are lost to follow up. SPEAKER 6 To follow after the final. SPEAKER 0 Yeah. Let's let's make the assumption that, you know, this person made it to the end of the 11th week. Is everybody following how we are accounting for person time at risk? SPEAKER 2 Okay. Oops. SPEAKER 0 So now using this idea, how do we calculate the incidence rate for for our this study right, which includes ten participants followed over 20 weeks of time. Right. So the numerator if you remember is the number of new cases. So how many cases happened over this 20 week period. SPEAKER 2 Three. SPEAKER 0 And then for the denominator you know how to calculate each participant's time at risk. And then you add that to be the denominator for calculating incidence rate. Okay. So let's look at that. So you already have the three. So now parties maybe. Let's go. SPEAKER 2 Back. SPEAKER 0 Participant one. How much time? 14. Participant two so 14 plus 20. SPEAKER 2 Participant 311. SPEAKER 0 Plus 11. Participant 411 526 710. SPEAKER 2 892. SPEAKER 0 Ten. SPEAKER 2 Okay. SPEAKER 0 You guys got this. SPEAKER 2 Huh? SPEAKER 0 And then you add it all. That is your total time at risk. Is everybody following how we got the denominator for incidence rate. And then you express that. So three over 138 that is 0.022 or 22 per thousand person time. And that time could be anything we could say person weeks person days depending on whatever your study parameters are. Everybody's following how we got the denominator and how it's diJerent. If I ask you to calculate the cumulative incidence or risk, what would that be? It would be the number of new cases. So the three would still be the same because that's the number of new cases. And the denominator was total number of people that are at risk. So what would the denominator be. Again. Correct. So that is diJerent from this. SPEAKER 2 Let me go ahead. SPEAKER 0 Before this. SPEAKER 2 What this means for the. SPEAKER 0 Blue color is the time at risk. The green. The green is when they are not at risk or they are lost to follow up. But they got the disease. Three so there are three cases, right? So three people out of the ten got the disease. So we have participant one, participant three and participant five. So that's three people. SPEAKER 2 Mhm. Yeah. SPEAKER 0 So those are the new cases. SPEAKER 2 Yes. What happened to patient seven this weekend? SPEAKER 0 No, he was lost. We just don't know. Lost to follow the yellow. We don't know. We don't know if they are at risk or not. Because once they leave your study and I'm unable to see what has happened exactly he or she. And then we could. I mean, they could have got the flu, but I just don't know as the researcher. Right. Because they are lost to follow up. I think everybody's following this. So this is a summary. I already went over this. The diJerence between both these measures. Your cumulative risk is easy to calculate and understand. You have new cases. You have the total number of people that are at risk. It's less accurate when you make the assumption that that everybody continued to be a part of your study. It's more useful for fixed population. Fixed population here means that population which does not have a lot of inflow or outflow of participants, uh, incidence rate obviously is more accurate because you're accounting for each participant's time at risk. Um, obviously this person time denominator is diJicult to calculate and understand. It's not as intuitive as like, you know, just saying percentage of something. Right. Uh, but it's more useful when you have a dynamic population, when you have people entering your study, leaving your study. Uh, it just makes more sense to calculate this. Everyone's following. This just is sort of a summary of what's the diJerence between prevalence and incidence. What you need to remember here is prevalence is existing cases. Incidence is new cases. And they're they're related. And we're going to see how. But before that, let's have a quiz question. And I'm going to put put a timer so you'll get like 1.5 minute to answer this question. Okay. Time starts now. SPEAKER 3 We don't have. SPEAKER 2 Time. Oh. SPEAKER 0 Really? SPEAKER 2 Yeah. SPEAKER 0 Oh, yeah, it is close. Sorry about that. Let me. SPEAKER 2 Uh, okay. SPEAKER 0 Then time starts again. SPEAKER 2 You. Okay. SPEAKER 0 Do you guys want to sit here? Like I just realized that you all are standing at the back. You can come sit here. Are you sure? SPEAKER 2 Okay. Great. Where is the diner for this? SPEAKER 0 I'll show up once everyone's done. SPEAKER 2 Okay. SPEAKER 0 You have 30 more seconds. All right. Time's up. Good job guys. That is the correct answer. So we are looking at new cases right? So we are looking at the frequency of new cases of TB in Boston. So the correct answer is cumulative incidence also known as incidence proportion. So good job. SPEAKER 2 Okay. SPEAKER 0 Next question. SPEAKER 2 Yes. Are. SPEAKER 0 Oh, and time starts now. SPEAKER 2 You. SPEAKER 0 30 more seconds. All right. Time's up. SPEAKER 2 Oh. Good job. SPEAKER 0 So the right answer is incidence rate, which 93% of the class got it right. So good job. Okay. So moving on now how is incidence and prevalence related. Right. So prevalence basically can be considered as a function of incidence times duration. And this figure can you know help you understand what that means is incidence. Remember is the new cases. So let's say we have this jar and we are pouring water. That's the new cases. Or forget water we are adding new marbles. It's easier to understand when you drop like one marble in a jar. So those are the new cases as the jar is getting filled with the marbles. Those are the existing cases. So your prevalence or your number of your marbles is increasing as new cases or new marbles are dropping inside the jar. Right. And then from a health perspective, what can happen is so that we get to draw out the marbles, either the disease you get cured of the disease. So you're no longer an existing case. Or you die, right? For whatever reason, does it have to be? SPEAKER 2 That's how you. SPEAKER 0 Can be removed or think of like diabetes, right? So you have a diabetes jar. Every year new cases are added. Then a diabetic case today, which is a new case for today, becomes a prevalent case for tomorrow because now it's already there. And then obviously, again, maybe diabetes is not a great example because you're not going to get cured, but you can. SPEAKER 2 You can. SPEAKER 0 Yeah you can. You can die and you can be out from that population of diabetics. Right. And for whatever reason, um, and it's not, not a great idea to say the word die so many times starting the new year. Uh, but but you guys get the point, right? So. Is everybody following what's happening and how prevalence and incidence is related? There is going to be a there are going to be two quiz questions. So make sure if you have any questions regarding how these two are related. Ask them now. So prevalence is related to incidence or it's a function of incidence time duration of a disease. SPEAKER 2 Okay. SPEAKER 0 So think of like maybe another like just pick one disease and then we'll sort of work through that Covid right. Covid right. So let's say you have a jar and then right at the in the middle of the pandemic, like, you know, during the Omicron wave, you had a lot of cases happening, right? So the incidence was really high. But doesn't always necessarily mean that your prevalence is also going to be as much right, depending on what treatment is available or you get cured or whatever that is happening. So you see how the prevalence and incidence are related by the duration of the illness itself. So think of exactly because let's say you have think of flu as a simple example. Right. So when you have like an increase in the incidence, the prevalence during that time also increases. But let's say you have a seven day duration for the disease and you're getting cured. And the same number of people are going to get removed from that jar. Right. So it's it's sort of this balance between the new cases and the existing cases based on what disease you're looking at, which is going to determine the duration. SPEAKER 2 Okay. SPEAKER 0 So let's think of a very rare type of I know I'm going to say death again, but let's think of like a rare type of cancer where the survival rate is very low. You know, once maybe your person gets diagnosed, uh, they're going to, um, die pretty pretty soon from it. So you won't see as much of the prevalence because you're going to quickly be the minute you enter the jar, you're going to be removed. So does that mean that cases are not happening? It's just dependent on this duration of the disease that you're interested in looking at. Is that relationship clear between how prevalence and incidence can uh or prevalence is the function of incidence and duration. SPEAKER 3 So if a person got Covid uh, so it's treated as a new case, uh, then got cured and then got Covid again after a month. So would that be considered as a new as incidence as new? SPEAKER 0 Depends on what time period you're looking at. So let's say if my study ran for six months and this person got Covid twice in six months, then they would be considered as two cases depending on what you're trying to calculate. So it's important to remember here that your accounting for that time that person would be an incident case. If my study just ran for like a month and they got Covid once. Does that clarify your question? So the time period here is important because let's say I ask you to calculate the incidence of diabetes for 2023. The people who are going to be in the numerator for 2023 are going to become prevalent cases when it's 2024, right? If I ask you to calculate the prevalence of diabetes for 2020, for those incidence cases of 2023 will be prevalent cases. So that mentioning that time period is very important. SPEAKER 2 Okay. SPEAKER 0 One more quick question. Time starts now. So. And these people are not there. They're surviving with the disease. So think of, like, a condition, like diabetes. Yeah. Because you're not going to get cured, right? 15 more seconds. All right. Good job again. So the prevalence will increase. And the reason behind that is think of this jar again. Right. Where did it go. So you have a steady number of incident cases. But now these people I think diabetes would be a good example here because of the treatments and drugs that are available. People are just living longer with that condition. Right. So the prevalence is going up. The number of cases each year pretty much could be consistent. But we say that the prevalence of this disease has gone up over the years because now people are just living longer with it. No, they're not cured. SPEAKER 2 Yeah. SPEAKER 0 Yeah. Like diabetes right? Like you're not cured of diabetes, but you can live with it. Yeah. Yeah. There's no cure. But yeah, it could be with all the anti retroviral treatments. Okay. One more question, guys. Is the polling closed again? No. It's open. Time starts now. SPEAKER 2 Discussion today. SPEAKER 0 So the question is telling you that there's this new treatment that prevents new cases of the disease. So in. SPEAKER 2 This case, do they have this? SPEAKER 0 No. These are they don't get the disease. So think of like a new new vaccine right. That just prevents the disease. SPEAKER 2 He's. SPEAKER 0 30 more seconds. SPEAKER 2 Everything else. SPEAKER 0 Whatever was the state of aJairs that continues? Yeah. SPEAKER 2 Yeah. Mhm. SPEAKER 0 All right. Time's up. Good job guys. All right so moving on to the next measure is mortality rate. So mortality mortality rate is a special type of incidence measure where you're dividing the number of deaths by the total population during a specified time period. Example would be in a year there were 1807 deaths from TB in the US. You have the denominator, which is the population of the US, and the mortality rate would be calculated by having the numerator as the number of deaths because of TB divided by the total population. So this is pretty straightforward. So let's say again a diJerent sort of way to quantify mortality. So in the first example we looked at mortality because of TB. Now we're looking at all cause mortality. So then again your numerator is the number of deaths divided by the total population size. So this is this is relatively straightforward okay. Then you have morbidity rate is the incidence of non-fatal cases of disease where people don't die because of the disease over a specified period in time. So again, same example, let's say 1982, there were 25,250 non-fatal cases of TB. That means these people were diagnosed with TB, but they didn't necessarily die during that year. Uh, and then, uh, you typically take the mid-year population and then morbidity rate of TB would be 25,000 to 50 over the total population size. So again this is also very straightforward. And then you have something called as the attack rate. So attack rate basically is the proportion of those exposed that develop the disease. And Covid again has shown us the importance of calculating attack rate. So think of attack rate as a group of people who are exposed, but not all go on to experience the disease. Right? We saw that with Covid, even if you are exposed to Covid, doesn't necessarily always mean that you're going to get Covid right. So that helps us quantify the attack rate, that is the proportion of those exposed that develop the disease. Okay. So a quick example of that would be let's say in the in this classroom we have like somebody who has Covid right. So all of us are exposed. So 100 people are exposed. And then five of us may be in the next 2 or 3 days. Go on. And why am I sounding so morbid at the start of it? Okay. So let's let's think of another group of 100 people of which one person has Covid. SPEAKER 2 Far. SPEAKER 0 Away. Exactly. Far, far away. Once upon a time, out of those 100, uh, one person has Covid, all 100 are exposed now and then five people go on to develop Covid, right? So the attack rate would be five over 100 because it is the proportion of people who go on to experience their disease. And they're also exposed to okay. So the denominator includes only those who are exposed, not everybody who is in this building. Or in the faraway land. SPEAKER 2 Do you count the person who. SPEAKER 0 In in. No. SPEAKER 2 No. People were. SPEAKER 0 No, because that person already has it. Right? SPEAKER 2 Yeah. SPEAKER 0 Okay. So now switching gears a little bit where we've covered the diJerent measures of frequency. Now let's look at measures of association. And again to provide some context as to why we are interested in quantifying these measures of association. And we are going to use what we've learned so far also. So make sure that you know, you don't have any questions so far. Is other measures of frequency clear before we okay. So basically, why do we care about these measures of association? So that brings us to this fundamental idea of what is a cause. Right. So in epidemiology remember that slide where we spoke about the objectives is to identify causes of diseases. Right. So this cause basically can be defined as an event a condition or a characteristic that preceded the disease event and without which the disease event would not have occurred at all or would have would have occurred until some later time. So the key words here is this event condition or a characteristic that preceded the disease. For something to be a cause of something, it has to be happening. It has to happen before it. Right. So in order for you to get Covid, you have to be exposed to the Covid virus before getting Covid, right? So that is the idea of a cause. Is everybody following. And this is the definition that we are going to use for class. Again, you don't have to memorize it. This helps you get, you know, or it helps you understand why we care about these measures of association. So in epidemiology we have this idea called as individual versus population cause. So what that means is an exposure can be a cause of disease, even if every individual who is exposed does not develop the disease. So this idea refers to a cause in the probabilistic sense. So those who are exposed have a higher risk of developing the disease. And we are not looking at cause in a deterministic sense where everyone exposed to an exposure gets their disease. A good example of that would be smoking and lung cancer. Do all smokers get lung cancer? No, but smoking increases your risk of getting lung cancer. So it is only fair in epidemiology. And we've accepted that as a general sort of statement that smoking causes lung cancer. Right. But it's this idea of probabilistic sense that not everybody who smokes gets lung cancer. Is everybody following? And think of a simple situation where you can think of a deterministic cause if you touch a heart store. Is everybody going to get a burn? Yes. So that is a cause in the deterministic sense, there is no probability that only half of us will get burned, right? All of us will get burned. So getting that burn from touching a hot stove is cause in the deterministic sense, whereas in the probabilistic sense, this idea of lung cancer and smoking in epidemiology, we think of this cause or this idea of a cause in the probabilistic sense, where people who are exposed have a higher risk of developing that outcome. So we can say that smoking causes lung cancer. Everyone's falling. SPEAKER 3 What about radiation exposure? SPEAKER 0 So again, depends on, uh, you know, you would have to be more specific. Is there a specific amount of radiation exposure, uh, or is there something more nuanced that you would be interested in looking at? Uh, but generally we've accepted that, you know, certain amount of exposure to radiation does increase risk for XY, uh, health or bad health outcomes. Right? So you would have to think more nuanced way of saying what exposure to radiation means. Maybe if I get like an x ray today, I'm exposed to radiation, but my risk is still pretty low, right? But now if I consistently get exposed to it, my risk is going to be really high. But that also doesn't mean that I'm necessarily going to experience everything bad that comes with radiation. So it's again, this idea of probabilistic sets. Is everyone following the distinction? And this is very important to understand how epidemiologists view a cause. So what is the diJerence between association versus causation. So the goal of any epidemiological study is to find causes of disease. Right. Because that is essentially the object of objective of the discipline. But oftentimes what happens is we have so many things going on when we are conducting our study with our study participants, the way we are measuring things. We oftentimes are going to fall short depending on the design that we've used. We are going to often see associations, or we are going to report our findings as associations, because there are many issues that may have not been accounted for in the design itself. In the next class, we'll be looking at diJerent study designs where I'll clarify, you know, what is a strong study design versus what is a weak study design. But for now, you need to understand that the purpose of any epidemiological studies to get to causation, because we want to identify causes. But oftentimes most of the designs, except for randomized controlled trials, which are experimental studies, are going to fall short of helping us identify a causation. So that's why we are going to use the word association. So what is the diJerence between these two terms. Again a very simple example. What does this figure show you. SPEAKER 2 Exactly. SPEAKER 0 So you see that as the ice cream sales is going up, the number of shark attacks go up. Okay. So from a public health perspective, there's something going on here. And I decided that I'm going to put this board up, that I'm going to restrict the number of ice cream sales in my community, because that's going to prevent shark attacks. Right. But is that fair? SPEAKER 2 No. SPEAKER 0 And why? SPEAKER 2 This is nothing. It's not. SPEAKER 0 So what's. What's going on? SPEAKER 2 Maybe somewhere. SPEAKER 3 There might. SPEAKER 4 Be some confounders. SPEAKER 0 Summertime. Exactly. So the weather here is to be blamed, right. Because we've seen during summertime ice cream sales go up. We've seen that the shark attacks also go up because they tend to swim in shallow waters for whatever their mating season or whatever it is. But we've seen that it's the summertime or the weather that can explain what we're seeing here, that ice cream sales is going up and shark attacks are also going up. But that doesn't mean that the ice cream sales are causing shark attacks. This is an association. It's the summer that is actually responsible or that can explain what we are seeing here. That is the idea of association. Is everybody following? SPEAKER 2 Okay. SPEAKER 0 So how would you distinguish between this. So let's say you have an exposure or risk factor denoted as x. You have a disease outcome denoted by y. And association is when x and y tend to occur together. Okay. And when you think of causation, the presence of X brings about the presence of Y. So that's the distinction between association and causation. And why do we need to, you know, learn this or care about this. Like I said, our epidemiological studies are going to have some weaknesses that prevent us from making causal inference or identifying causes. It's over a period of time body of evidence. That's when we can say that X causes Y right through more robust designs and accounting for any of the confounding, like the weather. If you don't look at the weather, we will say that ice cream sales is causing shark attacks. So that's why we rely on this term association where X and Y tend to occur together. And we need to further investigate whether X is bringing about y. Is everybody following? So there are diJerent models of causation. I've listed some of them. We are going to go over hills. Criteria for causality. Uh, germ theory. Uh, this miasma idea is pretty straightforward. Germ theory was very specific for, uh, infectious diseases. You had a bacteria, you had a virus causing, uh, a condition. But obviously the more complicated ones where we can think about chronic conditions, uh, where which are like multi, uh, causal, uh, you need to think of more, uh, better models. So Hill's criteria basically includes nine criteria. They are listed here. I'll go over some of the important ones. Uh, so Hill, uh, I don't know if you've read about it, but he was instrumental in actually, uh, getting consensus around, uh, the impact of smoking on lung cancer. Uh, he conducted a lot of studies. There was a lot of controversy at that time. Uh, physicians opposed even the idea that smoking actually causes, uh, lung cancer. And that's why he proposed these, uh, nine criteria to help us think through, uh, through diJerent, you know, results from diJerent studies, what can be considered as a cause, the associations that we are seeing, can we actually say that this is a cause of something? So he proposed this. This is not a checklist by any means. This is a guideline. Uh, it doesn't mean that, you know, uh, if X or you suspect that X causes Y, you're going to check oJ each of these criteria. If one criteria is not checked oJ, we are not going to think of this as a cause. It's just a guideline. It's not a checklist. It's not like some kind of, uh, formula that you go through. Uh, this was at that time proposed, uh, and is still used to an extent, uh, because there was so much of opposition, there was no consensus on what would be considered, uh, a causal relationship, especially when it comes to, uh, noninfectious diseases. Right. So let's look at the most important one, which is temporality, which is pretty straightforward. The exposure must precede the disease. And if you think of it simplistically, it's like for something to be a cause of something, it has to happen before it, right? For a smoking to be a cause of lung cancer, somebody has to smoke before they get lung cancer. It essentially just means that the exposure must precede the disease. And this is a very important criteria. It's diJicult to meet because with chronic conditions, let's say cardiovascular disease, hypertension, stroke, which have so many multifactorial causal mechanisms and pathways, this becomes very diJicult to tease out. Right. Sometimes. Uh, but it's but it's an important criteria that has to be met because for something to cause something, it has to happen before it. Okay. Next is strength of association, which is the magnitude of association. And that's that's where our measures of association come into play. Stronger the association more likely that it's going to be causal weaker the association, the more likely it is explained by bias, confounding or random error which we are going to cover in a couple of weeks. But again, there are exceptions that we are seeing here. So smoking and lung cancer really strong association. You see a very high association between smoking and lung cancer. But a very weak association exists between smoking and cardiovascular disease. But it is still considered a cause. So there could be exceptions to these criteria. And that's why I said that this is not a checklist of any sorts. This is just a guideline. Uh, and he proposed it. Uh, think of that time, right? When there was really no consensus around smoking and lung cancer to help us think through things. So there are going to be exceptions. Temporality obviously has no exception for common sense sake. But for something to cause something, it has to happen before it is everybody following. Dose response relationship. This is also going back to the point that you made. Higher amounts of exposure associated with higher risk of disease. You can see her mortality rate per 100,000 person years by the number of cigaret packets smoked, so the risk for never smoked is much lower as the amount of, uh, packets that you smoke per day increases, your risk for mortality increases. So this is dose response relationship. Very easy to correlate it to any chemistry experiment. Right. Like the amount of whatever reagent or catalyst that you're adding is going to give you a stronger reaction. Or think of drug dosing or anything of that sort where you see an incremental increase in in an outcome. In this case, it's a disease. Is everyone following? Replication of findings. Obviously, uh, the associations that you are observing should be demonstrated in multiple studies. And then if you see that in multiple studies, they are more likely to be causal. So consistency across multiple studies. So if x causes Y you're going to see a body of evidence around that where you're going to see that the same type of association is existing between x and y. Is everybody following. And that's when we can sort of draw consensus on that X is causing Y, because we have so many studies are showing that obviously the design of the study will matter if it is not a strong design. And if you have 100 of those, then you have to again start thinking about what are some of the weaknesses and what would make sense and explaining the association. So the association. So association basically is when x and y tend to occur together. Most of our studies, if they are not, uh, most of the observational studies, if it's not an experimental study, it's going to be this X and Y tending to occur together because it has diJerent limitations. But the idea or the ultimate goal is to get to causation, where we can identify causes of disease. And that's why we say these, uh, associations exist when we are either calculate the odds ratio or the risk ratio, and we see that something is going on between our, uh, exposure of interest and the outcome of interest. Biological plausibility is basically that there is a biological mechanism that can explain what is going on. So again, with smoking and lung cancer, you know that the chemicals in the smoke can alter or mutate your DNA, and that can cause, uh, you know, changes in the cells and, uh, that can result in lung cancer. So that's the criteria of biological plausibility, that there is some biological explanation as to why X is causing Y. So this just shows you an example. Gambling causes lung cancer. There is an association. But what do you think is going on gambling, gambling or not? Gambling gamblers are known to have this increased, uh, prevalence of smoking and that is actually causing. So there's no biological mechanism that can explain that you gambling can actually mutate your DNA, right? So that brings us to this idea of measures of disease association. The previous slides basically was to give you context as to why we call the measures of association and why we are quantifying this relationships, because the ultimate goal is to get to causation, identify causes of disease through diJerent study designs. In the next class, I'll I'll be talking more in detail about diJerent study designs, what are considered stronger designs, what are weaker and all of that okay. So a measure of association quantifies the relationship between the exposure and a disease. So you have two scales on which measures of association are reported the absolute scale and the relative scale. So as the name suggests, relative tells us the relative increase or decrease in the eJect of the exposure on the outcome. And the absolute diJerence tells us the absolute increase or decrease. So it's going to be a subtraction, whereas relative scale is going to be some type of division. Right. Because the relative change versus the absolute change. So we have these four measures on the relative scale. We have prevalence ratio which in theory can be calculated but oftentimes is not used as much. In epidemiology you have risk ratio, you have incidence rate ratio and then you have odds ratio. And we're going to learn how to calculate. Each of these is going to build on how we learned to calculate the diJerent measures of frequency. So prevalence ratio is the prevalence in the exposed divided by prevalence in the unexposed. So what we do is we calculate the prevalence in the exposed group separately. We calculate the prevalence in the unexposed group and then divide those two quantities. Like I said in theory you can calculate this but it's not used often. Next is risk ratio. This is important to know how to calculate this. So that is calculated by calculating the risk in the exposed which is your cumulative risk or cumulative incidence divided by the risk in the unexposed. It ranges from zero to positive infinity and it has no units of the same idea. You divide the population into two groups the exposed group. The unexposed group see who has the disease, who does not have the disease, and get the numerator and the denominator. It will be much clearer, clearer. When I walk you through an example. Again, you do not have to memorize the formulas, but but it will be, you know, once you work through examples, it will be it will stick the risk in the exposed over the risk in the unexposed. Same for incidence rate ratio. You have the incidence rate in the exposed divided by the incidence rate in the unexposed. It ranges from zero to positive infinity and it has no units. Odds ratio is the odds in the exposed divided by the odds in the unexposed. So now let's look at the example. So the easiest way to calculate any of these measures is to have this two by two table. And stick to this convention that I'm showing you. And you won't get the wrong answer. Not that if you don't use this convention you can you cannot calculate it. You can. This is just easy to remember where you have disease on top. So you have disease status and no disease. And over here you have your exposure status as exposed and not exposed. Then you have your numbers from whatever your study, uh, is, and then you have the totals here. Okay. So a is the number of people with disease who are exposed. What is B. Number of people without the disease but are still exposed. Only diJerence and no disease not exposed. Okay. And then the the column is just the edition okay. And across it's again just the edition. So the formula for calculating the risk ratio is a over a plus B in the exposed group. Risk in the unexposed is c over c plus d. And then you divide this. So a good way to remember this is to make a two by two table and then apply the formula. You don't have to memorize the formula, but you should know how to make the two by two table. Because you know what a, b, c, d stands for. Okay. You don't have to memorize this. You don't have to memorize any of this. This just shows you how we derived the formula. So basically odds ratio. Guys. Pay attention. Odds ratio is a cross product. It's a times D over C times B. So this is the only thing you're interested in. This just shows you how the formula was derived. Okay. So this is a cross product from the two by two table. Okay.