Causality and Experiments Lecture PDF
Document Details
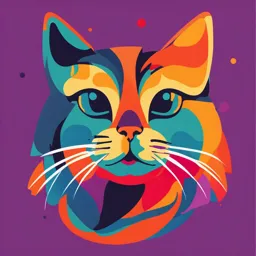
Uploaded by LongLastingGalaxy
Magnus Carlsson
Tags
Summary
This document presents a lecture on causality and experiments in economics, discussing topics such as the challenges in determining causal relationships, the concept of potential outcomes, and the use of statistical methods to analyze causal effects. It also covers the problems of selection bias and the importance of randomization in experimental design.
Full Transcript
Causality and Experiments 1 Magnus Carlsson Roadmap In the following two lectures, I will discuss: Why focus on causality? How economists view causality Why is it so difficult to estimate causal effects in the absence of experimental research design Why the random...
Causality and Experiments 1 Magnus Carlsson Roadmap In the following two lectures, I will discuss: Why focus on causality? How economists view causality Why is it so difficult to estimate causal effects in the absence of experimental research design Why the randomized trial should be regarded as a golden standard How to analyze data from experiments Why even a randomized trial sometimes fail to produce causal estimates Some examples, showing randomization at work in economics research Readings Angrist and Pischke, chapter 1 and 2 Wooldridge, chapter 1.4 Articles Why Focus on causality? In economics, the most interesting research is often about questions of cause and effect The reason is that a causal relationship is useful for making predictions about the consequences of changing policies or policies Purely descriptive research is not informative in this regard, although it may have other important roles Causal relations With casual relations as prime interest, the experimental approach takes a key role During recent decades, modern microeconomics has taken the scientific experiment as the “golden standard” for inference The experimental approach dates back already to the renaissance, where for instance Galileo conducted experiments that allowed him to test his theories of falling bodies During history, many scientific breakthroughs have occurred through experiments Causal relations Examples of questions involving causality in economics: Labour economics: ´how does participation in an active labor market program affect employment probabilities?´ Education economics: ´do smaller classes improve study results?´ Health economics: ´how does absolute/relative income affect health and mortality?´ Macroeconomics: ´what is the effect of an unanticipated change in the short-term interest rate on current and future economic activity?´ Crime economics: ´does more police reduce crime?´ Causal relations in economics The researcher is interested in obtaining the causal effect of participating in some treatment on future outcomes. In economic literature, models for analyzing causal effects are often called models for treatment/ policy/ program evaluation. Treatment is very broadly defined and may also refer to some choice variable of individuals, for example entering university education or not, getting married or not etc. An intuitive definition of causality Some action causes a particular effect if the effect would not have occurred if the action had not been performed Causality formalized We now want a more formal framework for thinking about causality Consider a population of N units. Units could be individuals, firms, countries, etc. For each unit we observe an outcome variable Y and a variable D. Assume that the variables Y and D are correlated. Does this correlation imply causation? The general answer is no. In which sense and under what assumptions can we conclude that D causes Y? Causal relations in economics Let 𝑖 denote an index for a particular unit in the population Let 𝐷𝑖 denote a “treatment” To simplify, assume that ”treatment” is either ”yes or no”. Such that: 𝐷𝑖 = 1 if unit 𝑖 is exposed to treatment 𝐷𝑖 = 0 if unit 𝑖 is not exposed to treatment 𝑌𝑖 (𝐷𝑖 ) is then the observed outcome Causal relations in economics Let us now define the potential outcomes of unit 𝑖 The potential outcome model is also referred to as the counterfactual framework. Each unit (individual, firm etc.) has two potential outcomes, 𝑌1𝑖 with treatment and 𝑌0𝑖 without treatment. Important to note: 𝑌1𝑖 and 𝑌0𝑖 refer to the potential outcomes as treated and non- treated for unit 𝑖, irrespective of wheatear or not the unit was actually treated or non-treated Examples of potential outcomes Consider an unemployed person seeking a job Assume the person could participate in a job search program 𝑌1𝑖 would refer to the potential labour market outcome if the person participated in the program 𝑌0𝑖 would refer to the potential labour market outcome if the person did not participate. Thus, these are potential outcomes that do not relate to actual treatment status Definition of a causal effect In the potential outcome framework, only one potential outcome is observed. In other words, in reality you can only either be treated or not treated! The unobserved potential outcome is also called the counterfactual outcome. For a unit, the effect of participating in the treatment equals ∆𝑖 = 𝑌1𝑖 − 𝑌0𝑖 Which is simply the difference in potential outcomes. This is also the definition of causal effect at the unit level Example of a causal effect: the effect of a job search program on employment Example: A naive comparison between unemployed workers who went to the program and unemployed workers who did not go may suggest that the program is bad for employment! But: Persons who/those workers who went to the program may have worse labour market prospects in the first place What we really would like to know are the potential outcomes: 𝑌1𝑖 : employment outcome of person 𝑖 of going to the program 𝑌0𝑖 : employment outcome of person 𝑖 not going to the program The causal effect of participating in the program is: ∆𝑖 = 𝑌1𝑖 − 𝑌0𝑖 The fundamental problem of causal interferance ∆𝑖 is unobservable, since only one of variables 𝑌1𝑖 and 𝑌0𝑖 is observed The counterfactual is inherently unobservable since units cannot receive and not receive treatment at the same time. Impossible to derive causality at the unit level! How do we ”solve” this difficult problem? “Scientific solution” Statistical solution Scientific solution to the counterfactual problem Consider a unit 𝑖 and the following assumptions: Temporal stability: The value of the outcome 𝑦𝑖 does not depend on when the treatment takes place Causal transience: The value of the outcome 𝑦𝑖 is not affected by a previous treatment Homogeneity of units: the existence of other units 𝑗 ≠ 𝑖 such that 𝑦𝑖 𝑥𝑖 = 𝑦𝑗 𝑥𝑗 for 𝑥𝑖 = 𝑥𝑗 These assumptions are used in natural sciences to infer causality Not likely to hold within the social sciences (basically since the environment is not perfectly controllable as it might be in lab) Statistical solution to the counterfactual problem The statistical solution uses methods to compute the average causal effect for the entire population or for some interesting subgroup In the economic literature an often used parameter of interest is the average treatment effect (ATE). The average treatment effect of 𝐷𝑖 = 1 describes how much on average an individual in the population benefits from receiving the treatment: 𝐴𝑇𝐸 = E ∆𝑖 = E 𝑌1𝑖 − 𝑌0𝑖 = E 𝑌1𝑖 − E 𝑌0𝑖 Here we are comparing the potential outcome when all units receive the treatment with the potential outcome when no units received treatment. Notice, neither is usually observed Average Treatment Effect on the Treated (ATET) Alternative parameter of interest in the average treatment effect on the Treated (ATET) The average treatment effect on the treated describes how much on average the individuals who actually received treatment benefit from the treatment: 𝐸 ∆𝑖 |𝐷𝑖 = 1 = 𝐸 𝑌1𝑖 − 𝑌0𝑖 |𝐷𝑖 = 1 = 𝐸 𝑌1𝑖 |𝐷𝑖 = 1 − 𝐸 𝑌0𝑖 |𝐷𝑖 = 1 (1) This still involves one unobserved counterfactual: 𝐸 𝑌0𝑖 |𝐷𝑖 = 1 , i.e. the potential outcome as untreated for those who actually received treatment! Example of ATE and ATET: Job search program In case of the job search program, the ATE answers the question: ´if all employed workers would participate in the job search program, how much would employment increase on average?´ The ATET answers the question: ´How much would employment increase on average for the workers who selected into the program?´ Both questions cannot be answered easily, because they require a comparison of the person´s observed outcome to the counterfactual outcome. Estimating ATE and ATET: the self-selection problem How can we solve the self-selection problem? What if we try to estimate the average treatment effect with: 𝐸 𝑌1𝑖 |𝐷𝑖 = 1 − 𝐸 𝑌0𝑖 |𝐷𝑖 = 0 (2) In other words, can´t we just compare the average outcomes of the ones who were actually treated and non-treated? Estimating ATE and ATET: the self-selection problem This would require that the average potential outcome as non-treated, for the actually treated ones, is the same as the average potential outcomes as non-treated for the actually non-treated ones, i.e that: 𝐸 𝑌0𝑖 |𝐷𝑖 = 1 = 𝐸 𝑌0𝑖 |𝐷𝑖 = 0 (3) Without an experiment (as we will see), this is an unlikely assumption! Example of self-selection Consider again the example of a job search assistance program One story would be that unemployed workers who are very motivated to find work are much more likely to participate in the program. BUT: Because these individuals are more motivated to find work, their potential outcomes, both as treated and untreated are probably better than the potential outcomes of less motivated unemployed workers. So, potential outcomes are not independent of actual treatment status Self-selection cont. With self-selection into the treatment participation, we have: E 𝑌0𝑖 |𝐷𝑖 = 1 ≠ E 𝑌0𝑖 |𝐷𝑖 = 0 and E 𝑌1𝑖 |𝐷𝑖 = 1 ≠ E 𝑌1𝑖 |𝐷𝑖 = 0 In other words: the potential outcomes as untreated, for the treated ones, E 𝑌0𝑖 |𝐷𝑖 = 1 , are not the same as the potential outcomes as untreated for those who were actually not treated E 𝑌0𝑖 |𝐷𝑖 = 0. Also the potential outcomes as treated, for the treated ones, E 𝑌1𝑖 |𝐷𝑖 = 1 , are not the same as the potential outcomes as treated for those who were not treated E 𝑌1𝑖 |𝐷𝑖 = 0. Formalizing the bias resulting from self- selection We can also formalize the bias arising from self selection. Again, lets say we naively try to estimate the ATE by: 𝐸 𝑌1𝑖 |𝐷𝑖 = 1 − 𝐸 𝑌0𝑖 |𝐷𝑖 = 0 (4) Now use the trick of adding and subtracting 𝐸 𝑌0𝑖 |𝐷𝑖 = 1 from the equation above. 𝐸 𝑌1𝑖 |𝐷𝑖 = 1 − 𝐸 𝑌0𝑖 |𝐷𝑖 = 1 + 𝐸 𝑌0𝑖 |𝐷𝑖 = 1 − 𝐸 𝑌0𝑖 |𝐷𝑖 = 0 (5) Formalizing the bias resulting from self- selection cont. Simplifying the terms yield: 𝐸 𝑌1𝑖 − 𝑌0𝑖 |𝐷𝑖 = 1 + 𝐸 𝑌0𝑖 |𝐷𝑖 = 1 − 𝐸 𝑌0𝑖 |𝐷𝑖 = 0 (6) Which can be written as 𝐸 𝑌1𝑖 − 𝑌0𝑖 |𝐷𝑖 = 1 + (𝑎 𝑏𝑖𝑎𝑠 𝑡𝑒𝑟𝑚) (7) This is the ATET + a bias term. Example of bias What would the bias term, 𝐸 𝑌0𝑖 |𝐷𝑖 = 1 − 𝐸 𝑌0𝑖 |𝐷𝑖 = 0 , be in our job search example? It would capture the difference in employment outcomes as non- treated between the ones who actually got treated and those who did not get treated In our example, 𝐸 𝑌0𝑖 |𝐷𝑖 = 1 − 𝐸 𝑌0𝑖 |𝐷𝑖 = 0 > 0, since those selecting treatment are more motivated to find work in the first place. Why is selection bias likely to be important? Theoretically, selection bias may result from rational actors making optimizing decisions about what markets to participate in – job, location, education, marriage, crime etc. Consider a simple model that we may call: ”I am in it, if it is worth it”: 𝐷 = 1 if 𝑌1𝑖 − 𝑌0𝑖 > 𝑐, (8) Where c denotes the monetary and mental cost of participating in the treatment. Why is selection bias likely to be important? Then we can write: E 𝑌0𝑖 |𝐷𝑖 = 1 = E 𝑌0𝑖 |𝑌0𝑖 < 𝑌1𝑖 −𝑐 E 𝑌0𝑖 |𝐷𝑖 = 0 = E 𝑌0𝑖 |𝑌0𝑖 > 𝑌1𝑖 −𝑐 (9) Then, in general: E 𝑌0𝑖 |𝐷𝑖 = 1 ≠ E 𝑌0𝑖 |𝐷𝑖 = 0 Because groups differ in terms of: Comparative advantages (𝑌1𝑖 - 𝑌0𝑖 is large for some, small for others). In the most simple instances: treatment participants have smaller 𝑌0𝑖 , thus larger potential gain Other sources of selection Selection into treatment could also be based on some administrative rule or by selection by the treatment providers. For instance: ”Cream-skimming”: or choosing ”the best”, which would suggest: E 𝑌0𝑖 |𝐷𝑖 = 1 > E 𝑌0𝑖 |𝐷𝑖 = 0 (10) Alternatively, negative selection, like putting the ”weak” kids in small classes: E 𝑌0𝑖 |𝐷𝑖 = 1 < E 𝑌0𝑖 |𝐷𝑖 = 0 (11) Key problem The key problem is thus that we cannot observe or estimate: E 𝑌0𝑖 |𝐷𝑖 = 1 , E 𝑌1𝑖 |𝐷𝑖 = 0 , 𝐸 𝑌1𝑖 , and 𝐸 𝑌0𝑖 (12) The key problem in treatment evaluation is to find something (exogenous variation) that does not affect potential outcomes but affects treatment assignment. Put differently, we want treatment status to be independent of potential outcomes in order to find a good counterfactual. In the job search example: we want the potential outcomes (employment) of the treated to be the same as the potential outcomes of the non treated. Randomization solves the selection problem In a social experiment, treatment assignment is randomized across individuals, through a lottery This implies that the treatment assignment will be statistically independent of potential outcomes (and other variables): 𝑌0𝑖 , 𝑌1𝑖 ⊥ 𝐷𝑖 In our job search example, it would mean that a lottery is used to assign unemployed persons to the job search program. Since it is random which person goes to the program, the treatment must be independent of potential outcomes Why does randomization solves the selection problem? Example In our previous job search example, one story was that motivated workers selected into treatment If we instead randomize treatment, there will on average be an equal distribution of motivated workers in both the treated and untreated group. In fact, since it is now completely random if one gets treated, the groups will look very similar on average, since there is no selection Since the only thing that differs between the groups is treatment status, the treated and non-treated groups can be used as each others counterfactuals Randomization solves the selection problem With randomization, treatment status is independent of potential outcomes and we have that: E 𝑌1𝑖 |𝐷𝑖 = 1 = E 𝑌1𝑖 |𝐷𝑖 = 0 = E[𝑌1𝑖 ] and E 𝑌0𝑖 |𝐷𝑖 = 1 = E 𝑌0𝑖 |𝐷𝑖 = 0 = E[𝑌0𝑖 ] In our job search program example, this has two important implications. Randomization solves the selection problem First, and most importantly, random assignment of 𝐷𝑖 eliminates the selection problem. We can now estimate ATET by: ATET = E 𝑌1𝑖 |𝐷𝑖 = 1 − E 𝑌0𝑖 |𝐷𝑖 = 1 E 𝑌1𝑖 |𝐷𝑖 = 1 − E 𝑌0𝑖 |𝐷𝑖 = 0 In other words, we can swap E 𝑌1𝑖 |𝐷𝑖 = 1 for E 𝑌0𝑖 |𝐷𝑖 = 0 since treatment status is now independent of potential outcomes Both E 𝑌1𝑖 |𝐷𝑖 = 1 and E 𝑌0𝑖 |𝐷𝑖 = 0 are observed Randomization makes ATE = ATET Second, the potential outcomes when all persons took the treatment (E 𝑌1𝑖 ) will now be the same as the potential outcomes on those who actually took the program E 𝑌1𝑖 |𝐷𝑖 = 1. This implies that with randomization, we have ATE = ATET. This is, again, because the treated and non treated groups are, on average, similar on all aspects despite treatment status. Their potential outcomes should therefore be the same. How important is randomization? Real world evidence In social sciences, randomization is often not possible. Instead, researchers use a control strategy, where it is assumed that one can control for all the factors that generate differences in potential outcomes between the treatment and control group (like in a OLS regression) Recently, however, there have been many occasions where both experiments and a control strategy have been applied to similar data This allow researchers to compare how well a control strategy can mimic the experimental ideal Unfortunately, studies from both medicine and economics shows that randomization outperforms a control strategy How important is randomization? Real world evidence from medicine Recent evidence comes from the evaluation of hormone replacement therapy (HRT) Evidence from Nurses Health Study, which is a large and influential nonexperimental survey of nurses, showed better health among the HRT users, using a control strategy. In contrast the results of two recently completed randomized trials on similar data shows that health risks exceeded benefits (Hsia, et al., 2006) The previously reported positive results most likely reflected that health-conscious women self-selected to treatment, which explains the positive results (remember ATET + bias term). How important is randomization? Real world evidence from economics Economics: Labour market training programs. Many non-experimental studies paradoxically find that participants in the program after the programs earn less than non-participants. Here, one may suspect selection bias, since such training programs are usually meant to serve men and woman with low earnings potential. Not surprisingly, therefore, naive comparisons of program participants with non-participants often show lower earnings for the participants. In contrast, randomized evaluations of training program generate mostly positive effects (see, e.g., Lalonde 1986; Orr, et al 1996).