Kimn10 Omics 2024 (PDF)
Document Details
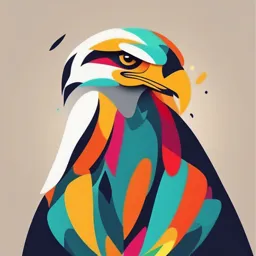
Uploaded by RoomyDravite
Lund University
2024
Fredrik Levander
Tags
Related
Summary
This document covers omics techniques for target identification and the development of biologics. It contains a lecture overview, learning objectives, and glossary related to omics, including genomics, transcriptomics, proteomics, and metabolomics.
Full Transcript
Biopharmaceuticals (2024) – Omics techniques for target identification and development of biologics [email protected] Lecture overview How omics can be used for target discovery Omics techniques (genomics, transcriptomics, proteomics and metabolomics) Analysis of omics data for...
Biopharmaceuticals (2024) – Omics techniques for target identification and development of biologics [email protected] Lecture overview How omics can be used for target discovery Omics techniques (genomics, transcriptomics, proteomics and metabolomics) Analysis of omics data for identification of targets Omics for personalised medicine Proteomics: focus and example applications Thanks to Ashfaq Ali and Magnus Jakobsson for some of the slides Learning objectives How omics can help in discovery of relevant targets and to develop biologics Conceptual understanding of some omics techniques Omics and concepts of personalised medicine Omics - studies of the entire collection of a type of molecules Genomics, Epigenomics Transcriptomics Proteomics Metabolomics OMICS glossary GENOMICS – The study of the set of genes contained in the chromosomes TRANSCRIPTOMICS – The study of the set of mRNA molecules being expressed at a given time under specified conditions PROTEOMICS – The study of the set of proteins being expressed at a given time under specified conditions and their state of modification METABOLOMICS – The study of the set of small molecules at a given time under specified conditions Phenotype Omics for target identification (target selection) Find molecular differences between healthy and disease. Samples: Patient biopsy samples, patient derived cell lines, etc. Compare groups of samples to distinguish variation due to disease from normal population variation Find out which differences are causative to pinpoint suitable targets Some techniques for Omics Genomics Whole genome Sequencing (WGS) and targeted resequencing using Next Generation Sequencing (NGS) Chip based variant detection and analyses using oligoneclotide probes Exome sequencing (NGS) Transcriptomics RNA-sequencing (NGS) cDNA microarrays Single cell sequencing (NGS) Proteomics Mass spectrometry Affinity-based proteomics Metabolomics NMR, Mass Spectrometry Metagenomics (NGS) Single-cell omics Epigenetics (NGS or microarrays) Spatial omics (Imaging and NGS) NGS –Massively parallel sequencing Cost of sequencing over time Sequencing: How does it work? https://doi.org/10.1089/wound.2012.0379 Brief about the bioinformatics workflow for NGS data Overlapping reads form contigs; contigs and gaps of known length form scaffolds. Paired end reads of next generation sequencing data mapped to a reference genome. Multiple, fragmented sequence reads must be assembled together on the basis of their overlapping areas. Detect sequence variation Third-generation sequencing (long-read sequencing) Longer reads (>1000 bases) as compared to second generation sequencing (20-400 bases). Several competitive techniques (PacBio, Oxford nanopore, etc) Facilitates mapping of reads and isoform determination, especially in difficult regions (repeats, etc) NGS applications in human health WGS, whole-genome sequencing; WES, whole-exome sequencing; Seq, sequencing; ITS, internal transcribed spacer; ChIP, chromatin immunoprecipitation; ATAC, assay for transposase-accessible chromatin; AMR, anti-microbial resistance. https://doi.org/10.3390/biology12070997 Genomics Find genome-level deviations that may be causing disease Point mutations, indels, … Copy number variations in cancers Associations between genotype and phenotype – GWAS (Genome wide association studies) – Large patient cohorts needed to obtain statistical power Transcriptomics Look at the genes that are actually expressed Techniques in Transcriptomics Transcriptomics technologies are the techniques used to study an organism’s whole transcriptome, the sum of all of its RNA transcripts. The information content of an organism is recorded in the DNA of its genome and expressed through transcription. A transcriptome captures a snapshot in time of the total transcripts present in a cell. Transcriptomics techniques include - DNA microarrays and - Next-generation sequencing technologies called RNA-Seq. - Transcription can also be studied at the level of individual cells by single-cell transcriptomics (ScRNASeq). - Spatial transcriptomics where location of expression is captured by imaging together with transcript read out using probes or NGS Increasingly popular as technologies evolve rapidly RNA-sequencing vs microarrays Source: Lowe et al (2017) PLoS Comput Biol 13(5): e1005457 https://doi.org/10.1371/journal.pcbi.1005457 Comparison of contemporary methods for bulk transcriptomics RNA-Seq Microarray Throughput 1 day to 1 week per experiment 1–2 days per experiment Input RNA amount Low ~ 1 ng total RNA High ~ 1 μg mRNA High (sample preparation and Labour intensity Low data analysis) None required, although a Reference genome/transcriptome Prior knowledge reference genome/transcriptome is required for design of sequence is useful probes >90% (limited by Quantitation accuracy ~90% (limited by sequence coverage) fluorescence detection accuracy) RNA-Seq can detect SNPsand Specialised arrays can detect mRNA splice variants (limited by splice variants (limited by probe design Sequence resolution sequencing accuracy of ~99%) and cross-hybridisation) 1 transcript per thousand 1 transcript per million Sensitivity (approximate, limited by (approximate, limited by fluorescence detection) sequence coverage) 100,000:1 (limited by 1,000:1 (limited by Dynamic range sequence fluorescence coverage) saturation) Technical reproducibility >99% >99% Data processing of analysis for target discovery using RNA-sequencing/Gene expression data QC: Filter low quality reads Alignment, count matrix Quality control on sample and gene level Need to reduce experimental noise Statistical analyses To find biologically relevant variation Systems Biology and enrichment analyses Data Visualization QC: PCA analyses for sample QC QC: Gene level fitering: Gene Dispersion estimates Data Normalisation Compensate for differences in sample amounts etc. General assumption is that most analytes do not differ between samples. Between sample normalisation log2 Global LOESS normalisation And then: Statistical tests to find significant differences Correction for multiple testing. Volcano plot Differential expression analyses – find differences between disease and control or subgroups of disese Found out if a gene is differentially expressed when comparing samples from different conditions Gene is found at higher or lower abundance (fluorescence in microarrays and read count in sequencing) Upregulated if found more in condition 2 compared to condition 1 Downregulated if found less in condition 2 compared Heatmap of gene co-expression patterns across different samples. to condition 1 (from Lowe et al https://doi.org/10.1371/journal.pcbi.1005457.g006) High expression in red, low expression in blue Gene expression varies across tissues and conditions Some genes are expressed in all tissues Others only expreesed in specific tissues/conditions One alternative is to look for interaction partners of condition/tissue specific genes Reality however is not that simple. To consider when selecting target Network Analyses and Systems Biology: How genes work together? Genes/proteins do not work alone Often many genes/proteins change expression level between conditions Networks and pathways enable us in interpreting global patterns in the data What are networks? - Networks are representations of complex systems (Cells, Tissues, Organisms) - Permit defining and studying global properties of interacting components - Give us insight not easily achieved by single gene approaches: - Comprehensive Coordinated - What is Systems Biology? Research to understanding at the level of the organism, tissue, or cell. - It’s in stark contrast to decades of reductionist biology, which involves taking the pieces apart. Pathway analyses, Visualisation for interpretation Integration with existing knowledge Gene set enrichment analyses With many genes diffentially expressed, how to pinpoint relevant hubs? Statistical methods to find pathways that contain more differentially expressed genes than expected by random Gene set enrichment analysis (GSEA) (also called functional enrichment analysis or pathway enrichment analysis). Multiple methods exist. Result is list of significant pathways or other defined gene sets Network analyses for signatures Genome scale models for organisms and tissue metabolism Complex models need accurate data Väremo et al, 2013 https://doi.org/10.3389/fphys.2013.00092 Topological analyses of networks Kiran Raosaheb Patil, and Jens Nielsen PNAS 2005;102:8:2685-2689 Things to consider Sample Size Correlation is not Bioinformatics Batch effects/randomization causation Validation Are the results reproducible and possible to replicate with other samples? Proteomics Closer to the phenotype Why bother? Isn’t RNA sequencing enough? Protein modifications (PTMs) affect protein function Protein localisation (secreted / membrane etc) Protein-protein interactions Sometimes low correlation between protein and RNA levels Omes and complexity (Bludau & Aebersold, Nat. Rev. Mol. Cell Biol, 2020) Term ”proteoform” to reflect diversity of proteins https://doi.org/10.1038%2Fnmeth.2369 Challenges 20,000 genes in the Genome but ca. 1,000,000 protein variants caused by Exon splicing, 300+ Post-translational modifications Dynamic Range Cell 106, Plasma 1012 The Dynamic Proteome Temporal (milliseconds, month) Spatial (cell, organelle), Developmental (100+ cell types in the body, years) All proteins exist in dynamic complexes This determines their function and is highly dynamic How to measure protein levels? Mass spectrometry – based analysis Affinity reagents. Antibodies (with different readouts, including MS) Basic principles of mass spectrometry Mass Spectrometer (Vacuum) Gas phase ions Ion sorting Ion detection Sample Ionization Source Analyzer Detector Mass spectrum -Peptides -Electrospray -Time-of-flight -Quadrapole -Proteins -MALDI signal -Ion traps -Orbirtrap -Metabolites -Nucleic acids m/z -etc Koichi Tananoka John B. Fenn Nobel Prize Chemistry 2002 Nobel Prize Chemistry 2002 (Fenn JB et al, Science, 1989) Mass Spectrometer (Exploris 480, Thermo scientific) A typical (bottom-up) mass spectrometry proteomic workflow The workhorse – tandem mass spectrometry Peptide fragments protein peptides ++ + + + ++ ++ + + + + + + + + ++ + Digestion Ionization Isolation Fragmentation Mass Analysis MS Isolation MS/MS m/z m/z m/z MS/MS peptide fragmentation (Plus internal ions, immonium ions, loss of water etc.) Source: http://www.matrixscience.com/help/fragmentation_help.html MS/MS of peptide: DDENVNSQPFMR Search space decreased as trypsin cleavage pattern is known K D >gi|532319|pir|TVFV2E|TVFV K S 275.3 K I 330.4 2E envelope protein K G 389.4 SIPETQKGVIFYESHGKLEHKDIPVP R S 406.5 R A 415.5 KPKANELLINVKYSGVCHTDLHAWHG K V 436.5 DWPLPVKLPLVGGHEGAGVVVGMGEN R E 443.5 VKGWKIGDYAGIKWLNGSCMACEYCE K D 461.4 K V 525.6 LGNESNCPHADLSGYTHDGSFQQYAT K Y 596.7 ADAVQAAHIPQGTDLAQVAPILCAGI K S 628.7 G 692.7 TVYKALKSANLMAGHWVAISGAAGGL R 801.8 GSLAVQYAKAMGYRVLGIDGGEGKEE K A 810.9 LFRSIGGEVFIDFTKEKDIVGAVLKA K W 813.9 K A 835.9 TDGGAHGVINVSVSEAAIEASTRYVR R E 893.1 ANGTTVLVGMPAGAKCCSDVFNQVVK R G 944.1 K Y 968.1 SISIVGSYVGNRADTREALDFFARGL K L 1013.2 VKSPIKVVGLSTLPEIYEKMEKGQIV K S 1136.2 GRYVVDTS K A 1241.4 R E 1251.4 R C 1312.4 K M 1386.6 K G 1447.6 K Y 2019.3 K L 2312.4 2418.7 Match scoring Many peptide candidates match with one or more fragments Algorithm needs to make assumptions about ion types and ion intensities, etc and generate probabilistic score Results depending on search space and not absoluely sure to be correct Large scale experiments need false discovery rate calculations – Typically target-decoy strategy. Adding equal part of reverse or random sequence proteins to search database to estimate fraction of random hits. Quantification? Peak intensities are relative to peptide levels when comparing samples. Good for relative quantification, but absolute quantification more tricky since peptides have different ionisation and fragmentation properties. Resolution of mass spectra and mass accuracy as well as dynamic range are important factors that affect quantification success. Possible to study multiple samples in one LC-MS/MS run using chemical labelling. Comparing peak intensities directly in spectra. What do to with quantitative proteomics data Similar to transcriptomics data (although RNA seq and MS proteomics data have different distributions) Differential abundance comparisons between sample groups Mapping to pathways etc Complicated by: – Mapping peptides to proteins and further to genes may be ambiguous – Post-translational modifications Benefits Possible to study relevant proteome for drug purposes, for example: – Secreted proteins (secretome) – Cell surface proteins – Phosphoproteome for signalling Omics and precision medicine Some diseases are heterogenous People are different some respond to treatment, while others don’t Use omics approaches to stratify disease and patients Can we find relevant biomarkers to measure in the clinic? Develop ’Companion diagnostics’ to identify responders, patient that risk side effects and/or to follow treatment Enable Precision medicine (personalised medicine) so each patient can get the best possible treatment Subclassification of disease using expression data Cancers are heterogenous. Subgroups may respond very differently to treatment Find markers for classification and personalised treatment Clustering of cancers using gene expression data may provide new subgroups (genetic typing can be done for known driver mutations in some cancers, but multiple mutations may have similar phenotypic effects). Breast cancer example Gene expression profiling has given rise to molecular subclassifications PAM50 classification using selected gene panel of 50 genes to identify intrinsic subtypes Used in the commercial Prosigna test – Intrinsic subtype and risk of distant recurrence – Helping in treatment decisions Selection of targeted therapies for some subtypes Immunological status may also be used Unsupervised clustering of tumor biopsies using PAM50 proteins and mRNAs Clinical markers Proteomics Transcriptomics Mosquim Junior et al, Cancers 2022, 14(23), 5761; https://doi.org/10.3390/cancers14235761 -> High diversity and complex profiles showing the need to measure multiple analytes Dynamic range problem Very high natural dynamic range of proteins Post-translational modifications at low stochiometry further complicates measuring all variants Analytical challenge for mass spectrometry-based proteomics Reference intervals for 70 protein analytes in plasma. N. Leigh Anderson, and Norman G. Anderson Mol Cell Proteomics 2002;1:845-867 © 2002 The American Society for Biochemistry and Molecular Biology Sample fractionation and new instruments help Some current figures With extensive separation and state of the art mass spectrometry 10-12000 proteins can be measured in a cell / tissue sample (24h) Can quantify 7-8000 proteins in a human cell lysate in 1-2 hours with optimal equipment. About 300-400 proteins with the same method in serum or plasma sample Dynamic range of proteins is the main problem. Can be more than 10 orders of magnitude in sample and the mass spectrometer handles ~3-4 orders of magnitude Rapid developments driven by improved lab workflows and instrumentation as well as novel software Affinity binder-based quantification Aptamer-based – Somascan technology: https://youtu.be/fg4mlG0nGLw Antibody+sequence-based. – Olink technology: https://youtu.be/itzfXoAcOe0 Dynamic range problem diminished Need specific reagents! – Quite low correlation between platforms Modified proteins may or may not change binding! Recent UK Biobank example Quantified 2923 plasma proteins (OLINK technology) in 54219 individuals in the UK biobank 14287 primary associations with genetic variants (exosome variants). Protein quantitative trait loci (pQTL) Associations with sex, age, BMI, etc, as well as diseases Available in online portal and can be used for ”development of https://doi.org/10.1038/s41586-023-06592-6 biomarkers, predictive models and therapeutics” Pan-cancer plasma proteomics https://doi.org/10.1038/s41467-023-39765-y Interaction Proteomics (Hein, Cell, 2015) (Keilhausser, MCP, 2015) (Huttlin, Cell, 2015) (Huttlin, Nature, 2017) (Luck, Nature, 2020) Interaction Proteomics – SARS-CoV-2 example https://doi.org/10.1038/s41586-020-2286-9 (Gordon, Nature, 2020) Protein-protein interactions (PPI) frequent drug targets Metabolomics Reflecting the phenotype Analytically challenging to capture all metabolites Mass spectrometry and NMR main techniques Target discovery followed by biologics development Interaction partners of biopharmaceuticals. Effect of biologics can be followed using omics Omics also useful for finding systemic side-effects. Summary Omics techniques can be used to find actionable molecular differences between sick and healthy and also further subtypes Data analysis critical Individual variation and co-variates need to be considered Complex network of biomolecules Choice of omics technique depends on nature of disease as well as availablility of samples. Rapid technology developments. Large multi-omics studies may pave the way for succesful precision medicine Linked references recommended for further reading