Introduction to Business Analytics - McGraw Hill - Chapter 2
Document Details
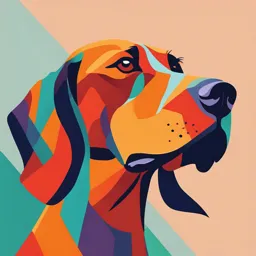
Uploaded by EnchantedOnyx3092
2022
Tags
Summary
This document is Chapter 2 of the "Introduction to Business Analytics" textbook from McGraw Hill. It covers key topics such as obtaining data, different types of data sources, and data preparation for analysis.
Full Transcript
Because learning changes everything. ® Optional: Include Cover Here Introduction to Business Analytics © 2022 McGraw Hill. All rights reserved. Authorized only for instructor...
Because learning changes everything. ® Optional: Include Cover Here Introduction to Business Analytics © 2022 McGraw Hill. All rights reserved. Authorized only for instructor use in the classroom. No reproduction or further distribution permitted without the prior written consent of McGraw Hill. © McGraw Hill LLC. All rights reserved. No reproduction or distribution without the prior written consent of McGraw Hill LLC. Obtain the Data: An Introduction to Business Data Sources Chapter 2 © 2022 McGraw Hill. All rights reserved. Authorized only for instructor use in the classroom2 No reproduction or further distribution permitted without the prior written consent of McGraw Hill. © McGraw Hill LLC. All rights reserved. No reproduction or distribution without the prior written consent of McGraw Hill LLC. Because learning changes everything. ® 2.1 2.4 Identify common internal and Understand how to prepare data for analysis. external sources of data. 2.5 2.2 Identify common tools used to prepare data for analysis. Identify the two most common formats requested for data. 2.6 Define data ethics and describe how 2.3 to gather and use personally identifiable information in an ethical Identify the different types of manner. structured data. © McGraw Hill LLC. All rights reserved. No reproduction or distribution without the prior written consent of McGraw Hill LLC. The SOAR Analytics Model 1. Specify the Question 2. Obtain the Data 3. Analyze the Data 4. Report the Results EXHIBIT 2.1 The SOAR Analytics Model 4 © McGraw Hill LLC. All rights reserved. No reproduction or distribution without the prior written consent of McGraw Hill LLC. Internal and External Data Sources LO 2.1 © 2022 McGraw Hill. All rights reserved. Authorized only for instructor use in the classroom. No reproduction or further distribution permitted without the prior written consent of McGraw Hill. 5 © McGraw Hill LLC. All rights reserved. No reproduction or distribution without the prior written consent of McGraw Hill LLC. Internal Data Sources Enterprise System (also called Enterprise Resource Planning Systems (ERP)) A Company’s Interconnected Information Systems that Connect to Each Other. Centralized Database Examples include SAP, Oracle, and Workday 6 © McGraw Hill LLC. All rights reserved. No reproduction or distribution without the prior written consent of McGraw Hill LLC. Three Basic Components of a Relational Database Relational Databases are an efficient means of storing data in one place, in one table instead of multiple places and have the following components: Tables – data organized into sets of columns (fields) and rows (records). Fields – these are the columns that contain descriptive information about the observations in the table (including primary and foreign keys). Records – these are the rows in a table; each row, or record, corresponds to a unique instance of what is being described in the table. 7 © McGraw Hill LLC. All rights reserved. No reproduction or distribution without the prior written consent of McGraw Hill LLC. How Tables Connect Together in a Relational Database Common fields connect individual tables to each other. Primary Key – Unique identifier in each table, Transaction_ID in Transaction Table; CustomerID in Customer Table Foreign Key - Exist to create relationships or links between two tables EXHIBIT 4.1 Transaction and Customers Tables 8 © McGraw Hill LLC. All rights reserved. No reproduction or distribution without the prior written consent of McGraw Hill LLC. External Data Sources Social Media Data Census Data Small Business Administration Data Publicly Available Data Financial Statements of All Publicly Traded Companies Stock Price Data Summarized Financial Data 9 © McGraw Hill LLC. All rights reserved. No reproduction or distribution without the prior written consent of McGraw Hill LLC. Example of Available External Data Sources 10 © McGraw Hill LLC. All rights reserved. No reproduction or distribution without the prior written consent of McGraw Hill LLC. The Four V’s of Big Data Volume Click stream Active/passive sensor Log Event Printed corpus Speech Social media Traditional Velocity Speed of generation Rate of analysis Variety Unstructured Semi-structured Structured Veracity Untrusted Uncleansed EXHIBIT 2.1 The Four Vs of Big Data: Volume, Variety, Velocity and Veracity Source: EY, “Big Data: Changing the Way Businesses Compete and Operate,” Insights on Governance, Risk, and Compliance, April 2014, p. 2, https://www.ey.com/ Publication/vwLUAssets/ EY_-_Big_data:_changing _the_way_businesses _operate/%24FILE/ EY-Insights-on-GRC -Big-data.pdf. 11 © McGraw Hill LLC. All rights reserved. No reproduction or distribution without the prior written consent of McGraw Hill LLC. Variety of Data Structured Data – Highly Organized Data that Fit Nicely in a Table or Database Financial Statements Database of Customer Orders and Preferences Unstructured Data – Data without Organization or Structure Blogs, tweets, pictures Semi-structured Data – Elements of Both Structured and Unstructured Data 12 © McGraw Hill LLC. All rights reserved. No reproduction or distribution without the prior written consent of McGraw Hill LLC. Progress Check 2.1 Q: How would you rate Tesla’s Financial Statements published four times a year based on the four V’s of Big Data (Volume, Variety, Velocity, and Veracity)? 13 © McGraw Hill LLC. All rights reserved. No reproduction or distribution without the prior written consent of McGraw Hill LLC. © 2022 McGraw Hill. All rights reserved. Authorized only for instructor use in the classroom. No reproduction or further distribution permitted without the prior written consent of McGraw Hill. Obtaining the Data LO 2.2 14 © McGraw Hill LLC. All rights reserved. No reproduction or distribution without the prior written consent of McGraw Hill LLC. © 2022 McGraw Hill. All rights reserved. Authorized only for instructor use in the classroom. No reproduction or further distribution permitted without the prior written consent of McGraw Hill. Data Formats Text Data Tweets, Hashtags, Word Document, etc. Tabular Data Data Structured into Rows and Columns 15 © McGraw Hill LLC. All rights reserved. No reproduction or distribution without the prior written consent of McGraw Hill LLC. File Formats Data usually delivered as comma-separated files with the file extension.csv .csv files store data as text, but they convert rapidly to a tabular format when they are imported into spreadsheet applications or used with programming languages. .csv files do not have the same row and column limits that Excel has, which allows.csv files to hold Big Data that exceed Excel’s size limit. 16 © McGraw Hill LLC. All rights reserved. No reproduction or distribution without the prior written consent of McGraw Hill LLC. Level of Aggregation How do you want it? Aggregated Data Data Already Processed and Transformed Already Combined into Subtotals, Counts, Sums or Averages Raw Data Give the Analyst the Flexibility to Process Data as They See Fit 17 © McGraw Hill LLC. All rights reserved. No reproduction or distribution without the prior written consent of McGraw Hill LLC. Progress Check 2.2 Q. When would the analyst prefer raw data to aggregated data? 18 © McGraw Hill LLC. All rights reserved. No reproduction or distribution without the prior written consent of McGraw Hill LLC. © 2022 McGraw Hill. All rights reserved. Authorized only for instructor use in the classroom. No reproduction or further distribution permitted without the prior written consent of McGraw Hill. Structured Data Types: Categorical versus Numerical LO 2.3 19 © McGraw Hill LLC. All rights reserved. No reproduction or distribution without the prior written consent of McGraw Hill LLC. © 2022 McGraw Hill. All rights reserved. Authorized only for instructor use in the classroom. No reproduction or further distribution permitted without the prior written consent of McGraw Hill. Data Types Categorical - tend to be Numerical - meaningful represented by words – numbers, such as such as categorizing a transaction amount, net group of people by income, age, or the score gender (male, female, on an exam. nonbinary), or categorizing transaction types (sales versus returns). 20 © McGraw Hill LLC. All rights reserved. No reproduction or distribution without the prior written consent of McGraw Hill LLC. Categorical Data Nominal Data – Categorical data that cannot be ranked Gender – Male or Female Transaction Type – Sale or Return Location of Sale EXHIBIT 2.9 Analysis of Categorical Data (Online vs. In-Person Transactions Summarize by Counting and grouping Proportion 21 © McGraw Hill LLC. All rights reserved. No reproduction or distribution without the prior written consent of McGraw Hill LLC. Categorical Data Ordinal Data – Categorical data that allows/implies ranking and sorting Gold, Silver and Bronze Survey Answers: Agree, Indifferent, Disagree Transaction Dates Summarize by Counting and grouping EXHIBIT 2.10 Count of Transactions by Date Proportion Ranking (Because Ordinal Data is Ranked) 22 © McGraw Hill LLC. All rights reserved. No reproduction or distribution without the prior written consent of McGraw Hill LLC. Numerical Data Interval Data – an equal Ratio Data – numerical interval between each data with an equal and observation, so that not only definitive ratio between does summing the data make each data point and sense, so does multiplication absolute “zero” (meaning and other more complex absence of) in ratio data is numerical calculations. the point of origin. SAT Scores Height, Weight Fahrenheit Temperature Most accounting Scale figures, sales, net income, depreciation Summarize by expense Counting and grouping Kelvin Temperature Proportion Scale Summing Averaging 23 © McGraw Hill LLC. All rights reserved. No reproduction or distribution without the prior written consent of McGraw Hill LLC. Tide PODS and Data Types 24 © McGraw Hill LLC. All rights reserved. No reproduction or distribution without the prior written consent of McGraw Hill LLC. Progress Check 2.3 Q: In general, how do categorical and numerical data differ? Why is it important to collect both types of data to perform your analysis? 25 © McGraw Hill LLC. All rights reserved. No reproduction or distribution without the prior written consent of McGraw Hill LLC. Preparing Data for Analysis LO 2.4 26 © McGraw Hill LLC. All rights reserved. No reproduction or distribution without the prior written consent of McGraw Hill LLC. © 2022 McGraw Hill. All rights reserved. Authorized only for instructor use in the classroom. No reproduction or further distribution permitted without the prior written consent of McGraw Hill. Preparing Data for Analysis Ensure Data Quality Data Types for each Attribute are Appropriate Validate the Data for Completeness and Integrity Were the Data Completely Extracted from Original Source? Were any Data Manipulated or Tampered during Extraction Process? Cleanse the Data Use Trim and Clean Function to Prepare the Data for Analysis Address Missing Data Perform Preliminary Exploratory Analysis 27 © McGraw Hill LLC. All rights reserved. No reproduction or distribution without the prior written consent of McGraw Hill LLC. Preparing Data for Analysis 28 © McGraw Hill LLC. All rights reserved. No reproduction or distribution without the prior written consent of McGraw Hill LLC. Different Functions to Trim and Clean the Data Trim Functions Remove White Space on Either Side of a Cell of Text Clean Functions Remove Nonprintable Characters 29 © McGraw Hill LLC. All rights reserved. No reproduction or distribution without the prior written consent of McGraw Hill LLC. Three Choices Regarding Missing Values Leave the Missing Values As Is. Remove the Records that Have Missing Values. Impute Values to Replace the Missing Values. 30 © McGraw Hill LLC. All rights reserved. No reproduction or distribution without the prior written consent of McGraw Hill LLC. Progress Check 2.4 Q. What is the Difference Between Data Completeness and Data Integrity? Why are Both Important? 31 © 2022 McGraw Hill. All rights reserved. Authorized only for ©instructor McGraw Hill useLLC. in All therights reserved. NoNo classroom. reproduction or distribution reproduction without or further the prior written distribution consent ofwithout permitted McGraw Hill theLLC. prior written consent of McGraw Hill. Tools Used to Prepare Data for Analysis LO 2.5 32 © McGraw Hill LLC. All rights reserved. No reproduction or distribution without the prior written consent of McGraw Hill LLC. © 2022 McGraw Hill. All rights reserved. Authorized only for instructor use in the classroom. No reproduction or further distribution permitted without the prior written consent of McGraw Hill. Available Software Tools ► Microsoft Excel – basic data analysis ► Tableau – basic data visualizations ► Microsoft Power BI – basic data visualizations ► Microsoft Power Query – Used to Manipulate and Clean Data in Preparation for Analysis ► Tableau Prep – Used to Manipulate and Clean Data in Preparation for Analysis ► Alteryx – Used to Manipulate and Clean Data in Preparation for Analysis ► Open-source Tools – Free Software Tools Used with Tableau and Excel ► Gretl – Primary used to Run Statistical Tests on the Data ► R and Python – Programming Languages Used to Clean Data and Perform Data Analysis 33 © McGraw Hill LLC. All rights reserved. No reproduction or distribution without the prior written consent of McGraw Hill LLC. Progress Check 2.5 Q: Which software tools emphasize data preparation vs. data visualization? 34 © McGraw Hill LLC. All rights reserved. No reproduction or distribution without the prior written consent of McGraw Hill LLC. Gathering and Protecting Data Ethically LO 2.6 35 © McGraw Hill LLC. All rights reserved. No reproduction or distribution without the prior written consent of McGraw Hill LLC. © 2022 McGraw Hill. All rights reserved. Authorized only for instructor use in the classroom. No reproduction or further distribution permitted without the prior written consent of McGraw Hill. Definition of Data Ethics Data Ethics refers to the moral responsibility associated with gathering, using and protecting personally identifiable information. 36 © McGraw Hill LLC. All rights reserved. No reproduction or distribution without the prior written consent of McGraw Hill LLC. Gathering Data Questions a Company Must Address to Handle Data Ethically Does the company send a privacy notice to individuals when their personal data are collected? Can individuals opt out of personal data collection? Do the company’s third-party data providers follow ethical practices when gathering and sharing sensitive data? 37 © McGraw Hill LLC. All rights reserved. No reproduction or distribution without the prior written consent of McGraw Hill LLC. Protecting Data Questions a Company Must Address to Handle Data Ethically If credit card information is taken, what assurance do customers have that their credit card number will be protected? Does the company keep the data secure and private, and does it have safeguards in place to protect the data? Has the company established effective practices to mitigate the risks of data misuse? Are penalties enforced for data misuse? 38 © McGraw Hill LLC. All rights reserved. No reproduction or distribution without the prior written consent of McGraw Hill LLC. Progress Check 2.6 Q. Why would an address be considered personally identifiable information? 39 © 2022 McGraw Hill. All rights reserved. Authorized only for ©instructor McGraw Hill useLLC. in All therights reserved. NoNo classroom. reproduction or distribution reproduction without or further the prior written distribution consent ofwithout permitted McGraw Hill theLLC. prior written consent of McGraw Hill. Labs Associated with Chapter 2 Lab # Lab Name 2.1 Excel: Identifying and Working with Different Data Types 2.2 Tableau: Preparing Different Data Types for Analysis 2.2 Power BI: Preparing Different Data Types for Analysis 2.3 Tableau: Conducting Preliminary Data Analysis 2.3 Power BI: Conducting Preliminary Data Analysis 2.4 Excel: Aggregating and Visualizing Different Data Types © McGraw Hill LLC. All rights reserved. No reproduction or distribution without the prior written consent of McGraw Hill LLC.