Stat 5870 Key Points and Formulae (Week 6) PDF
Document Details
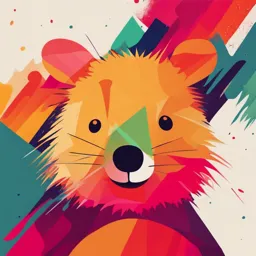
Uploaded by BreathtakingNewOrleans
Iowa State University
Tags
Summary
This document provides key points and formulas for statistical methods, including hypothesis testing and model comparison. It covers various statistical concepts and applications.
Full Transcript
Stat 5870: Key points and formulae Week 6 Importance of the assumptions / consequences of violating them: (reminder) Independence: crucial / wrong se so wrong p-value, wrong ci. Equal variance: depends on equality of sample sizes / when unequal, wro...
Stat 5870: Key points and formulae Week 6 Importance of the assumptions / consequences of violating them: (reminder) Independence: crucial / wrong se so wrong p-value, wrong ci. Equal variance: depends on equality of sample sizes / when unequal, wrong se Normality: low when same shape. Outliers always a concern A: Overview of last 5 weeks, how to choose a method Expanded version of the book’s process in section 3.4. Consistent with the book’s recommendations where they overlap Goal is to provide a justifiable analysis. No single right way to choose. My suggestion based on: Question → (Design) → Data → Analysis (makes assumptions) which we saw in the first week My suggested approach: What is the question? Differences in location (mean, median)? Differences in spread? What is the study design? experimental or observational? (i.e., causal conclusions?) Are data paired or not? Any concerns about independence: eu = ou? serial effects? Do I want a confidence interval? or just a p-value? What assumptions are reasonable (or not badly violated)? skewed distribution of errors? apparent outliers? reasonably equal variances? If you want a ci, use t-based methods, transform responses to improve assumptions If assumptions are good, perhaps after transformation use a t-test If assumptions are not good even after transformation use a non-parametric test (on ranks) or randomization test (on data values) If some observations are censored, or want resistance to outliers use a non-parametric test (on ranks) This is the end of the material covered on midterm I. 1 Stat 5870: Key points and formulae Week 6 Seriously non-normal data: Response is Yes/No (Bernoulli data) or a count (0, 1, 2, · · · ) Discrete responses: 1/0 for Yes/No, integers for counts If statistics is a collection of named methods, need lots of new names General principles are identical to what we’ve already seen (or will see) details are different computing much harder, but that’s what computers take care of B: Equality of two proportions: example Vit C study (Case study 18.2), notation: Treatment # not # cold Row total Placebo 76 335 R1 = 411 Vit. C 105 302 R2 = 407 Col. total C1 = 181 C2 =637 N = 818 Bernoulli data: Response is Yes or No Focus (usually) on proportion of Yes (or No) within a group Proportion = # Yes / # tries Common to code Yi = 1 (Yes) or 0 (No) Then proportion is the average Yi , p = ΣYi /N Percent = 100 × proportion Precision: q depends on population proportion, π: se p = π(1−π) N Not constant! (big difference from normally distrib. data) largest when π = 0.5 q (see figure on board) estimate of se p = p(1−p)N (plug-in p for π) Inference: ci for π: p ± z1−α/2 se p se computed using p, i.e., plugging p into se formula 95% ci: z0.975 = 1.96 Endpoints can be < 0 or > 1. Lots of other ways to compute CI for a proportion One group, test π = π0 : Z = (p − π0 )/se p se computed using π0 , i.e., plugging π0 into se formula both use z scores, not t scores, because not estimating s Z has a normal distribution with mean 0, variance 1 equivalent to T distribution with ∞ d.f. Bernoulli and Binomial distributions: Two different ways of describing yes/no data 1) Focus on individuals: Y = 1 or 0 i.e. event happened (1) or it didn’t (0) This is a Bernoulli distribution. 2 Stat 5870: Key points and formulae Week 6 Has 1 parameter, the probability of the event, π Y ∼ Bernoulli(π) 2) Focus on number of times an event “happens” out of N “tries” This is a Binomial distribution Z ∼ Binomial(N, π) If N individuals have the same π, number of events is: N X Z= Yi ∼ Binomial (N, π) i=1 Tests of whether two groups have same proportion, i.e., π1 = π2 : Could construct a Z test of π1 − π2 = 0 Need to compute se π̂1 − π̂2 when Ho true, i.e., π1 = π2 Requires P[cold] ignoring treatment group, use total # colds, total # individuals In terms of above table, overall P[cold] = π̂ = C2 /N Chi-square test of equal proportions Chi-square test uses model comparison, instead of a Z test for one parameter Simpler way to do the computations Generalizes to more than 2 groups (or more than 2 responses) C: Model comparison, using T-test as example: Model I: two groups have the same population mean Group A YAi = µ + εAi Group B YBi = µ + εBi Model II: two groups have different population means Group A YAi = µA + εAi Group B YBi = µB + εBi Model I expresses the null hypothesis of the test Model II: expresses “not the null hypothesis” Model II is more flexible, will always fit as well or better never worse than model I If Ho is true, model II will fit a little bit better than Model I If Ho is false (means not the same) model II will fit a lot better than model I For normally distributed data, use Sums-of-squared errors as measure of fit Leads to an F test Will see all the details when we cover ANOVA and F tests 3 Stat 5870: Key points and formulae Week 6 Model comparison, for yes/no responses: Model I: two groups have the same proportion of Yes (= had a cold) Vit C Y1i ∼ Bernoulli(π) Control Y2i ∼ Bernoulli(π) Model II: two groups have different proportions of Yes Vit C Y1i ∼ Bernoulli(π1 ) Control Y2i ∼ Bernoulli(π2 ) Or: Model I: two groups have the same proportion of Yes (= had a cold) Vit C Z1 ∼ Binomial(N1 , π) Control Z2 ∼ Binomial(N2 , π) Model II: two groups have different proportions of Yes Vit C Z1 ∼ Binomial(N1 , π1 ) Control Z2 ∼ Binomial(N2 , π2 ) Use Chi-square statistic as measure of fit Because Bernoulli or Binomial data have different properties than Normal data se π̂ not constant, not dependent on a separately estimated s D: Chi-square statistics: Compare observed counts to what is expected given a model Observed counts and notation Treatment # not # cold Row total Placebo O11 O12 R1 Vit. C O21 O22 R2 Col. total C1 C2 N Expected cell counts when Ho true (π = π1 = π2 ) Treatment # not # cold Row total Placebo E11 = R1 (1 − π) E12 = R1 π R1 Vit. C E21 = R2 (1 − π) E22 = R2 π R2 Col. total C1 C2 N Remember when Ho is true, estimate π̂ = C2 /N , the overall proportion of the Cold event 4 Stat 5870: Key points and formulae Week 6 Treatment # not # cold Row total Placebo E11 = R1 C1 /N E12 = R1 C2 /N R1 Vit. C E21 = R2 C1 /N E22 = R2 C2 /N R2 Col. total C1 C2 N Logic: Ho is π1 = π2. Reject when observed counts (Oij ) are far from their expected counts (Eij ) Use Chi-square statistic as a measure of fit X (Oij − Eij )2 C= ij Eij Similar to sum of squares; denominator accounts for unequal variance Model comparison: Full model: Two P[cold], one for placebo, one for Vit. C, Fits perfectly, C = 0 Reduced model: One P[cold] = π, C computed as above Large values ⇒ observed far from expected, reject Ho Theory: when π1 = π2 and sample size sufficiently large, C ∼ χ2k Chi-square distribution with k df df = (# Rows - 1) (# Cols -1 ) When is sample size sufficiently large? Common advice: all Eij ≥ 1 and most (80% +) ≥ 5 When sample size not large, usual small sample procedure is Fisher’s exact test Optional: demonstration that C = 0 for the full model (two P[cold]) Will show that under the full model Eij = Oij for every cell Under the full model, P[cold — group ] = proportion of colds in a group O12 /R1 for placebo group O22 /R2 for Vit C group Substituting into table of expected counts: Treatment # not # cold Row total Placebo E11 = R1 O11 /R1 E12 = R1 O12 /R1 R1 Vit. C E21 = R2 O21 /R2 E22 = R2 O22 /R2 R2 Col. total C1 C2 N Treatment # not # cold Row total Placebo E11 = O11 E12 = O12 R1 Vit. C E21 = O21 E22 = O22 R2 Col. total C1 C2 N 5 Stat 5870: Key points and formulae Week 6 E: Sampling models: How to obtain the data in above table; three common ways Prospective Binomial sample: e.g., Vit C data Two (or more) groups, then observe events, estimate P[event | group] Retrospective Binomial sample: e.g., case-control study (Case study 18.3). Especially useful when event is rare Sample C1 events and C2 , observe group for each can estimate P[group | event] but not P[event | group] (without more info) can estimate odds ratio (see below) Multinomial sample: e.g. genetic linkage study observe 4 groups defined by row and column labels Q concerns independence of the row and column classifications These differ by what is fixed by the design Prospective Binomial: Number in each group (row totals) Retrospective Binomial: Number of events and non-events (column totals) Multinomial: Total number of subjects (only N ) There are still more sampling models, but they are much less frequently used Theory ⇒ use Chi-square test for all three (when sample size large) Different small sample methods F: Odds ratios to describe differences in two proportions: Difference, p1 − p2 , has issues when applied to a wide range of populations Vit. C: P[cold | Placebo] = 0.82, P[cold | Vit. C] = 0.74, Estimated diff. is 8% What if a year or place when colds infrequent, e.g. 6% on placebo. Would estimate P[cold | Vit.C] in that place as 6% - 8% = −2% ??? Odds ratios quantify relationship between two proportions that is applicable across a wide range of baseline proportions Odds = π/(1 − π). related to betting: horse is a 2:1 favorite. range from 0 (Prob = 0) to ∞ (Prob = 1) Odds = 1 ⇒ Prob = 0.5 Statistical analysis commonly uses log odds range from −∞ (Prob = 0) to ∞ (Prob = 1) log Odds = 0 ⇒ Prob = 0.5 Odds ratio: comparison between two groups π1 (1 − π2 ) Odds1 /Odds2 = (1 − π1 )π2 Odds ratio = 1 when proportions equal, > 1 when π1 > π2 commonly use log odds ratio: = 0 when Odds1 = Odds2 or π1 = π2 6 Stat 5870: Key points and formulae Week 6 G: Inference for log odds ratio O12 O21 estimate = log O11 O22 Vit C study: Choose to use log odds of cold in placebo - log odds of cold in VitC odds of cold in placebo = O12 /O11 = 335/76 = 4.41 odds of cold in Vit C = O22 /O21 = 2.88 log odds ratio = log (4.41/2.88) = log 1.53 = 0.43 Easy to misinterpret as odds of “not cold’ in placebo vs in vitC or odds of cold in Vit C vs in placebo Sign q difference (+ or -1). If it matters, I check against proportions 1 1 1 1 √ se ≈ O11 + O12 + O21 + O22 = 0.029 = 0.17 ci for log odds ratio: estimate ±z1−α/2 se 95% ci: z0.975 = 1.96 exponentiate to get ci for odds ratio On log odds scale: 0.43 ± (1.96)(0.17) = (0.096, 0.76) For odds ratio: (exp(0.096), exp(0.76)) = (1.10, 2.14) 7