Geog 380: Geospatial Communication Data Measurement & Classification PDF
Document Details
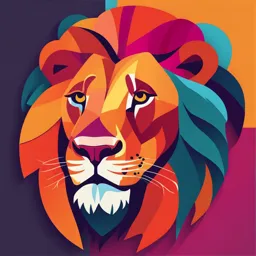
Uploaded by HotInfinity5397
2023
Geoffrey Hay
Tags
Related
- Geog 380: Geospatial Communication Data Measurement and Classification PDF
- Geog 380: Geospatial Communication Mapping Techniques PDF
- Geog 380: Geospatial Communication PDF
- Geog 380: Geospatial Communication - Vector Analysis PDF
- Geog 380: Geospatial Communication Topic 14: GNSS PDF
- Geog 380: Geospatial Communication PDF
Summary
These are lecture notes for a geospatial communication course, focusing on data measurement and classification. The notes cover topics like feature dimensionality, measurement levels (nominal, ordinal, interval, ratio), histograms, modality, symmetry, data standardization, and various classification methods.
Full Transcript
Geog 380: Source: https://earth.nullschool.net Geospatial Communication Topic 05: Data Measurement and Classification © Geoffrey Hay (2023) Next few topics Topic 04: Topic 05: Topic 06: Map types/techniques Data measurement and classification Colour and Symbology GEOG 380 (Topic 05) 2...
Geog 380: Source: https://earth.nullschool.net Geospatial Communication Topic 05: Data Measurement and Classification © Geoffrey Hay (2023) Next few topics Topic 04: Topic 05: Topic 06: Map types/techniques Data measurement and classification Colour and Symbology GEOG 380 (Topic 05) 2 Learning outcomes By the end of this topic and associated readings, a successful student will be able to: § Describe how some univariate classification techniques operate § Describe these techniques’ limitations and strengths GEOG 380 (Topic 05) 3 Feature dimensionality § Point – 0-D § Has location – is infinitely small § Line: 1-D § Has length § Polygon/region/area: 2-D § Has length and width https://www.slideshare.net/iivanoo/mission-planning-of-autonomous-quadrotors § ‘Functional surface’: 2.5-D § Continuous surface which can have only one z-value of an attribute for any x, y location (e.g., elevation) § Volumes: 3-D § Can have multiple values of attribute at each x,y location § Or, in other words, has an attribute for each x,y,z location GEOG 380 (Topic 04) 4 Feature measurement level § Nominal: § Names, labels, and categories with no assumptions made regarding relationship between categories § Place names, addresses, land cover class § Ordinal: § Numbers or values represent rank order (e.g., greater than/lesser than), but nothing more § Soil quality, crown closure class, “best places to live” § Interval: § Additions/subtractions are meaningful, but zero is arbitrary § Fahrenheit and Celsius scales of temperature Field 2018; Pg. 273 § Ratio: § Zero is not arbitrary, and ratios make sense § snow depth, mass, Kelvin scale of temperature § Cyclic § Wind direction, phenology Types of Data: https://www.youtube.com/watch?v=hZxnzfnt5v8 5 What is a histogram? § § Is a graph showing the number or frequency of measurements/ observations plotted against the range of observations for a single variable An important data exploration and summary tool Fig 7.11c; Lillesand et al. (2004) § Gives us a graphical representation of the distribution of observations for a single variable GEOG 380 (Topic 05) 6 Modality and symmetry § Symmetrical distribution § Mode, median, and mean are coincident § Modality Figs 3.1 and 3.2; McGrew and Monroe (2000) § When there are more than one value with a high frequency § Greatly impacts the use of median and mean measures GEOG 380 (Topic 05) 7 To Standardize or not to Standardize? Slocum et al. 2005, Figure 16.18 GEOG 380 (Topic 05) 8 Data Standardization § ArcGIS Pro refers to this as “normalization” § Raw totals - numerator (above the fraction line) are standardized against a denominator (below the fraction line) § Population vs. Population Density § In this example, the Jenks optimization method (a data clustering method) is used – also known as the goodness of variance fit (GVF). It is used to minimize the squared deviations of the class mean. That is: it seeks to reduce the variance within classes and maximize the variance between classes. GEOG 380 (Topic 05) 9 Standardization: further considerations § Not all variables need to be standardized § If it’s already an average, does not need to be standardized § Results can be proportions or percentages https://www.edplace.com/blog/home_learning/fractions-decimals-and-percentages § You must indicate this on your map! § Best to convert proportions to percentage (hint: format labels) § For example: Major repairs (60) / Total number of occupied private dwellings by condition of dwelling (600) = 0.1 or 10% GEOG 380 (Topic 05) 10 Data Classification Considerations § § § § https://utkuufuk.com/2018/06/03/one-vs-all-classification/ Grouping of numerical data into classes for mapping Each class is represented by an individual symbol Class interval: where to put breaks in the data Number of intervals: § Typically between 4-7 § Rarely over 10 § § GEOG 380 (Topic 05) Difficult to create distinguishable symbols if # of intervals too high Classifying to generalize and structure a distribution 11 Common methods of univariate data classification § § § § § § § Equal intervals Quantiles Mean – standard deviation Maximum breaks (aka Defined interval) Natural breaks Jenks (optimal?) Geometrical interval https://www.analyticsvidhya.com/blog/2020/07/univariate-analysisvisualization-with-illustrations-in-python/ GEOG 380 (Topic 05) univariate data: consists of observations on only a single characteristic or attribute. 12 https://pro.arcgis.com/en/pro-app/latest/help/mapping/layer-properties/data-classification-methods.htm Univariate classification in ArcGIS Pro GEOG 380 (Topic 05) 13 Slocum et al. 2005; Table 5.2 Classification example: Foreign-born in Florida GEOG 380 (Topic 05) 14 Slocum et al. 2005; Fig 5.4 Slocum et al. 2005; Fig 5.2 Equal Intervals § Equal intervals/steps along the number line Slocum et al. 2005; Figs 5.2 & 5.4 § Calculation of classes: § Determine data range § Divide by number of classes GEOG 380 (Topic 05) 16 Quantiles § Each class contains the same number of observations/values § Calculation of classes: Slocum et al. 2005; Figs 5.2 & 5.4 § Determine number of observations/values § Divide by number of classes GEOG 380 (Topic 05) 17 Mean-Standard Deviation § Derive classes from descriptive statistics of overall data distribution § Calculation of classes: Slocum et al. 2005; Figs 5.2 & 5.4 § Calculate mean and standard deviation § Compute class limits by adding/subtracting multiples of the standard deviation GEOG 380 (Topic 05) 18 Maximum Breaks (or Defined Interval) § Derive classes from groups of similar data values according to local criterion § Calculation of classes: Slocum et al. 2005; Figs 5.2 & 5.4 § Order data from low to high § Calculate differences between adjacent values § Use largest differences as class breaks GEOG 380 (Topic 05) 19 Natural Breaks § Subjective, visual/manual determination of logical breaks in data distribution in dispersion graph or histogram § Calculation of classes: Slocum et al. 2005; Figs 5.2 & 5.4 § Minimize differences within classes and maximize differences between classes GEOG 380 (Topic 05) 20 Geometric(al) interval § § § § Class breaks are based on a geometric series Good for highly skewed data Usually written as: a + ar + ar2 + ar3 + ar4 +… § a is the coefficient for each term § r is the common ratio § 2 + 6 + 18 + 54 + … § 2 is the coefficient § 3 is the ratio GEOG 380 (Topic 05) 21 Optimal (multiple techniques) Slocum et al. 2005; Figs 5.2 & 5.4 § Computational approaches to minimizing classification error § Fisher-Jenks/Jenks Natural breaks/Jenks optimal method is the most common § This method seeks to reduce the variance within classes and maximize the variance between classes GEOG 380 (Topic 05) 22 Rating of Classification Methods Slocum et al. 2005; Fig 5.7 § Rating system depends on map user’s knowledge and map purpose GEOG 380 (Topic 05) 23