Geog 380: Geospatial Communication Data Measurement and Classification PDF
Document Details
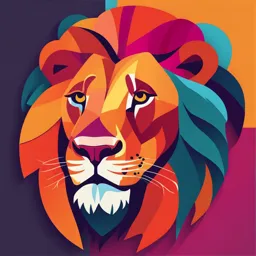
Uploaded by HotInfinity5397
2023
Geoffrey Hay
Tags
Related
- Geog 380: Geospatial Communication Data Measurement & Classification PDF
- Geog 380: Geospatial Communication Mapping Techniques PDF
- Geog 380: Geospatial Communication PDF
- Geog 380: Geospatial Communication - Vector Analysis PDF
- Geog 380: Geospatial Communication Topic 14: GNSS PDF
- Geog 380: Geospatial Communication PDF
Summary
This document details a lecture on geospatial communication, specifically focusing on data measurement and classification for geographical data. Methods like univariate classification are discussed and presented visually.
Full Transcript
Geog 380: Source: https://earth.nullschool.net Geospatial Communication Topic 05: Data Measurement and Classification © Geoffrey Hay (2023) Next few topics Topic 04: Topic 05: Topic 06: Map types/techniques Data measurement and classification Colour and Symbology GEOG 380 (Topic 05) 2...
Geog 380: Source: https://earth.nullschool.net Geospatial Communication Topic 05: Data Measurement and Classification © Geoffrey Hay (2023) Next few topics Topic 04: Topic 05: Topic 06: Map types/techniques Data measurement and classification Colour and Symbology GEOG 380 (Topic 05) 2 Learning outcomes By the end of this topic and associated readings, a successful student will be able to: § Describe how some univariate classification techniques operate § Describe these techniques’ limitations and strengths GEOG 380 (Topic 05) 3 Feature dimensionality § Point – 0-D § Has location – is infinitely small § Line: 1-D § Has length § Polygon/region/area: 2-D § Has length and width https://www.slideshare.net/iivanoo/mission-planning-of-autonomous-quadrotors § ‘Functional surface’: 2.5-D § Continuous surface which can have only one z-value of an attribute for any x, y location (e.g., elevation) § Volumes: 3-D § Can have multiple values of attribute at each x,y location § Or, in other words, has an attribute for each x,y,z location GEOG 380 (Topic 04) 4 Feature measurement level § Nominal: § Names, labels, and categories with no assumptions made regarding relationship between categories § Place names, addresses, land cover class § Ordinal: § Numbers or values represent rank order (e.g., greater than/lesser than), but nothing more § Soil quality, crown closure class, “best places to live” § Interval: § Additions/subtractions are meaningful, but zero is arbitrary § Fahrenheit and Celsius scales of temperature Field 2018; Pg. 273 § Ratio: § Zero is not arbitrary, and ratios make sense § snow depth, mass, Kelvin scale of temperature § Cyclic § Wind direction, phenology Types of Data: https://www.youtube.com/watch?v=hZxnzfnt5v8 5 What is a histogram? § § Is a graph showing the number or frequency of measurements/ observations plotted against the range of observations for a single variable An important data exploration and summary tool Fig 7.11c; Lillesand et al. (2004) § Gives us a graphical representation of the distribution of observations for a single variable GEOG 380 (Topic 05) 6 Modality and symmetry § Symmetrical distribution § Mode, median, and mean are coincident § Modality Figs 3.1 and 3.2; McGrew and Monroe (2000) § When there are more than one value with a high frequency § Greatly impacts the use of median and mean measures GEOG 380 (Topic 05) 7 To Standardize or not to Standardize? Slocum et al. 2005, Figure 16.18 GEOG 380 (Topic 05) 8 Data Standardization § ArcGIS Pro refers to this as “normalization” § Raw totals - numerator (above the fraction line) are standardized against a denominator (below the fraction line) § Population vs. Population Density § In this example, the Jenks optimization method (a data clustering method) is used – also known as the goodness of variance fit (GVF). It is used to minimize the squared deviations of the class mean. That is: it seeks to reduce the variance within classes and maximize the variance between classes. GEOG 380 (Topic 05) 9 Standardization: further considerations § Not all variables need to be standardized § If it’s already an average, does not need to be standardized § Results can be proportions or percentages https://www.edplace.com/blog/home_learning/fractions-decimals-and-percentages § You must indicate this on your map! § Best to convert proportions to percentage (hint: format labels) § For example: Major repairs (60) / Total number of occupied private dwellings by condition of dwelling (600) = 0.1 or 10% GEOG 380 (Topic 05) 10 Data Classification Considerations § § § § https://utkuufuk.com/2018/06/03/one-vs-all-classification/ Grouping of numerical data into classes for mapping Each class is represented by an individual symbol Class interval: where to put breaks in the data Number of intervals: § Typically between 4-7 § Rarely over 10 § § GEOG 380 (Topic 05) Difficult to create distinguishable symbols if # of intervals too high Classifying to generalize and structure a distribution 11 Common methods of univariate data classification § § § § § § § Equal intervals Quantiles Mean – standard deviation Maximum breaks (aka Defined interval) Natural breaks Jenks (optimal?) Geometrical interval https://www.analyticsvidhya.com/blog/2020/07/univariate-analysisvisualization-with-illustrations-in-python/ GEOG 380 (Topic 05) univariate data: consists of observations on only a single characteristic or attribute. 12 https://pro.arcgis.com/en/pro-app/latest/help/mapping/layer-properties/data-classification-methods.htm Univariate classification in ArcGIS Pro GEOG 380 (Topic 05) 13 Slocum et al. 2005; Table 5.2 Classification example: Foreign-born in Florida GEOG 380 (Topic 05) 14 Slocum et al. 2005; Fig 5.4 Slocum et al. 2005; Fig 5.2 Equal Intervals § Equal intervals/steps along the number line Slocum et al. 2005; Figs 5.2 & 5.4 § Calculation of classes: § Determine data range § Divide by number of classes GEOG 380 (Topic 05) 16 Quantiles § Each class contains the same number of observations/values § Calculation of classes: Slocum et al. 2005; Figs 5.2 & 5.4 § Determine number of observations/values § Divide by number of classes GEOG 380 (Topic 05) 17 Mean-Standard Deviation § Derive classes from descriptive statistics of overall data distribution § Calculation of classes: Slocum et al. 2005; Figs 5.2 & 5.4 § Calculate mean and standard deviation § Compute class limits by adding/subtracting multiples of the standard deviation GEOG 380 (Topic 05) 18 Maximum Breaks (or Defined Interval) § Derive classes from groups of similar data values according to local criterion § Calculation of classes: Slocum et al. 2005; Figs 5.2 & 5.4 § Order data from low to high § Calculate differences between adjacent values § Use largest differences as class breaks GEOG 380 (Topic 05) 19 Natural Breaks § Subjective, visual/manual determination of logical breaks in data distribution in dispersion graph or histogram § Calculation of classes: Slocum et al. 2005; Figs 5.2 & 5.4 § Minimize differences within classes and maximize differences between classes GEOG 380 (Topic 05) 20 Geometric(al) interval § § § § Class breaks are based on a geometric series Good for highly skewed data Usually written as: a + ar + ar2 + ar3 + ar4 +… § a is the coefficient for each term § r is the common ratio § 2 + 6 + 18 + 54 + … § 2 is the coefficient § 3 is the ratio GEOG 380 (Topic 05) 21 Optimal (multiple techniques) Slocum et al. 2005; Figs 5.2 & 5.4 § Computational approaches to minimizing classification error § Fisher-Jenks/Jenks Natural breaks/Jenks optimal method is the most common § This method seeks to reduce the variance within classes and maximize the variance between classes GEOG 380 (Topic 05) 22 Rating of Classification Methods Slocum et al. 2005; Fig 5.7 § Rating system depends on map user’s knowledge and map purpose GEOG 380 (Topic 05) 23