GENE3340 Genetic Variation PDF
Document Details
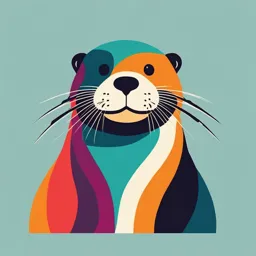
Uploaded by JovialKineticArt
UWA
Mark Cruickshank
Tags
Related
- 2024 Human Genome Lecture Notes PDF
- Fundamental Topics in Biology 2X Lecture 3 Molecular Biology III (2024-2025) PDF
- Tema 3 Alteraciones Genéticas I PDF
- Evolution of Human Genome - Sara V - PDF
- Central Dogma and Genetic Variation Lecture PDF
- BCH I Patterns of Single Gene Inheritance Molecular and Biochemical Basis of Genetics Diseases PDF
Summary
These lecture notes cover genetic variation, including single nucleotide variants, polymorphisms, and structural variations. They discuss the nomenclature for describing these variations and their potential effects on phenotype. The notes also explore the scale of human genetic variation based on large-scale sequencing projects and databases. Concepts of mutation, inheritance, and DNA repair are briefly covered.
Full Transcript
Molecular Genetics GENE3340 Genetic Variation I & II Dr. Mark Cruickshank, Ph.D. School of Biomedical Sciences, UWA Cancer Genomics and Epigenetics Laboratory M-block Room 2.26 Email: [email protected] These le...
Molecular Genetics GENE3340 Genetic Variation I & II Dr. Mark Cruickshank, Ph.D. School of Biomedical Sciences, UWA Cancer Genomics and Epigenetics Laboratory M-block Room 2.26 Email: [email protected] These lecture slides and associated unit materials are the intellectual property of Dr Mark N. Cruickshank and should not be shared without his permission. Sharing course materials without permission breaches UWA’s student conduct regulations and may constitute a breach of the Copyright Act 1968 Learning Objectives: 1. Understand the terms variant and polymorphism and the scale of human variation 2. Be able to describe SNVs, SNPs, indels & CNV and their nomenclature 3. Understand genetic variation may/may not have functional consequences 4. Be able to describe different types of repetitive regions within the genome, how replication slippage affects genetic diversity and how/why they are used in genetic fingerprinting 5. Be able to describe the concept of structural variation i.e. balanced vs unbalanced 6. Be able to describe how exogenous/endogenous factors can induce DNA damage 7. Be able to describe common types of DNA damage and how they are repaired 8. Be able to describe the aims of the HapMap project 9. Understand the 1000 genomes project. 10. Understand a range of databases used to curate human genetic variation and their consequences Additional Reading: Chapter 4, Genetics and Genomics in Medicine (1st Edition), Strachan Background on seminal findings on DNA function pre-1950 FYI 1859 – Charles Darwin publishes “On the origins of species” 1866 – Gregor Mendel inheritance of crop traits (phenotype segregation patterns). 1869 – Friedrich Miescher isolated “nuclein” (DNA). 1881 – Albrecht Kossel isolated basic building blocks of DNA and RNA: A, T, G, C, U. 1882 – Walther Flemming observed chromosome doubling. Early 1900s – Theodor Boveri and Walter Sutton developed the chromosome theory. 1944 – Oswald Avery outlined DNA as the transforming principle. 1944 – 1950 – Erwin Chargaff discovered that DNA is responsible for heredity and that it varies between species. Late 1940s – Barbara McClintock discovered the “jumping gene,” or the idea that genes can move on a chromosome. Automated Sanger sequencing FYI The synthesis of oligonucleotides containing an aliphatic amino group at the 5' terminus: synthesis of fluorescent DNA primers for use in DNA sequence analysis. L M Smith, S Fung, M W Hunkapiller, T J Hunkapiller, and L E Hood. Nucleic Acids Res, 13(7): 2399–2412 (1985). Fluorescence Detection in Automated DNA Sequence Analysis. L M Smith, J Z Sanders, R J Kaiser, P Hughes, C Dodd, C R Connell, C Heiner, S B Kent, L E Hood. Nature, 321: 674-9 (1986). Human genome project 1 https://www.genome.gov/human-genome-project “The Human Genome Project was a large, well-organized, and highly collaborative international effort that generated the first sequence of the human genome and that of several additional well-studied organisms. Carried out from 1990–2003, it was one of the most ambitious and important scientific endeavors in human history.” 1999 – Human chromosome 22 sequenced. Dunham, I., Hunt, A., Collins, J. et al. The DNA sequence of human chromosome 22. Nature 402, 489–495 (1999). https://doi.org/10.1038/990031 2001 – Initial sequencing and analysis of the human genome. International Human Genome Sequencing Consortium. Initial sequencing and analysis of the human genome. Nature 409, 860–921 (2001). https://doi.org/10.1038/35057062 2001 – Initial sequencing and comparative analysis of the mouse genome. Mouse Genome Sequencing Consortium. Initial sequencing and comparative analysis of the mouse genome. Nature 420, 520–562 (2002). https://doi.org/10.1038/nature01262 2002 – The complete sequence of a human genome. The complete sequence of a human genome. Science 376, 44–53 (2002). https://www.science.org/doi/10.1126/science.abj6987 1 ~ 3.2 billion base pair (Long terminal repeat) (Short interspersed nuclear element) (Long interspersed nuclear element) Adapted from T. R. Gregory Nat Rev Genet. 9:699-708, 2005 based on International Human Genome Sequencing Consortium. Initial sequencing and analysis of the human genome. Nature 409:860 2001 1 The Scale of Human Genetic Variation Human genetic variation = changes to base sequence, two categories: – Do not affect DNA content (number nucleotides unchanged) Single nucleotide is replaced Rarely – multiple nucleotides move location without net loss/gain (translocations/inversions) – Causes a net loss/gain of DNA sequence Change in copy number of DNA sequence (large or small) Abnormal chromosome segregation Deletion/insertion of a single nucleotide or short sequences to Mb DNA Overall, the most common DNA changes are on small scale. May or may not have effect on phenotype (many unknown à variants of unknown significance!). 3 Variant Nomenclature The HGVS (Human Genome Variation Society) Nomenclature is an internationally- recognized standard for the description of DNA, RNA, and protein sequence variants. It is used to convey variants in clinical reports and to share variants in publications and databases. All variants should be described at the most basic level, the DNA level. Descriptions on the RNA and/or protein level may be given in addition. https://hgvs-nomenclature.org/stable/ 3 Variant Nomenclature NC_000023.10:g.33038255C>A Variant Approved identifier Base position (recommended reference is based on Reference base genome build GRCh38/hg38) https://hgvs- Reference type nomenclature.org/sta c for a coding DNA reference sequence, ble/background/refse g for a linear genomic reference sequence, q/ m for a mitochondrial DNA reference sequence, n for a non-coding DNA reference sequence, o for a circular genomic reference sequence, p for a protein reference sequence, r for an RNA reference sequence (transcript). https://hgvs-nomenclature.org/stable/ 3 Variant Nomenclature > (greater than) indicates a substitution (DNA and RNA level); g.123456G>A, r.123c>u a substitution on the protein level is described as p.Ser321Arg del indicates a deletion; c.76del dup indicates a duplication; c.76dup ins indicates an insertion; c. **note that duplicating insertions are described as duplications, not as insertions. inv indicates an inversion; c.76_83inv (see DNA, RNA). Not used on protein level. fs indicates a frameshift; p.Arg456GlyfsTer17 (or p.Arg456Glyfs*17). (Ter, termination or *) 3 Variant Nomenclature NC_000023.10:g. 32862923_32862924insCCT the insertion of nucleotides CCT between Approved identifier Base positions nucleotides (recommended reference is based on flanking insertion g.32862923 and genome build g.32862924. GRCh38/hg38) https://hgvs- Reference type nomenclature.org/sta c for a coding DNA reference sequence, ble/background/refse g for a linear genomic reference sequence, q/ m for a mitochondrial DNA reference sequence, n for a non-coding DNA reference sequence, o for a circular genomic reference sequence, p for a protein reference sequence, r for an RNA reference sequence (transcript). 3 Point Mutations Do Not Always Have Phenotypic Effect. Type of DNA sequence Amino acid sequence mutation ATG CAG GTG ACC TCA GTG M Q V T S V None ATG CAG GTT ACC TCA GTG M Q V T S V Silent ATG CAG GTA ACC TCA GTG M Q L T S V Conservative Non- ATG CCG GTG ACC TCA GTG M P V T S V conservative ATG CAG GTG ACC TGA GTG M Q V T STOP Nonsense ATG CAG GTG AAC CTC AGT G M Q V N L S Frameshift 1 Definitions of genetic variation Mutations result in alternative forms of DNA that are generally known as DNA variants. Alleles are the alternative forms of a gene sequence found at the same location on a chromosome. For any locus, if more than one DNA variant is common in the population (above frequency of 0.01) = polymorphism. DNA variant frequencies less than 0.01 = rare variants. Our knowledge of variants comes from analyzing DNA from multiple individuals: – Mammals are diploid with two nuclear genomes, one from each parent. Mitochondria also contain DNA but are exclusively inherited from mother to offspring. – There is a need to compare many different individuals. – Data from the human genome project (1990-2003) was derived from many individuals and represents a patchwork of sequences. 1 Extent of Variation in Human Genome? Craig Venter (2007) & James Watson (2008) diploid genome sequenced, compared to reference: https://doi.org/10.1038/nature06884 1 Extent of Variation in Human Genome? Craig Venter (2007) & James Watson (2008) diploid genome sequenced, compared to reference: https://doi.org/10.1371/journal.pbio.0050254 1 Extent of Variation in Human Genome? Craig Venter & James Watson diploid genome sequenced, compared to reference: – 12 million nucleotides differed from ref seq – Majority non-coding – 3.2 million SNPs – 44% CraigV genes had sequence variant 17% encoded an altered protein – 290,000 heterozygous insertion/deletion variants (ranging from 1-571bp) – 559,000 homozygous insertion/deletion variants (ranging from 1-82,711bp) – 90 large inversions – 62 large-copy-number variants Single nucleotide variants and single 1, 2 nucleotide polymorphisms Most common variation due to single nucleotide substitution: Type of variant produces single nucleotide variants (SNVs). If two or more alternative DNA variants exceed frequency of 0.01 in population = single nucleotide polymorphism (“SNIP”) 1, 2 Pattern of SNV in human genome is nonrandom Most common variation due to single nucleotide substitution: Type of variant produces single nucleotide variants (SNVs). If two or more alternative DNA variants exceed frequency of 0.01 in population = single nucleotide polymorphism (SNP) Pattern of SNV not random: Regional intolerance to genetic variation. Mitochondrial DNA higher than nuclear. Excess of C-T substitutions (methylation). Evolutionary ancestry Why certain nucleotides polymorphic, others rarely show variants. 1.1-1.4 x 10-8 per base pair per generation à ∼74 novel SNVs per genome per generation. Alternative SNPs mark alternative ancestral chromosome segments common in present day population Imprecise cut-off between indels and Copy Number 1, 2 Variants (CNV) Some point mutations create variants differ by presence of absence of nucleotide Eg of insertion/deletion (indel) variation Imprecise cut-off between indels and Copy Number 1, 2 Variants (CNV) Strictly – indels should be copy number variants Heterozygous deletion of a single nucleotide at a defined position on chromosome has one copy of that nucleotide instead of two Modern convention is that indel describes deletions/insertions ~50-100 nucleotides in length. Term copy number variation = change in copy number of sequences result in larger deletions/insertions (more than ~100 nucleotides) Frequency of indels 1/10th of single nucleotide substitution Short insertions more common than long 90% = 1-10 nucleotides 9% = 11-100 1% = greater than 100 1, 2 Size distribution of CNVs in human genome Microsatellites and other polymorphisms can arise 3 due to variable number tandem repeats Repetitive DNA accounts for large fraction of human genome Tandem copies (1bp to 200bp) are common, those with multiple repeats prone to variation satellite DNA – length = 20kb to many 100’s kb; located at centromeres, heterochromatic regions minisatellite DNA –length = 100bp to 20kb; located primarily at telomeres and subtelomeric regions microsatellite DNA length = fewer than 100bp; widely distributed through euchromatin Short Tandem Repeats (STRs) = 1-6 bp; Instability of repeat sequence –variants differ in number of repeats Variation in copy number results from replication slippage or unequal crossover Length Polymorphism in a microsatellite 3 Unlike SNPs (two alleles), microsatellites have multiple alleles 3 Dark blue = new (nascent) DNA strand from pale blue template During replication, nascent strand partly dissociates from template, then reassociates. Nascent strand may mispair - new strand has more repeat units OR…… 3 3 Microsatellite Markers Primer 1 CA CA CA Primer 2 CA CA CA CA CA CA CA CA CA CA CA CA CA CA CA 3 Genotyping Results Been genetic marker of choice since 1990s More informative than SNPs for distinguishing between individuals or following chromosome segments Child 1 through pedigree Child 2 Early years HGP largely devoted to defining and mapping microsatellites. Child 3 ~150,000 identified Father Not as easy to automate as SNP (later) Mother D13S121- dinucleotide Unequal crossing over is a type of gene 3 duplication or deletion event that deletes a sequence in one strand and replaces it with a duplication from its sister chromatid in mitosis or from its homologous chromosome during meiosis. Unequal crossing over is a type of gene 3 duplication or deletion event that deletes a Meiotic recombination sequence in one strand and replaces it with a between mispaired repeats duplication from its sister chromatid in mitosis = changes in unit number or from its homologous chromosome during meiosis. Main mechanism for minisatellite diversity During meiosis, misaligned chromatids can be on homologous chromosomes causing UEC During mitosis, homologous recombination between sister chromatids mediates DNA repair but can be misaligned causing UESCE Result in two chromatids – one with extra repeat, the other with unit missing 5 Structural Variation and low copy number variation Until recently, study of human variation largely focused on small- scale – SNVs and microsatellites Variation due to moderately large-scale changes in DNA is very common Structural Variation – Balanced SV – DNA variants have same DNA content but differ in some DNA sequences are located in different positions in genome Chromosomes break and fragments are incorrectly rejoined, w/out loss or gain of DNA = inversions and translocations – Unbalanced SV – DNA variants differ in DNA content. Rare case where person gained/lost chromosomal region, often results in disease Also includes commonly occurring CNV, variants differ in number of copies of moderately long to very long DNA sequence. Some CNVs contribute to disease, others normal 5 Balanced SV = large scale changes variants with same number of = inversion nucleotides Note: 1 and 2 represents alternative variants = translocations Note: 1 and 2 represents 5 alternative variants Unbalanced SV = unbalanced inversions/translocations & low CNV. CNV have different numbers of copies of sequence (shown as box marked A) (i) = insertion/deletion of element (ii) = CNV due to tandem duplication Additional insertion/inversion events can result in interspersed duplication (iii) = normal orientation (iv) = inversion of copy 5 Map of segmental duplications in the human genome Map of human chromosomes 1, 2 and 3 showing positions of duplications greater than 10 kb in size. Blue connecting lines – intrachromosomal duplications. Red bars – Interchromosomal duplications. A and B – hotspots where recombination gives rise to genetic disorders 5 Long-read sequencing Short-read sequencing requires generating data from overlapping contigs Long-read technologies can generate continuous sequences ranging from 10 kilobases to several megabases in length Useful for sequencing repetitive regions Many different technologies including single-molecule sequencing Useful for generating high-quality genome assemblies Allowing interrogation of diversity of SVs in humans Long-read sequencing technologies have been used to resolve some of the most challenging regions of the human genome, detect 5 previously inaccessible structural variants and generate some of the first telomere-to-telomere assemblies of whole chromosomes. Logsdon, G.A., Vollger, M.R. & Eichler, E.E. Long-read human genome sequencing and its applications. Nat Rev Genet 21, 597–614 (2020). https://doi.org/10.1038/s41576-020-0236-x 5 b | The panel on the left shows a heatmap of differentially expressed genes located near structural variants (SVs) in chimpanzees and humans. Differences in macaque, chimpanzee and human brains for genes that have a human-specific SV within 50 kb of the transcription start or stop site. Structural changes, such as a deletion of an enhancer region as shown on the right, can cause changes in gene expression fundamental to brain development30. Part a is adapted from ref.66, Springer Nature Limited. a | The NOTCH2NLA, NOTCH2NLB, and NOTCH2NLC genes are located within chromosome band 1q21.1, a segmental duplication (SD)-rich region of the genome partially assembled by Pacific Biosciences (PacBio) continuous long read (CLR) sequencing of bacterial artificial chromosome clones116. The region was originally incorrectly assembled in the human reference genome116. Deletions (del) and duplications (dup) mediated by the SD-rich region can cause thrombocytopenia– absent radius syndrome166 as well as distal 1q21.1 deletion/duplication syndrome119,167. High-quality sequencing of the region allowed the breakpoints of these disease-causing rearrangements to be better defined and improved the annotation of human-specific NOTCH2NL duplicate genes116. Subsequent sequencing of this region in patients with neuronal intranuclear inclusion disease and leukoencephalopathy by PacBio and Oxford Nanopore Technologies long-read sequencing recently identified a GGC repeat expansion in exon 1 of NOTCH2NLC in affected patients66 (exons are in red, untranslated regions (UTRs) are in grey). Expansion of the repeat is associated with the production of antisense transcripts whose role is uncertain but may interfere with the expression and regulation of the gene family. Figure: SVs, including multi-exon deletions are found in medically relevant genes Phased IGV view from LRS data showing a CYP2D6 full gene deletion on one haplotype (HP1) and a hybrid tandem arrangement (*36+*10) represented by an insertion on the second haplotype (HP2) in HG02396, compared to short-read whole genome sequence data from the same sample in which the complex nature of this event cannot be resolved. Nanopore sequencing of 1000 Genomes Project samples to build a comprehensive catalog of human genetic variation https://doi.org/10.1101/2024.03.05.24303792 medRxiv preprint Origins of DNA Variation Some DNA variants arise from errors in DNA replication or recombination Errors in replication unavoidable but majority of times, errors quickly corrected by DNA polymerase itself Errors in chromosome segregation results in abnormal gametes, fewer or more chromosomes than normal. Various natural errors give rise to altered copy number of specific sequence within a DNA strand. Crossover errors Various endogenous/exogenous sources can cause damage to DNA by altering chemical structure Chemical Damage to DNA….. Cleavage of covalent bonds in sugar-P backbone Cleavage of N-glycosidic bond, base to sugar Replacing certain groups on bases, adding a chemical group (red) Involves formation of Eg (i) 8-oxyguanine, base covalent bonds between two pairs to adenine bases (crosslinking). Modified bases may block polymerases Endogenous chemical damage to DNA Three major types of damage Hydrolytic –disrupts bonds that hold bases to sugars. Also strips amino groups from some bases Oxidative – Metabolism generates ROS. Attack covalent bonds in sugars, strand breakage. Also attack DNA bases (purines). Methylation - SAM, methyl donor Frequent types of DNA Damage and how they are repaired Base excision repair Lesions where single base modified or excised - replace modified base, specific DNA glycosylase cleaves sugar- base bond to delete base (abasic site) - residual sugar-P removed by endonuclease & phosphodiesterase - Gap filled by DNA polymerase and ligase Nucleotide excision repair Repair of bulky, helix-distorting DNA lesions - Lesion detected, damaged site opened out, DNA cleaved some distance either side - generating ~30bp oligonucleotide which is discarded - synthesis of DNA performed using opposite strand as template - DNA polymerase & ligase Repair of lesions affect both strands Homologous recombination- mediated DNA repair DSB is repaired using undamaged strands in sister chromatid as template Cut back the 5’ ends at DSB to leave protruding ss with 3’ ends After strand invasion, each ss region forms duplex with undamaged complementary strand from sister chromatid Acts as template for new DNA synthesis. Ends sealed by DNA ligase Health Consequences of Defective DNA Damage Response/Repair C = cancer susceptibility; P = progeria (premature aging); N = neurological features; I = immunodeficiency 8 HapMap 2002-2009 1. Human Genome Project à Good for consensus, not good for individual differences Sept 01 Feb 02 April 04 Oct 04 2. Identify genetic variants à Anonymous with respect to traits. April 1999 – Dec 01 3. Assay genetic variants à Verify polymorphisms, catalogue correlations amongst sites à Anonymous with respect to Oct 2002 - 2009 traits 8 HapMap project “The goal of the International HapMap Project is to determine the common patterns of DNA sequence variation in the human genome, their allele frequencies and the degree of association between them”. 2002 à 2009 Cell lines from participants Four populations: CEPH (Europe), Yoruban (Africa), Japanese/Chinese (Asian) Mainly utilized microarray technology Phase I o 1 million common SNPs (every 5 kb across the genome) were genotyped in 269 DNA. Phase II o 3.1 million common SNPs were genotyped in 270 DNA samples from four populations. 8 HapMap $ 45 Million!!! Finding SNPs: Marker Discovery and Methods Goals: Identify 300,000 SNPs Determine allele frequency of SNPs Infer haplotype structure – ie correlation of SNPs across the genome 8 HapMap https://www.genome.gov/11511175/about-the-international-hapmap-project-fact-sheetThe goal of the International HapMap Project was to develop a haplotype map of the human genome. Often referred to as the HapMap, it describes the common patterns of human genetic variation. The HapMap provides a key resource for researchers to use to find genes affecting health, disease and responses to drugs and environmental factors. The information produced by the project is now freely available in public databases to researchers around the world. The International HapMap Project officially started with a meeting, held from Oct. 27 to 29, 2002, and achieved its goal of completing the map within three years. The project was a collaboration among researchers at academic centers, non-profit biomedical research groups and private companies in Japan, the United Kingdom, Canada, China, Nigeria and the United States. A list of participating and funding institutions is available at: http://hapmap.ncbi.nlm.nih.gov/groups.html. HapMap The International HapMap Consortium. A haplotype map of the human genome. Nature 437, 1299–1320 (2005). https://doi.org/10.1038/nature04226 Redon, R., Ishikawa, S., Fitch, K. et al. Global variation in copy number in the human genome. Nature 444, 444–454 (2006). https://doi.org/10.1038/nature05329 The International HapMap Consortium. A second generation human haplotype map of over 3.1 million SNPs. Nature 449, 851–861 (2007). https://doi.org/10.1038/nature06258 8 Genealogical relationships among haplotypes and r2 values in a region without obligate recombination events. The region of chromosome 2 (234,876,004–234,884,481 bp; NCBI build 34) within ENr131.2q37 contains 36 SNPs, with zero obligate recombination events in the CEU samples. The left part of the plot shows the seven different haplotypes observed over this region (alleles are indicated only at SNPs), with their respective counts in the data. Underneath each of these haplotypes is a binary representation of the same data, with coloured circles at SNP positions where a haplotype has the less common allele at that site. Groups of SNPs all captured by a single tag SNP (with r2 ≥ 0.8) using a pairwise tagging algorithm53,54 have the same colour. Seven tag SNPs corresponding to the seven different colours capture all the SNPs in this region. On the right these SNPs are mapped to the genealogical tree relating the seven haplotypes for the data in this region. 9 1000 genomes project 1000 genomes project Phase 1 – Genotyping 1092 individuals – 14 populations – Europe, East Asia, sub-Saharan Africa, Americas – Whole-genome (low coverage; 2-6x) and exome sequencing (deep coverage; 50-100x) Most recent = Phase 3 completed 2015 – 2504 individuals – 26 populations – Both exome and whole-genome data 9 Taking stock of human genetic variation – 1000 Genomes Study individuals 2,504 individuals from 26 populations – 84.7 million SNPs (1 per 100 nucleotide) A typical genome differs from the reference human genome at 4.1 million to 5.0 million sites Vast majority are rare in any population However, most variants observed in a single genome are common Thus, in an individual – two haploid genomes, for any SNP loci, many will be homozygous Typical genome contains an estimated 2,100 to 2,500 structural variants affecting ,20 million bases of sequence. Data from population-based genome indicate single nucleotide changes most common type of variation. >99.9% of variants consist of SNPs and short indels. Structural variants affect more bases: the typical genome contains an estimated 2,100 to 2,500 structural variants 9 Variation within and between human populations – 1000 Genomes Within populations: ~33% of protein-encoding loci are polymorphic. additional nucleotide diversity in introns, regulatory sequences, flanking sequences. ~85% of total genetic variation is found within populations Between populations: frequencies of alleles may vary, especially for morphological traits FYI Campbell, M.C. & Tishkoff, S.A. The evolution of human genetic and phenotypic variation in Africa. Curr Biol 20, R166-73 (2010). https://www.sciencedirect.com/science/article/pii/S096098220902065X FYI The serial founder model in human evolution. A schematic of the model. Each color represents a distinct allele. Migration events outward from Africa tend to carry with them only a subset of the genetic diversity from the source population, and some alleles are lost during migration events. Genetic Diversity and Societally Important Disparities. Noah A. Rosenberg and Jonathan T. L. Kang Genetics September 1, 2015 vol. 201 no. 1 1-12; https://doi.org/10.1534/genetics.115.176750 Various databases curate data on genetic variation 10 Database Description Website dbSNP SNP database https://www.ncbi.nlm.nih.gov/SNP/ dbVar Genomic structural variation https://www.ncbi.nlm.nih.gov/dbvar/ DGV Genomic structural variation dgv.tcag.ca/ ExAC 60,706 exomes http://exac.broadinstitute.org/ gnomAD 125,748 exomes and 15,708 genomes https://gnomad.broadinstitute.org/ HGV Database Searchable online database of peer-reviewed http://www.hgvd.genome.med.kyoto-u.ac.jp/ genome variations ClinVar Public archive of the relationships among https://www.ncbi.nlm.nih.gov/clinvar/ human variations and phenotypes Clinical Genome Resource Curation of genes/regions that are dosage https://clinicalgenome.org/ (ClinGen) sensitive Online Mendelian An Online Catalog of Human Genes and https://www.omim.org/ Inheritance in Man® Genetic Disorders (OMIM®) Three Million African Genomes (3MAG) UK Biobank GTEx 1,000 individuals, 54 tissues, RNA-Seq https://gtexportal.org/home/ exome/genome sequencing data. OMIM = “Online Mendelian Inheritance in Man” 10 It is a system of cataloging human genes and genetic diseases. - It was first created by Dr. Victor McKusick of Johns Hopkins. ClinVar 10 It is a system of cataloging human genetic variation in disease. 8 Functional Genetic Variation & Protein Polymorphism Most genetic variation has a neutral effect on the phenotype but small fraction is harmful (beneficial?) Functional variants that are primarily studied are those that have an effect on gene function Estimating how much of genome is functionally important is not straight forward Even within the small target of sequences that are important for gene function, many small DNA changes may still have no effect (coding, regulatory, noncoding RNA). GENE3340 Molecular Genetics II Linkage and Association Studies I, II Belinda Kaskow School of Biomedical Sciences, UWA Email: [email protected] Acknowledgement of country The University of Western Australia acknowledges that its campus is situated on Noongar land, and that Noongar people remain the spiritual and cultural custodians of their land, and continue to practise their values, languages, beliefs and knowledge. Artist: Dr Richard Barry Walley OAM Learning Outcomes Understand evidence used to determine if disease/trait is genetic in origin. Be able to describe the difference between mendelian and complex diseases. Be able to describe general approaches used for disease gene identification and the use of genetic markers. Understand the process of meiotic recombination and how to assess non- recombinants and recombinants. Understand what recombination fractions are used for. Be able to define the term linkage analysis and how it is used to determine the location of a disease gene within the genome Describe the difference between parametric and non-parametric linkage analysis Understand the significance of LOD scores Why do we care about variations? underlie cause phenotypic inherited differences diseases allow tracking ancestral human history If you are interested in studying a human disease, how do you find out which gene, when mutated, causes that disease? You can find that position by genetic mapping. John Chase http://www.chasetoons.com What is the evidence that a disease or trait is genetic? Twin Studies Compare Monozygotic and Dizygotic Twins Monozygotic Twins : Genetically identical Dizygotic Twins : Like siblings (1/2 genome shared) Compare concordance rates of MZ and DZ twins MS Example: @salyerstwins- Instagram Monozygotic Twins : Concordance rate 25-30% Dizygotic Twins : Concordance rate 2-5% What is the evidence that a disease or trait is genetic? Family Segregation Increased risk for disease among family members of an affected individual Compare frequency of disease among first degree relatives of affected individuals with the frequency of the disease in the general population. MS Example: Risk to 1st-degree relatives (Parents, siblings, children): 2-5% General Population: 0.1-0.2% The goal of a genetic study: Identify genetic risk factors (genetic locations) causing human diseases. Types of Genetic (inheritable) Disease Single gene (Mendelian) disorders Obvious they are genetic Gene segregation within pedigrees (families) Rare Complex Diseases / Complex genetic disorders There may be no recognizable pattern of inheritance Likely due to the action of multiple genes Genes may be interacting with each other to result in disease phenotypes Environmental factors/gene-environmental interactions may affect disease. Schriml et al, 2023, Modelling the enigma of complex disease etiology. J. Transl. Med. Monogenic Disorder Complex Disorder Peltonen et al, 2001, Genomics and Medicine. Dissecting Human Disease in the Postgenomic Era. Science Gene Identification Approaches Positional cloning (Reverse Genetics) Disease Function Gene Map Examples: Cystic Fibrosis (CFTR) The identification of a gene based solely on its position in the genome Most widespread strategy in human genetics in the last 20-35 years Strengths: – No knowledge of gene product required – Very strong track record in single-gene disorders Weaknesses: – Understanding of function not a certain outcome – Poor track record with multifactorial traits Gene Identification Approaches Candidate Gene Approach Candidate genes are genes located in a chromosome region suspected of being involved in the expression of disease traits Limit to the known biological functions of a particular disease. Can be identified by association and linkage with phenotypes. Whole Genome Screen Approach Scan the whole genome without any prior information. Can discover potential genes playing roles in diseases. Linkage: 6 or 10K SNPs enough Association: 500K or 1 million SNPs are recently available Summary: Gene Identification Approaches Approach Starting Point Key Method Use Case Identifying genes Genetic Linkage analysis, linked to specific Positional Cloning marker/location chromosomal mapping chromosomal regions Investigating specific Candidate Gene Suspected gene based Genetic association genes based on prior Approach on function studies knowledge Unbiased discovery Whole Genome GWAS, whole-genome Entire genome of genetic factors for Screening sequencing complex traits Gene mapping technique Disease Phenotype GENE MAPPING: Biology Linkage/Association Analysis Disease Marker Genotype Genotype Proximity Principle: People who have similar phenotypic values (ie DISEASE) should have higher chance of sharing of genetic material near the genes that influence those traits. How to identify genes contributing to disease? Linkage Mapping Measures the segregation of alleles and a phenotype within a family. Use crossover occurring during meiosis II Genes that are physically close together are more likely to be co-inherited Genes that are physically far apart are less likely to be co-inherited. Detect over broad chromosomal regions on the genome. Linkage disequilibrium (Association Study) Evaluate the evidence of a direct correlation between a marker allele and a disease risk allele. Sharing of genetic material: actual sharing of the same allele (Linkage disequilibrium: LD) Genetic Markers A genetic marker is a polymorphic DNA sequence with a known location on a chromosome that can be used to identify individuals MARKER No. of loci Advantage Disadvantage Abundant in the genome Often Bi-allelic (two High, occur Provide high-resolution possible alleles) which SNP ~1 in every mapping limits information from one 100-300 bp Low mutation rate (stable) location Many technologies available SSR (short- High, occurs Lower abundance sequence Multi-allelic: each SSR can ~ every 2-30 more labour-intensive repeat/micro have multiple alleles kb Higher mutation rate satellite) Meiosis and Recombination Father Mother During meiosis, the chromosomes duplicate, then cross over (‘recombine’) to produce a haploid gamete (sperm/ egg) Meiosis Sperm Egg The gamete derives genetic variants from both parents Fertilisation Meiosis is the basis for heredity Child Figure 17.4 Human Molecular Genetics. Strachan & Read. 5th Ed. Markers and Inheritance Father Mother 1 2 4 3 2 1 3 4 2 3 1 2 2 3 1 4 3 1 Child Polymorphic loci whose locations are known Most often SNPs or microsatellites Inherited within the chromosomes For linkage analysis, we need informative meiosis Example 1 Two loci (A and B) with two alleles (A1, A2, B1, B2) In generation III, we can determine whether individuals are: Nonrecominant* = N = A1B1 or A2B2 *But only from the sperm. The mother II2 is homozygous for these two loci so we cannot Recominant* =R = A1B2 or A2B1 determine if the oocytes are N or R Figure 17.3 Human Molecular Genetics. Strachan & Read. 5th Ed. Markers and Inheritance A) Two loci in this pedigree on different chromosomes. Assort independently. 5R and 5NR = RF 0.5 B) Two loci are only a few megabases apart on the same chromosome. Show linkage. 9NR and 1R = RF 0.1 Linkage Only ~1 recombination per chromosome/meiosis → Loci that are close together on the same chromosome tend to be inherited together (‘linked’ or ‘in LD’ = linkage disequilibrium) The closer the loci, the more linkage → Degree of linkage is a measure of genetic distance Linkage is measured by the recombination fraction, θ = proportion of recombinants θ = 0 complete linkage θ = 0.5: no linkage Two loci on the same chromosome, only a cross-over event will separate them. What is recombinant fraction? θ is a measure of genetic distance Further apart two loci, the more likely a crossover event will occur (θ value will increase) Centimorgan (cM) is a genetic distance unit 1 cM = 1% chance of recombination 1 cM approx. 1000 kb = 1 mb Obtaining enough family material to test multiple meiosis is difficult for rare diseases TABLE 14.2 THE NUMBER OF INFORMATIVE MEIOSES NEEDED TO OBTAIN EVIDENCE OF LINKAGE BETWEEN TWO LOCI Recombination Fraction 0 0.05 0.10 0.15 0.20 0.40 Minimum no. of informative meioses 10 14 19 26 36 343 Higher RF between two loci, more meioses needed to obtain evidence that they are linked Scoring recombinants in human pedigrees not always simple Table 14.2 Human Molecular Genetics. Strachan & Read. 4th Ed. Linkage mapping: is a marker “linked” to the disease gene Collect families with affected individuals Genome Scan - Test markers evenly spaced across the entire genome (~every 10cM, ~400 markers) Lod score (“log of the odds”) – what are the odds of observing the family marker data if the marker is linked to the disease (less recombination than expected) compared to if the marker is not linked to the disease Test to estimate whether the likelihood that TWO LOCI ARE LINKED is greater than likelihood that THE TWO LOCI BEING UNLINKED LIKELIHOOD OF LINKAGE (θ < 0.5) Z = LOG10 LIKELIHOOD LOCI ARE UNLINKED (θ = 0.5) Linkage Mapping Parametric method Non--parametric method – Estimate recombination fraction – Count the number of alleles between a marker locus and an two affected sibs share unobserved trait locus. identical by descent (IBD). Father Mother DA da 12 34 12 34 12 34 da da 13 13 13 14 13 24 Mother=> da da da da IBD=2 IBD=1 IBD=0 Father => DA da dA Da Non-Recombinants Recombinants – If the marker is linked to the disease locus, the affected sibs will tend to share the – Out of 4 informative meioses, disease allele more often than they would 2 are recombinants => 1/2 at a marker unlinked to the disease locus. Statistical significance of Lod Scores Z >3.0 2.0< Z< 3.0 -2.0 < Z 3.0 2.0< Z< 3.0 -2.0 < Z 0.05) GWAS: 101 500-1000 Cases 500-1000 Controls Extract DNA – Genotype* Currently: ~$300 per individual (by standard methods) (n = 2000 x $300 = $600,000)*** Calculate which of 300-500,000 SNPs and/or haplotypes are more frequent in cases than controls Haplotype blocks Attempts to define ancestral chromosome segments = high-resolution haplotype structure. Suggests our DNA is composed of defined blocks of limited haplotype diversity Genotyping 8 SNP (5q31) loci reveals 84kb haplotype block. Just two haplotypes account for vast majority of chromosomes from European pop. * Remember: 8 x SNP = 28 = 256 haplotypes possible Haplotype blocks Adjacent haplotype blocks at 5q31 – blocks 1, 2, 3, 4 were genotyped at 5, 9, 11 SNP loci and had between two & four haplotypes with certain population frequencies. Dashed black lines = locations where >2% of all chromosome 5 are seen to switch between haplotypes Imputing SNPs Doing a GWAS GWAS Steps Using HapMap data (map LD), representative SNPs selected which differentiate (tag) the common haplotypes (A, B, C) at each locus. Locus 1 = tagged by 4 SNPs Locus 2 = tagged by 2 SNPs Tagged SNPs are genotyped in disease cases & controls using microarrays Allele frequencies for each SNP compared in two groups SNPs associated with disease (statistical threshold) are genotyped in 2nd independent cohort Which associations are robust Figure 8.13 Genetics and Genomics in Medicine. Strachan & Lucassen. 2nd Ed. Visualising genome-wide association data: Quantile-quantile (Q-Q-plots) - Two types of distribution of observed test statistics generated in GWAS - In case-control studies a chi-squared comparison of absolute genotype counts is calculated for each variant - Red = idealized test results - Blue = expected values under null hypothesis of no association Manhattan Plots C) Coronary artery disease - blue = new loci - red = previously discovered loci Threshold = 7.3 p = 5 x 10-8 p = 0.05 / 1 million tests = 5 x 10-8 Bonferroni Correction- adjusts the conventional p value by dividing by the number on independent tests. Figure 8.14 Genetics and Genomics in Medicine. Strachan & Lucassen. 2nd Ed. GWAS and Odds Ratio (OR) Odds ratio: An effect size estimate of a risk factor that quantifies the increased odds of having the disease per risk allele count in genome-wide association studies (GWAS) Each SNP is an independent test. Associations are tested by comparing the frequency of each allele in cases and controls Allele counting method Association of rs6983267 with colorectal cancer C allele T allele Cases a 875 c 675 C is the risk allele Controls b 1860 d 1940 a: Number of individuals with the allele and the trait. b: Number of individuals with the allele but without the trait. c: Number of individuals without the allele but with the trait. d: Number of individuals without the allele and without the trait. The odds ratio (OR) is calculated as: "⁄# ÷ %⁄& = (875/675) ÷ (1860/1940) = 1.35 GWAS and Odds Ratio (OR) OR > 1: The presence of the SNP is associated with higher odds of the trait or disease. (RISK ALLELE) OR < 1: The presence of the SNP is associated with lower odds of the trait or disease. (PROTECTIVE ALLELE) OR = 1: No association between the SNP and the trait or disease. GWAS: Multiple Sclerosis IMSGC, 2011, Ann Neurol Limitations of GWAS Despite initial hopes, common disease variants identified by GWAS have very weak effects. Exceptions, novel factors that strongly predispose – e.g. Age-related macular degeneration Even cumulative contributions of identified variants are small. Available GWAS data explain only small proportion of genetic variance of complex diseases = missing heritability. GWAS: Multiple Sclerosis Genome-wide associations in MS 32 MHC 1 X chromosome 200 outside of MHC all in immune pathways “we can now explain ~39% of the genetic predisposition to MS with the validated susceptibility alleles” IMSGC, 2019, Science Missing Heritability Figure 18.8 Human Molecular Genetics. Strachan & Read. 5th Ed. Common Disease – Common Variant Hypothesis Common Disease – common variant hypothesis Different combinations of variants at multiple loci aggregate in specific individuals to increase disease risk In other words: SNPs at relatively large frequency in the population (>1%), but with relatively low penetrance (probability that a carrier will express the disease) are the major contributors to genetic susceptibility to common disease Explains why steep falling away of disease risk in relatives of probands with a common disease Common variants are expected to be of ancient origin. They are merely susceptibility factors and so have typically weak deleterious effects (ie mild missense mutation or changes in gene expression) Rare variants are expected to be of comparatively recent origin Common Disease – Rare Variant Hypothesis Moderately rare variants may have moderate effects, very rare variants expected to have rather strong effects, highly penetrant. Would not appear on common haplotype blocks – ancient origins. Common Disease – rare variant hypothesis Many complex diseases have known mendelian subsets in which pathogenesis is due to rare mutations of extremely strong effect In other words: multiple rare DNA sequence variations (10 million (https://www.23andme.com) ancestry AncestryDNA Genealogical, personal ancestry 2002 >16 million (https://www.ancestry.com/dna/) (autosomal only) FamilyTreeDNA Genealogical, personal ancestry 1999 >1.1 million (https://www.familytreedna.com) (autosomal only) GEDmatch 2010 >1.3 million Genetic genealogy search (https://www.gedmatch.com) MyHeritage Genealogical, personal ancestry 2003 >3 million (https://www.myheritage.com) (autosomal only) Bonomi, L., Huang, Y. & Ohno-Machado, L. Privacy challenges and research opportunities for genomic data sharing. Nat Genet 52, 646–654 (2020). https://doi.org/10.1038/s41588-020-0651-0 Forensic Investigative Genetic Genealogy https://www.nature.com/articles/d41586-018-05029-9 “The case of the Golden State Killer, linked to at least 50 rapes and 12 murders between 1976 and 1986, had gone cold — although investigators believed they had a reliable sequence of the perpetrator’s DNA. Next they needed a match. So, according to reports, they uploaded the data to a popular website that compares people’s genetic information to trace their relatives — in effect, creating a profile for him. They got lucky: a match with family members led them to identify and arrest Joseph James DeAngelo.” NSW Police https://www.police.nsw.gov.au/abo ut_us/information_of_interest_to_t he_community/forensic_investigati ve_genetic_genealogy Yaniv Erlich et al., Identity inference of genomic data using long-range familial searches. Science 362,690-694(2018). https://doi.org/10.1126/science.aau4832 Fig. 3 Tracing a 1000Genomes sample using a long- range familial search. The CEU pedigree is shown in black. To respect the privacy of the family, we omitted the sample identifiers and the exact pedigree structure. A GEDmatch search of the person of interest (black circle) returned two males (squares with gray dots) with a total IBD sharing of 180 and 171 cM to the target, respectively, and 62 cM between themselves. Using public genealogical records, we identified the ancestral couple (asterisk) of the matches and the person of interest. “We selected a female from the CEU (Utah residents with Northern and Western European ancestry) cohort, whose husband has been identified using surname inference (17). We extracted her genome from the (publicly available) 1000Genomes data repository, reformatted her genotype to resemble a file released by DTC providers, and uploaded the genotype to GEDmatch. Searching GEDmatch returned two relatives, one from North Dakota and one from Wyoming, with sufficient genetic and genealogical details (Fig. 3). Both relatives shared about 170 to 180 cM with the 1000Genomes sample, which corresponds to six to seven degrees of separation.” 3. Gene expression and chromatin/transcription factor data portals Fantom https://fantom.gsc.riken.jp/ ENCODE https://www.encodeproject.org/ The International Human Epigenome Consortium (IHEC) https://ihec- epigenomes.org/ Human Cell Atlas https://data.humancellatlas.org/ GTeX https://gtexportal.org/home/ PsychENCODE Consortium https://www.psychencode.org/ 3. Gene expression and chromatin/transcription factor data portals Fantom https://fantom.gsc.riken.jp/ ENCODE https://www.encodeproject.org/ The International Human Epigenome Consortium (IHEC) https://ihec- epigenomes.org/ Human Cell Atlas https://data.humancellatlas.org/ GTeX https://gtexportal.org/home/ PsychENCODE Consortium https://www.psychencode.org/ Fig. 1 Sample and data types in the GTEx v8 study. (A) Illustration of the 54 tissue types examined (including 11 distinct brain regions and two cell lines), with sample numbers from genotyped donors in parentheses and color coding indicated in the adjacent circles. Tissues with 70 or more samples were included in QTL analyses. (B) Illustration of the core data types used throughout the study. Gene expression and splicing were quantified from bulk RNA-seq of heterogeneous tissue samples, and local and distal genetic effects (cis-QTLs and trans-QTLs, respectively) were quantified across individuals for each tissue. Science 11 Sep 2020 Vol 369, Issue 6509 pp. 1318-1330 https://doi.org/10.1126/science.aaz1776 The ENCODE Project Consortium., Moore, J.E., Purcaro, M.J. et al. Expanded encyclopaedias of DNA elements in the human and mouse genomes. Nature 583, 699– 710 (2020). https://doi.org/10.1038/s41 586-020-2493-4 Fig. 1: ENCODE phase III data production. The ENCODE Project Consortium., Moore, J.E., Purcaro, M.J. et al. Expanded encyclopaedias of DNA elements in the human and mouse genomes. Nature 583, 699– 710 (2020). https://doi.org/10.1 038/s41586-020- 2493-4 Aviv Regev Human Cell Atlas Aviv Regev: https://www.genome.gov/Multimedia/Slides/GSPFuture2014/10_Regev.pdf PsychENCODE PsychENCODE Consortium https://www.psychencode.org/